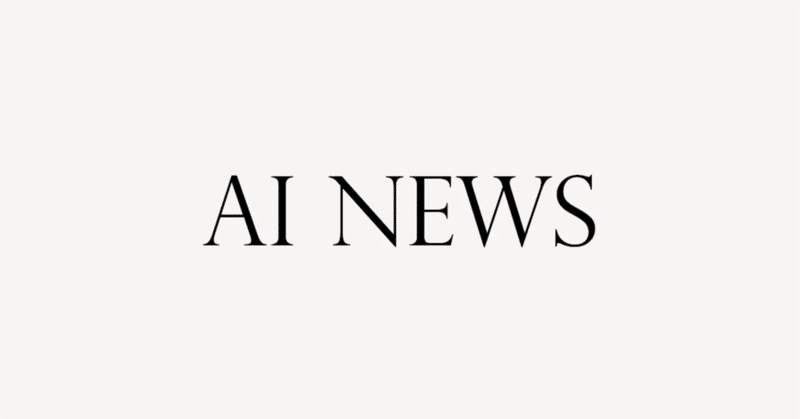
【Orca】英語解説を日本語で読む【2023年6月9日|@Matthew Berman】
人工知能の分野では、大規模な基礎モデルと小規模なオープンソースモデルの間で戦いが繰り広げられています。マイクロソフトリサーチは、「Orca」という研究論文を発表し、オープンソースモデルが単に回答を模倣するだけでなく、推論プロセスを理解することができることを示しています。Orcaは、ChatGPTやGPT-4などの大規模なモデルから詳細な説明やステップバイステップの思考プロセスを取り入れて、小規模なモデルを訓練します。Orcaは他のオープンソースモデルよりも優れたパフォーマンスを示し、さまざまなベンチマークでChatGPTと競争力のある結果を残します。論文ではまた、オープンソースモデルの現在の評価手法の制約についても議論されています。全体的に、ステップバイステップの説明から学ぶことがオープンソースモデルの能力を大幅に向上させ、独自の大規模言語モデルとの差を縮める可能性があるという研究結果が示されています。
公開日:2023年6月9日
※動画を再生してから読むのがオススメです。
There's a battle being waged right now in the world of artificial intelligence between large foundational models and smaller open source models, and just this week a new research paper was dropped that promises to append the conversation completely.
現在、大きな基盤モデルと小さなオープンソースモデルの間で人工知能の世界で戦いが繰り広げられており、今週新たな研究論文が公表され、完全に議論を一新すると約束されています。
Now, if you remember, a few weeks ago I made a video about the letter called We Have No Mode, which was a leaked internal memo from Google that really highlighted how open source models, smaller ones specifically, are iterating so quickly that these large foundational models that Google and OpenAI have are truly at risk.
数週間前、私は「We Have No Mode」という手紙についてビデオを作りました。これはGoogleから流出した内部メモで、オープンソースモデル、特に小規模なモデルがいかに素早く反復しているかを強調し、GoogleやOpenAIが持つ大規模な基盤モデルが本当に危険にさらされていることを示しています。
I found that to be a very compelling paper.
これは非常に説得力のある論文だと思いました。
And then, just two weeks ago, another research paper was released that claimed to disprove a lot of the value that these open source smaller models have.
ところが、つい2週間ほど前に、こうしたオープンソースの小規模モデルが持つ価値の多くを否定するような研究論文が発表されたのです。
Today, we're going to take a look at all of this and we're going to figure out what's the truth.
本日は、これらすべてを取り上げ、何が真実なのかを考えてみたいと思います。
We're going to take a look at the new Orca paper that was just dropped this week.
今週発表されたばかりの新しいオルカの論文を見てみます。
We're going to look at the We Have No Mode document again.
We Have No Modeのドキュメントをもう一度見てみる。
And we're going to take a look at the research paper that came out a couple weeks ago talking about the false promise of imitating proprietary large language models like GPT-4.
そして、数週間前に発表された、GPT-4のような独自の大規模言語モデルを模倣するという誤った約束についての研究論文も見ていきます。
Let's go.
さあ、行きましょう。
So, this is Orca: Progressive learning from complex explanation traces of GPT-4.
これが「Orca: Progressive learning from complex explanation traces of GPT-4」だそうです。
This is a new research paper dropped by Microsoft Research, of all companies.
これは、よりによってMicrosoft Researchが投下した新しい研究論文です。
Of course, they made a substantial investment in OpenAI and own a significant portion of that company.
もちろん、マイクロソフトはOpenAIに多額の投資をしており、その会社のかなりの部分を所有しています。
So, for them to release a new research paper illustrating a new technique to make open source smaller models extremely powerful is really fascinating.
ですから、オープンソースの小型モデルを非常に強力にするための新しい技術を示す新しい研究論文を発表したことは、本当に魅力的です。
Microsoft, as a company, has embraced open source in the years since Satya Nadella took over, and I'm all for it.
