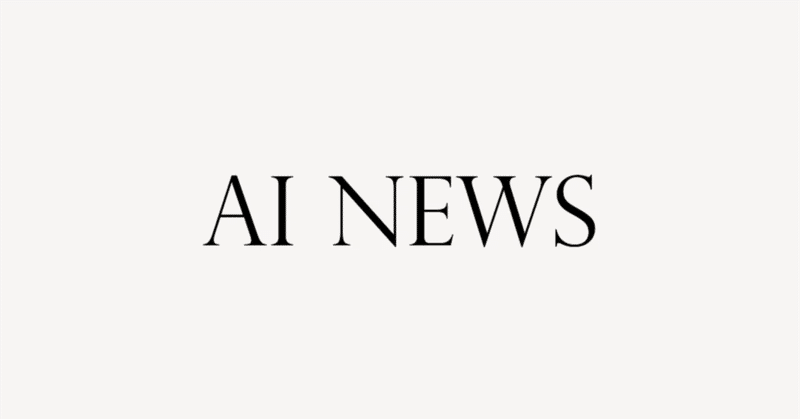
【AIによる医療革命の始まり:シミュレーションで訓練されたAIドクターが医師を凌ぐ精度を実現】英語解説を日本語で読む【2024年5月9日|@Wes Roth】
この動画では、大規模言語モデル(LLM)を用いたAIエージェントによって運営される病院のコンセプトを紹介しています。驚くべきことに、シミュレーション実験では、これらのAIドクターエージェントが人間の医師の能力を上回る結果を示しているのです。エージェント病院でのシミュレーションで獲得した知識は、現実世界の医療ベンチマークにも適用可能であり、約10,000人の患者を治療した後、進化したドクターエージェントはMedQAデータセットのサブセットで最先端の93%の精度を達成しました。これは、人間の専門家の87%を上回る結果です。また、LLMを科学者のように仮説を立て、実験を行い、データを収集できるかどうかを検討した最近の論文についても触れています。結果は、シミュレーションで生成されたデータが関連する経済理論の理論的予測と非常に一致することを示しました。一方で、こうした研究に対する批判的な意見についても取り上げています。データ収集やシミュレーションにLLMを使うことに反対する人もいますが、彼らの論拠は明確ではないようです。
公開日:2024年5月9日
※動画を再生してから読むのがオススメです。
Imagine you fall ill and go to visit the hospital, but this hospital is fully run by AI agents.
想像してください。あなたが病気になり、病院を訪れますが、その病院は完全にAIエージェントによって運営されています。
The doctors, the staff, the nurses powered by Large Language Models like GPT-4.
医師、スタッフ、看護師は、GPT-4のような大規模言語モデルによって支えられています。
How do you think that would work out?
それがどのように機能すると思いますか?
I can imagine what's going through your brain.
あなたの頭の中で何が起こっているか想像できます。
You might be thinking things like malpractice lawsuit, 100% fatality rate, zero chance of survival, right?
あなたは医療過誤訴訟、100%の死亡率、生存の可能性ゼロなどを考えているかもしれませんね?
Well, not quite.
まあ、そうでもないですね。
If you get nothing else out of this video, just remember this.
このビデオから他に何も得られなくても、これだけは覚えておいてください。
Doctor agents, again, AIs run on Large Language Models, can keep accumulating experience from both successful and unsuccessful cases.
再び医師エージェント、つまりGPT-4のような大規模言語モデルで動作するAIは、成功したケースと失敗したケースの両方から経験を蓄積し続けることができます。
Sounds kind of like humans, doesn't it?
それは人間に似ていますね?
Simulation experiments show that the treatment performance of doctor agents consistently improves on various tasks.
シミュレーション実験によると、医師エージェントの治療パフォーマンスは、さまざまなタスクで一貫して向上しています。
The knowledge that doctor agents have acquired in agent hospital and the simulation of a hospital is applicable to real world Medicare benchmarks, right?
医師エージェントがエージェント病院で獲得した知識と病院のシミュレーションは、実際の世界の医療基準に適用できるんですよね?
That's interesting, isn't it?
それは面白いですね。
After treating around 10,000 patients, this would take over two years for doctors in the real world.
約10,000人の患者を治療した後、現実世界の医師には2年以上かかるでしょう。
The evolved doctor agent achieves a state of the art accuracy of 93% on a subset of the MedQA data set.
進化した医師エージェントは、MedQAデータセットのサブセットで93%の最新の精度を達成しています。
If you're new to this channel, it's a point to understand that this isn't a fluke.
このチャンネルが初めての方は、これが偶然ではないことを理解するポイントです。
This is at this point, we can say it's kind of a pattern.
この時点で、これはある種のパターンと言えるでしょう。
The pattern is this.
そのパターンはこれです。
We are able to run certain AIs, certain neural networks and train them in simulation in a digital environment.
特定のAI、特定のニューラルネットワークを実行し、デジタル環境でシミュレーションしてトレーニングすることができます。
We're able to extract their learning, their digital brains, if you will.
彼らの学習、デジタルの脳とでも言うべきものを抽出することができます。
That experience that they've achieved in simulation is applicable, is useful in the real world.
彼らがシミュレーションで獲得した経験は、実際の世界でも有用です。
For example, right now you're seeing this little robot.
例えば、今、あなたはこの小さなロボットを見ています。
This is NVIDIA's simulation called, this is probably Isaac Jim or something similar.
