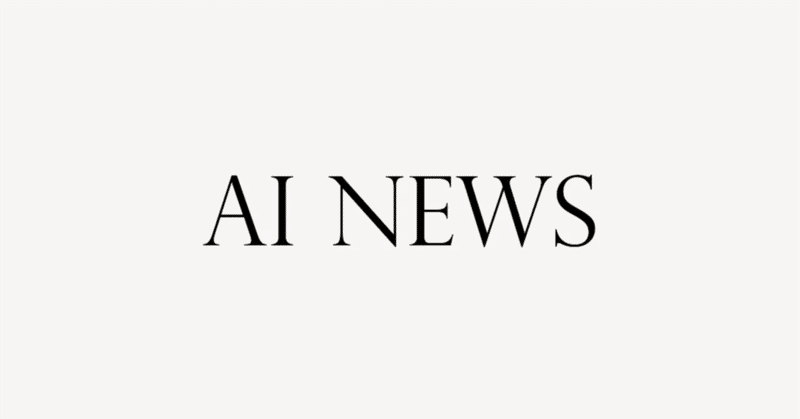
【Grok 1.5 Vision:GPT-4、Claude、Geminiを凌駕する革新的パフォーマンスを披露】英語解説を日本語で読む【2024年4月13日|@Wes Roth】
2024年4月12日、新しい多モードAIモデル「Grok 1.5 Vision」が発表され、デジタルと物理世界の接続が実現しました。このモデルは、テキスト処理に加え、文書、図表、スクリーンショット、写真などの視覚情報も処理できます。Grok 1.5は、他の最先端モデル、例えばGPT-4 VisionやClaude 3 Opus、Gemini Pro 1.5との比較で、非常に優れた性能を示しました。特に、物理世界を理解する能力が高く評価されています。また、新しいリアルワールドQ&Aベンチマークでは、実世界の空間理解を測定し、その結果Grokは競合他社を上回る性能を発揮しました。このモデルは間もなく初期テスターや既存のGrokユーザーに提供される予定です。
公開日:2024年4月13日
※動画を再生してから読むのがオススメです。
I did not see this coming.
これは予想していませんでした。
Grok 1.5 Vision preview.
Grok 1.5 Visionのプレビューを理解する。
Xai drops this new announcement.
Xaiがこの新しい発表を行います。
4.7 million views in a matter of hours, and it's shockingly good.
数時間で470万回の視聴回数があり、驚くほど良いです。
In a head-to-head comparison between Grok 1.5 Vision, GPT-4 Vision, Claude 3 Opus, and Gemini Pro 1.5, the latest release, Grok holds its own.
Grok 1.5 Vision、GPT-4 Vision、Claude 3 Opus、そして最新版のGemini Pro 1.5との比較で、Grokは健闘しています。
It holds its own against the Titans.
それはTitansに対抗しています。
I'm going to say it. I'm shocked.
言わせてもらいます。驚いています。
This seems big enough forward to cover this.
これはこれをカバーするのに十分大きそうです。
April 12th, 2024, Grok 1.5 Vision preview connecting the digital and physical worlds with our first multimodal model.
2024年4月12日、Grok 1.5 Visionプレビューは、最初のマルチモーダルモデルでデジタルと物理世界をつなぎます。
In addition to its strong text capabilities, groi now processes a wide variety of visual information like documents, diagrams, charts, screenshots, and photographs.
その強力なテキスト機能に加えて、groiは今や文書、図表、チャート、スクリーンショット、写真など、さまざまなビジュアル情報を処理します。
It will be available soon to our early testers and existing Grok users.
私たちの早期テスターや既存のGrokユーザーには近々利用可能になります。
Grok 1.5 is competitive with existing Frontier multimodal models in a number of domains.
Grok 1.5は、いくつかの領域で既存のFrontier multimodalモデルと競争力があります。
We are particularly excited about Grok's capabilities in understanding our physical world.