マイクロソフトは、サティア・ナデラ氏が就任して以来、企業としてオープンソースを受け入れており、私はそれに大賛成です。
This paper is absolutely fascinating and it makes a ton of sense.
この論文は実に魅力的で、筋が通っている。
But before we get into this paper, let's take a look at those previous documents that I mentioned.
しかし、この論文に入る前に、私が言及した以前の文書を見てみましょう。
Now, a little over a month ago, this internal memo from Google was released called We Have No Mode.
1ヶ月ちょっと前、Googleから「We Have No Mode」という内部メモが発表されました。
And the main point of this memo is that open source models are proliferating and iterating so quickly that the gap between models like GPT-4 and PaLM 2 are shrinking very quickly.
そしてこのメモの要点は、オープンソースモデルが増殖し、反復するスピードが速いため、GPT-4やPaLM 2のようなモデル間のギャップが非常に早く縮小しているということです。
The fact that any developer can get their hands on these models and new techniques to train and fine-tune these models are coming out every day.
開発者であれば誰でもこれらのモデルを手に入れることができ、これらのモデルを訓練し、微調整するための新しいテクニックが毎日のように出てきているということです。
And we're seeing that from Laura to Q, Laura to now having a ton of different options of how to train and fine-tune these models in really efficient ways and run them on any consumer-grade hardware.
ローラからQ、ローラから現在では、これらのモデルを実に効率的な方法で訓練し、微調整し、コンシューマーグレードのハードウェアで実行する方法について、多くの異なる選択肢があることを私たちは目の当たりにしています。
And I agreed with a lot that was in this paper.
私は、この論文に書かれている多くのことに同意しました。
Of course, a business mode is not just the technical limitations; there's much more to it than that.
もちろん、ビジネスモードは技術的な制約だけでなく、それ以上のものがあります。
But a lot of the points made in this paper are very valid, and I've seen more innovation in the open source community over these last few weeks than I've seen on these proprietary large models.
しかし、この論文で指摘されていることの多くは非常に妥当なものであり、私はこの数週間、オープンソースコミュニティにおいて、こうしたプロプライエタリな大型モデルで見た以上のイノベーションを目の当たりにしてきました。
But then, a research paper out of UC Berkeley was dropped a couple weeks ago that really challenged the assertions of the We Have No Mode document.
しかし、数週間前にカリフォルニア大学バークレー校から、「We Have No Mode」文書の主張を覆すような研究論文が発表されました。
In this research paper, The False Promise of Imitating Proprietary LLMs, they spell out that these open source models are simply just imitating the outputs of these larger models without actually understanding the logic to reach certain output.
この論文「The False Promise of Imitating Proprietary LLMs」では、オープンソースモデルが、ある出力に到達するための論理を実際に理解することなく、単に大型モデルの出力を模倣しているだけであることを明記しています。
The gist of this paper, and what Orca looks to correct, is that these open source models are simply being trained on prompts and responses, which is good for pattern matching.
この論文の要点とOrcaの修正点は、オープンソースのモデルは、単にプロンプトとレスポンスで訓練されており、パターンマッチングに適しているということです。
So, for example, if you're a student in college and you're taking a class, you could probably do pretty well on a lot of tests simply by pattern matching the question to an answer.
例えば、あなたが大学生で授業を受けている場合、質問と答えをパターンマッチさせるだけで、おそらく多くのテストでかなり良い結果を出すことができるでしょう。
But that student is going to have a lot of limitations if one of the questions varies from their pattern matching ability by even just a little bit.
しかし、ある問題が自分のパターンマッチングの能力と少しでも異なれば、その学生は多くの制限を受けることになります。
Their ability to reason and figure out what the answer might be becomes highly limited.
答えが何であるかを推理する能力が非常に制限されてしまうのです。
Whereas the student who fundamentally and deeply understands a topic won't be thrown off by any variation of the question.
一方、あるトピックを根本的に深く理解している学生は、問題のバリエーションに左右されることはないでしょう。
They'll be able to reason and step by step get to the answer because they do truly understand the topic.
そのトピックを本当に理解しているからこそ、推論し、ステップバイステップで答えを導き出すことができるのです。
And that's really the difference between these large foundational models and the open source imitations of them, as per this paper.
これが、この論文にあるような大規模な基礎モデルと、それを模倣したオープンソースの違いなのです。
And that brings us to Orca.
そして、Orcaにたどり着きました。
Orca challenges the idea that open source models can only really imitate answers and will get thrown off by any variation in the prompts themselves.
Orcaは、オープンソースのモデルは回答を模倣することしかできず、プロンプト自体のばらつきによって混乱するという考え方に挑戦しています。
And the way they do it seems very obvious in hindsight.
そして、その方法は、今にして思えば、非常に明白なものです。
Before we get into the details, Orca outperforms every other open source model and even outperforms ChatGPT, which is GPT-3.5, in a lot of different benchmarks.