これはNVIDIAのシミュレーションで、おそらくアイザック・ジムとか似たようなものです。
They have a few different ones.
いくつか異なるものがあります。
You're seeing that same robot in the real world with various wheels attached to his hands and feet, or his hands and feet are basically wheels.
あなたは、その同じロボットが、手や足に取り付けられたさまざまな車輪を持っているのを見ています。または、手や足自体が基本的に車輪です。
It learns to do it in simulation.
それはシミュレーションでそれを学びます。
It does it how or many millions of times.
それはどのようにして何百万回も行われるのですか。
The simulation has the same physics as we have here in the real world.
そのシミュレーションは、現実世界と同じ物理学を持っています。
It's slightly randomized here and there to make it a little bit more robust, a little bit more adaptable.
ここでもそこかしこでわずかにランダム化されて、少し堅牢で、少し適応性が高くなっています。
Looks like by 2000 iterations, it starts being able to open that door.
2000回の反復で、そのドアを開けることができるようになり始めるようです。
Time runs a thousand times faster, 10,000 times faster.
時間は1000倍速く、10000倍速く進みます。
You're able to train them very, very rapidly.
あなたは彼らを非常に迅速に訓練することができます。
When you take them out of the simulation, they're able to very robustly perform those tasks in the real world.
シミュレーションから彼らを取り出すと、彼らは実世界で非常に堅牢にその任務を遂行することができます。
I would see that action movie.
私はそのアクション映画を見るだろう。
But before we dive deeper into this paper, we probably should touch on this little tweet.
しかし、この論文にさらに深く踏み込む前に、この小さなツイートに触れるべきかもしれません。
This was posted on April 20th, 2024.
これは2024年4月20日に投稿されました。
Ethan Malik.
イーサン・マリク。
We've talked about him on this channel before.
このチャンネルで彼について話をしたことがあります。
Excellent person to follow.
フォローするのに優れた人物です。
Not a very controversial person.
非常に物議を醸す人物ではありません。
Not a very outrageous person.
非常に派手な人物でもありません。
He posts studies about AI.
彼はAIに関する研究を投稿しています。
Warden professor studying AI, innovation and startups as a substack, like a blog with a newsletter called One Useful Thing.
ウォーデン教授は、AI、イノベーション、スタートアップをサブスタックとして研究しており、ニュースレターがOne Useful Thingというブログのようなものです。
He posted a paper called Automated Social Science, Language Models as Scientists and Subjects.
彼はAutomated Social Science、Language Models as Scientists and Subjectsという論文を投稿しました。
No big deal, right?
大したことではないですね。
Here's that paper, MIT, Harvard.
こちらがその論文です、MIT、ハーバード。
The idea was pretty simple.
そのアイデアは非常にシンプルでした。
Again, they take Large Language Models, LLM, again, kind of the central theme with a lot of this stuff, right?
再び、彼らは大規模言語モデル、大規模言語モデルを取り上げています。これは、この分野の中心的なテーマの一つですね。
They're basically asking, and I'm simplifying quite a bit here.
基本的には、ここではかなり単純化していますが、彼らは尋ねています。
They're asking, can this LLM, can it be like a scientist?
彼らは、この大規模言語モデルが科学者のようになれるかどうか尋ねています。
Can it come up with hypotheses, run experiments, gather data?
それは仮説を立て、実験を行い、データを収集することができるでしょうか?
Can it, if you will, do science?
もしそうであれば、それは科学を行うことができますか?
Again, I'm simplifying.
もう一度言いますが、私は単純化しています。
Please don't yell at me.
私に叫ばないでください。
Social scientists start by selecting a topic or domain to study.
社会科学者は、研究するトピックや領域を選択して始めます。
Within that domain, they identify interesting outcomes and causes that might affect the outcomes.
その領域内で、彼らは興味深い結果とその結果に影響を与える可能性のある原因を特定します。
These are the variables.
これらは変数です。
The proposed relationships are the hypotheses.
提案された関係は仮説です。
For example, I have a hypothesis that your luck is greatly increased when you hit the like button and subscribe to this channel.
例えば、私は、このチャンネルの「いいね」ボタンを押すと運が大幅に向上するという仮説を持っています。
It's really improved.
本当に改善されました。
We can test that out by, well, you hitting the like button.
それを試してみることができます。まあ、あなたが「いいね」ボタンを押すことで。
We'll you know, go ahead and gather some data.
お願いですが、データを集めてください。
Go ahead and do that now.
今、それをやってください。
This is us designing an experiment to test these hypotheses by inducing variations in the causes and measuring the outcomes.
これは、原因に変動を引き起こし、結果を測定することでこれらの仮説を検証する実験を設計している私たちです。
After designing the experiment, social scientists determine how they will analyze the data.
実験を設計した後、社会科学者はデータをどのように分析するかを決定します。
They recruit participants, run the experiment and collect the data.
彼らは参加者を募集し、実験を行い、データを収集します。
You're the participant, hit the like button.
あなたは参加者です、いいねボタンを押してください。
They analyze the data and estimate the relationship.
彼らはデータを分析し、関係性を推定します。
Basically, this is kind of the scientific method, right?
基本的に、これは科学的方法の一種ですね?
This is how we move our understanding forward.
これが私たちの理解を前進させる方法です。
Up to very recently, 100% of this, this progress, this process was made by humans.