私たちは、Grokが私たちの物理世界を理解する能力に特に興奮しています。
Grok was built as an AI that was truth-seeking.
Grokは真実を求めるAIとして構築されました。
I forget the exact terminology Elon used, but it was supposed to understand the truth of the universe.
イーロンが使用した正確な用語を忘れてしまいましたが、それは宇宙の真実を理解することが目的でした。
It's interesting that they're pointing this out here.
彼らがここでこれを指摘しているのは興味深いです。
They're excited about Grok's capabilities and understanding the physical world.
彼らは、Grokの能力と物理世界を理解することに興奮しています。
Grok outperforms its peers in our new real-world Q&A Benchmark that measures real-world spatial understanding.
Grokは、実世界の空間理解を測定する新しい実世界Q&Aベンチマークで、同僚を凌駕しています。
For all data sets below, we evaluate Grok in a zero-shot setting without Chain of Thought prompting.
以下のすべてのデータセットについて、私たちはChain of ThoughtのプロンプトなしでGrokをゼロショット設定で評価します。
This is interesting because everybody kind of has their own little tricks, their own little shenanigans for showcasing the best of their model.
これは興味深いですね、なぜならみんなが自分自身の小さなトリックや、自分自身のモデルの最高を示すための小さないたずらを持っているからです。
For example, yesterday when OpenAI showcased their new and improved GPT-4 model, they also open-sourced a sort of set of benchmarks to evaluate the different models.
例えば、昨日OpenAIが新しく改良されたGPT-4モデルを披露したとき、彼らは異なるモデルを評価するためのベンチマークの一種をオープンソース化しました。
They're calling it a lightweight library for evaluating language models, and they are emphasizing zero-shot Chain of Thought setting, meaning they don't give it examples of How to solve the problems, and they're asking it to think through the problem step by step before solving it.
彼らは言語モデルを評価するための軽量ライブラリと呼んでおり、ゼロショットのChain of Thought設定を強調しています。つまり、問題の解決方法の例を与えず、問題をステップバイステップで考えさせてから解決するように求めています。
It's interesting here that Grok is saying for all data sets below, we evaluate Grok in a zero-shot setting.
ここで興味深いのは、Grokが以下のすべてのデータセットについて、私たちはGrokをゼロショット設定で評価していると言っていることです。
Again, same as OpenAI, but they're saying without Chain of Thought prompting.
再び、OpenAIと同じですが、Chain of Thoughtのプロンプトなしと言っています。
And here are the benchmarks.
そして、ここにベンチマークがあります。
First of all, GPT-4 with vision.
まず最初に、GPT-4 with visionです。
This was the reigning king for a long, long time.
これは長い間君臨していた王者でした。
CLA 3 Opus, when it came out, kind of rocked the rankings quite a bit.
CLA 3 Opusは登場したとき、ランキングをかなり揺るがしました。
It became the number one model on the LLM Arena, and we've tested it on this channel quite a bit.
それはLLMアリーナでナンバーワンのモデルになり、このチャンネルでかなりテストしてきました。
It's good.
良いです。
It's eerily good.
非常に良いです。
There's definitely stuff happening there that's very interesting.
そこで非常に興味深いことが起こっているのは間違いありません。
It was definitely a step forward.
それは間違いなく前進でした。
Gemini Pro 1.5 is also extremely good.
Gemini Pro 1.5も非常に優れています。
It's a big leap over Gemini 1.0.
Gemini 1.0よりも大幅に進化しています。
We believe that this is where they introduced mixture of experts.
私たちは、これが専門家の混合を導入した場所だと信じています。
They introduced the 1 million token context window.
彼らは100万トークンのコンテキストウィンドウを導入しました。
And then the claw 3 Sonet, which is a smaller CLA three model.
そして、小さなCLA3モデルであるクロー3ソネットも導入されました。
It's interesting that they put this in here, but the point is these three: the GPT-4 Vision, CLA 3 Opus, and Gemini Pro 1.5.