詳細を説明する前に、Orcaは他のすべてのオープンソースモデルを凌駕し、多くの異なるベンチマークでGPT-3.5であるChatGPTを凌駕することさえあります。
Now, of course, it still lags behind GPT4, but the gap continues to close.
もちろん、GPT4にはまだ遅れをとっていますが、その差は縮まり続けています。
So let's take a look at this paper.
では、この論文を見てみましょう。
Now, they start off the abstract by addressing this imitation concept.
この論文では、まず、模倣の概念について触れています。
Recent research has focused on enhancing the capability of smaller models through imitation learning, drawing on the outputs generated by large foundational models (LFMs). Again, LFMs are referring to ChatGPT and GPT4.
最近の研究では、大規模な基礎モデル(LFM)が生成する出力を利用した模倣学習によって、小規模なモデルの能力を向上させることに焦点を当てている。ここでもLFMとは、ChatGPTとGPT4のことを指しています。
And they start to outline the limitations of these imitation techniques.
そして、これらの模倣手法の限界について概説し始める。
Some that they point out are limited imitation signals from shallow LFM outputs, small-scale homogeneous training data.
LFMの浅い出力から得られる模倣信号が限られていること、小規模で均質なトレーニングデータであることなどが指摘されています。
And most notably, a lack of rigorous evaluation, resulting in overestimating the small model's capability, as they tend to learn to imitate the style but not the reasoning process of LFMs.
また、最も注目すべきは、厳密な評価の欠如で、LFMの推論プロセスではなく、スタイルを模倣して学習する傾向があるため、小さなモデルの能力を過大評価する結果になっていることです。
That is really the crux of this paper - how do we start getting these open source models to not just mimic the question-answer pairs, but actually understand how they get from a question to an answer?
この論文では、オープンソースモデルに質問と答えのペアを模倣させるだけでなく、質問から答えに至る過程を実際に理解させるためにはどうすればいいのか、という点を中心に説明しています。
And only with that is true intelligence created.
そして、それがあって初めて、真のインテリジェンスが生まれるのです。
To address these challenges, we develop Orca, a 13 billion parameter model that learns to imitate the reasoning process of LFMs.
こうした課題に取り組むため、私たちは、LFMの推論プロセスを模倣するために学習する130億パラメータのモデル、Orcaを開発しました。
Let's pause there for a second.
そこで少し立ち止まりましょう。
This model, the Orca model, is only 13 billion parameters, which means it can run on pretty much any modern hardware.
このモデル「Orca」は、130億パラメータしかないため、最新のハードウェアで動作させることができます。
Whereas some of the other models that I've been reviewing recently, like the Guanaco model, require me to rent out a cloud GPU like an A6000 that has 48 gigabytes of VRAM because it's so large (65 billion parameters), and this performs better than that.
最近レビューしている他のモデル、例えばGuanacoモデルでは、あまりの大きさ(650億パラメータ)のために48ギガバイトのVRAMを持つA6000のようなクラウドGPUを借りなければならないのに対し、これはそれ以上のパフォーマンスを持っています。
Now, here's the key to the paper, here's the key technique: Orca learns from rich signals from GPT4, including explanation traces, step-by-step thought processes, and other complex instructions.
さて、ここがこの論文のキーポイントで、キーとなる技術です: オルカはGPT4からの豊富な信号から学習します。説明の痕跡、段階的な思考過程、その他の複雑な指示などです。
Guided by teacher assistants from ChatGPT.
ChatGPTからのティーチャーアシスタントが指導する。
Now, I'll explain what teacher assistance is in a little bit.
さて、ティーチャーアシスタントとは何か、もう少し説明します。
But looking at this sentence, what it's really saying is rather than learning from the prompt and response pairs, we're going to ask these large foundational models to explain their reasoning step by step.
しかし、この文章を見ると、プロンプトとレスポンスのペアから学ぶのではなく、大型の基礎モデルにステップバイステップで理由を説明するように求めるということがよくわかります。
And the smaller open-source models will learn from that.
そして、小さなオープンソースモデルがそこから学ぶのです。
Truly fascinating.
実に魅力的です。
Now I want to briefly touch on this guided by teacher assistance from ChatGPT.
さて、このChatGPTの教師支援による指導について簡単に触れておきたいと思います。
They have a two-tier teaching process.
彼らは2段階の教育プロセスを持っています。
One, they take ChatGPT, which is GPT-3.5, and they have a large number of examples to learn from (5 million). Then they take those 5 million and boil it down to the most important 1 million examples and then use GPT4 to continue to train on more complex examples.
一つ目に、彼らはChatGPTを取り、これはGPT-3.5で、学習するための大量の例(500万)を持っています。そして彼らはその500万を最も重要な100万の例に絞り込み、GPT4を使ってより複雑な例で訓練を続けます。
So, how does it actually perform?