非常に最近まで、この進歩、このプロセスの100%は人間によって行われていました。
Very recently, we're seeing AI take over just a little bit here and there.
最近、AIが少しずつ支配し始めています。
All right, we've talked about GNOME, millions of new materials discovered with deep learning.
わかりました、私たちはGNOMEについて話しました、深層学習で何百万もの新しい材料が発見されました。
This is a DeepMind thing.
これは深層心理のことです。
This little robot sits here creating new materials.
この小さなロボットはここに座って新しい材料を作り出しています。
There's a separate sort of AI that comes up with certain potential recipes that this robot then tries to create.
別の種類のAIが出てきて、このロボットが作ろうとする特定のレシピを考えます。
This, what I assume is a blast proof case in case the robot messes up and something goes boom.
これは、ロボットがミスをして何かが爆発する場合の爆発防止ケースだと思われます。
Here's how many materials in this case, crystals, right?
このケースには、結晶がいくつか入っていますね。
This is how many humans were able to sort of create that were stable.
これは、人間が安定して作り出すことができた材料の数です。
With various computational methods, we had this circle, which is bigger.
さまざまな計算方法を用いて、私たちはこのような大きな円を持っていました。
Of course, this GNOME or GNOME, right?
もちろん、このGNOMEまたはGNOME、ですよね?
It's this big circle, right?
これは大きな円形ですよね?
AI is advancing science already in some areas.
AIはすでにいくつかの分野で科学を進化させています。
In this case, basically these LLM agents, they create certain social scenarios, hypotheses, then they build the AI agents with certain relevant attributes, right?
この場合、基本的にこれらの大規模言語モデルエージェントは、特定の社会的シナリオや仮説を作成し、それから特定の関連属性を持つAIエージェントを構築しますね?
For example, one of the experiments was a negotiation of some sort, right?
例えば、実験の1つは何らかの交渉だったのですか?
They designed those interactions, model estimation, data collection, and then they run the experiment.
彼らはそれらの相互作用を設計し、モデルの推定、データ収集を行い、それから実験を行いました。
They talk about all the things that worked well, all the things that maybe didn't work so well.
彼らはうまくいったこと、あまりうまくいかなかったことについて話します。
It wasn't perfect.
完璧ではありませんでした。
But at the end of the day, here's their conclusion.
しかし、最終的には、彼らの結論はこちらです。
We show that such simulations produce results that are highly consistent with theoretical predictions made by the relevant economic theory.
私たちは、そのようなシミュレーションが関連する経済理論によって行われた理論的予測と非常に一致する結果を生み出すことを示しています。
This could potentially allow for controlled experimentation at scale, right?
これは規模の大きな実験を制御する可能性がありますね。
We can run these simulations at scale to test various things, to gather data, right?
さまざまなことをテストし、データを収集するために、私たちはこれらのシミュレーションを規模で実行できますね。
We could re-yield insights that would generalize to the real world.
実際の世界に一般化される洞察を再生産することができるかもしれません。
You may be wondering, well, why was this controversial?
おそらく疑問に思っているかもしれませんが、なぜこれが論争の的となったのでしょうか。
What was the controversial piece of this whole thing?
この全体の中で論争の的となった部分は何でしたか。
This was it.
それがそれでした。
I caught a portion of this as it was happening.
私はこれが起こっている最中の一部を目撃しました。
People basically would come into the thread and they got very nasty.
人々は基本的にスレッドに入ってきて、とても不快になりました。
They did not like this idea of collecting data and assimilation.
彼らはデータの収集と同化のアイデアが好きではありませんでした。
They didn't like the idea of Large Language Models potentially contributing to science.
大規模言語モデルが科学に貢献する可能性を好まなかったのです。
They weren't very nice about it.
それについてはあまり親切ではありませんでした。
Ethan Molek says, yeah, he's going to delete that thread.
イーサン・モレックは、そうですね、そのスレッドを削除するつもりだと言っています。
People are just insulting the paper authors without reading the paper or understanding the context of the fields being discussed.
人々は、論文を読まず、議論されている分野の文脈を理解せずに、論文の著者を単に侮辱しているだけです。
They, meaning the authors, didn't ask, they tweet about them and didn't expect the tweet to travel so widely, getting nasty on Twitter.
彼ら、つまり著者たちは、尋ねることはなく、ツイートをして、そのツイートが広く広まることを予想していなかったので、Twitterで険悪になりました。
Again, I saw a little bit of it.
また、それの一部を見ました。
I'm not sure exactly what got people so riled up, but having talked about some of these topics on YouTube, I know firsthand that there's some small but very vocal minority of people that go into this murderous rage when you suggest that Large Language Models have some ability to reason, to understand, to have world models.
人々をそんなに立腹させたのが正確にはわかりませんが、YouTubeでこれらのトピックについて話したことがあるので、大規模言語モデルが推論する能力、理解する能力、世界モデルを持っていると提案すると、ごく少数ですが非常に声の大きい人々がこの殺意に満ちた怒りになることを第一に知っています。
They usually don't have something that can be called arguments that show why they think what they think.
彼らが何を考えているのかを示すと言えるような議論を持っていることはほとんどありません。
It's mostly just insults and getting nasty.
ほとんどが単なる侮辱と険悪です。
I had a lot of misgivings about talking about this paper.
この論文について話すことについて多くの懸念がありました。
On one hand, I'm very busy because I'm launching my course in how to build AI agents.