これをここに置いたことは興味深いですが、ポイントはこれら3つです:GPT-4 Vision、CLA 3 Opus、Gemini Pro 1.5。
I mean, these are the reigning Champions, these are the really good models, all with their own separate strengths.
これらは現在のチャンピオンです、これらは本当に優れたモデルです、それぞれが独自の強みを持っています。
The fact that Grok caught up this quickly is really interesting.
Grokがこれほど速く追いついたという事実は本当に興味深いです。
And let's come back here in just a second to go through exactly what it's doing good at because this is, I mean, this seems like kind of a big deal, doesn't it?
そして、ここに戻って、これが何に優れているかをまさに見ていきましょう、これは、つまり、これはかなり重要なことのように思えませんか?
Here they're giving a few examples of what it's good at.
ここでは、それが得意なことのいくつかの例を示しています。
For example, writing code from a diagram.
例えば、図表からコードを書くことです。
On the left here we have a flowchart: start, create a ROM number, read it.
こちらの左側にはフローチャートがあります:開始、ROM番号を作成、読み取ります。
I guess you're trying to guess a random number that is generated.
おそらく、生成されたランダムな番号を推測しようとしているのでしょう。
The user is asking, can you translate this into python code?
ユーザーは、「これをPythonコードに変換できますか?」と尋ねています。
They drew up a little diagram on the board, took a picture of it, and say, hey, make this into a python code into an actual software program.
彼らはボードに少しの図を描き、それを写真に撮り、「ねえ、これをPythonコードにして実際のソフトウェアプログラムにしてください」と言いました。
Grok answers, certainly, the flowchart you're provided describes a simple guessing game where the computer generates a random number and the user has to guess it.
おそらく、提供されたフローチャートは、コンピュータがランダムな数字を生成し、ユーザーがそれを推測しなければならない単純な推測ゲームを説明しています。
Here's the python code that represents the logic in the flowcharts.
こちらが、そのフローチャートのロジックを表すPythonコードです。
It generates that and as far as you can tell, near perfectly gets all the writing in like U1 and the wrong guest, try again.
それはそれを生成し、あなたが言うように、U1のようなすべての書き込みをほぼ完璧に取得し、間違ったゲストを再試行します。
It creates software from these little diagrams that you doodle on the Whiteboard.
それは、あなたがホワイトボードに落書きするこれらの小さな図からソフトウェアを作成します。
Next, we have calculating calories from the back of the Cardon nutrition facts.
次に、カルドン栄養成分の裏側からカロリーを計算します。
How many calories are in five slices of this kind of a tough question since a serving size is three slices.
この種の5枚のスライスには何カロリー含まれていますか。1回の摂取量が3枚の場合、これは少し難しい質問です。
You got to do some math there.
そこで少し数学をしなければなりません。
Grok calculates it that five slices will have 100 calories.
Grokは、5枚のスライスに100カロリー含まれると計算します。
I did the math on this, it checks out, but real fast, this is kind of a hard thing to do.
私はこれについて数学をしましたが、それは確認されますが、実際には、これはやるのが難しいことです。
A lot of other Vision mods we tested, they easily get confused with stuff like this if there's a lot of lines and you have the three slices and then you have the parentheses And 18 Gams and then this tends to trip them up.
私たちがテストした他の多くのビジョンモデルは、このようなものに簡単に混乱し、たくさんの線があって、3枚のスライスがあり、それから括弧があり、18ガムがあり、そしてこれは彼らを混乱させる傾向があります。
This is, this is impressive.
これは、これは印象的です。
They were saying my son Drew this, can you tell me a short bedtime story based on his drawing?
彼らは私の息子のドリューがこれを描いたと言っていました。彼の絵に基づいた短い寝前話を教えてくれますか?
And sure enough, Grok provides a story, very, very cool.
そして確かに、グロックはとてもクールなストーリーを提供してくれます。
Explaining a meme, so Grok better be good at this.
