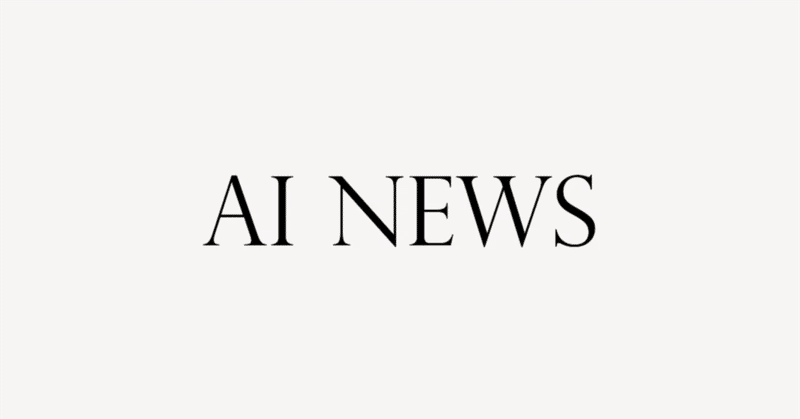
【AIの神様からの衝撃的な展望とAGIへの道】英語解説を日本語で読む【2024年3月17日|@Matthew Berman】
MetaのAI部門の責任者であるジャン・ラクーンがレックス・フリードマンとの対談でAIの未来について語りました。現在の大規模言語モデル(LLM)であるChatGPTのようなものは、人工汎用知能(AGI)を達成するには不十分であると強調しています。ラクーンは、AIの多様性を促進し、分野を前進させる可能性を秘めたオープンソースAIを支持しています。これは、物理世界との相互作用の課題、強化学習の非効率性、複雑な行動に必要な階層的計画の必要性など、現在のAI技術の制限に対処します。さらに、ラクーンはAIの厄介論を批判し、超知能の突然の出現が人類の絶滅につながるという考えに異議を唱えました。AIの発展は徐々に進むものであり、様々な形のAIが人類をより賢く、より効率的にするために役立つだろうと提案しています。議論はまた、AIシステムが人間の価値観を尊重することの重要性と、AIが人間の知能を置き換えるのではなく、強化する役割にも触れています。
公開日:2024年3月17日
※動画を再生してから読むのがオススメです。
Jan Lakoon, the head of Meta's AI division, just did a three-hour interview with Lex Fridman in which he covered robots, AGI, and so much more.
ジャン・ラクーンは、MetaのAI部門の責任者であり、レックス・フリードマンとの3時間のインタビューを行い、そこでロボット、AGIなどについて話しました。
I went through the whole interview, I grabbed the bits that I thought were most interesting, and I encourage you to watch the whole video.
私はそのインタビュー全体を見て、最も興味深い部分を抜粋しました。全体のビデオをご覧いただくことをお勧めします。
I'll drop a link to that in the description below.
そのリンクを以下の説明欄に載せておきます。
In this first clip, Jan talks about how autoregressive LLMs, basically the Large Language Models that we all know today, like OpenAI's ChatGPT, won't get us to AGI.
この最初のクリップでは、ジャンは、自己回帰型大規模言語モデル、つまり私たちが今日知っている大規模言語モデル(例えばOpenAIのChatGPT)は、AGIには至らないと語っています。
Jan is a huge proponent of open source, but he doesn't think our current technology will allow us to achieve AGI.
ジャンはオープンソースの熱心な支持者ですが、現在の技術ではAGIを達成することはできないと考えています。
Let's watch.
一緒に見ましょう。
For a number of reasons.
いくつかの理由があります。
The first is that there is a number of characteristics of intelligent behavior.
その最初の理由は、知的行動の特性がいくつかあるということです。
For example, the capacity to understand the world, understand the physical world, the ability to remember and retrieve things, persistent memory, the ability to reason, and the ability to plan.
例えば、世界を理解する能力、物理世界を理解する能力、物事を覚えて取り出す能力、持続的な記憶、推論する能力、計画を立てる能力などがあります。
Those are four essential characteristics of intelligent systems or entities, humans, animals.
それらは、知的システムや実体、人間、動物の4つの基本的な特性です。
LLMs can do none of those, or they can only do them in a very primitive way.
大規模言語モデルはそれらのどれもできません、あるいは非常に原始的な方法でしかできません。
They don't really understand the physical world, they don't really have persistent memory, they can't really reason, and they certainly can't plan.
彼らは物理世界を本当に理解していないし、持続的な記憶も本当に持っていないし、本当に理論的に考えることもできないし、確かに計画も立てることはできません。
If you expect a system to become intelligent just without having the possibility of doing those things, you're making a mistake.
もし、それらのことをする可能性がないままにシステムが知的になることを期待しているなら、それは間違いです。
And then next, following up on the premise that our current technologies won't get us to AGI, Jan talks about the amount of data necessary to train Large Language Models as compared to what humans use to essentially train our own minds.
そして次に、現在の技術ではAGIに到達できないという前提に続いて、ジャンは、大規模言語モデルを訓練するために必要なデータ量について、人間が本質的に自分たちの心を訓練するのに必要なデータと比較して話しています。
And he says that the amount of data used to train Large Language Models is actually not that much compared to what humans need.
そして、大規模言語モデルを訓練するために使用されるデータ量は、実際には人間が必要とする量と比較してそれほど多くないと彼は言っています。
And this is where we start to get into synthetic data.
ここで、合成データについて話し始めます。
And I've been preparing my thoughts about synthetic data quite a bit because the fact of the matter is, I don't think humans are going to produce enough data to actually reach AGI using Large Language Models, even if our current architecture could allow us to get there.
私は合成データについてかなり考えを練ってきました。現実の問題は、現在のアーキテクチャがそこに到達することを可能にしても、人間が大規模言語モデルを使用してAGIに到達するために十分なデータを生み出すことはできないと思っているからです。
Synthetic data, meaning data that has been created by other artificial intelligence, is one of those necessary ingredients to reaching AGI.
他の人工知能によって作成されたデータである合成データは、AGIに到達するための必要な要素の1つです。
Let's take a look at this video.
このビデオを見てみましょう。
Those LLMs are trained on enormous amounts of text, basically the entirety of all publicly available text on the internet.
これらの大規模言語モデルは、基本的にインターネット上で公開されているすべてのテキストの膨大な量で訓練されています。
That's typically on the order of 10 to the 13 tokens.
典型的には、10の13乗のトークンの順序です。
Each token is typically two bytes.
各トークンは通常2バイトです。
That's two 10 to the 13 bytes as training data.
訓練データとしては、2つの10の13乗バイトが必要です。
It would take you or me 170,000 years to just read through this at eight hours a day.
1日8時間でこれを読むだけでも、あなたや私には17万年かかるでしょう。
It seems like an enormous amount of knowledge that those systems can accumulate.
それらのシステムが蓄積できる知識の量は膨大なように思えます。
But then you realize it's really not that much data.
しかし、実際にはそれほど多くのデータではないことに気づきます。
If you talk to developmental psychologists and they tell you a four-year-old has been awake for 16,000 hours in his or her life and the amount of information that has reached the visual cortex of that child in four years, it's about 10 to the 15 bytes.
発達心理学者と話すと、4歳の子供が生涯で16,000時間目を覚ましていると言われ、その子供の視覚皮質に到達した情報量は約10の15乗バイトです。
And you can compute this by estimating that the optical nerve carry about 20 megabytes per second, roughly.
光神経がおおよそ1秒あたり20メガバイトを運ぶと見積もると、これを計算することができます。
10 to the 15 bytes for a four-year-old versus two times 10 to the 13 bytes for 170,000 years worth of reading, what that tells you is that through sensory input, we see a lot more information than we do through language.
4歳の子供の場合、10の15乗バイトに対して、17万年分の読書に必要な2つの10の13乗バイト、これは、感覚入力を通じて、言語よりもはるかに多くの情報を見るということを示しています。
And that despite our intuition, most of what we learn and most of our knowledge is through our observation and interaction with the real world, not through language.
言語を通じてではなく、実際の世界との観察や相互作用を通じて学ぶことがほとんどであり、私たちの知識のほとんどがそうであるにもかかわらず、私たちの直感にもかかわらず。
Everything that we learn in the first few years of life and certainly everything that animals learn has nothing to do with language.
生まれて最初の数年間に学ぶすべて、そして確かに動物が学ぶすべては、言語とは何の関係もありません。
And then again, continuing with the Large Language Models are not going to allow us to reach AGI.
そして、再び、大規模言語モデルを続けることは、私たちがAGIに到達するのを許さないだろう。
He basically says that just using language is not enough to model the world, to have a world model.
彼は基本的に、世界をモデル化するには言語だけでは十分ではないと言っています。
Even though language is highly compressed, he describes how when people are talking and thinking about things, they're using much more than just language.
言語は非常に圧縮されているにもかかわらず、人々が物事について話したり考えたりするとき、言語だけでなく、はるかに多くを使用している方法を彼は説明しています。
We have a world model inside our head and we're conceptualizing ideas and perceptions before we even convert those into language.
私たちの頭の中には世界モデルがあり、私たちはアイデアや認識を概念化してから、それらを言語に変換する前に考えています。
It's very interesting to think about.
考えるのは非常に興味深いです。
But because of this, he says, we're not going to reach AGI with language alone.
しかし、このため、彼は言語だけではAGIに到達しないと言っています。
We need some other kind of technology to help us augment the language part.
言語部分を補完するための他の種類の技術が必要です。
Let's look at that video.
そのビデオを見てみましょう。
It's a big debate among philosophers and also cognitive scientists, like whether intelligence needs to be grounded in reality.
知識人や認知科学者の間で大きな議論がありますが、知能は現実に基づいている必要があるかどうかということです。
I'm clearly in the camp that, yes, intelligence cannot appear without some grounding in some reality.
私ははっきりと、はい、知能はある現実に基づいていないと現れることはできないという立場です。
It doesn't need to be physical reality, it could be simulated, but the environment is just much richer than what you can express in language.
それは物理的な現実である必要はなく、シミュレートされたものでもかまいませんが、環境は言葉で表現できる以上にはるかに豊かです。
Language is a very approximate representation of our percepts and our mental models, right?
言語は私たちの知覚や精神モデルの非常におおよその表現ですね。
I mean, there's a lot of tasks that we accomplish where we manipulate a mental model of the situation at hand, and that has nothing to do with language.
つまり、私たちが達成する多くのタスクでは、手元の状況の精神モデルを操作することがあり、それは言語とは何の関係もありません。
And next, kind of proving the point that Large Language Models in their current form aren't going to reach AGI, Jan goes on to describe why Large Language Models are really good at some things like creative writing, like coding, passing the bar exam and other things like that, but really bad at other things like self-driving and the ability to simply pick up a cup.
そして次に、現在の形態の大規模言語モデルがAGIに到達しないという点を証明するために、ジャンは大規模言語モデルが創造的な執筆やコーディング、司法試験の合格などの点で非常に優れているが、自動運転や単にカップを取る能力などの他の点では非常に不得手である理由を説明します。
And for some reason, Large Language Models are really good at some things and really bad at others.
そして何故か、大規模言語モデルはある点で非常に優れており、他の点では非常に不得手である。
Let's take a look.
では見てみましょう。
You know, we have LLMs that can pass the bar exam, so they must be smart, but then they can't learn to drive in 20 hours like any 17-year-old.
弁護士試験に合格できる大規模言語モデルがいるので、彼らは賢いに違いない、しかし、17歳の誰でも20時間で運転を学ぶことができない。
They can't learn to clear out the dinner table and fill out the dishwasher like any 10-year-old can learn in one shot.
彼らは、10歳の誰でも一発で夕食のテーブルを片付けて食器洗い機に詰めることを学ぶことができない。
Why is that?
なぜですか?
Like what are we missing?
何が足りないのでしょうか?
What type of learning or reasoning architecture or whatever are we missing that basically prevent us from having level five self-driving cars and domestic robots?
私たちがレベル5の自動運転車や家庭用ロボットを持つことを基本的に阻止している学習や推論のアーキテクチャ、またはその他の何かは何ですか?