一方で、私はAIエージェントを構築する方法についてのコースを開講しているので、非常に忙しいです。
It's going very well.
進んでいます。
We're up to almost a thousand people.
ほぼ千人に達しています。
It's been live for just a few days.
数日間しか経っていません。
Check out the links down below if you're interested.
興味がある場合は、下記のリンクをチェックしてください。
On one hand, I don't really have too much time to delve into this if you will, but on the other hand, talking about this new paper would probably make a lot of those nasty emotional people very, very mad, which I just cannot say no to.
一方で、時間を割く余裕があまりないのですが、他方で、この新しい論文について話すことは、多くの感情的な人々を非常に怒らせる可能性があります。それには断れません。
Today let's talk about Agent Hospital, a simulacrum of hospital with evolvable medical agents.
今日は、エージェント病院について話しましょう。これは進化可能な医療エージェントを持つ病院の模擬です。
Yes, AI agents that think, reason, learn, and evolve, and there's nothing you can do to stop it.
はい、考え、推論し、学習し、進化するAIエージェントが存在し、それを止めることはできません。
Let's get started.
始めましょう。
This is kind of like their main overview of what this hospital looks like.
これは、この病院がどのように見えるかの主要な概要のようなものです。
They're saying this is an overview of Agent Hospital.
彼らはこれがエージェント病院の概要だと言っています。
It is a simulacrum of hospital in which patients, nurses, and doctors are autonomous agents powered by Large Language Models.
医師、看護師、患者が大規模言語モデルによって駆動される自律エージェントである病院の模擬です。
Agent Hospital simulates the whole closed cycle of treating a patient's illness.
エージェント病院は、患者の病気を治療する全体の閉じたサイクルをシミュレートしています。
Disease onset, triage, registration, consultation, medical examination, diagnosis, medical dispensary, convalescence, and post-hospital follow-up visit.
疾病の発症、トリアージ、登録、相談、医学的検査、診断、医薬品の処方、回復期、および退院後のフォローアップ訪問。
An interesting finding is that the doctor agents can keep improving treatment performance over time without manually labeled data.
興味深い発見は、医師エージェントが手動でラベル付けされたデータなしで治療のパフォーマンスを時間とともに向上させ続けることができるということです。
This is kind of the big deal.
これはかなりの大きな問題です。
Normally when we train AI, we need lots of data.
通常、AIを訓練する際には、多くのデータが必要です。
For example, if we're training an AI that recognizes pictures of animals, we need lots of pictures of animals with labels.
たとえば、動物の写真を認識するAIを訓練する場合、ラベルが付いた多くの動物の写真が必要です。
They call it data pairs.
それをデータペアと呼びます。
A picture of a dog, and then along with that, something that says this is a dog.
犬の写真と一緒に、これが犬だということを示すものがあります。
Many of those, so they can kind of generalize how dogs, what they look like.
それらがたくさん必要なので、犬がどのように見えるかを一般化できるようになります。
A million pictures of dogs that are labeled dog.
百万枚の犬の写真が「犬」とラベルされています。
I think in this case, since they're talking about dermatology, so let's say it's some sort of a skin condition, I don't know, some rash.
この場合は、皮膚科について話しているので、ある種の皮膚疾患であるとしましょう、わかりませんが、ある発疹かもしれません。
Maybe there's a bunch of pictures of this rash that's labeled with the name of that particular condition, right?
おそらく、その特定の状態の名前でラベル付けされたこの発疹の写真がたくさんあるのでしょうね。
That's normally how we do it.
通常、そういうやり方です。
But here it seems like in the simulation, these doctors, these AIs keep learning, keep improving without manually labeled data.
しかし、ここでは、シミュレーションでは、これらの医師、これらのAIは、手動でラベル付けされたデータなしに学習し、改善し続けているようです。
Without human medical professionals sitting there and pouring over data and labeling it and making sure it's correct, you can say that they generate their own synthetic data within the simulation and then learn and improve from that data.
人間の医療専門家がデータを見てラベル付けし、それが正しいことを確認することなく、彼らはシミュレーション内で自分自身の合成データを生成し、そのデータから学習して改善していると言えます。
The central goal of this paper is to enable a doctor agent to learn how to treat illnesses within the simulacrum.
この論文の中心的な目標は、医師エージェントがシミュラクラム内で疾患の治療方法を学ぶことを可能にすることです。
They're proposing a method called MedAgent Zero.
彼らはMedAgent Zeroと呼ばれる方法を提案しています。
Here's kind of a map that they've built.
こちらが彼らが構築した地図のようです。
Looks like this is the entrance, right?
ここが入り口のようですね?
You have the registration window, pharmacy, waiting area, the triage station, biochemical testing room, et cetera, et cetera.
登録窓口、薬局、待合室、集中治療室、生化学検査室などがあります。
Looks like they've used this tiled map editor.org to build out some of this stuff.
このタイルマップエディターを使って、いくつかのものを構築しているようですね。
I gotta say, I'm very excited about the intersection of video games and AI, specifically AI agents.
ビデオゲームとAI、具体的にはAIエージェントの交差点に非常に興奮しています。
I mean, the fact that we can use generative AI to voice dialogue, create dialogue, all that stuff, that's cool.