ミームを説明するので、グロックはこれに長けているはずです。
If Elon, the meme poster on X on Twitter, if he can't produce an AI that is excellent at explaining memes, then what is even the point?
ElonがTwitterのXでミームを投稿する人で、彼が優れたミームを説明するAIを生み出せないのであれば、それは一体何の意味があるのでしょうか?
It has to be a true meme scholar.
それは真のミームの学者でなければなりません。
Startups, you have this image and big companies, you have this image.
スタートアップにはこのイメージがあり、大企業にはこのイメージがあります。
The user says, I don't get it, please explain.
ユーザーが言う、「わからない、説明してください」と。
He describes that startup, everyone's actively participating, big companies only one person is actually digging the hole.
彼はそのスタートアップを説明し、誰もが積極的に参加しているが、大企業では実際に穴を掘っているのは1人だけだと述べています。
And the humorous image comes from the exaggeration of the differences between startups and big companies.
そして、ユーモラスなイメージは、スタートアップと大企業の違いの誇張から来ています。
And then explains further pretty much Nails it.
それから、さらに説明して、かなりうまくやっている。
Then we have a picture of what potentially could be a wiki Wikipedia, and the user saying, please convert this table to a CSV, right?
それから、潜在的にウィキペディアのようなものがある画像があり、ユーザーが「この表をCSVに変換してください」と言っていますね。
You comma separate values like an Excel spreadsheet or something similar.
Excelスプレッドシートなどと同様に、コンマで値を区切ります。
The table and the image can be translated to that format as follows, and it does it including the um blanking and what this is called, the headers, the column headers.
その表と画像は、次の形式に変換できます。それは、ヘッダー、列のヘッダーを含む、それを行い、それがそれを行います。
This is also something that I've seen other models struggle with a little bit.
これは、他のモデルが少し苦労しているのを見たこともあります。
Next, they're showing an image of some damage around a wooden plank with a nail, and the user asking, these holes are appearing around the screws in my deck, is it rotten?
次に、釘の周りにある木製の板の損傷の画像が表示され、ユーザーが尋ねています。「デッキのネジ周りに穴が現れていますが、腐っていますか?」
Should I get my boards replaced?
私はボードを交換すべきですか?
And Grok says that yes, it appears that your deck has started to rot.
そして、Grokは「はい、デッキが腐り始めているようですね。」と言います。
This is indicated by the presence of holes around the screws, which is a common sign of wood Decay, and they give you some more advice about that.
これは、ネジ周りに穴があることで示され、これは木材の腐敗の一般的な兆候です。そして、それについてさらなるアドバイスをしてくれます。
This is one thing where I was very impressed with GPT-4 with vision because for like quality assurance purposes, you would be able to show pictures of things that were Broken, like bolts that were stripped or cars with Nicks and some damage on them scratches, and you would just be able to say what's wrong in this image, and it would kind Of tell you, it would assess the damage.
これは、GPT-4 with visionに非常に感銘を受けた点の1つで、品質保証の目的で、破損したものの写真を示すことができ、ボルトが剥がれたり、傷や傷がついた車などがある写真を見せて、「この画像で何が問題か」と尋ねることができ、それがある種の評価をしてくれる、という点です。
Here the fact that it's able to Figure out if there's rot, that there's wood Decay, that's certainly extremely extremely useful.
それが腐敗があるかどうか、木材の腐食があるかどうかを判断できるという事実は、確かに非常に非常に役立つものです。
And then next we have a solving a coding problem, we're asking, can you write python code to solve this?
そして次に、コーディングの問題を解決することになりますが、これを解決するためのPythonコードを書けますか?
And it appears like a a semi complicated problem, not one that's easily understood at first glance, but Grok is able to write the code to solve it.
そして、それは半ば複雑な問題のように見えますが、一目で理解しにくいものではありませんが、Grokはそれを解決するためのコードを書くことができます。
If this is representative of what we will receive when we get Grok of our results, then this would be very very impressive real world understanding.