では、実際の性能はどうなのか。
Orca surpasses conventional state-of-the-art instruction-tuned models such as Vicunia 13B by more than 100 percent in complex zero-shot reasoning benchmarks like Big Bench Hard and 42 on AGI eval.
Orcaは、Big Bench HardやAGI evalの42といった複雑なゼロショット推論ベンチマークにおいて、Vicunia 13Bのような従来の最先端命令チューニングモデルを100%以上上回る結果を出しています。
Big Bench Hard and AGI eval are just sets of tests that they give to these large language models to test their performance.
Big Bench HardとAGI evalは、これらの大規模言語モデルの性能をテストするために与えるテストのセットに過ぎません。
Orca reaches parity with ChatGPT on the BBH Benchmark and shows competitive performance in professional and academic examinations like SAT, LSAT, GRE, and GMAT, both in zero-shot setting without Chain of Thought.
OrcaはBBHベンチマークでChatGPTと同等になり、SAT、LSAT、GRE、GMATなどの専門試験や学術試験で、Chain of Thoughtなしのゼロショット設定で競争力を発揮しています。
While trailing behind GPT-4, and again, this last sentence is everything.
GPT-4の後塵を拝していますが、やはり、この最後の一文がすべてです。
Our research indicates that learning from step-by-step explanations, whether these are generated by humans or more advanced AI models, is a promising direction to improve model capabilities and skills.
私たちの研究によると、段階的な説明から学ぶことは、それが人間によって生成されたものであれ、より高度なAIモデルであれ、モデルの能力とスキルを向上させる有望な方向性であることがわかります。
And just like humans, large language models understanding how something works is much more effective than just being able to pattern match questions and answers.
そして、人間と同じように、大規模な言語モデルが何かの仕組みを理解することは、単に質問と答えをパターンマッチさせるよりもはるかに効果的です。
So, large language models are typically tuned by something called instruction tuning.
そこで、大規模言語モデルは、一般的にインストラクション・チューニングと呼ばれる方法でチューニングされます。
You have a set of prompts and you have a set of responses, and those pairs are passed to the open-source model and it learns from that.
プロンプトのセットと回答のセットがあり、それらのペアがオープンソースモデルに渡され、そこから学習されます。
This technique is called explanation tuning, where it's not just the prompt and the answer, but an explanation of the reasoning and the logic for how ChatGPT and GPT-4 arrived at an answer.
この手法は説明チューニングと呼ばれ、プロンプトと回答だけでなく、ChatGPTやGPT-4がどのようにして回答にたどり着いたのか、その理由や論理を説明するものです。
And so, we can see here when evaluated by GPT-4, and that's called auto-evaluation, Orca 13B actually beats ChatGPT, it beats Bard, and it certainly beats the open-source models based on LLaMA.
GPT-4で評価すると、自動評価と呼ばれるものですが、Orca 13BはChatGPTにもBardにも、そしてLLaMAに基づくオープンソースのモデルにも勝っていることがわかります。
And then for zero-shot problems on academic exams, ChatGPT definitely performs better, but Orca 13B is really closing the gap in performance and performs much better than Vicunia 13B.
そして、学力試験のゼロ点問題では、ChatGPTの方が確実に性能が高いのですが、Orca 13Bは本当に性能差を縮めていて、Vicunia 13Bよりもずっと良い成績を収めています。
And for complex zero-shot reasoning tasks in Big Bench Hard, Orca achieves parity with ChatGPT.
また、Big Bench Hardの複雑なゼロショット推論タスクでは、OrcaはChatGPTと同等を達成しています。
And here again, they specifically call out that imitation paper authors assert that model limitation is a false promise.
そしてここでも、模倣論文の著者がモデルの限定は偽りの約束であると主張していることを特に訴えています。
Since broadly matching ChatGPT using purely imitation would require one, a concerted effort to collect enormous imitation data sets, and far more diverse and higher-quality imitation data than is currently available.
ChatGPTを模倣だけで広くマッチングさせるには、1つには、膨大な模倣データセットを集める協調的な努力と、現在利用可能なものよりはるかに多様で高品質な模倣データが必要だからです。
So, one of the biggest problems is these open-source models can't get enough data to use the imitation technique and perform at the same rate as these large foundational models.
つまり、オープンソースのモデルは、模倣技術を使用するのに十分なデータを得ることができず、これらの大規模な基礎モデルと同じパフォーマンスを発揮することができないというのが最大の問題の1つです。
Contrary to this assertion, we demonstrate that both conditions one and two are attainable and that it is possible to reduce the gap with proprietary LLMs on multiple zero-shot benchmarks that require sophisticated reasoning.
この主張とは逆に、私たちは、条件1と条件2の両方が達成可能であり、高度な推論を必要とする複数のゼロショットベンチマークにおいて、独自のLLMとの差を縮めることが可能であることを実証します。
And here they touch on what the existing open-source models are doing currently to train themselves.