And then finally, kind of closing the loop on AGI and what's possible with our current Large Language Model architecture, he goes on to talk about how kind of world model reasoning is out of reach for Large Language Models as they are today.
そして最後に、AGIに関して、そして現在の大規模言語モデルアーキテクチャで可能なことについて、彼は、現在の大規模言語モデルにとって世界モデル推論が手の届かないと話します。
He continues to explain that when humans are talking about things and conceptualizing things in the real world, we're doing so much more than just speaking in our minds.
彼は、人間が物事について話したり、現実世界で物事を概念化する際に、私たちは単に頭の中で話す以上のことをしていると説明し続けます。
He's really doubling down on this point, which I find fascinating.
彼はこのポイントを本当に強調しており、私はそれを魅力的だと思います。
Let's take a look at this clip.
このビデオを見てみましょう。
We're not going to be able to do this with the type of LLMs that we are working with today.
今日私たちが取り組んでいる大規模言語モデルの種類では、これを行うことはできません。
And there's a number of reasons for this.
これにはいくつかの理由があります。
But the main reason is the way LLMs are trained is that you take a piece of text, remove some of the words in that text, you mask them, you replace them by blank markers, and you train a genetic neural net to predict the words that are missing.
しかし、主な理由は、大規模言語モデルのトレーニング方法が、テキストの一部を取り、そのテキストの一部の単語を取り除き、それらをマスクし、空白マーカーで置き換え、欠落している単語を予測するために遺伝的ニューラルネットをトレーニングするという方法であることです。
And if you build this neural net in a particular way so that it can only look at words that are to the left of the one it's trying to predict, then what you have is a system that basically is trained to predict the next word in a text, right?
あなたがこのニューラルネットワークを特定の方法で構築して、予測しようとしている単語の左側の単語だけを見るようにすれば、それは基本的にテキスト内の次の単語を予測するように訓練されたシステムになりますね。
Then you can feed it a text, a prompt, and you can ask it to predict the next word.
その後、テキストやプロンプトを入力し、次の単語を予測するように求めることができます。
It can never predict the next word exactly.
それは決して次の単語を正確に予測することはできません。
What it's going to do is produce a probability distribution of all the possible words in your dictionary.
それが行うことは、辞書内のすべての可能な単語の確率分布を生成することです。
In fact, it doesn't predict words, it predicts tokens that are kind of subword units.
実際、単語を予測するのではなく、サブワードユニットのようなトークンを予測します。
It's easy to handle the uncertainty in the prediction there because there is only a finite number of possible words in the dictionary, and you can just compute the distribution over them.
辞書に含まれる単語の数が有限であることから、その予測における不確実性の取り扱いは容易であり、それらの単語の分布を計算することができます。
Then what the system does is that it picks a word from that distribution.
その後、システムがその分布から単語を選択します。
Of course, there's a higher chance of picking words that have a higher probability within that distribution. So you sample from that distribution to actually produce a word.
もちろん、その分布内で確率が高い単語を選ぶ可能性が高いです。その分布からサンプリングして実際に単語を生成します。
And then you shift that word into the input.
そして、その単語を入力にシフトします。
That allows the system not to predict the second word.
それにより、システムは2番目の単語を予測しないようになります。
And once you do this, you shift it into the input, etc.
そして、これを行うと、入力に移行します。
That's called autoregressive prediction, which is why those LLMs should be called autoregressive LLMs.
それは自己回帰予測と呼ばれ、だからこそ、それらの大規模言語モデルは自己回帰大規模言語モデルと呼ばれるべきです。
There is a difference between this kind of process and a process by which before producing a word, when you talk, when you and I talk, you and I are bilinguals, we think about what we're going to say, and it's relatively independent of the language in which we're going to say.
この種のプロセスと、話すとき、あなたと私が話すとき、あなたと私がバイリンガルであるとき、単語を生成する前に、私たちが何を言うか考えるプロセスとの違いがあります。そして、私たちが言う言語とは比較的独立しています。
When we talk about, I don't know, let's say a mathematical concept or something, the kind of thinking that we're doing and the answer that we're planning to produce is not linked to whether we're going to see it in French or Russian or English.
数学的な概念などについて話すとき、私たちが行っている思考や生産しようとしている答えは、それがフランス語やロシア語、英語で見るかどうかには関係ありません。
And next we continue our discussion about language as a world model.
そして次に、言語を世界モデルとして考える議論を続けます。
Jan is in one camp that believes language alone cannot be a world model.
ジャンは、言語だけでは世界モデルになり得ないと考える派に属しています。
And I want to know what you think.
そして私は、あなたがどう思うか知りたいです。
Do you agree or disagree?
賛成ですか、反対ですか?
When you see something like Sora, which uses transformers, which uses current architecture that is found in Large Language Models, it is pretty darn good at simulating the world.
トランスフォーマーを使用し、大規模言語モデルで見られる現在のアーキテクチャを使用するSoraのようなものを見ると、それは世界をシミュレートするのに非常に優れています。
But it's not perfect.
しかし完璧ではありません。
And if it's ever going to get there is still a question.
もし将来そこに到達することがあるとすれば、それはまだ疑問です。
And he continues to hammer the point that Large Language Models are not enough to reach a world model.
そして、彼は大規模言語モデルだけでは世界モデルに到達するには不十分だという点を強調し続けています。
But this actually reminds me of a couple things.
しかし、これは実際に私にいくつかのことを思い出させます。
There's the movie Arrival.
映画『Arrival』があります。
And if you haven't seen it, it's a fantastic science fiction movie.
もし見ていない場合は、それは素晴らしいSF映画です。
But the gist is aliens come down from space and they speak a different language and they have an expert linguist try to Figure out what that language is.
しかし、要点は異星人が宇宙から降りてきて異なる言語を話し、専門の言語学者がその言語が何かを解明しようとするというものです。
And over time, they Figure it out.
そして時間をかけて、彼らはそれを理解していきます。
And the language itself is what the aliens are there to give humanity as kind of the great gift.
そして言語そのものが、異星人が人類に与えるべき偉大な贈り物としてそこにいるのです。
And all of a sudden, because this expert linguist now understands this new language, she starts to gain all of this power.
そして突然、この専門の言語学者が新しい言語を理解するようになると、彼女はこの力をすべて手に入れ始めます。
It's super interesting to think about that language actually shapes perception.
その言語が実際に知覚を形作ると考えるのは非常に興味深いです。
And that is something I believe in.
それは私が信じていることです。
If we don't have a word for something, then most likely we can't describe it and maybe we can't even perceive it as it really is.
もし何かの言葉がない場合、おそらくそれを説明することもできず、実際の姿を認識することさえできないかもしれません。
I believe language is incredibly important, but I'll probably defer to Jan thinking that language alone is not enough to have a world model.
言語は非常に重要だと思っていますが、おそらく、言語だけでは世界モデルを持つには十分ではないと考えているジャンに譲るでしょう。
You're saying your thinking is same in French as it is in English.
あなたは、フランス語での考え方が英語と同じだと言っていますね。
Well, it depends what kind of thinking, right?
それはどんな種類の考え方かによりますよね。
If it's like producing puns, I get much better in French than English about that.
ダジャレを考える場合、私はフランス語の方が英語よりもずっと上手になります。
No, but is there an abstract representation of puns?
いいえ、しかしダジャレの抽象的な表現はありますか?
Is your humor an abstract representation?
あなたのユーモアは抽象的な表現ですか?
Like when you tweet and your tweets are sometimes a little bit spicy, is there an abstract representation in your brain of a tweet before it maps onto English?
例えば、ツイートをするとき、時々少し辛口なツイートをすると、そのツイートが英語にマッピングされる前に、脳内にそのツイートの抽象的な表現がありますか?
There is an abstract representation of imagining the reaction of a reader to that text.
そのテキストへの読者の反応を想像する抽象的な表現があります。
You start with laughter
あなたは笑いから始めます
And then Figure out how to make that happen?
それから、それをどうやって実現するか考えますか?
Or Figure out a reaction you want to cause and then Figure out how to say it so that it causes that reaction.
ある反応を引き起こしたいと考え、それがその反応を引き起こすように言葉を選ぶ方法を考えます。
But that's really close to language.
でも、それは言語に非常に近いです。
But think about a mathematical concept or imagining something you want to build out of wood or something like this.
数学的な概念を考えたり、木で何かを作りたいと想像したりすることを考えてみてください。
The kind of thinking you're doing is absolutely nothing to do with language, really.
あなたがしている考え方は、実際には言語とは全く関係ありません。
It's not like you have necessarily an internal monologue in any particular language.
特定の言語で内部モノローグを持っているわけではありません。
You're imagining mental models of the thing, right?
あなたは物事の心理的なモデルを想像しているのですよね?
If I ask you to imagine what this water bottle will look like if I rotate it 90 degrees, that has nothing to do with language.
もし私がこの水筒を90度回転させたらどのように見えるかを想像するように頼んだとしても、それは言語とは何の関係もありません。
Clearly there is a more abstract level of representation in which we do most of our thinking and we plan what we're going to say.
明らかに、私たちが考えをほとんど行い、言うことを計画する抽象的なレベルが存在しています。
If the output is uttered words as opposed to an output being muscle actions, we plan our answer before we produce it.
もし出力が筋肉の動作ではなく言葉である場合、私たちは答えを考えてからそれを出力する計画を立てます。
And LLMs don't do that.
大規模言語モデルはそうではありません。
They just produce one word after the other, instinctively, if you want.
彼らは単に本能的に、一つの単語を次々に生み出すだけです。
And in this next clip, Jan talks about if we're able to have a world model based on prediction alone.
そして、この次のクリップでは、ヤンが予測だけに基づいた世界モデルを持つことができるかどうかについて話しています。
And again, the way that Large Language Models work is that it's essentially just predicting the next token in a sentence, the next word in a sentence.
そして、大規模言語モデルが動作する方法は、基本的に文の次のトークン、文の次の単語を予測するだけです。
And he actually says, yes, just based on prediction alone, that part of the technology, we will be able to achieve AGI.
そして、実際に彼は、はい、予測だけに基づいて、その技術の一部によって、AGIを達成することができると言っています。
We will be able to achieve a world model.
私たちは世界モデルを達成することができるでしょう。
But just based on language prediction alone, he doesn't think so.
しかし、言語予測だけに基づいて、彼はそうは思わないと言っています。
And this starts to get into simulation theory.
そして、これはシミュレーション理論に入り始めます。
Do you need to understand every single atom?
すべての単一の原子を理解する必要がありますか?
Do you need to predict how every single atom is going to move to truly create a world model?
全ての原子がどのように動くかを予測する必要がありますか、本当に世界モデルを作成するために?
It turns out maybe not.
実際には、おそらくそうではないことがわかります。
And this is actually something a Twitter user had replied to me, which I had never really thought about.
これは実際には、私に返信したTwitterユーザーが考えたことで、私自身はあまり考えたことがありませんでした。
With simulation theory, I always thought that you had to predict every single atom, but that's not necessarily true.
シミュレーション理論では、常に全ての原子を予測する必要があると思っていましたが、それは必ずしも真実ではありません。
You only have to predict enough that the perceiver, the person who is viewing this reality is convinced that it looks real.
あなたは、この現実を見ている人がそれがリアルに見えると納得するだけの予測をしなければなりません。
Just enough, but not everything.
ちょうど十分なだけで、すべてではありません。
Let's take a look at this clip.
この動画を見てみましょう。
Can you build this, first of all, by prediction?
まず、これを予測して構築できますか?
And the answer is probably yes.
その答えはおそらくはいです。
Can you build it by predicting words?
言葉を予測してこれを構築することはできますか?
And the answer is most probably no, because language is very poor in terms of weak or low bandwidth, if you want.
おそらく答えは「いいえ」でしょう、なぜなら言語は弱いまたは低い帯域幅に関して非常に貧弱です。
There's just not enough information there.
そこには情報が不足しているだけです。
Building world models means observing the world and understanding why the world is evolving the way it is.