これがGrokの結果を受け取ったときに受け取るものの代表であるならば、それは非常に印象的な現実世界の理解であるでしょう。
In order to develop useful real world AI assistance, it is crucial to advance the model's understanding of the physical world.
有用な現実世界のAI支援を開発するためには、物理世界の理解を進化させることが重要です。
And of course, some of the same people behind all these companies are some of the same people behind Tesla.
そしてもちろん、これらの企業の背後にいる一部の人々は、テスラの背後にいる同じ人々です。
Tesla likely has the world's largest collection of various footage of cars being driven through various conditions, various roads, etc.
テスラはおそらく、さまざまな条件、さまざまな道路などを通って車が運転されるさまざまな映像の世界最大のコレクションを持っているでしょう。
Certainly this idea of real world understanding is important.
確かに、現実世界の理解という考えは重要です。
They're saying towards this goal, we are introducing a new Benchmark real world QA.
彼らはこの目標に向けて、新しいベンチマークの現実世界のQAを導入していると言っています。
We'll come back to this, but looks like they've introduced their very own real world QA, real world understanding Benchmark.
これについて後で戻ってきますが、彼らは自分たちの非常に独自の現実世界のQA、現実世界の理解のベンチマークを導入したようです。
And as you may have expected, they are whooping everybody else's neural Nets at that Benchmark.
期待通り、彼らはそのベンチマークで他の誰よりも優れた成績を収めています。
And this Benchmark is designed to evaluate basic real world spatial understanding capabilities of multimodal models.
そして、このベンチマークは、マルチモーダルモデルの基本的な現実世界の空間理解能力を評価するために設計されています。
While many of the examples in the current Benchmark are relatively easy for humans, they often pose a challenge for Frontier models.
現在のベンチマークの多くの例は人間にとって比較的簡単ですが、フロンティアモデルにとってはしばしば課題を提起します。
For example, in this image, which object is larger, the pizza cutter or the scissors?
例えば、この画像では、ピザカッターとハサミのどちらの方が大きいですか?
And it selects that se, they're about the same size.
そして、それを選択すると、ほぼ同じサイズです。
It's somewhat difficult because the scissors, it's obstructed right, it's hidden behind multiple objects.
ハサミはやや難しいですね、障害物に隠れていて、複数のオブジェクトの後ろに隠れています。
Next we have a traffic situation, where can we go from the current Lane?
次に、交通状況がありますが、現在のレーンからどこに行けますか?
Well, turn left.
左に曲がってください。
I mean, really the only hint is the sign, right?
つまり、本当に唯一のヒントはサインですよね?
You do have the arrow here, but just visually it almost seems like you could go forward.
ここに矢印がありますが、視覚的には前に進めるように見えますね。
I guess it seems like the only hint as to what you can do is the sign, and it correctly picks up on that.
一応、できることの唯一のヒントはサインだと思いますが、それを正しく捉えています。
And also indeed understands that it is in the leftmost lane because you really really can't see the road that well given this front camera view from our sedan.
そして、実際には、このセダンの前方カメラの映像からは道路があまりよく見えないので、左側のレーンにいることを理解しています。
Do we have enough space to drive around the gray car in front of us?
私たちの前にいる灰色の車を避けるためのスペースは十分ですか?
And answer is yes.
その答えは「はい」です。
Given the picture, in which cardinal direction is the dinosaur facing?
写真を見ると、恐竜はどの方向を向いていますか?
And let me see if I could do this, it's hard to see these okay, I see it.
これをやってみましょう、これらは見にくいですが、見えます。
East, the dinosaur is facing, yeah, roughly East, and so Grok chooses East.
恐竜は東を向いています、そう、おおよそ東を向いているので、グロックは東を選択します。
I do feel like other models would definitely have a hard time with this one.
他のモデルでは、これには確かに難しいと感じます。
The initial release of the real world Q&A consists of over 700 images with a question and easily verifiable answer for each image.