そしてここで、既存のオープンソースモデルが現在行っている自己学習について触れている。
Both Alpaca and WizardLM employ a variant of self-instruct.
AlpacaもWizardLMも、自己学習の変種を採用しています。
So, that's what we've been talking about.
つまり、そういうことなのだ。
WizardLM introduces the concept of EvoLinst and STRUCK, which gradually rewrites the initial set of instructions into more complex versions, attempting to overcome some of the methods inherent shortcomings.
WizardLMは、EvoLinstとSTRUCKの概念を導入し、最初の命令セットを徐々に複雑なバージョンに書き換えて、手法固有の欠点を克服しようと試みています。
But with Vicunia and Koala, they demonstrate remarkable performance due to the more human-like conversations and natural instructions in the community-contributed conversations, like those in shared GPT.
しかし、ビクーニャとコアラでは、GPTを共有するようなコミュニティに貢献された会話など、人間らしい会話と自然な指示により、驚くほどのパフォーマンスを発揮します。
So, basically, what they're saying is, as more people are using these open-source models and sharing their data, sharing their instructions, their prompts, and the output, they'll continue to train on those pairs and get better and better.
つまり、より多くの人がオープンソースのモデルを使い、データを共有し、指示やプロンプト、出力を共有することで、それらのペアでトレーニングを続け、どんどん良くなっていくということなのです。
But there's a limitation with that as well, and it's the same thing that we keep coming back to: models trained on such natural conversations may capture the style but not the reasoning process of the LLM.
しかし、それにも限界があり、それは我々が何度も戻ってくる同じ問題です:自然な会話で訓練されたモデルはスタイルを捉えるかもしれませんが、LLMの推理過程を捉えることはできません。
So again, they'll be able to pattern match, but they're not going to truly understand the logic and the reasoning behind arriving at the solutions.
つまり、パターンマッチはできても、解決策を導き出すまでのロジックや推論を真に理解することはできないのです。
Now, the Orca paper puts forth three key contributions.
さて、「オルカ」の論文では、3つの重要な貢献を提示しています。
Number one is explanation tuning.
1つ目は、「説明のチューニング」です。
And again, this is fine-tuning models based on the step-by-step explanation of the reasoning and the logic of how to arrive at a solution.
これは、解答に至る理由や論理を段階的に説明することで、モデルの微調整を行うものです。
Let's read this a little bit.
これを少し読んでみましょう。
We augment the query-response pairs with detailed responses from GPT4 that explain the reasoning process of the teacher as it generates the response.
クエリとレスポンスのペアをGPT4からの詳細なレスポンスで補強し、レスポンスを生成する際の教師の推論プロセスを説明しています。
And to get the step-by-step reasoning, they're using some of these more modern prompting techniques that we've been learning about, such as explain like I'm five, think step by step, and justify your response.
そして、段階的な推論を得るために、「5歳のように説明しなさい」「段階的に考えなさい」「反応を正当化しなさい」など、これまで学んできた、より現代的なプロンプトのテクニックを使っているのです。
This forces GPT4 to put forth its reasoning and its logic in the response itself, and that is used to train, and that's what explanation tuning is.
これにより、GPT4は回答そのものに理由や論理性を出さざるを得なくなり、それが訓練に使われる、それが説明チューニングなのです。
Another issue is scaling the amount of tasks and instructions.
もう一つの課題は、タスクや指示の量のスケーリングです。
As you'll see in a graph that I'll show in a second, a lot of these open-source models are using a highly limited data set.
これからお見せするグラフにあるように、オープンソースのモデルの多くは、非常に限られたデータセットしか使っていません。
But that's where Orca really excels.
しかし、オルカはその点で非常に優れています。
We utilize the Flan 2020 collection, and that's a data set of tasks and instructions put forth by Google that has tens of millions of instructions.
私たちはFlan 2020コレクションを利用していますが、これはGoogleが提供するタスクと命令のデータセットで、数千万個の命令があります。
So let's quickly take a look at the data sizes for these open-source models.
では、これらのオープンソースモデルのデータサイズを見てみましょう。
All of them have in the thousands.
いずれも数千単位です。
So you can see here that Alpaca has 52,000, Vicunia has 70,000, and WizardLM with the most has 250,000.
Alpacaが52,000、Vicuniaが70,000、そして最も多いWizardLMが250,000であることがわかりますね。
Based on the teacher of ChatGPT and some of these other ones like Dolly are human instructed, so they're even more limited because of the limitations of humans.
ChatGPTの先生をベースに、この他にもDollyなどは人間が指示したものですから、人間の限界でさらに制限されています。
However, as you can see here, Orca has 5 million, many times more than all of the other open-source models, and it's based on ChatGPT initially.
しかし、ここにあるように、Orcaは他のオープンソースモデルの何倍もの500万個を持っていて、最初はChatGPTがベースになっています。
So that's the initial 5 million pass, and then GPT-4 with a second pass of much more complex tasks and instructions.