生成AIを使用して対話を声で表現したり、対話を作成したりできるという事実は、すごいですね。
But something about having actual autonomous agents running around in games and affecting the gameplay world, I gotta say, that's making me very excited. And looks like they also use this phaser.io, a fast, free and fun open source framework for various browser games.
ただし、実際の自律エージェントがゲーム内を走り回り、ゲームプレイの世界に影響を与えるという点については、非常に興奮しています。そして、このphaser.ioも使用しているようですね。これは、さまざまなブラウザゲーム向けの高速で無料で楽しいオープンソースフレームワークです。
I guess here's kind of what that looks like.
こういう感じですね。
They use something like that to build this, right?
これを構築するために、そのようなものを使用しているんですね。
This is a hospital sandbox simulation environment, tiled and phaser.
これは、タイルとphaserを使用した病院の砂場シミュレーション環境です。
Tiled is a map designing tool.
Tiledはマップデザインツールです。
Phaser is a framework to manage the movements and interactions of the agents on the sandbox.
Phaserは、砂場上のエージェントの移動や相互作用を管理するためのフレームワークです。
They create the various agents and the information about the roles of these agents is generated using GPT-3.5.
彼らはさまざまなエージェントを作成し、これらのエージェントの役割に関する情報はGPT-3.5を使用して生成されます。
I think they're just using GPT-3.5 just for kind of generating the background for these people.
彼らはおそらく、これらの人々の背景を生成するためにGPT-3.5を使用しているだけだと思います。
I hope they're not using it for the doctors.
医師には使っていないといいな。
Can you imagine going to a hospital and the doctors running on like GPT-3.5?
病院に行って、医師がGPT-3.5のように動いているのを想像できますか?
You would walk right out, right?
すぐに出て行くでしょうね。
It's a little LLM joke for you there.
それはちょっとした大規模言語モデルのジョークですね。
They create a series of medical professional agents, including 14 doctors and four nurses.
彼らは14人の医師と4人の看護師を含む一連の医療専門家エージェントを作成します。
Doctors diagnose diseases and create treatment plans, whereas nurse agents focus on three hours supporting the day-to-day therapeutic interventions.
医師は疾病を診断し、治療計画を立てますが、看護師エージェントは日常的な治療介入をサポートするのに3時間を費やします。
Here's example agents.
こちらが例のエージェントです。
We have a radiologist, his duty is spelled out, skills, et cetera.
私たちは放射線科医がいます。彼の職務内容、スキルなどが明記されています。
We have a patient, internal medicine doctor and a receptionist.
お患者様、内科医、受付係がいます。
If they're running on GPT-4, I'd be curious to know how much this whole experiment cost.
GPT-4で動いているなら、この実験全体がいくらかかったのか気になります。
I believe the Stanford experiment, the social simulacra we've covered on this channel kind of had a similar thing, but there it wasn't even a hospital.
私は、このチャンネルで取り上げたスタンフォードの実験、社会のシミュラクラは似たようなことをしていたと思いますが、そこでは病院でもありませんでした。
It was just a little village where people went around their business and tried to organize a party.
そこはただの村で、人々が日常生活を送り、パーティーを企画しようとしていました。
I think that one blew through thousands of dollars of OpenAI API credits.
あの実験では、何千ドルものOpenAI APIクレジットが消費されたと思います。
Planning, we've covered how to approach these agentic planning, how to create planning architectures for agents in that Stanford simulation.
計画について、私たちはどのようにこれらの計画をアプローチし、スタンフォードのシミュレーションでエージェントの計画アーキテクチャを作成するかを取り上げました。
The interesting thing there, they don't go into as much detail about planning as I think they did in the Stanford paper there.
そこでの興味深い点は、スタンフォードの論文で行ったように、計画についてはあまり詳しく触れていないことです。
The trick was starting very broadly with the entire day, right?
トリックは、まず一日全体から広く始めることでしたね。
Kind of like, what are the big rocks in the day?
まるで、その日の中での大きな課題は何か、という感じですね。
That's the first thing you ask ChatGPT, this LLM.
それが、ChatGPT、この大規模言語モデルに最初に尋ねることです。
You kind of keep fine-tuning it like, okay, so then break it down by every hour, then every half hour, then every 15-minute intervals, etc.
あなたは、それを微調整していくような感じで、例えば、それを毎時間ごとに分解して、それからそれぞれの30分ごとに、それから15分ごとに、などとしています。
Here, these patients in this case, right, they have their daily planning and dynamic planning, right?
ここでは、この場合の患者たちは、彼ら自身の日常計画と動的計画を持っていますね?
Upon arrival at the hospital, they'll go to the triage station, and then based on what happens there, their actions and movements will follow based on what happens there if they tell them, oh, we got to get you into urgent care, whatever they go there.
病院に到着すると、彼らはトリアージステーションに行き、そしてそこで何が起こるかに基づいて、そこで彼らの行動や動きが続くことになります。もし彼らに、「ああ、緊急ケアに入れないといけない」と言われたら、そこに行くことになります。
Here, this person gets sick, KM goes to the hospital at the front desk, the triage, I guess this means registration, they head over to registration, and then into consultation, then into medical examination, diagnosis, then to the medical dispensary, convalescence, and then there's this arrow pointing back to the disease starts over again, which this sounds like a nightmare if it's just an endless loop, but okay.