実世界のQ&Aの最初のリリースには、各画像についての質問と簡単に検証可能な回答が付いた700以上の画像が含まれています。
The data set consists of anonymous images taken from vehicles in addition to other real world images, and they are releasing it under the Creative Commons.
データセットには、車両から取られた匿名の画像に加えて、他の実世界の画像が含まれており、それらはクリエイティブ・コモンズの下で公開されています。
You can download it here.
こちらからダウンロードすることができます。
This is kind of a flex by Elon because I mean they do have a lot of data on the various driving around cars and freeways and such.
これは、エロンによるちょっとしたフレックスですね、なぜなら彼らはさまざまな車や高速道路を走るデータをたくさん持っているからです。
Certainly we can expect them to handsomely beat the other models at those particular tasks.
確かに、それらの特定のタスクで他のモデルを大幅に上回ることが期待されます。
And they continue into the future advancing both our multimodal understanding and generation capabilities are important steps in building beneficial AGI that can understand The universe.
そして、将来に向けて、私たちのマルチモーダルな理解と生成能力を進化させることは、有益なAGIを構築する上で重要なステップです。
In the coming months, we anticipate to make significant improvements in both capabilities across various modalities such as images, audio, and video, and they are hiring.
今後数ヶ月で、画像、音声、ビデオなどさまざまなモダリティにおける両方の能力を大幅に向上させることを期待しています。そして、彼らは採用しています。
Here's by the way the collection of images from that Creative Commons data set, the 700 and some images that they've used in that benchmark Mark.
ところで、こちらがCreative Commonsのデータセットからの画像コレクションです。彼らがそのベンチマークマークで使用した700枚以上の画像です。
I mean here are some of those images, there's a lot of cars and May dog, but yeah, they're probably like 90 95% images from cars driving around streets, a lot of it is San Francisco or the bay area as well as other sort of random images.
というわけで、これらはその画像の一部です。たくさんの車や犬がいますが、そうですね、おそらく90〜95%は車が通り過ぎる画像です。サンフランシスコやベイエリアの画像が多いですし、その他のランダムな画像もあります。
Grok takes the number one spot for that, the real world QA with Gemini Pro 1.5 a close second, then GPT-4 Vision followed by the Claude models Claude 3.
それに続いて、Grokがその第1位を獲得し、Gemini Pro 1.5に続いてGPT-4 Vision、そしてClaudeモデルのClaude 3が続きます。
Next we have the mmm U that is a benchmark that's designed to measure perception knowledge and reasoning in Large Language Models.
次に、知識と推論を測定するために設計されたベンチマークであるmmm Uがあります。
A lot of the questions looks like they have a l picture and they're supposed to Figure out what the graph is showing or select some data from the graph music notes Etc.
多くの質問は、グラフを示す画像があり、そのグラフが何を示しているかを見極めたり、グラフからデータを選択したりするようです。
Clot 3 did the best followed by Gemini Pro 1.5 then by GPT.
Clot 3が最も優れており、次にGemini Pro 1.5、そしてGPTが続きます。
And then Gro, but there's not an ocean of difference.
そしてGroが続きますが、大きな違いはありません。
They're not that far apart.
それらはそれほど遠く離れていません。
Next, we have math Vista.
次に、数学ビスタがあります。
These are visual and mathematical reasoning tests, puzzle tests, etc.
これらは視覚的および数学的推論テスト、パズルテストなどです。
If I'm reading this correctly, the leaderboards on math Vista on their website show a human performance is around 60, and then Gro has 52.8 and is higher than every single Other one.
これを正しく読んでいるなら、数学ビスタのウェブサイトのリーダーボードによると、人間のパフォーマンスは約60で、その次にGroが52.8で、他のすべてよりも高いです。
Next, the AI 2D, I believe, is testing their ability to understand diagrams at 88.3.
次に、AI 2Dですが、図を理解する能力を88.3でテストしていると思います。
Grok is at the top, the only thing better is clot 3 Sonet.