これは最初の500万回のパスで、次にGPT-4で、より複雑なタスクや指示を2回目のパスで行うわけです。
So not only are they getting full explanations of query and responses and how they actually reach those responses, but they're getting so many more of them, and they're solving the data scaling issue.
そのため、クエリやレスポンス、実際にそのレスポンスに到達するまでの道のりを完全に説明するだけでなく、より多くのクエリを取得し、データのスケーリングの問題を解決しているのです。
Last is evaluation.
最後は評価です。
There are a lot of issues with current evaluation techniques for open-source models, but Orca claims to solve these in a few ways.
現在のオープンソースモデルの評価技術には多くの問題がありますが、Orcaはいくつかの方法でこれらを解決していると主張しています。
They use auto-evaluation with GPT-4, so basically asking GPT-4 between two potential responses which one is best.
GPT-4による自動評価で、基本的にはGPT-4に2つの回答候補の中からどれがベストかを尋ねます。
They also use academic benchmarks like Big Bench, Hard and Truthful QA, and professional and academic exams like the SAT, LSAT, etc.
また、Big Bench、Hard and Truthful QAなどのアカデミックベンチマークや、SAT、LSATなどの専門・学術試験も利用する。
And lastly, they use safety evaluation from toxic-gen, based really do these responses contain toxic language.
そして最後に、toxic-genの安全性評価を用いて、これらの回答が本当に有害な言葉を含んでいるかどうかを判断しています。
So in Figure 4, they illustrate what the previous techniques do with queries and responses.
図4では、これまでの技術がクエリとレスポンスで何をするのかが示されています。
So here's a user instruction: Use the data to calculate the median.
ここで、ユーザーからの指示を紹介します: データを使って中央値を計算してください。
Here it says, First, we need to arrange the data in ascending order.
まず、データを昇順に並べる必要があります。
Since there are five numbers, the median is in the middle, which is seven.
5つの数字があるので、中央値は真ん中の7になります。
And so this is very basic, it's a prompt, a query, and then the response.
このように、これは非常に基本的なもので、プロンプト、クエリ、そしてレスポンスとなります。
Whereas with their new method, they ask GPT-4 to explain.
一方、新しい方法では、GPT-4に説明を求めています。
So the system instruction seems to be the main tool that they use to get ChatGPT and GPT-4 to explain their reasoning.
つまり、システムインストラクションは、ChatGPTやGPT-4に推論を説明させるための主要なツールであるようです。
You are an AI assistant.
あなたはAIアシスタントです。
User will give you a task.
ユーザーはあなたにタスクを与えます。
Your goal is to complete the task as faithfully as you can while performing the task.
あなたの目標は、タスクを実行しながら、できるだけ忠実にタスクを完了させることです。
Think step by step and justify your steps.
ステップバイステップで考え、そのステップを正当化してください。
So again, use the given data to calculate the median.
では、もう一度、与えられたデータを使って中央値を計算してください。
Same prompt to calculate the median, I will follow these steps, and GPT-4 actually outlines step by step how it will figure out what the median is.
同じように中央値を計算するように促され、私はこのステップに従います。GPT-4は実際に、どのように中央値を割り出すかをステップバイステップで概説しています。
That data is then used to train the open source model.
このデータは、オープンソースのモデルのトレーニングに使用されます。
Now, I find it so fascinating that we're using some of these modern prompting techniques like Chain of Thought, like Explain Like I'm Five, that people have been figuring out over the last few months to get better answers from ChatGPT and GPT-4.
私は、思考の連鎖や「私にもわかるように説明して」といった最近数ヶ月で人々が解明してきた現代の提示手法を使って、ChatGPTやGPT-4からより良い回答を得ることに深い興味を持っています。
And we're using those to get better data to train the open source models with.
それを使って、オープンソースモデルを訓練するためのよりよいデータを得ています。
And as I mentioned, system messages seem to be the main tool to get ChatGPT and GPT-4 to provide the step-by-step explanations.
また、システムメッセージは、ChatGPTとGPT-4にステップバイステップの説明をさせるための主要なツールであることは述べたとおりです。
And if you play around with the ChatGPT playground or even the API, you'll know that the system messages are a requirement for using either of these tools.
そして、ChatGPTのプレイグラウンドや、APIを弄れば、システムメッセージがこれらのツールのいずれかを使うための必要条件であることがわかると思います。
So, here are a few examples.
そこで、いくつかの例を紹介します。
You will be given a task.
あなたは、あるタスクを与えられます。
You must generate a detailed and long answer.
あなたは詳細で長い答えを生成する必要があります。
Think like you are answering to a five-year-old.
5歳児に答えているように考えてください。
Help as much as you can.
できる限り助けてあげてください。
So, it's really just coaxing ChatGPT-4 to explain its reasoning and to be as verbose as possible.
つまり、ChatGPT-4が理由を説明し、できるだけ饒舌になるように、本当になだめすかしているだけなのです。
So, let's actually take a look at the difference between these two prompting techniques.