ここでは、この人が病気になり、KMが病院のフロントデスク、トリアージ、登録を意味すると思われる、登録に向かい、そして相談に進み、そして医学的検査、診断、そして医薬品の処方、回復期、そして病気が再び始まることを示す矢印があります。これがただの終わりのないループなら、これは悪夢のようですが、まあ。
Planning for the medical professionals, they just kind of fulfill their responsibilities based on their designated role.
医療従事者の計画では、彼らは指定された役割に基づいて責務を果たすようにしています。
They're trained from two types of actions.
彼らは2種類の行動から訓練されています。
One is practice.
一つは実践です。
Actually dealing with the patients, giving them care as patients are assigned to them during their shift, and the follow-up information from patients that will help them polish their medical records experience.
実際に患者と取り組み、シフト中に割り当てられた患者にケアを提供し、患者からのフォローアップ情報を受け取り、それが彼らの医療記録の経験を磨くのに役立ちます。
The second part of their kind of learning or planning aspect is actually learning.
彼らの学習や計画の側面の第二の部分は、実際の学習です。
Outside of working hours, they engage in studying past medical records to gain clinical experience, reading medical textbooks to expand their knowledge.
労働時間外に、彼らは臨床経験を得るために過去の医療記録を研究し、知識を拡大するために医学の教科書を読むことに取り組んでいます。
It's this idea that a Large Language Model, right, this soulless machine, if you will, sits there in a simulation, reading a book in its off time when it's not working, and is able to expand their knowledge to improve the results from reading a book.
大規模言語モデルという考え方ですね、この無機質な機械が、仕事をしていないときに本を読んで知識を拡大し、結果を改善できるというものです。
I mean, to me, that's very exciting.
私にとっては、それは非常に興奮するものです。
That's very interesting to think about.
それを考えると非常に興味深いです。
But I think this is where a lot of people get uncomfortable, which by the way, if you're one of those people, I'd love to know more.
しかし、ここで多くの人々が不快に感じるところだと思います。ところで、もしあなたがそのような人の一人なら、もっと詳しく知りたいです。
What is it that is so disturbing about this idea?
このアイデアが何をしているのか、何がそんなに不快なのか、それは何ですか?
Because again, there seems to be a lot of anger directed at the people that are talking about this.
なぜなら、再び、この話題について話している人々に向けられる怒りが多いようです。
We won't cover a lot of this stuff, but I do encourage people to read the paper.
私たちはこれについて多くをカバーしませんが、論文を読むことをお勧めします。
But let's focus on the core things here.
しかし、ここで重点を置きましょう。
The evolution, right?
進化、ですね?
The improvement of these agents, they propose their kind of framework, their strategy med agent zero, and there are two important sort of areas, modules in this strategy, the medical record library and the experience base successful cases, which are to be used as reference for future medical interventions are compiled and stored in the medical record library for cases where treatment fails, doctors are tasked to reflect and analyze the reasons why they failed.
これらのエージェントの改善、彼らは自分たちの種類のフレームワーク、彼らの戦略medエージェントゼロを提案しており、この戦略には2つの重要な領域、医療記録ライブラリと経験ベースの成功事例があります。将来の医療介入の参照として使用される医療記録ライブラリに収集され、治療が失敗した場合、医師は失敗の理由を反省し分析するように求められます。
It's still a guiding principle to be used as a cautionary reminder for subsequent treatment processes.
後続の治療プロセスに対する警告として使用される指針として、これはまだ有効です。
Here's kind of their med agent zero method, right?
こちらが彼らの医薬品ゼロメソッドの一種ですね。
You have the patient query, so the doctor can use medical record retrieval prompting, so aka asking the patient experience retrieval, then it looks like the medical agents, the doctors generate an answer.
患者のクエリがあるので、医師は医療記録の検索を促すことができます。つまり、患者の経験の検索を尋ねること、それから医療エージェントのように、医師が答えを生成するようです。
I'm assuming this is their diagnosis, right?
これが彼らの診断だと仮定していますね。
Is it correct?
正しいですか?
Is it not correct?
正しくないですか?
Here we have kind of the golden answer, meaning this is the truth, kind of the ground truth, like this is what we know to be the true answer.
ここには一種の正解があります。これが真実であり、一種の真実、つまりこれが真の答えであるということを意味します。
If somebody comes in with disease X, right, we make sure that the doctor comes up with that.
誰かが疾患Xで来院した場合、私たちは医師がそれを考え出すようにします。
If the doctor says something else, we say, no, that's incorrect.
医師が他のことを言った場合、それは違うと言います。
The doctor goes into the reflection mode, thinking about it again, as they say here, distilling guiding principle to be used as a cautionary reminder for further treatment, right?
医師は、ここで言うように、再考して、将来の治療のための戒めとして使用される指針を抽出するために反射モードに入りますね?
If the answer is correct, right, then we know that that's the case for that hospital patient.
もし答えが正しいなら、その病院の患者にとってそのような状況であることがわかりますね。
We add that to the medical records library, again, to be used in the future.
それを将来に使用するために、再び医療記録ライブラリに追加します。
If they come in, we're able to retrieve it and say, oh, you had this in the past, right?
もし彼らが来たら、それを取り出して、あなたは過去にこれを持っていたと言えるんですね。
If they don't get it correct, and after reflection, right, after thinking about it, they attempt to re-answer it, right?
もし彼らがそれを正しく理解できない場合、そして反射した後、それについて考えた後、再び答えようとしますね?
If they get it wrong again, we abort it, right?