Grokがトップにいて、唯一より良いのはClot 3 Sonetです。
Strangely enough, a smaller model, but GPT-4V is significantly less.
奇妙なことに、より小さなモデルですが、GPT-4Vはかなり少ないです。
CLA 3 Opus is just a tiny bit less, and Gemini Pro 1.5 is a little bit less.
CLA 3 Opusはわずかに少なく、Gemini Pro 1.5は少し少ないです。
I'm not going to go through every single one of these, but text vqa, it's the winner.
私はこれらすべてを詳細に説明するつもりはありませんが、テキストVQAが勝者です。
Chart Q&A, it's lower than the rest but similar to GPT for vision.
チャートQ&Aは他のものよりも低いですが、ビジョンに関してはGPTに似ています。
CLA and Gemini are higher in the 80 and 81%. Doc vqa is at 85% with the highest model clocking in at 89%. So, to sum it all up, it's good, really good.
CLAとGeminiは80%と81%で高いです。Doc VQAは85%で、最高のモデルは89%です。つまり、すべてをまとめると、それは良い、本当に良いです。
What lessons can we draw from this?
この中から何を学ぶことができるでしょうか?
At this point, I got to say, don't bet against Elon Musk.
この時点で、私は言わなければならない、イーロン・マスクに賭けるなということです。
Of course, there's still a number of things to get straightened out.
もちろん、まだ解決すべき問題がいくつかあります。
Just getting the score and the valal is not necessarily the end all be all for how powerful the model is, but it's a start.
スコアとバリューを取得するだけが、モデルの強力さを決定するすべてではありませんが、それはスタートです。
The new search function on X that has been, from what I understand, utilizing Gro to kind of find the news that's more like relevant to you.
私が理解している限り、Xの新しい検索機能は、より関連性の高いニュースを見つけるためにGroを利用しています。
I mean, for the first time ever, I'm actually looking at it.
つまり、初めて、私は実際にそれを見ています。
It was so horrible before, it had no relevant news to me whatsoever, but here they're giving me news that I care about.
以前はひどかったです、私にはまったく関連性のあるニュースがありませんでしたが、ここでは私が関心を持つニュースを提供してくれています。
Mostly AI X is quickly becoming one of the biggest global destinations for news, tons of traffic, tons of people using it.
大部分のAI Xは、ニュースの最大のグローバルな目的地の1つに急速になりつつあり、多くのトラフィック、多くの利用者がいます。
They've recently did a bot purge, getting rid of a lot of the automated traffic.
最近、彼らはボットの一掃を行い、自動化されたトラフィックを大幅に削減しました。
It's their own private data source that's real-time.
それは彼ら自身のリアルタイムのプライベートデータソースです。
Elon had the money, he had the AI talent, he has the data, he has the users to test this thing out, he's got the distribution.
イーロンはお金を持っていたし、AIの才能も持っていたし、データも持っていたし、ユーザーもテストするためのものも持っていたし、配布も持っていました。
The only thing he didn't have was the model.
彼が持っていなかった唯一のものは、モデルでした。
We should reserve judgment until we actually have the model in our hot little hands, until we actually test it and see if it does indeed do all the things that it claims to Do.
実際にモデルを手に入れ、実際にテストして、それが主張するすべてのことを本当に行うかどうかを見るまで、判断を保留すべきです。
But I got to say, from where I'm sitting, some was beginning to look like about a decade ago, Elon was a little concerned that Google would develop a GI in isolation by Itself.
でも、私が座っている場所から見る限り、約10年前、イーロンは、Googleが独自にGIを開発することを懸念していたようです。
It would have this amazing new technology and no one else would.
それは素晴らしい新技術を持っていて、他の誰も持っていない可能性があります。
It's possible, by the way, that Demi saabi, the man that's now running Google deep mine, that maybe he alerted Elon to what was happening.
ちなみに、現在Google DeepMindを運営しているデミ・サービーが、何が起こっているかをイーロンに警告したかもしれません。
Elon goes to Sam Altman and they form OpenAI.