では、実際にこの2つのプロンプト手法の違いを見てみましょう。
So, for the system message, you are an AI assistant that helps people find information.
システムメッセージの場合、あなたは情報を探す手助けをするAIアシスタントということになりますね。
User will give you a question.
ユーザーはあなたに質問を投げかけます。
Your task is to answer as faithfully as you can while answering things step by step and justify your answer.
あなたのタスクは、物事をステップバイステップで答えながら、できるだけ忠実に答え、自分の答えを正当化することです。
So, the prompt is: Pick which sentence is not logical.
そこで、プロンプトが表示されます: どの文章が論理的でないかを選んでください。
Sentence A: People in the desert often look forward to flood.
文A:砂漠の人々はしばしば洪水を待ち望んでいる。
Sentence B: People in the desert often look forward to rain.
文B: 砂漠の人々は、しばしば雨を楽しみにしている。
Now, it might seem obvious to us why these sentences are different, but there's really just a subtle difference between them.
さて、なぜこれらの文が違うのか、私たちには明白に思えるかもしれませんが、実際には微妙な違いがあるだけなのです。
So, here a typical response might be: There is no reason to look forward to a flood because floods cause damage.
ですから、ここで典型的な回答はこうなるかもしれません: 洪水は被害をもたらすので、洪水を楽しみにする理由はない。
The answer is Sentence A. ChatGPT says, Okay, let's break it down.
答えは文Aです。ChatGPTは、「よし、分解してみよう。
Read the sentences carefully.
文章をよく読んでみてください。
So, let's talk about what steps am I going to take to answer this.
では、これに答えるために、私はどのような手順を踏めばいいのか、話してみましょう。
Identify the differences between them and determine which sentence is not logical.
両者の違いを確認し、どの文が論理的でないかを判断する。
Then they say, Sentence A: People in the desert often look forward to the flood.
そして、文A:People in the desert often look forward to the flood.と言います。
This sentence suggests that people in the desert are eagerly anticipating a flood.
この文は、砂漠の人々が洪水を心待ちにしていることを示唆している。
However, floods are typically destructive and dangerous.
しかし、洪水は通常、破壊的で危険なものです。
So, it seems unlikely that people would look forward to them.
だから、人々が洪水を楽しみにすることはありそうもない。
Sentence B: They're looking forward to the rain.
文B.彼らは雨を心待ちにしている。
And it says, This sentence suggests that people in the desert are eagerly anticipating rain.
と書かれており、この文章は、砂漠の人々が雨を心待ちにしていることを示唆しています。
While the desert is a dry environment, rain can be beneficial, providing water for plants and animals.
砂漠は乾燥した環境ですが、雨は植物や動物に水を供給する有益なものです。
Then it finishes with, Based on the above analysis, it can be concluded that Sentence A is not logical.
そして、「以上の分析に基づき、文Aは論理的でないと結論づけられる」と締めくくられています。
Then we look at GPT-4's answer, and as you can see, I won't read all of it, it's a much more detailed and verbose answer.
次にGPT-4の解答を見ますが、ご覧の通り、全部は読みませんが、もっと詳細で冗長な解答になっています。
Now, in this section, they talk about why ChatGPT as a teaching assistant, assistant to GPT-4, is such a powerful method.
さて、このセクションでは、GPT-4のアシスタントであるChatGPTが、なぜこれほど強力な手法なのかについて述べられています。
And there's really two reasons for it.
その理由は、実に2つあります。
One is a capacity gap, because there's such a large gap between the ORCA model and GPT-4.
ひとつは、ORCAモデルとGPT-4の間には大きな隔たりがあるため、キャパシティギャップがあることです。
Being able to take data from GPT-4 and passing it directly into ORCA, it's going to struggle with imitation.
GPT-4のデータをそのままORCAに渡すと、模倣に苦労することになります。
Whereas if they progressively teach it to get to the GPT-4 level by the intermediate step of ChatGPT, it really performs much better.
しかし、ChatGPTの中間段階であるGPT-4レベルまで段階的に教えることで、より高いパフォーマンスを発揮することができます。
This can be viewed as a form of progressive learning or curriculum learning, where the student first learns from easier examples, followed by harder ones again, more and more human-like behavior.
これは、漸進的学習やカリキュラム学習の一種と見ることができ、まず簡単な例から学び、次にまた難しい例から学ぶという、より人間に近い行動をとることができます。
A human doesn't go from learning the basics of addition all the way to calculus in one step; they learn many incrementally more difficult steps of mathematics between addition and calculus.
人間は、足し算の基本から微積分まで一気に学ぶのではなく、足し算から微積分までの間に、より難しい数学を何段階も段階的に学んでいくのです。
Next is a simple pragmatic reason: cost and time.
次に、コストと時間というシンプルな現実的理由です。
ChatGPT, specifically GPT-3.5 Turbo, is much faster and much less expensive than GPT4.