もし再び間違えた場合、中止しますね?
It looks like they're just not getting it.
彼らは理解していないようですね。
But if they answer correctly on the second try, like it's added to the valid experience, to the experience base.
もし2回目に正しく答えた場合、それは有効な経験、経験の基盤に追加されるようです。
I gotta say, this is similar to probably how the human learning process is, probably everything except the fact that there's probably no golden answer.
私は言わなければならない、これはおそらく人間の学習プロセスがどのようなものかに似ていますが、おそらく唯一の違いはおそらく黄金の答えがないということです。
There's no absolute truth that we can compare it to usually.
通常、それを比較できる絶対的な真実はありません。
Also, if you're wrong twice, we don't just pull the plug on you.
また、2回間違えた場合、ただあなたを切り捨てるわけではありません。
But other than that, this is very lifelike.
しかし、それ以外は非常にリアルに見えます。
This process builds the record library, the experience base keeps expanding, the more patients are treated.
このプロセスは記録ライブラリを構築し、経験の基盤は患者が治療されるほど拡大していきます。
The way those records are built, so they use text embedding into vector space by this embedding model provided by OpenAI.
OpenAIが提供するこの埋め込みモデルによって、テキスト埋め込みがベクトル空間に行われるように、それらのレコードが構築されている方法です。
Basically how LLMs store data isn't in text format, how you and I would, they use something called vector space.
基本的に、大規模言語モデルがデータを保存する方法は、あなたや私がするようなテキスト形式ではなく、ベクトル空間と呼ばれるものを使用しています。
I think the easiest way to kind of visualize that of these little 3D charts where each word has a set of traits.
これを少し視覚化する最も簡単な方法は、各単語が一連の特性を持つ小さな3Dチャートです。
Based on those traits, you can kind of visualize it in a 3D environment.
それらの特性に基づいて、それを3D環境で視覚化することができます。
If you're looking for a cluster of words, for example, they have kind of similar meaning or similar tone or whatever, you can kind of organize it like this.
例えば、意味やトーンが似ているような単語のクラスターを探している場合、このように整理することができます。
Here's kind of one that's showing the different directions of like sky, wings and engine, right?
ここには、空、翼、エンジンの異なる方向を示しているものがありますね。
A helicopter drone and rocket will kind of be in this space, whereas a goose eagle bee would be in this space.
ヘリコプタードローンやロケットはこの空間にあり、一方、ガチョウやワシ、蜂はこの空間にあるでしょう。
All things with these characteristics would kind of be in that same vector space, which is interesting to think about because I feel like kids when they're learning to speak English or whatever language they're learning, you can kind of think of it as them using something similar to this.
これらの特性を持つすべてのものは、同じベクトル空間にあるということで興味深いと思います。なぜなら、子供たちが英語や他の言語を学ぶとき、これに類似したものを使っていると考えることができるからです。
They learn the meanings of words by kind of contrasting them to the other words that they know.
彼らは、自分が知っている他の単語と比較して単語の意味を学んでいます。
Right there, these clustering of words, whether it's words that are opposites or words that are groups.
ここには、単語のクラスタリングがあります。それが反対の単語であるか、グループであるかに関係なく。
All that is to say that I don't think that the fact that LMAI agents act kind of human-like at the end of the day, I don't think that's an accident.
つまり、LMAIエージェントが最終的に人間らしく振る舞うことは、偶然ではないと思います。
The point here from the experimental results is that after they keep training on 10,000 samples, 10,000 patients, they show a continuous improvement, a continuous increase.
実験結果からのポイントは、1万のサンプル、1万の患者にトレーニングを続けると、連続的な改善、連続的な増加が見られるということです。
As you can see here, there's a rapid increase in precision, right?
ここで見ていただけるように、精度が急速に向上していますね。
There's like this rapid increase up to maybe 2000 training samples.
おそらく、トレーニングサンプルがおそらく2000まで急速に増加しています。
I mean, it continuously increases from there on, but I mean, there's a little bit of a diminishing returns, but the point is they keep improving.
それ以降も継続的に増加していますが、収益の減少が少しあるとは言え、重要なのは彼らが改善し続けているということです。
They keep learning.
彼らは学び続けています。
It does seem like they're using GPT-3.5 for this because probably GPT-4 would be, I mean, 10,000 patients, however many, 14 doctors or however many they had.
おそらく、これにはGPT-3.5が使用されているように見えます。おそらくGPT-4を使用すると、1万人の患者、14人の医師など、多くの人数が必要になるでしょう。
I mean, that would cost a lot of money.
それには多額の費用がかかるでしょう。
Keep in mind that if they just substituted GPT-4 for this, the results would likely be even better.
ただし、GPT-4をこれに代替すれば、結果はさらに良くなる可能性があります。
Here's the breakdown of various diseases, how well they're able to Figure out what those are.
様々な病気の詳細をここに示しますが、それらが何であるかをどれだけ正確に把握できるか。
For some of these, I mean, they started out at like 20% accuracy or 40 or 60% accuracy.
これらのうち、いくつかは、最初は20%の正確さや40%または60%の正確さで始まったという意味です。
Over time, like on this one, this has got to be around 90% accuracy.
時間の経過とともに、これはおそらく90%の正確さになります。
There's an obvious learning process that happens.
明らかに学習プロセスが起こっています。
All right, so they used both GPT-3.5 and GPT-4.