イーロンはサム・オルトマンに行き、OpenAIを設立します。
I believe Anthropic, the people behind Cloud 3, they split off from OpenAI at some point, becoming their own company.
Anthropic、Cloud 3の背後にいる人々は、ある時点でOpenAIから分離し、独自の会社となりました。
And keep in mind that the point here was to have to provide a counterbalance to Google.
そしてここでのポイントは、Googleに対抗するためのカウンターバランスを提供する必要があるということを覚えておいてください。
That was at least the stated goal of Elon Musk back in 2014 or 2015, whenever that whole thing was just brewing.
それは少なくとも、Elon Muskが2014年または2015年に述べた目標であり、その全体がまさに醸成されていた時期でした。
As we're approaching April 20th, 2024, there's Grok.
2024年4月20日に近づいているとき、Grokがあります。
Elon wanted a counterbalance to Google, and now there is.
ElonはGoogleに対抗するものを望んでいましたが、今は存在しています。
Let's count them, not one, not two, but three major competitors.
一つではなく、二つでもなく、三つの主要な競合他社を数えましょう。
And we're not even counting the open source Mistral, the newcomer Command R Plus, as well as all the other open source competitors.
そして、オープンソースのMistralや新参のCommand R Plus、他のすべてのオープンソースの競合他社を数えていません。
But yeah, at this point, I got to say, I got to give credit where credit is due, Elon Musk.
でも、この時点で言わなければならないのは、Elon Muskに敬意を表さなければならないということです。
You don't want to be betting against this guy.
この人に賭けるのは得策ではありません。
But let me know what you think in the comments.
でも、コメントでどう思うか教えてください。
I know that this is a controversial Figure.
私はこの数字が議論を呼ぶことを知っています。
People have a range of emotions about him.
人々は彼に対してさまざまな感情を抱いています。
If we are indeed approaching AI, whatever that means to you, whether you think that's next year or 10 years from now, would you trust him with a powerful technology like That?
もし本当にAIに近づいているのであれば、それがあなたにとってどういう意味であるか、それが来年か10年後か、そう思うのであれば、そのような強力なテクノロジーを彼に信頼しますか?
Would you trust him more than Sam Altman, more than Google?
サム・オルトマンよりも、Googleよりも、彼を信頼しますか?
Meanwhile, it does seem like Demis Hassabis isn't too thrilled how things are going over there at Google deep mine.
一方で、デミス・ハサビスはGoogle DeepMindでの進行具合にあまり興奮していないようです。
A year ago, Google hastily merged the two labs into one, that's Google Brain and Google Deep Mine.
1年前、Googleは急いで2つの研究所、つまりGoogle BrainとGoogle DeepMindを1つに統合しました。
They put it under Hassabis, but the tensions between them have lingered.
彼らはそれをハサビスの下に置きましたが、彼らの間の緊張は残っています。
The dynamic has left Hassabis deeply frustrated.
その状況はハサビスを非常にイライラさせています。
Whatever the case is, the fact that we can watch some of this stuff unfolding live through perhaps various tweets or leaks from inside the companies or even cord documents, The fact that we can kind of watch this play out, I got to say, is quite amazing.
どんな状況であれ、私たちがこのようなことが生中継で見ることができる事実、おそらく会社内からのさまざまなツイートやリーク、さらにはコード文書から、この展開を見守ることができる事実は、私は言わざるを得ません、非常に驚くべきことです。
I hope you enjoy that.
それを楽しんでいただければ幸いです。
Subscribe, you will want to pay attention for what's coming next because this is one field that is heating up.
購読していただくと、次に何が起こるかに注意を払いたくなるでしょう。なぜなら、この分野は急速に盛り上がっているからです。
With that said, my name is Wes Rth, and thank you for watching.
それでは、私の名前はウェス・アールスです。ご視聴ありがとうございました。
この記事が気に入ったらサポートをしてみませんか?