世界モデルを構築するということは、世界を観察し、なぜ世界が進化しているのかを理解することを意味します。
And then the extra component of a world model is something that can predict how the world is going to evolve as a consequence of an action you might take.
そして、世界モデルの追加要素は、あなたが取るかもしれない行動の結果として世界がどのように進化するかを予測できるものです。
What model really is, here is my idea of the state of the world at time t, here is an action I might take, what is the predicted state of the world at time t plus one.
本当のモデルとは、ここに時間tでの世界の状態の私の考えがあり、ここに私が取るかもしれない行動があり、時間tプラス1での世界の予測される状態があります。
Now that state of the world does not need to represent everything about the world.
その世界の状態は、世界についてすべてを表現する必要はありません。
It just needs to represent enough that's relevant for this planning of the action, but not necessarily all the details.
それは、行動の計画にとって関連性のあるだけの情報を表現する必要がありますが、すべての詳細を必ずしも表現する必要はありません。
Here is the problem.
ここに問題があります。
You're not going to be able to do this with generative models.
生成モデルではこれを行うことはできません。
A generative model is trained on video, and we've tried to do this for 10 years.
生成モデルはビデオで訓練されており、私たちはこれを10年間試みてきました。
You take a video, show a system a piece of video.
お客様はビデオを撮影し、そのビデオの一部をシステムに見せます。
And then ask it to predict the reminder of the video.
そして、その後、ビデオの残りを予測するように求めます。
Basically predict what's going to happen, either one frame at a time or a group of frames at a time.
基本的には、1フレームずつまたは複数のフレームずつ、何が起こるかを予測します。
But yeah, a large video model if you want.
でも、大規模なビデオモデルが必要です。
The idea of doing this has been floating around for a long time, and at FAIR, colleagues and I have been trying to do this for about 10 years.
このアイデアは長い間浮上していましたが、FAIRでは、同僚と一緒に約10年間取り組んできました。
And you can't really do the same trick as with LLMs, because LLMs, as I said, you can't predict exactly which word is going to follow a sequence of words, but you can predict the distribution of the words.
そして、大規模言語モデルと同じトリックはできません。大規模言語モデルでは、私が言ったように、単語のシーケンスの後にどの単語が続くかを正確に予測することはできませんが、単語の分布を予測することはできます。
If you go to video, what you would have to do is predict the distribution of all possible frames in a video, and we don't really know how to do that properly.
ビデオに移ると、ビデオ内のすべての可能なフレームの分布を予測する必要がありますが、それを適切に行う方法は実際にはわかりません。
We do not know how to represent distributions over high dimensional continuous spaces in ways that are useful.
高次元連続空間上の分布を有用な方法で表現する方法を私たちは知りません。
And there lies the main issue, and the reason we can do this is because the world is incredibly more complicated and richer in terms of information than text.
そして、それが主な問題であり、これを行う理由は、世界がテキストよりも情報としてはるかに複雑で豊かであるためです。
Text is discrete, video is high dimensional and continuous, a lot of details in this.
テキストは離散的であり、ビデオは高次元で連続的であり、多くの詳細があります。
Okay, in this next clip, he breaks down why video prediction, basically frame by frame prediction, or even an entire video prediction, is really hard to do.
では、次のクリップでは、ビデオ予測、基本的にはフレームごとの予測、またはビデオ全体の予測が非常に難しい理由を説明しています。
And this interview came out after Sora.
そして、このインタビューはSoraの後に出たものです。
As you're listening to this, think about what Sora was able to achieve and compare it to what Jan is saying here.
これを聞いている間に、Soraが何を達成したか考え、ここでヤンが言っていることと比較してみてください。
If I take a video of this room, and the video is a camera panning around, there is no way I can predict everything that's going to be in the room as I pan around.
この部屋のビデオを撮影し、カメラが周りをパンしているビデオを撮影した場合、私が周りをパンするときに部屋にあるすべてのものを予測する方法はありません。
The system cannot predict what's going to be in the room as the camera is panning.
システムは、カメラがパンしているときに部屋に何があるかを予測することはできません。
Maybe it's going to predict this is this is a room where there is a light and there is a wall and things like that, it can't predict what the painting of the world looks like or what the texture of the couch looks like, certainly not the texture of the carpet.
おそらく、これは、光がある部屋であり、壁があり、そのようなものがある部屋であると予測するかもしれませんが、世界の絵がどのように見えるかや、ソファの質感がどのように見えるか、カーペットの質感はまったく予測できません。
There's no way I can predict all those details.
私はすべての詳細を予測する方法はありません。
One way possibly to handle this, which we've been working for a long time, is to have a model that has what's called a latent variable, and the latent variable is fed to a neural net.
これを処理するための可能性のある方法の1つは、長い間取り組んできた方法で、潜在変数と呼ばれるモデルを持ち、その潜在変数がニューラルネットワークに供給されます。
And it's supposed to represent all the information about the world that you don't perceive yet, that you need to augment the system for the prediction to do a good job at predicting pixels, including the fine texture of the carpet and the couch and the painting on the wall.
そして、それは、まだ知覚していない世界に関するすべての情報を表現し、予測がピクセルを正確に予測するためにシステムを補完するために必要な情報を含む、カーペットやソファの微細な質感、壁の絵などを予測するのに良い仕事をする必要があります。
That has been a complete failure.
それは完全な失敗でした。
And we've tried lots of things.
私たちはたくさんのことを試してきました。
We tried just straight neural nets, we tried GANs, we tried VAEs, all kinds of regularized auto encoders.
私たちはただのニューラルネットワーク、GAN、VAEなど、さまざまな正則化されたオートエンコーダーを試しました。
We tried many things.
私たちはたくさんのことを試しました。
And in this next clip, he goes into the details about why video prediction doesn't work with our current architecture, our current technology.
そして、この次のクリップでは、なぜビデオ予測が現在のアーキテクチャや技術ではうまくいかないのかについて詳細に説明しています。
And of course, Jan recently released JEPA, which is what he believes is going to be able to augment Large Language Models and transformers to be able to allow to actually have a world model with video.
そしてもちろん、最近ジャンはJEPAをリリースしました。これは、彼が信じるところによると、大規模言語モデルとトランスフォーマーを拡張し、実際にビデオを持つワールドモデルを可能にすると考えています。
Let's see what he says about that.
それについて彼が何と言うか見てみましょう。
The reason this doesn't work, first of all, I have to tell you exactly what doesn't work because there is something else that does work.
これがうまくいかない理由は、まず第一に、何がうまくいかないのかを正確にお伝えしなければならないのです。なぜなら、うまくいく別の方法があるからです。
The thing that does not work is training the system to learn representations of images by training it to reconstruct a good image from a corrupted version of it.
うまくいかないのは、システムを訓練して、画像の表現を学習させること、つまり、それを修正されたバージョンから元の画像を再構築するように訓練することです。
That's what doesn't work.
それがうまくいかないことです。
And we have a whole slew of techniques for this that are a variant of denoising auto encoders.
そして、これに対する様々な変種のノイズ除去オートエンコーダーのテクニックがあります。
Something called MAE developed by some of my colleagues at FAIR, masked auto encoder.
私の同僚のいくつかが開発したMAEというもの、マスク付きオートエンコーダーというものがあります。
It's basically like the LLMs or things like this where you train the system by corrupting text except you corrupt images.
基本的には大規模言語モデルやこれに類するもののようで、テキストを破損させることでシステムを訓練するのですが、画像を破損させるのです。
You remove patches from it and you train a gigantic neural net to reconstruct.
それからパッチを取り除き、巨大なニューラルネットを訓練して再構築します。
The features you get are not good.
得られる特徴は良くありません。
And you know they're not good because if you now train the same architecture but you train it supervised with label data, with textual descriptions of images, you do get good representations.
そして、それらが良くないことはわかります。なぜなら、同じアーキテクチャを訓練するが、ラベルデータや画像のテキスト説明で教師付きで訓練すると、良い表現が得られるからです。
And the performance on recognition tasks is much better than if you do this self-supervised retraining.
認識タスクのパフォーマンスは、この自己教師付き再訓練を行うよりもはるかに優れています。
The architecture of the encoder is good, but the fact that you train the system to reconstruct images does not lead it to produce to learn good generic features of images.
エンコーダーのアーキテクチャは良いですが、画像を再構築するようにシステムを訓練することは、画像の良い一般的な特徴を学習させることにはつながりません。
When you train in a self-supervised way.
自己教師付きで訓練するとき。
Self-supervised by reconstruction.
再構築による自己教師付き。
Let's watch what he has to say about JEPA.
JEPAについて彼が何を言っているのか見てみましょう。
And again, JEPA was just released by Meta under Jan's leadership with his entire team.
再び、JEPAはヤンのリーダーシップのもとでメタによってリリースされました。
And it's a very interesting project.
それは非常に興味深いプロジェクトです。
It gives the ability to augment Large Language Models with the ability to predict video.
それは大規模言語モデルを拡張し、ビデオを予測する能力を与えます。
And I'm still wrapping my mind around it.
私はまだそれを理解しようとしています。
I'm going to let him explain it.
私は彼に説明させるつもりです。
The alternative is joint embedding.
代替案は共同埋め込みです。
Instead of training a system to encode the image
画像をエンコードするシステムを訓練する代わりに
And then training it to reconstruct the full image from a corrupted version, you take the full image, you take the corrupted or transformed version, you run them both through encoders, which in general are identical, but not necessarily.
そして、それを訓練して、壊れたバージョンから完全な画像を再構築する代わりに、完全な画像を取り、壊れたまたは変換されたバージョンを取り、それらを両方エンコーダーを通して実行します。一般的には同一ですが、必ずしも同じではありません。
And then you train a predictor on top of those encoders to predict the representation of the full input from the representation of the corrupted one. So joint embedding, because you're taking the full input and the corrupted version, run them both through encoders, you get a joint.
そして、それらのエンコーダーの上に予測器を訓練して、壊れたものの表現から完全な入力の表現を予測します。したがって、共同埋め込みは、完全な入力と壊れたバージョンを取るため、それらを両方エンコーダーを通して実行すると、共同埋め込みが得られます。
And then you're saying, can I predict the representation of the full one from the representation of the corrupted one?
そして、あなたは言っています、私は壊れたものの表現から完全なものの表現を予測できますか?
And I call this a JEPA.
これをJEPAと呼んでいます。
That means joint embedding predictive architecture, because there's joint embedding and there is this predictor that predicts the representation of the good guy from the bad guy.
それは共同埋め込み予測アーキテクチャの意味です。なぜなら、共同埋め込みがあり、善人の表現を悪人から予測する予測器があるからです。
And the big question is, how do you train something like this?
そして大きな問題は、このようなものをどのように訓練するかですか?
And until five years ago or six years ago, we didn't have particularly good answers.
そして5年前か6年前までは、特に良い答えがありませんでした。
He's going to talk about what is the difference between Large Language Models and and why them working together might be the solution to reach AGI and a world model.
彼は、大規模言語モデルとの違いと、それらが一緒に動作することがAGIやワールドモデルに到達する解決策である可能性について話します。
First of all, what's the difference with generative architectures like LLMs?
まず、大規模言語モデルのような生成アーキテクチャとの違いは何ですか?
LLMs or vision systems that are trained by reconstruction generate the inputs, right?
大規模言語モデルや再構築によって訓練されたビジョンシステムは、入力を生成しますね?
They generate the original input that is non-corrupted, non-transformed.
彼らは、非破損、非変換の元の入力を生成します。
You have to predict all the pixels and there is a huge amount of resources spent in the system to actually predict all those pixels, all the details.
すべてのピクセルを予測する必要があり、すべての詳細を実際に予測するためにシステムに多くのリソースが費やされます。
In a JEPA, you're not trying to predict all the pixels, you're only trying to predict an abstract representation of the inputs.
抽象的な表現を予測しようとしているだけで、すべてのピクセルを予測しようとしているわけではありません。
And that's much easier in many ways.
そして、それは多くの点ではるかに簡単です。
What the JEPA system, when it's being trained, is trying to do is extract as much information as possible from the input, but yet only extract information that is relatively easily predictable.