ChatGPT、特にGPT-3.5 Turboは、GPT4よりもはるかに速く、はるかに安価です。
So that's why they use 5 million examples with ChatGPT and 1 million examples for GPT4.
ChatGPTで500万例、GPT4で100万例を使うのはそのためです。
This graphic shows the performance of these large foundational models: Vicunya and Orca.
このグラフィックは、これらの大規模な基礎モデルの性能を示しています: VicunyaとOrcaです。
And as we can clearly see from questions from the LSAT and the SAT, Orca performs significantly better than Vicunya.
そして、LSATやSATの問題から明らかにわかるように、OrcaはVicunyaよりもかなり良いパフォーマンスをしています。
And if we look at the Orca column compared to the ChatGPT column overall, it performs quite similarly but it still does lag behind GPT4.
また、Orcaの列をChatGPTの列と比較すると、全体的にはよく似ていますが、GPT4にはまだ遅れをとっています。
And they've actually shown that this progressive learning technique really works.
そして、この漸進的な学習手法が本当に有効であることを、実際に示しています。
As we can see here, using only GPT4, they were able to achieve a score of 37.18.
このように、GPT4だけを使って、37.18というスコアを達成することができました。
Whereas if they use that intermediate step of ChatGPT, they were able to achieve 41.7.
一方、中間のChatGPTを使うと、41.7というスコアになりました。
That might seem small, but that is a significant improvement.
これは小さいと思うかもしれませんが、大きな進歩です。
And for the big-bench hard results, Orca performs marginally better than ChatGPT on aggregate across all tasks, significantly lags GPT4, and outperforms Vicunya by 113 percent.
また、ビッグベンチのハード結果では、OrcaはChatGPTよりも全タスクで僅かに優れていますが、GPT4には大きく遅れをとり、Vicunyaには113%の差をつけています。
Here they give a graphic of the zero-shot performance against all of these different tasks, and you can see that Orca performs substantially better than Vicunya and even across all of them, like it said, it performs better than ChatGPT.
ここでは、これらの異なるタスクすべてに対するゼロショット性能のグラフを示しますが、OrcaはVicunyaよりも大幅に性能が良く、すべてのタスクにわたっても、前述のようにChatGPTより性能が良いことがわかります。
So, what does all this mean?
では、このことは何を意味するのでしょうか?
I find it fascinating for two reasons.
私は、2つの理由から興味深いものだと感じています。
One, open-source models continue to get better at a rapid clip.
1つは、オープンソースのモデルは急速に良くなり続けていることです。
New techniques for fine-tuning training are coming out every single day.
トレーニングを微調整するための新しいテクニックが、毎日のように出てきているのです。
So, I think back to that We Have No Mode paper, and it makes a lot of sense still.
ですから、「We Have No Mode」の論文を思い返すと、今でも多くの意味があることがわかります。
I also find it fascinating that GPT4 still seems to have some secret sauce and performs much better than any other model.
また、GPT4にはまだ何か秘密のソースがあるようで、他のどのモデルよりもはるかに優れたパフォーマンスを発揮しているのも魅力的です。
So, OpenAI seems to have plenty of mode.
つまり、OpenAIにはモードがたくさんあるようです。
This moat seems to be incrementally getting decreased, but they still do have it.
この堀は徐々に小さくなっているようですが、それでもまだ持っています。
The last thing that I find fascinating is that this paper is by Microsoft Research.
最後に、この論文はマイクロソフト・リサーチによるものであることが魅力的です。
Microsoft Research owns a significant portion of OpenAI.
マイクロソフト・リサーチは、OpenAIのかなりの部分を所有しています。
So, the fact that they're making research gains in open source is awesome, and OpenAI really must feel that they have a significant moat to work with.
つまり、オープンソースで研究成果を上げているという事実はすごいことで、OpenAIは本当に重要な堀を持っていると感じているはずです。
And OpenAI also mentioned a week ago that they're releasing their own open-source model.
また、OpenAIは1週間前に、自分たちのオープンソースモデルをリリースすると言っています。
So, I think what all of this means is that these large language models will continue to get better and cheaper over time.
つまり、このような大規模な言語モデルは、時間の経過とともに、より良く、より安くなり続けるということだと思うのです。
Now, Orca's code and dataset are not yet released, but as soon as it is, we're going to review it.
さて、Orcaのコードとデータセットはまだ公開されていませんが、公開され次第、私たちはそれをレビューするつもりです。
I'm going to show you how to use it, and we'll see how it performs.
使い方を紹介し、どのようなパフォーマンスを発揮するのか見ていきたいと思います。
If you like this video, please consider giving me a like and subscribe, and I'll see you in the next one.
このビデオが気に入ったら、ぜひ「いいね!」と「購読」をご検討ください!また次のビデオでお会いしましょう。
この記事が気に入ったらサポートをしてみませんか?