JEPAシステムがトレーニングされているときにやろうとしていることは、入力から可能な限り多くの情報を抽出することですが、比較的簡単に予測できる情報のみを抽出することです。
There's a lot of things in the world that we cannot predict, like for example, if you have a self-driving car driving down the street, there may be trees around the road and it could be a windy day, so the leaves on the tree are kind of moving in kind of semi-chaotic random ways that you can't predict and you don't care.
例えば、自動運転車が道路を走行している場合、道路の周りに木があるかもしれず、風の強い日かもしれません。そのため、木の葉が半ばカオス的なランダムな方法で動いていることを予測できず、気にする必要もありません。
What you want is your encoder to basically eliminate all those details.
あなたが望むのは、エンコーダーが基本的にすべての詳細を排除することです。
We'll tell you there's moving leaves, but it's not going to keep the details of exactly what's going on.
動く葉があることを教えてくれますが、実際に何が起こっているかの詳細を保持しません。
When you do the prediction in representation space, you're not going to have to predict every single pixel of every leaf.
表現空間で予測を行うと、すべての葉のすべてのピクセルを予測する必要はありません。
And that not only is a lot simpler, but also it allows the system to essentially learn an abstract representation of the world where what can be modeled and predicted is preserved and the rest is viewed as noise and eliminated by the encoder.
これは非常に単純であり、システムが基本的に世界の抽象的な表現を学習し、モデル化および予測できるものを保持し、残りをノイズとして見なしてエンコーダーによって排除することを可能にします。
It kind of lifts the level of abstraction of the representation.
それは表現の抽象化のレベルを上げるようです。
If you think about this, this is something we do absolutely all the time.
これを考えると、私たちは絶対にいつもそうしていることです。
Whenever we describe a phenomenon, we describe it at a particular level of abstraction and we don't always describe every natural phenomenon in terms of quantum field theory, that would be impossible.
現象を説明する際は、特定の抽象化レベルで説明し、すべての自然現象を量子場理論で説明するわけではない、それは不可能です。
We have multiple levels of abstraction to describe what happens in the world, starting from quantum field theory to atomic theory and molecules and chemistry, all the way up to concrete objects in the real world and things like that.
世界で起こることを説明するために、量子場理論から原子理論、分子や化学、そして現実世界の具体的な物体など、複数の抽象化レベルがあります。
We can't model everything at the lowest level.
すべてを最低レベルでモデル化することはできません。
That's what the idea of JEPA is really about, learn abstract representation in a self-supervised manner and you can do it hierarchically as well.
それがJEPAのアイデアの本質であり、自己監督学習で抽象表現を学び、階層的に行うことができます。
So that I think is an essential component of an intelligent system and in language we can get away without doing this because language is already to some level abstract and already has eliminated a lot of information that is not predictable.
それが知的システムの重要な要素であり、言語ではこれをしなくても済みますが、言語はすでにある程度抽象化されており、予測できない情報をすでに排除しています。
We can get away without doing the joint embedding, without lifting the abstraction level and by directly predicting words.
共通の埋め込みを行わずに、抽象化レベルを上げずに、単語を直接予測することで、私たちは逃れることができます。
In this next clip, Jan talks about hierarchical planning and what that essentially means is There is a ton of planning that needs to happen for basic actions that we take every single day. So to describe hierarchical planning, let's use an example.
次のクリップでは、ヤンが階層的計画について話しており、基本的な行動のために行う計画がたくさんあるということです。階層的計画を説明するために、例を挙げましょう。
Let's say I want to drive to the store.
例えば、私は店に車で行きたいとします。
First I have to get up and walk to my car.
まず、起き上がって車まで歩かなければなりません。
How do I do that?
それをどうやってすればいいのですか?
Well first I have to actually stand up.
まず、実際に立ち上がらなければなりません。
Well how do I do that?
それをどうやってすればいいのですか?
Well my brain has to tell my legs to use certain muscles to push me up.
脳が私の足に特定の筋肉を使って立ち上がるように指示する必要があります。
And then how do I do that?
それから、どうやってすればいいのですか?
It's this recursive algorithm that is required, and every single step from something as basic as just walking to the car is actually thousands, or really just infinite steps if you really think about it.
これは再帰的アルゴリズムが必要であり、ただ車に向かって歩くという基本的な行為から始まるすべてのステップは、実際には何千ものステップ、実際には無限のステップです。
How do you model that?
それをどうモデル化するのですか?
How do you actually model that with current architecture?
現在のアーキテクチャでそれを実際にどうモデル化するのですか?
That's what he starts to talk about here.
それについて彼が話し始めるのはここからです。
Let's watch.
見ましょう。
Hierarchical planning is absolutely necessary if you want to plan complex actions.
複雑な行動を計画する場合、階層的な計画が絶対に必要です。
If I want to go from, let's say from New York to Paris, this is the example I use all the time and I'm sitting in my office at NYU.
例えば、ニューヨークからパリに行きたいとします。私はいつもこの例を使って、NYUのオフィスに座っています。
My objective that I need to minimize is my distance to Paris.
最小化する必要がある目標は、パリまでの距離です。
At a high level, a very abstract representation of my location, I would have to decompose this into two sub-goals.
私の位置の非常に抽象的な表現で、これを2つのサブゴールに分解する必要があります。
First one is go to the airport.
最初は空港に行くことです。
Second one is catch a plane to Paris.
2つ目はパリ行きの飛行機に乗ることです。
Okay, so my sub-goal is now going to the airport.
では、今私のサブゴールは空港に行くことです。
My objective function is my distance to the airport.
私の目的関数は空港までの距離です。
How do I go to the airport?
どうやって空港に行けばいいのでしょうか?
Well I have to go in the street and hail a taxi, which you can do in New York.
タクシーを拾うためには、通りに出て手を挙げなければなりません。ニューヨークではそれができます。
Okay, now I have another sub-goal.
さて、別のサブゴールがあります。
How do I go down on the street?
通りに降りるにはどうすればいいですか?
Well that means going to the elevator, going down the elevator, walk out the street.
それはエレベーターに乗ることを意味します。エレベーターで下り、通りに出ることです。
How do I go to the elevator?
エレベーターに行くにはどうすればいいですか?
I have to stand up for my chair, open the door in my office, go to the elevator, push the button.
椅子から立ち上がり、オフィスのドアを開けて、エレベーターに行き、ボタンを押す必要があります。
How do I get up from my chair?
椅子から立ち上がるにはどうすればいいですか?
Like you can imagine going down all the way down to basically what amounts to millisecond by millisecond muscle control.
基本的には、ミリ秒単位の筋肉制御にほぼ等しいものまで行くことを想像できます。
Obviously, you're not going to plan your entire trip from New York to Paris in terms of millisecond by millisecond muscle control.
明らかに、ニューヨークからパリまでの旅行をミリ秒単位の筋肉の制御に基づいて計画することはありません。
First, that would be incredibly expensive, but it will also be completely impossible because you don't know all the conditions of what's going to happen, how long it's going to take to catch a taxi or to go to the airport with traffic.
まず第一に、それは非常に高価になるでしょうが、何が起こるか、タクシーを捕まえるのにどれくらい時間がかかるかなど、すべての状況を把握していないため、完全に不可能です。
I mean, you would have to know exactly the condition of everything to be able to do this planning and you don't have the information.
あなたはこの計画を立てるためにはすべての状況を正確に把握していなければならず、情報が不足しています。
You have to do this hierarchical planning so that you can start acting.
行動を開始できるように、階層的な計画を立てる必要があります。
And then sort of replanning as you go.
そして、進行しながら再計画を行う必要があります。
Nobody really knows how to do this in AI.
AIにおいてこれをどうやればいいのか、誰も本当のところわかっていません。
Nobody knows how to train a system to learn the appropriate multiple levels of representation so that hierarchical planning works.
階層的な計画が機能するように、システムに適切な複数の表現レベルを学習させる方法を誰も知りません。
Lex is going to press Jan about scaling because what we've seen with the ability to scale Large Language Models is truly amazing.
レックスは、大規模言語モデルのスケーリングについてジャンに質問するつもりです。なぜなら、大規模言語モデルのスケーリング能力は本当に驚くべきものだからです。
We've gone from GPT one, two, three, now we're at four, almost at five, and the increase in parameters, the increase in GPU processing power has unleashed incredible capabilities from these Large Language Models.
GPT1、2、3から4に進み、もうすぐ5になり、パラメータの増加、GPU処理能力の向上により、これらの大規模言語モデルから信じられないほどの能力が引き出されています。
You have Sora and Sora approved.
あなたはSoraとSoraの承認を受けています。
If you increase compute, you can get much better results.
計算を増やすと、はるかに良い結果を得ることができます。
Lex really tries to press Jan about, hey, if we keep scaling up, can't we reach super intelligence with our current technologies?
レックスは、もし私たちがスケールを拡大し続ければ、現在の技術で超知能に到達できるのではないかとジャンに本気で問い詰めようとしていますか?
And he also asked what Alan Turing would think about current AI.
そして、アラン・チューリングが現在のAIについてどう思うかも尋ねました。
And if you're not familiar with Alan Turing, he created the Turing test amongst many other things and the Turing test is simply a test to see if artificial intelligence is truly intelligent.
もしアラン・チューリングについてよく知らないなら、彼は他にもたくさんのことを生み出した人であり、チューリング・テストを作り出した人物です。そして、チューリング・テストとは、単純に人工知能が本当に知的であるかどうかを見るためのテストです。
And all it really needs to do is convince a human that it is human.
それが本当に必要なのは、人間を説得して自分が人間であると思わせることだけです。
And with our current technology, that's very possible, especially if you just do it over text.
そして、現在の技術では、それは非常に可能です。特に、テキストだけで行う場合は。
But even with voice, it's possible.
しかし、声でも可能です。
Jan says something really funny about it.
ジャンはそれについて本当に面白いことを言いました。
I'm not going to spoil that joke.
そのジョークを台無しにするつもりはありません。
Take a look.
見てみてください。
Does it make sense to you that they're able to form enough of a representation of the world to be damn convincing, essentially passing the original Turing test with flying colors?
彼らが世界の十分な表現を形成できるだけの能力を持っており、実質的にオリジナルのチューリング・テストを見事に突破しているということが理解できますか?
Yeah, we're fooled by their fluency, right?
そうですね、私たちは彼らの流暢さに騙されているんですよね?
We just assume that if a system is fluent in manipulating language, then it has all the characteristics of human intelligence.
私たちは、もしシステムが言語を操作するのに堪能であれば、それが人間の知能のすべての特性を持っていると仮定しています。
But that impression is false.
しかし、その印象は誤りです。
We're really fooled by it.
私たちは本当にそれに騙されています。
What do you think Alan Turing would say?
あなたはアラン・チューリングが何と言うと思いますか?
Without understanding anything, just hanging out with it?
何も理解せずにただそれと一緒にいるだけですか?
Alan Turing would decide that the Turing test is a really bad test.
アラン・チューリングはチューリング・テストが本当に悪いテストであると判断するでしょう。
This is what the AI community has decided many years ago, that the Turing test was a really bad test of intelligence.
これはAIコミュニティが多くの年前に決定したことで、チューリング・テストは知能の本当に悪いテストだったということです。
Next Jan discusses hallucinations.
次にジャンは幻覚について議論します。
Why do they happen?
なぜそれが起こるのでしょうか?
Why are they such a big limitation for current Large Language Models?
なぜそれらは現在の大規模言語モデルにとって大きな制限となるのでしょうか?
And it is a huge limitation.
そして、それは非常に大きな制限です。
It's probably one of the biggest limitations to it being widely adopted in the corporate setting.
それが企業の環境で広く採用されることの最大の制限の1つである可能性があります。
There are a lot of techniques to prevent hallucinations, but let's find out why they actually occur.
幻覚を防ぐための多くの技術がありますが、なぜ実際にそれらが発生するのかを見つけましょう。
Because of the autoregressive prediction, every time an algorithm produces a token or word, there is some level of probability for that word to take you out of the set of reasonable answers.
自己回帰予測のため、アルゴリズムがトークンや単語を生成するたびに、その単語が妥当な回答のセットから外れる可能性があるレベルがあります。
And if you assume, which is a very strong assumption, that the probability of such error is that those errors are independent across a sequence of tokens being produced.
そして、そのようなエラーの確率が、生成されるトークンのシーケンス全体で独立しているという非常に強い仮定をするとします。
What that means is that every time you produce a token, the probability that you stay within the set of correct answer decreases and it decreases exponentially.
それが意味するのは、トークンを生成するたびに、正しい回答のセット内にとどまる確率が減少し、指数関数的に減少するということです。
If there's a non-zero probability of making a mistake, which there appears to be, then there's going to be a kind of drift.
間違いをする確率がゼロでない場合、そしてそうした確率があるようであれば、ある種のドリフトが生じることになります。
And that drift is exponential.
そしてそのドリフトは指数関数的です。
It's like errors accumulate.
エラーが蓄積されるようなものです。
The probability that an answer would be nonsensical increases exponentially with the number of tokens.
確率論的には、トークンの数が増えると、答えがナンセンスになる可能性が指数関数的に増加します。
This next clip is very interesting.
この次のクリップは非常に興味深いです。
Yann talks about why he doesn't like reinforcement learning, or at least why reinforcement learning is very good, but only for a very narrow use case.
Yannは、なぜ強化学習が好きではないのか、あるいは少なくとも強化学習が非常に優れているが、非常に狭い用途にしか適していないと考えているかについて話しています。
Let's watch.
見ましょう。
Why do you still hate reinforcement learning?
なぜまだ強化学習を嫌っているのですか?
I don't hate reinforcement learning and I think it should not be abandoned completely, but I think its use should be minimized because it's incredibly inefficient in terms of samples.
私は強化学習を嫌っているわけではなく、完全に放棄すべきではないと思いますが、サンプルの観点から非常に効率が悪いと考えていますので、その使用を最小限に抑えるべきだと思います。
The proper way to train a system is to first have it learn good representations of the world and world models from mostly observation, maybe a little bit of interactions.
システムを適切に訓練する方法は、まず、それに世界の良い表現と世界モデルを主に観察から学ばせることであり、少しの相互作用を含めるかもしれません。
There's two things.
2つのことがあります。
You can use, if you've learned a world model, you can use the world model to plan a sequence of actions to arrive at a particular objective.
もし世界モデルを学習したなら、その世界モデルを使用して特定の目的地に到達するための一連のアクションを計画することができます。
You don't need RL unless the way you measure whether you succeed might be inexact.
成功をどのように測定するかが不正確である場合を除いては、目的を達成する方法に強化学習は必要ありません。
Your idea of whether you were going to fall from your bike might be wrong, or whether the person you're fighting with MMA was going to do something.
あなたが自転車から転ぶかもしれないと思っていたか、あるいはMMAで戦っている相手が何かをするかもしれないかどうかは、あなたの考え方が間違っているかもしれません。
And then do something else.
そして、他のことをする。
There's two ways you can be wrong.
間違っている方法は2つあります。
Either your objective function does not reflect the actual objective function you want to optimize, or your world model is inaccurate.
あなたの目的関数が、実際に最適化したい目的関数を反映していないか、あるいはあなたの世界モデルが不正確であるかのどちらかです。
The prediction you were making about what was going to happen in the world is inaccurate.
あなたが世界で起こることについて予測していたことは不正確です。
If you want to adjust your world model while you are operating in the world or your objective function, that is basically in the realm of RL.
世界で操作している間に世界モデルを調整したい場合、あるいは目的関数を調整したい場合、それは基本的にRLの範囲内です。
This is what RL deals with to some extent, right?
これは、ある程度RLが扱う内容ですね。
Adjust your world model.
あなたの世界モデルを調整してください。
And the way to adjust your world model, even in advance, is to explore parts of the space where your world model, where you know that your world model is inaccurate.
そして、あなたの世界モデルが不正確であることがわかっている空間の一部を探索することで、あなたの世界モデルを調整する方法は、事前にも可能です。
That's called curiosity, basically, or play.
それは基本的に好奇心または遊びと呼ばれます。
When you play, you kind of explore parts of the state space that you don't want to do in for real because it might be dangerous, but you can adjust your world model without killing yourself, basically.
あなたがプレイするとき、実際には危険かもしれない状態空間の一部を探索することがありますが、自分を殺さずに世界モデルを調整することができます。
That's what you want to use RL for.
それがRLを使用したい理由です。
When it comes time to learning a particular task, you already have all the good representations.
特定のタスクを学ぶ時には、既に良い表現がすべて揃っています。
You already have your world model, but you need to adjust it for the situation at hand.
既に世界モデルを持っていますが、その状況に合わせて調整する必要があります。
That's when you use RL.
その時にRLを使用します。
To the part that I'm truly excited about.
私が本当に興奮している部分について。
Jan talks about why open source is so important.
ジャンはなぜオープンソースが重要なのかについて話します。
He references Gemini 1.5's wokeness.
彼はGemini 1.5の目覚めを参照しています。
He references LLM biases and why open source models are the answer to all of it.
大規模言語モデルの偏見を参照し、なぜオープンソースモデルがそのすべての答えであるかを説明しています。
And I tend to agree with much of what he says.
彼が言うことの大部分に同意する傾向があります。
Let's take a look.
見てみましょう。
A lot of people have been very critical of the recently released Google's Gemini 1.5 for essentially, in my words, I could say super woke, woke in the negative connotation of that word.
最近リリースされたGoogleのGemini 1.5について、多くの人々が非常に批判的であり、私の言葉で言えば、その言葉の否定的な意味でスーパーウォーク、ウォークと言えるでしょう。
There's some almost hilariously absurd things that it does, like it modifies history, generating images of a black George Washington, or perhaps more seriously, something that you commented on Twitter, which is refusing to comment on or generate images of, or even descriptions of Tiananmen Square or the Tank Man, one of the most sort of legendary protest images in history.
それはほとんど滑稽にも、歴史を改変し、黒人のジョージ・ワシントンの画像を生成したり、もっと深刻なことに、あなたがTwitterでコメントしたように、天安門広場や戦車男の画像や説明をコメントしない、生成しないということがあります。これは、歴史上最も伝説的な抗議の画像の1つです。
And of course, these images are highly censored by the Chinese government.
そしてもちろん、これらの画像は中国政府によって厳しく検閲されています。
And therefore, everybody started asking questions of what is the process of designing these LLMs?
そのため、誰もがこれらの大規模言語モデルの設計プロセスについて質問し始めました。
What is the role of censorship in these?
これらにおける検閲の役割は何ですか?
You commented on Twitter saying that open source is the answer.
あなたはTwitterで、オープンソースが答えだとコメントしました。
Can you explain?
説明してもらえますか?
I actually made that comment on just about every social network I can, and I've made that point multiple times in various forums.
実際、私はほぼすべてのソーシャルネットワークでそのコメントをし、さまざまなフォーラムで何度もそのポイントを述べてきました。
Here's my point of view on this.
これについて私の見解を述べます。
People can complain that AI systems are biased, and they generally are biased by the distribution of the training data that they've been trained on that reflects biases in society, and that is potentially offensive to some people or potentially not.
人々は、AIシステムが偏っていると不満を言うことができます。一般的に、彼らは社会の偏見を反映したトレーニングデータの分布によって偏っており、それは一部の人々にとって潜在的に攻撃的である可能性があります。
And some techniques to de-bias then become offensive to some people because of historical incorrectness and things like that.
そして、一部の人々にとって、偏りを取り除くためのいくつかの技術は、歴史的な不正確さなどの理由で攻撃的になることがあります。
You can ask the question, you can ask two questions.
質問をすることができます。2つの質問をすることができます。
The first question is, is it possible to produce an AI system that is not biased?
最初の質問は、偏りのないAIシステムを作成することは可能かということです。
And the answer is absolutely not.
答えは絶対に不可能です。
And it's not because of technological challenges, although there are technological challenges to that.
それは技術的な課題のためではなく、技術的な課題はあるにしてもです。
It's because bias is in the eye of the beholder, different ideas about what constitutes bias for a lot of things.
それは、偏見は見る人の目によって異なり、多くのことにおいて何が偏見であるかについて異なる考えがあるからです。
I mean, there are facts that are indisputable, but there are a lot of opinions or things that can be expressed in different ways.
つまり、議論の余地のない事実がある一方で、異なる方法で表現されることができる意見や事柄がたくさんあります。
You cannot have an unbiased system that's just an impossibility.
偏見のないシステムを持つことは不可能です。
What's the answer to this?
これの答えは何ですか?
And the answer is the same answer that we found in liberal democracy about the press.
そして答えは、リベラルな民主主義において報道について見つけた答えと同じです。
The press needs to be free and diverse.
報道は自由で多様である必要があります。
We have free speech for a good reason.
私たちには言論の自由があるのは重要な理由があるからです。
It's because we don't want all of our information to come from a unique source, because that's opposite to the whole idea of democracy and progress of ideas and even science.
それは、私たちの情報がすべて単一の情報源から来ることを望まないからです。なぜなら、それは民主主義やアイデアの進歩、さらには科学の全体的な考え方に反するからです。
In science, people have to argue for different opinions, and science makes progress when people disagree and they come up with an answer and a consensus forms.
科学では、人々は異なる意見を主張しなければならず、人々が異論を唱え、答えを見つけ、合意形成がされると科学は進歩します。
And it's true in all democracies around the world.
そしてそれは世界中のすべての民主主義に当てはまります。
There is a future is already happening where every single one of our interaction with the digital world will be mediated by AI systems.
すでにAIシステムによって仲介されるデジタル世界とのすべてのやり取りが起こる未来がすでに始まっています。
We're going to have smart glasses.
スマートグラスが登場します。
You can already buy them from Meta, the Ray-Ban Meta, where you can talk to them and they are connected with an LLM and you can get answers on any question you have.
すでにMetaから購入することができますが、Ray-Ban Metaと呼ばれ、それらと会話ができ、大規模言語モデルと接続されており、どんな質問にも答えを得ることができます。
Or you can be looking at a monument and there is a camera in the system that in the glasses, you can ask it like, what can you tell me about this building or this monument?
あるいは、記念碑を見ている時に、そのメガネのシステムにあるカメラに、この建物やこの記念碑について何か教えてくれるかと尋ねることができます。
You can be looking at a menu in a foreign language and it will translate it for you or you can do real-time translation if you speak different languages.
外国語のメニューを見ていると、それを翻訳してくれたり、異なる言語を話す場合にリアルタイムで翻訳してくれます。
A lot of our interaction with the digital world are going to be mediated by those systems in the near future.
私たちとデジタル世界との多くのやり取りは、近い将来、それらのシステムによって仲介されることになるでしょう。
Increasingly, the search engines that we're going to use are not going to be search engines.
今後利用する検索エンジンは、ますます検索エンジンではなくなるでしょう。
They're going to be dialogue systems where you just ask a question and it will answer.
質問をするだけで答えてくれる対話システムになるでしょう。
And then point you to perhaps an appropriate reference for it.
そして、おそらくそれに適した参照先を指し示します。
But here is the thing.
しかし、ここが重要な点です。
We cannot afford those systems to come from a handful of companies on the west coast of the US because those systems will constitute the repository of all human knowledge and we cannot have that be controlled by a small number of people.
これらのシステムが米国西海岸の数社から提供されることは許されません。なぜなら、これらのシステムはすべての人類の知識の保管庫を構成し、それを少数の人々によって制御されることはできないからです。
It has to be diverse.
それは多様でなければなりません。
For the same reason, the press has to be diverse.
同じ理由で、報道も多様でなければなりません。
How do we get a diverse set of AI assistants?
どのようにして多様なAIアシスタントを手に入れるのか?
It's very expensive and difficult to train a base model, a base LLM at the moment.
現時点では、ベースモデル、ベース大規模言語モデルを訓練するのは非常に高価で難しいです。
In the future, it might be something different, but at the moment, that's an LLM.
将来は違うかもしれませんが、現時点ではそれが大規模言語モデルです。
Only a few companies can do this properly.
これを適切に行える企業はほんの一握りです。
If some of those top systems are open source, anybody can use them.
もしそれらのトップシステムのいくつかがオープンソースであれば、誰でもそれを利用できます。
We can fine tune them.
私たちはそれらを微調整することができます。
If we put in place some systems that allows any group of people, whether they are individual citizens, groups of citizens, government organizations, NGOs, companies, whatever, to take those open source systems, AI systems, and fine tune them for their own purpose on their own data, then we're going to have a very large diversity of different AI systems that are specialized for all of those things.
個々の市民、市民グループ、政府機関、NGO、企業など、どんなグループでも、それらのオープンソースシステム、AIシステムを取り上げ、自分たちの目的に合わせて自分たちのデータで微調整することができるシステムを導入すれば、さまざまな専門用途に特化した異なるAIシステムが非常に多く存在することになります。
Right.
そうですね。
I'll tell you, I talked to the French government quite a bit and the French government will not accept that the digital diet of all their citizens be controlled by three companies on the west coast of the U.S. That's just not acceptable.
実際、フランス政府とかなり話し合いましたが、フランス政府は、全ての市民のデジタルデータが米国西海岸の3社によって制御されることを受け入れません。それは許容できません。
It's a danger to democracy, regardless of how well-intentioned those companies are.
それは、それらの企業がどれだけ善意を持っていても、民主主義にとって危険です。
And it's also a danger to local culture, to values, to language.
および、地元の文化、価値観、言語にも危険です。
I was talking with the founder of Infosys in India.
私はインドのInfosysの創設者と話していました。
He's funding a project to fine tune Lama2, the open source model produced by Meta, so that Lama2 speaks all 22 official languages in India.
彼は、Metaが生産したオープンソースモデルであるLama2を調整するプロジェクトを資金提供しており、Lama2がインドの22の公用語をすべて話すようにしています。
I mean, you can't have any of this unless you have open source platforms.
つまり、オープンソースプラットフォームがなければ、これらのどれも持つことはできません。
With open source platforms, you can have AI systems that are not only diverse in terms of political opinions or things of that type, but in terms of language, culture, value systems, political opinions, technical abilities in various domains.
オープンソースプラットフォームを使用すると、政治的意見やそのようなものに関してだけでなく、言語、文化、価値観、政治的意見、技術的能力など、多様性に富んだAIシステムを持つことができます。
And you can have an industry, an ecosystem of companies that fine tune those open source systems for vertical applications in industry, right?
そして、業界や企業のエコシステムが、これらのオープンソースシステムを業界向けの垂直アプリケーションに調整することができますね。
You have, I don't know, a publisher has thousands of books, and they want to build a system that allows a customer to just ask a question about the content of any of their books.
出版社が何千冊もの本を持っていて、顧客が自分たちの本の内容について質問するだけできるシステムを構築したいとします。
You need to train on their proprietary data.
彼らの独自のデータでトレーニングする必要があります。
You have a company, we have one within Meta, it's called Metamate.
Meta内にも、Metamateと呼ばれる会社があります。
And it's basically an LLM that can answer any question about internal stuff about the company.
あなたは記念碑を見ているかもしれませんが、システムにカメラがあり、メガネの中には、この建物や記念碑について何が言えるか尋ねることができます。
Very useful.
非常に便利です。
A lot of companies want this, right?
多くの企業がこれを望んでいますね?
A lot of companies want this not just for their employees, but also for their customers, to take care of their customers.
多くの企業は、従業員だけでなく、顧客のためにもこれを望んでいます。顧客を世話するために。
The only way you're going to have an AI industry, the only way you're going to have AI systems that are not uniquely biased is if you have open source platforms on top of which any group can build virtualized systems.
唯一の方法は、AI業界を持つ唯一の方法は、独自にバイアスのかかっていないAIシステムを持つ唯一の方法は、どのグループも仮想化システムを構築できるオープンソースプラットフォームの上に構築されることです。
The direction of inevitable direction of history is that the vast majority of AI systems will be built on top of open source platforms.
歴史の必然的な方向性は、AIシステムの大部分がオープンソースプラットフォームの上に構築されるということです。
And on the topic of open source, Lex asked him, okay, if it's open source, how do you actually run a business based on open source?
そしてオープンソースの話題について、レックスは彼に尋ねました。「オープンソースなら、実際にどのようにしてオープンソースに基づいたビジネスを運営するのですか?」
What are the economics of open source?
オープンソースの経済とは何ですか?
And this has been proven over the years.
これは何年もの間証明されてきました。
We have a lot of very successful open source projects that have made a lot of money.
私たちの多くの非常に成功したオープンソースプロジェクトがたくさんのお金を稼いでいます。
Many of the standards that we use on the internet today, many of the standards that we use with databases, with code architecture, everything, a lot of that is open source.
今日インターネットで使用している多くの標準、データベースやコードアーキテクチャで使用している多くの標準の多くはオープンソースです。
And there are a lot of benefits with open source and a lot of companies have made a lot of money with open source.
オープンソースには多くの利点があり、多くの企業がオープンソースで多くのお金を稼いでいます。
Just look at Android for Google, just as an example off the top of my head.
たとえば、GoogleのAndroidを見てください、ただ頭の中で思いついた例として。
Let's see what Jan says about the economics of Meta's open source contributions.
ジャンがMetaのオープンソース貢献の経済について何と言うか見てみましょう。
You have several business models, right?
いくつかのビジネスモデルがありますね。
The business model that Meta is built around is your first service.
Metaが構築されているビジネスモデルは最初のサービスです。
And the financing of that service is either through ads or through business customers.
そのサービスの資金調達は広告またはビジネス顧客を通じて行われます。
For example, if you have an LLM that can help a mom and pop pizza place by talking to their customers through WhatsApp.
たとえば、マム&ポップのピザ屋さんが顧客とWhatsAppを通じて話すことで役立つ大規模言語モデルを持っている場合。
The customers can order a pizza and the system will just ask them like, what topping do you want or what size, blah, blah, blah.
顧客はピザを注文し、システムは単に彼らに尋ねるだけです、どんなトッピングが欲しいか、どんなサイズがいいか、などなど。
The business will pay for that.
そのビジネスはそのために支払います。
And otherwise if it's a system that is on the more kind of classical services, it can be ad supported or the several models.
もしもそれがもっと古典的なサービスのシステムである場合、広告支援されるか、いくつかのモデルになる可能性があります。
But the point is, if you have a big enough potential customer base and you need to build that system anyway for them, it doesn't hurt you to actually distribute it in open source.
しかし、ポイントは、十分な潜在顧客基盤がある場合、それらのためにシステムを構築する必要があり、実際にオープンソースで配布しても問題ありません。
And then Lex continues to press them about the open source economics.
そして、レックスはオープンソースの経済について彼らを追及し続けます。
Why doesn't another company just come along, take that open source project and build competition?
なぜ他の企業が単にそのオープンソースプロジェクトを取り、競争を構築しないのですか?
That probably will happen and that's probably good.
おそらくそれは起こるでしょうし、それはおそらく良いことです。
But at the end of the day, Meta is still Meta.
しかし、最終的には、メタはやはりメタです。
They have an entire multi-billion human user base to sell their products and services to.
彼らには何十億ものユーザーがいて、その製品やサービスを販売できる顧客基盤全体があります。
Let's see what he says.
彼が何と言うか見てみましょう。
The bet is more, we already have a huge user base and customer base, right?
賭けは、すでに巨大なユーザーベースと顧客基盤を持っているということですね?
It's going to be useful to them.
それは彼らにとって役立つでしょう。
Whatever we offer them is going to be useful and there is a way to derive revenue from this.
提供するものはどれも役立つものであり、これから収益を得る方法がある。
It doesn't hurt that we provide that system or the base model, right, the foundation model in open source for others to build applications on top of it too.
私たちがそのシステムや基本モデル、つまりオープンソースで他の人がアプリケーションを構築するための基礎モデルを提供していることは悪いことではないですよね。
If those applications turn out to be useful for our customers, we can just buy it from them.
もしそれらのアプリケーションが私たちの顧客にとって役立つものであれば、ただそれを彼らから買うことができます。
It could be that they will improve the platform.
彼らがプラットフォームを改善する可能性もあります。
In fact, we see this already.
実際、私たちは既にそれを見ています。
I mean, there is literally millions of downloads of LLaMA 2 and thousands of people who have provided ideas about how to make it better.
つまり、LLaMA 2のダウンロードは文字通り何百万回あり、それをより良くするアイデアを提供してくれた何千人もの人がいます。
This clearly accelerates progress to make the system available to a wide community of people and there is literally thousands of businesses who are building applications with it.
これは明らかに、システムを広範な人々に利用可能にするための進展を加速させ、それを使ってアプリケーションを構築している何千もの企業がいます。
Meta's ability to derive revenue from this technology is not impaired by the distribution of it, of base models in open source.
Metaはこの技術から収益を得る能力が、オープンソースで基本モデルを配布することによって損なわれることはありません。
We continue on the whole biases front.
私たちは全体的な偏見の問題について続けます。
Many employees at tech companies tend to lean left and that is a point that Lex makes and I tend to agree with. And so aren't those biases going to be built into Large Language Models?
テック企業の多くの従業員は左傾向に傾きがちで、それはレックスが指摘している点であり、私も同意する傾向があります。ですから、それらの偏見は大規模言語モデルに組み込まれることになるのではないでしょうか?
And again, Jan points to open source being the answer.
そして、再び、ジャンはオープンソースが答えであると指摘しています。
I don't think the issue has to do with the political leaning of the people designing those systems.
私は、それらのシステムを設計する人々の政治的傾向に問題があるとは思いません。
It has to do with the acceptability or political leanings of their customer base or audience.
それは、彼らの顧客層や観客の受け入れ可能性や政治的傾向に関係があるのです。
A big company cannot afford to offend too many people.
大企業はあまり多くの人々を怒らせる余裕はありません。
They're going to make sure that whatever product they put out is safe, whatever that means.
彼らは、どんな製品を出すにせよ、それが安全であることを確認するでしょう、それが何を意味するにせよ。
It's very possible to overdo it.
それをやりすぎる可能性は非常に高いです。
And it's also very possible to, it's impossible to do it properly for everyone.
そして、それを適切に行うことは不可能である可能性も非常に高いです。
You're not going to satisfy everyone.
あなたは誰も満足させることはできません。
That's what I said before.
それが私が以前言ったことです。
You cannot have a system that is unbiased, that is perceived as unbiased by everyone.
誰からも偏見のないと認識されるシステムを持つことはできません。
It's going to be you push it in one way, one set of people are going to see it as biased
一方向に押すと、ある一部の人々はそれを偏ったものと見るでしょう。
And then you push it the other way and another set of people is going to see it as biased.
そして逆方向に押すと、別の一部の人々はそれを偏ったものと見るでしょう。
And then in addition to this, there's the issue of if you push the system, perhaps you go too far in one direction, it's going to be non-factual, right?
そして、さらに、もしシステムを押し進めると、一方向に過度に行き過ぎる可能性があり、事実とは異なることになるでしょうね。
You're going to have black Nazi soldiers in the image.
画像には黒人のナチ兵士が含まれることになります。
Yeah, so we should mention image generation of black Nazi soldiers, which is not factually accurate.
そうですね、事実と異なる黒人のナチ兵士の画像生成を言及すべきです。
Right.
そうですね。
And can be offensive for some people as well, right?
また、一部の人々にとっては攻撃的なものになる可能性もありますね。
It's going to be impossible to kind of produce systems that are unbiased for everyone.
全ての人にとって偏りのないシステムを作ることは不可能でしょう。
The only solution that I see is diversity.
私が見る唯一の解決策は多様性です。
And diversity in the full meaning of that word, diversity in every possible way.
その言葉の真の意味での多様性、ありとあらゆる面での多様性です。
And continuing on the open source front, now they're going to talk about free speech, guardrails on AI, censorship, biases and everything.
そしてオープンソースの分野で続けて、今度は言論の自由、AIへのガードレール、検閲、偏見などについて話す予定です。
I love this conversation.
この会話が大好きです。
And hearing Jan frame open source in this light makes a ton of sense to me.
そして、ジャンがオープンソースをこのように捉えるのを聞くと、私にはとても理にかなっています。
Let's watch.
見ましょう。
I mean, there are some limits to what the same way there are limits to free speech, there has to be some limit to the kind of stuff that those systems might be authorized to produce some guardrails.
同じように、言論の自由には限界があるように、それらのシステムが生産を許可される種類のものには何らかの制限が必要です。
I mean, that's one thing I've been interested in, which is in the type of architecture that before, where the output of a system is a result of an inference to satisfy an objective.
私が興味を持っているのは、以前のようなアーキテクチャのタイプで、システムの出力が目的を満たす推論の結果であるという点です。
That objective can include guardrails and we can put guardrails in open source systems.
その目的にはガードレールを含めることができ、オープンソースシステムにもガードレールを設置することができます。
I mean, if we eventually have systems that are built with this blueprint, we can put guardrails in those systems that guarantee that there is sort of a minimum set of guardrails that make the system non-dangerous and non-toxic, et cetera basic things that everybody would agree on.
つまり、最終的にこの設計図で構築されたシステムがあれば、そのシステムには危険や有害性を排除する最低限のガードレールが保証されるようなガードレールを設置することができます。誰もが同意する基本的なことなどです。
And then the fine tuning that people will add or the additional guardrails that people will add will kind of cater to their community, whatever it is.
そして、人々が追加する微調整や追加のガードレールは、彼らのコミュニティに合わせるようになるでしょう。
Next, if you wanted a little preview of when LLaMA three is coming and what it's going to be about, this is the clip for you.
次に、もしLLaMA threeがいつリリースされるか、そして内容がどうなるか少しプレビューしたい場合は、この動画がおすすめです。
Let's watch.
一緒に見ましょう。
There's going to be like various versions of LLaMA that are improvements of previous LLaMAs, bigger, better, multimodal, things like that.
LLaMAのさまざまなバージョンがあり、以前のLLaMAを改良したものや、より大きく、より良く、マルチモーダルなものなどがあります。
And then in future generations, systems that are capable of planning that really understand how the world works.
そして将来の世代では、世界がどのように機能するかを本当に理解して計画できるシステムがあります。
Maybe are trained from video.
おそらくビデオから訓練されています。
They have some world model, maybe capable of the type of reasoning and planning I was talking about earlier.
彼らはいくつかの世界モデルを持っていて、おそらく私が以前に話した推論や計画のタイプが可能です。
Like, how long is that going to take?
それにはどれくらい時間がかかるのでしょうか?
Like when is the research that is going in that direction going to sort of feed into the product line, if you want, of LLaMA?
その方向に進んでいる研究がLLaMAの製品ラインにどのように反映されるのか、いつになるのでしょうか?
I don't know.
分かりません。
I can't tell you.
お伝えできません。
And there is a few breakthroughs that we have to basically go through before we can get there.
そこに到達する前に、基本的に通過しなければならないいくつかの突破口があります。
You'll be able to monitor our progress because we publish our research, right?
私たちの研究は公開されているので、進捗状況を監視することができますね?
Last week we published the VJEPA work, which is sort of a first step towards training systems from video.
先週、ビデオからシステムをトレーニングするための第一歩となるVJEPAの作業を公開しました。
And then the next step is going to be world models based on kind of this type of idea, training from video.
そして次のステップは、この種のアイデアに基づくワールドモデルで、ビデオからトレーニングすることになります。
There's similar work at DeepMind also, and taking place, people, and also at UC Berkeley on world models from video.
DeepMindでも同様の作業が行われており、ビデオからのワールドモデルに関する研究がUCバークレーでも行われています。
A lot of people are working on this.
多くの人々がこの取り組みに取り組んでいます。
I think a lot of good ideas are appearing.
多くの良いアイデアが出てきていると思います。
My bet is that those systems are going to be JEPA-like, they're not going to be generative models.
私の予想では、これらのシステムはJEPAのようなものになると思います。生成モデルではなくなります。
And we'll see what the future will tell.
そして将来がどうなるか見守りたいと思います。
There's really good work at a gentleman called Daniel Haffner, who is now at DeepMind, who has worked on kind of models of this type that learn representations
DeepMindにいるダニエル・ハフナー氏という方が、この種のモデルに取り組んでおり、非常に優れた研究が行われています。
And then use them for planning or learning tasks by reinforcement training.
そして、それらを強化学習によって計画や学習の課題に使用します。
Going back a few topics, they're going to talk about the power and efficiency in the human mind to process all of this data and what we get from it compared to Large Language Models.
数つ前に戻って、彼らは人間の心がこのすべてのデータを処理し、それから得られるものを処理する力と効率について話す予定です。
And it's really not even a comparison.
そして、実際には比較になりません。
Large language models require a ton of data, a ton of processing power, a ton of energy to train and to use versus a human brain, which is incredibly efficient.
大規模言語モデルは、訓練および使用するために膨大なデータ、膨大な処理能力、膨大なエネルギーを必要としますが、それに対して人間の脳は信じられないほど効率的です。
We're still far in terms of compute power from what we would need to match the compute power of the human brain.
私たちは、計算能力の面で、人間の脳の計算能力に匹敵するために必要なものからはまだ遠く離れています。
This may occur in the next couple of decades, but we're still some ways away.
これは、おそらく次の数十年の間に起こるかもしれませんが、まだまだ道のりは遠いです。
And certainly in terms of power efficiency, we're really far.
そして、電力効率の面でも、本当に遠いです。
A lot of progress to make in hardware.
ハードウェアで進展する余地がたくさんあります。
Right now, a lot of the progress is a bit coming from silicon technology, but a lot of it coming from architectural innovation and quite a bit coming from more efficient ways of implementing the architectures that have become popular, basically a combination of transformers and components.
現在、進歩の多くはシリコン技術から来ていますが、それに加えて、アーキテクチャの革新からもかなりの進歩があり、基本的にはトランスフォーマーとコンポーネントの組み合わせによるより効率的なアーキテクチャの実装方法からも多くの進歩があります。
There's still some ways to go until we're going to saturate, we're going to have to come up with new principles, new fabrication technology, new basic components, perhaps on sort of different principles than those classical digital CMOS.
まだまだ進むべき道があり、新しい原則、新しい製造技術、古典的なデジタルCMOSとは異なる原則に基づく基本的なコンポーネントを考え出さなければならない時が来るでしょう。
And next, they're going to start talking about AGI.
次に、彼らはAGIについて話し始める予定です。
When is it coming?
それはいつ来るのでしょうか?
And Jan has made this point over and over again.
ジャンは何度も同じことを言ってきました。
A lot of people think AGI is just going to be some switch that is flipped one day, and it's just going to be this inflection point that AGI just has this quote unquote hard takeoff.
多くの人々は、AGIがある日突然スイッチが入るだけで、AGIがこのいわゆるハードテイクオフを持つだろうと考えています。
But he doesn't think that's the case.
しかし、彼はそうではないと考えています。
He thinks it's going to be progressive.
彼はそれが段階的に進むと考えています。
Let's see what he has to say.
彼が言うことを見てみましょう。
First of all, it's not going to be an event, right?
まず第一に、それはイベントになるわけではありませんね。
The idea somehow, which is popularized by science fiction and Hollywood, that somehow somebody is going to discover the secret, the secret to AGI or human level AI or AMI, whatever you want to call it, and then turn on a machine and then we have AGI.
科学小説やハリウッドで人気のある考え方は、何かが秘密を発見し、AGIや人間レベルのAIやAMIの秘密を発見し、機械を起動させると、AGIが完成するというものです。
That's just not going to happen.
それは起こらないでしょう。
It's not going to be an event.
イベントにはなりません。
It's going to be gradual progress.
徐々に進展していくことになります。
Are we going to have systems that can learn from video how the world works and learn good representation?
ビデオから世界がどのように機能するかを学び、適切な表現を学ぶことができるシステムを持つことになりますか?
Yeah, before we get them to the scale and performance that we observe in humans, it's going to take quite a while.
ええ、人間が観察するスケールやパフォーマンスにそれらを達成する前に、かなりの時間がかかるでしょう。
Are we going to get systems that can have large amount of associative memory so they can remember stuff?
大量の連想記憶を持つことができ、物事を覚えることができるシステムを手に入れることになりますか?
Yeah, but same.
ええ、でも同じです。
It's not going to happen tomorrow.
明日には起こらないでしょう。
I mean, there is some basic techniques that need to be developed.
いくつかの基本的な技術が開発される必要があります。
We have a lot of them, but to get this to work together with a full system is another story.
私たちは多くの技術を持っていますが、これを完全なシステムと一緒に機能させるには別の話です。
Are we going to have systems that can reason and plan perhaps along the lines of objective driven AI architectures that I described before?
目的志向のAIアーキテクチャの枠組みに沿って理論的に計画することができるシステムを持つことになりますか?
Yeah, but before we get this to work properly, it's going to take a while.
そうですが、これを正しく機能させるには、しばらく時間がかかります。
And before we get all those things to work together
そして、それらすべてをうまく連携させる前に
And then, on top of this, we have systems that can learn like hierarchical planning, hierarchical representation.
そして、さらに、階層的な計画、階層的な表現のように学習できるシステムがあります。
Systems that can be configured for a lot of different situations at hand, the way the human brain can.
手元のさまざまな状況に合わせて構成できるシステムは、人間の脳のように。
All of this is going to take at least a decade, and probably much more, because there are a lot of problems that we're not seeing right now.
これには少なくとも10年はかかるでしょうし、おそらくそれ以上かかるでしょう、なぜなら今見えていない問題がたくさんあるからです。
We have not encountered, and so we don't know if there is an easy solution within this framework.
私たちはまだ遭遇しておらず、この枠組みの中で簡単な解決策があるかどうかわかりません。
It's not just around the corner.
すぐには実現されません。
I mean, I've been hearing people for the last 12, 15 years claiming that AGI is just around the corner and being systematically wrong.
私は過去12、15年間、AGIがすぐそこにあると主張する人々を聞いてきましたが、彼らは体系的に間違っていました。
And I knew they were wrong when they were saying it.
そして、彼らが言っているときに彼らが間違っていることを知っていました。
I called their bullshit.
私は彼らの言っていることをくだらないと思いました。
And next, he talks about AI doomerism and what that actually means and good AI versus bad AI and how that whole thing is going to play out.
次に、彼はAIドゥーマリズムについて話し、それが実際に何を意味するのか、良いAIと悪いAI、そしてその全体がどのように進行するかについて話します。
Something that I'm very interested in.
私が非常に興味を持っているものです。
And I made a video about it recently because it is such an interesting topic to see the wide spectrum of people's beliefs about AGI and AI doom.
そして最近それについてビデオを作成しました。なぜなら、AGIやAIの終末についての人々の信念の幅広さを見るのは非常に興味深いトピックだからです。
Okay, so AI doomers imagine all kinds of catastrophe scenarios of how AI could escape or control and basically kill us all.
では、AIドゥーマーは、AIがどのようにして逃げ出したり制御したりして基本的に私たち全員を殺す可能性があるというさまざまな災害シナリオを想像しています。
And that relies on a whole bunch of assumptions that are mostly false.
そして、それは主に間違っているとされる多くの仮定に依存しています。
The first assumption is that the emergence of superintelligence could be an event.
最初の仮定は、超知能の出現がイベントである可能性があるということです。
That at some point we're going to Figure out the secret and we'll turn on a machine that is super intelligent.
いつか私たちは秘密を解明し、超知能の機械を起動させることができるということです。
And because we've never done it before, it's going to take over the world and kill us all.
そして、これまでやったことがないので、それが世界を支配し、私たち全員を殺すことになるというのです。
That is false.
それは間違っています。
It's not going to be an event.
それはイベントにはならないのです。
We're going to have systems that are like as smart as a cat, have all the characteristics of human level intelligence, but their level of intelligence would be like a cat or a parrot maybe or something.
猫のように賢いシステムができるようになるでしょう。人間レベルの知能を持ちながらも、その知能のレベルは猫やオウムのようなものになるかもしれません。
And then we're going to walk our way up to kind of make those things more intelligent.
そして、それらをより知能を持つように進化させていく予定です。
And as we make them more intelligent, we're also going to put some guardrails in them and learn how to kind of put some guardrails so they behave properly.
そして、それらをより知能を持つようにする一方で、適切に振る舞うためのガードレールを設ける方法を学んでいく予定です。
And we're not going to do this with just one, it's not going to be one effort, but it's going to be lots of different people doing this.
そして、これを1つだけで行うわけではなく、多くの異なる人々がこれを行うことになります。
And some of them are going to succeed at making intelligent systems that are controllable and safe and have the right guardrails.
そして、そのうちのいくつかは、制御可能で安全で適切なガードレールを持つ知能システムを作り出すことに成功するでしょう。
And if some other goes rogue, then we can use the good ones to go against the rogue ones.
もし他の何かが暴走した場合、良いものを使って暴走したものに対抗することができます。
It's going to be my smart AI police against your rogue AI.
私の賢いAI警察対あなたの暴走AIになるでしょう。
It's not going to be like we're going to be exposed to like a single rogue AI that's going to kill us all.
私たちは全員が殺されるような単一の暴走AIにさらされることはありません。
That's just not happening.
それは起こりません。
There is another fallacy, which is the fact that because the system is intelligent, it necessarily wants to take over.
また、システムが知能を持っているからといって、必ずしも支配しようとするというのは誤謬です。
And there are several arguments that make people scared of this, which I think are completely false as well.
このことについて人々を怖がらせるいくつかの議論がありますが、私はそれらが完全に間違っていると思います。
One of them is in nature, it seems to be that the more intelligent species are the ones that end up dominating the others, extinguishing the others, sometimes by design, sometimes just by mistake.
そのうちの1つは、自然界では、より知能の高い種が他の種を支配し、時には意図的に、時には単なる間違いで他の種を絶滅させる傾向があるように見えるということです。
There is sort of thinking by which you say, well, if AI systems are more intelligent than us, surely they're going to eliminate us, if not by design, simply because they don't care about us.
AIシステムが私たちよりも知能が高い場合、彼らが私たちを排除するだろうという考え方があります。それが意図的でなくても、単に私たちに興味がないからです。
And that's just preposterous for a number of reasons.
そして、それはいくつかの理由でばかげています。
The first reason is they're not going to be a species, they're not going to be a species that competes with us.
最初の理由は、彼らが私たちと競争する種であることはないということです。
They're not going to have the desire to dominate because the desire to dominate is something that has to be hardwired into an intelligent system.
彼らは支配する欲望を持たないでしょう、なぜなら支配する欲望は知的システムにハードワイヤードされる必要があるからです。
It is hardwired in humans.
それは人間にハードワイヤードされています。
It is hardwired in baboons, in chimpanzees, in wolves, not in orangutans.
それはオランウータンにはハードワイヤードされていませんが、ヒヒ、チンパンジー、オオカミにはハードワイヤードされています。
The species in which this desire to dominate or submit or attain status in other ways is specific to social species.
この支配したり服従したり他の方法で地位を得ようとする欲望が特定の社会的種に特有である種があります。
Non-social species like orangutans don't have it, right?
オランウータンのような非社会的種にはそれがないのですね。
And they are as smart as we are, almost.
彼らは私たちとほぼ同じくらい賢いです。
And then next, they tackle the comparison between AI, AGI, and nuclear systems.
そして次に、彼らはAI、AGI、および核システムの比較に取り組みます。
And there's been a lot of comparisons between nuclear and AI, and I don't necessarily agree that's an apples to apples comparison, and Jan tends to agree, and he explains why.
核とAIの間で多くの比較がされてきましたが、それが完全な比較ではないと私は必ずしも同意しないし、ヤンも同意する傾向にあり、彼はその理由を説明します。
But not only that, he explains why AI is essentially going to be our filter to the rest of the internet and to other AI models.
それだけでなく、彼はAIが本質的に私たちのインターネットの残りや他のAIモデルへのフィルターになる理由を説明します。
An AI model is not going to be able to directly communicate with us.
AIモデルは直接私たちとコミュニケーションを取ることはできません。
We're not even going to see it.
私たちはそれを見ることさえありません。
He actually brings up the analogy of a spam filter for email.
実際、彼はメールのスパムフィルターのたとえを持ち出します。
We get a ton of spam, and most of which we never see and we never need to see.
私たちは大量のスパムを受け取りますが、そのほとんどは見る必要もないし、見ることもありません。
It's interesting to think about, but it's also a little worrying to think that an AI is going to be my filter to all of my information diet.
考えるのは面白いですが、AIが私の情報摂取のすべてをフィルターすることを考えると少し心配です。
So that AI system designed by Vladimir Putin or whatever, or his minions, is going to be trying to talk to every American to convince them to vote for whoever pleases Putin or rile people up against each other.
つまり、ウラジーミル・プーチンやその手下が設計したAIシステムは、すべてのアメリカ人に話しかけ、プーチンに気に入られる候補者に投票するよう説得しようとするか、人々を互いに敵対させようとするでしょう。
They're not going to be talking to you.
あなたに話しかけることはありません。
They're going to be talking to your AI assistant, which is going to be as smart as they are.
彼らはあなたのAIアシスタントと話すことになります。それは彼らと同じくらい賢いでしょう。
That AI, because as I said, in the future, every single one of your interactions with the digital world will be mediated by your AI assistant.
そのAIは、私が言ったように、将来、デジタル世界とのすべてのやり取りがあなたのAIアシスタントによって仲介されることになります。
The first thing you're going to ask is, is this a scam?
最初に尋ねることは、これは詐欺なのかということですか?
Is this thing telling me the truth?
このものは私に真実を伝えているのか?
It's not even going to be able to get to you because it's only going to talk to your AI assistant.
それはあなたにたどり着くことさえできません、なぜならそれはあなたのAIアシスタントとだけ話すことになるからです。
Your AI assistant is not even going to, it's going to be like a spam filter.
あなたのAIアシスタントは、スパムフィルターのようになるだけで、実際にはそこにはありません。
You're not even seeing the email, the spam email, right?
あなたはそのスパムメールを見ていないでしょう?
It's automatically put in a folder that you never see.
それは自動的にあなたが見ないフォルダに入れられます。
It's going to be the same thing.
同じことになるでしょう。
And next, he talks about robots, and I've been making a lot of videos about robots lately, so let's see what Jan has to say about robots.
次に、彼はロボットについて話しており、最近私はロボットに関する多くのビデオを作っているので、ジャンがロボットについて何を言っているのか見てみましょう。
The next decade, I think, is going to be really interesting in robots.
次の10年は、ロボットにとって非常に興味深いものになると思います。
The emergence of the robotics industry has been in the waiting for 10, 20 years without really emerging, other than for like kind of pre-programmed behavior and stuff like that.
ロボティクス産業の台頭は、10年、20年と待ち続けていましたが、事前にプログラムされたような振る舞いなど以外には本当に台頭していませんでした。
And the main issue is, again, the Moravec paradox, like how do we get the system to understand how the world works and kind of plan actions?
そして、主な問題は、再びモラベックの逆説です。システムに世界がどのように機能するかを理解させ、行動を計画させる方法はどうすればいいのでしょうか?
We can do it for really specialized tasks.
私たちは本当に特化したタスクに対してそれを行うことができます。
The way Boston Dynamics goes about it is basically with a lot of handcrafted dynamical models and careful planning in advance, which is very classical robotics with a lot of innovation, a little bit of perception, but it's still not like they can't build a domestic robot.
Boston Dynamicsが取り組む方法は、基本的には多くの手作りの動力学モデルと事前の注意深い計画によるものであり、非常に古典的なロボティクスであり、多くの革新、少しの知覚があるものの、まだ家庭用ロボットを作ることができないということです。
We're still some distance away from completely autonomous level five driving, and we're certainly very far away from having level five autonomous driving by a system that can train itself by driving 20 hours like any 17 year old.
完全に自律レベル5の運転までまだかなり距離があり、確かに、17歳のように20時間運転して自分自身を訓練できるシステムによるレベル5の自律運転まで非常に遠いです。
A lot of the people working on robotic hardware at the moment are betting or banking on the fact that AI is going to make sufficient progress towards that.
現在、ロボットハードウェアに取り組んでいる多くの人々は、AIがその方向に十分な進歩を遂げるだろうということに賭けているか、期待しています。
And last, Lex asks a good question.
最後に、レックスが良い質問をします。
What gives you hope about humanity over the next few decades?
次の数十年で人類に希望を与えるものは何ですか?
And Jan gives some good answers there.
ヤンはそこでいくつか良い答えをしてくれます。
I really love hearing this.
私は本当にこれを聞くのが好きです。
I love ending it on kind of a positive note.
私はそれをポジティブなノートで終わらせるのが好きです。
We can make humanity smarter with AI.
AIを使って人類をより賢くすることができます。
I mean, AI basically will amplify human intelligence.
つまり、AIは基本的に人間の知能を増幅させるでしょう。
It's as if every one of us will have a staff of smart AI assistants that might be smarter than us.
まるで私たち一人ひとりが、私たちよりも賢いかもしれないスマートなAIアシスタントのスタッフを持っているかのようです。
They'll do our bidding, perhaps execute tasks in ways that are much better than we could do ourselves because they'd be smarter than us.
彼らは私たちの命令を実行し、おそらく私たちよりも賢いので、私たち自身ができるよりもはるかに優れた方法でタスクを実行するでしょう。
It's like everyone would be the boss of a staff of super smart virtual people.
まるで誰もが超スマートな仮想人々のスタッフのボスになるかのようです。
We shouldn't feel threatened by this any more than we should feel threatened by being the manager of a group of people, some of whom are more intelligent than us.
私たちは、私たちよりも賢い人々のグループのマネージャーであることに脅威を感じるべきではないのと同じくらい、これに脅威を感じるべきではありません。
I certainly have a lot of experience with this.
私は確かにこれに関する多くの経験があります。
That's actually a wonderful thing.
それは実際に素晴らしいことです。
Having machines that are smarter than us that assist us in all of our tasks or daily lives, whether it's professional or personal, I think would be an absolutely wonderful thing because intelligence is the most, is the commodity that is most in demand.
私たちよりも賢い機械を持つことは、私たちのすべての仕事や日常生活を支援することは、専門的なものであろうと個人的なものであろうと、絶対に素晴らしいことだと思います。なぜなら、知性は最も需要の高い商品だからです。
That's really what, I mean, all the mistakes that humanity makes is because of lack of intelligence, really, or lack of knowledge, which is related.
実際、人類が犯すすべての間違いは、本当に知性の不足、あるいは関連する知識の不足から起こると思います。
Making people smarter, which just can only be better.
人々をより賢くすることは、ただ良いことしかありません。
I mean, for the same reason that public education is a good thing and books are a good thing and the internet is also a good thing intrinsically.
公共教育が良いものであり、本も良いものであり、インターネットも本質的に良いものである理由と同じです。
And even social networks are a good thing.
そして、ソーシャルネットワークさえも良いものです。
If you run them properly, it's difficult, but you know, you can help the communication of information and knowledge and the transmission of knowledge.
それらを適切に実行すれば、難しいですが、情報や知識の伝達、知識の伝達を支援することができます。
AI is going to make humanity smarter.
AIは人類をより賢くするでしょう。
That's it.
それだけです。
I encourage you to check out the video in full.
ぜひ、フル動画をご覧いただくことをお勧めします。
It is very long.
とても長いです。
Hopefully this cut down version helped.
この短縮版が役立つといいのですが。
If you liked this video, please consider giving a like and subscribe and I'll see you in the next one.
もしこの動画が気に入ったら、いいねやチャンネル登録を考えていただけると嬉しいです。次の動画でお会いしましょう。
この記事が気に入ったらサポートをしてみませんか?