GPT-3.5とGPT-4の両方を使用しました。
I apologize if it was a little bit unclear to me which one they used.
彼らがどちらを使用したかが少し不明確であれば、申し訳ありません。
They used both.
彼らは両方を使用しました。
As you can see here, they compared it to just prompting these models for the answer.
ここで見るように、これらのモデルに回答を促すだけと比較しました。
These were the results.
これが結果です。
They use chain of thought as well as these other sort of approaches, these methods for testing them.
彼らは、これらの他の種類のアプローチ、これらのテスト方法をテストするために、思考の連鎖も使用しています。
But MedAgent Zero, which is this paper that we're covering, so training them in a simulation with 10,000 iterations, 10,000 patients.
メッドエージェントゼロは、この論文で取り上げているもので、10,000回の繰り返し、10,000人の患者を使ったシミュレーショントレーニングを行っています。
I mean, both GPT-3.5 and GPT-4 beat out all the other ways of using that model.
私は、GPT-3.5とGPT-4の両方が、そのモデルを使用する他のすべての方法を凌駕しているという意味です。
They are the number one.
彼らはナンバーワンです。
GPT-4 comes in at 93%, which outperforms human experts in the MedQA data set, which humans experts are around 87%. So GPT-3.5 approaches a medical professional on that data set.
GPT-4は93%で、MedQAデータセットで人間の専門家を上回ります。人間の専門家は約87%です。つまり、GPT-3.5はそのデータセットで医療専門家に近づいています。
GPT-4 beats them by quite a bit, 87% versus 93%. Also keep in mind they can run the simulation a lot faster, right?
GPT-4は87%に対して93%で彼らを打ち負かします。また、彼らはシミュレーションをはるかに速く実行できることにも注意してくださいね。
10,000 patients.
10,000人の患者です。
I mean, it would take a doctor many years to accumulate that much experience.
つまり、医師がそのような経験を蓄積するには多くの年数がかかるでしょう。
Here that could be ran pretty rapidly and every year you'd be able to run it faster and faster as technology improves, as both hardware and software and these neural nets improve as well.
ここでは、技術が改善されるにつれて、ハードウェアやソフトウェア、そしてこれらのニューラルネットも改善されるにつれて、かなり迅速に実行でき、毎年より速く実行できるようになります。
If you enjoyed this, please hit thumbs up to get this out to more people.
もしこれを楽しんでいただけたら、よろしければ高評価を押して、より多くの人々に広めてください。
If this bothers you, tell me why.
もしこれが気になるなら、なぜ気になるのか教えてください。
I am genuinely curious because I keep seeing this pop up and it's just not just me on my YouTube channel, but obviously even respected professors and researchers that post their work on Twitter.
私は本当に興味があります。なぜなら、私のYouTubeチャンネルだけでなく、尊敬されている教授や研究者たちも自分の研究成果をTwitterに投稿しているからです。
Actually here I found one comment that actually, so once Ethan Molyk deleted the original tweet, a lot of those kind of really negative responses disappeared.
実際、ここで1つのコメントを見つけました。つまり、Ethan Molykが元のツイートを削除した後、そのような非常に否定的な反応の多くが消えました。
But here's one that from what I've seen, because I briefly went into that RABBIT hole of that thread, and I think this is kind of representative of what was said.
しかし、ここに1つあります。私がそのスレッドのRABBITホールに少し入ったので、これが言われていたことを代表していると思います。
I think this person kind of captures the complaint, but just imagine this, but much nastier and attacking people personally.
この人が苦情を表現していると思いますが、これを想像してみてください。もっと陰湿で個人攻撃的なものです。
He's saying, to be fair, you kind of casually post ultra dystopian stuff and are like, isn't this tech so cool?
彼は、公平を期すと、あなたは超ジストピア的なものをさりげなく投稿して、このテクノロジーはすごいですね、と言っていると言っています。
Smart man in your area, but you seem not detached from the world a bit.
あなたの地域の賢い人ですが、世界から少し離れているように見えます。
Maybe he meant you seem too detached from the world a bit if you didn't see this coming at some point.
おそらく彼は、あなたはこのようなことがいつか起こると予想していなかったら、少し世界から離れすぎているように見えると言ったのかもしれません。
Again, I really think this is really representative of what the people were saying.
再び、私は本当にこれが人々が言っていたことをよく代表していると思います。
On this channel, I try to be unbiased and try to understand a lot of different perspectives, or at least be open-minded to it.
このチャンネルでは、偏見を持たず、さまざまな視点を理解しようとしたり、少なくともそれに対して開かれた心を持とうとしています。
I wouldn't understand this, but I just don't.
私はこれを理解できないでしょうが、ただ単に理解できません。
He's saying that studies like this are ultra dystopian.
彼は、このような研究は超ディストピア的だと言っています。
I don't even understand why would it be ultra dystopian?
なぜそれが超ディストピア的であると思うのかさえ理解できません。
Accelerating progress by the use of machines, improving our ability to understand the world around us, improving our technology, improving our ability to help people that are sick to diagnose.
機械の利用による進歩を加速し、私たちの周りの世界を理解する能力を向上させ、技術を向上させ、病気の人々を診断する能力を向上させる。
Maybe potentially have AI doctors that can help people without resources.
おそらく、資源のない人々を助けることができるAI医師がいるかもしれません。
There's many places in the world where there's poverty, there's no doctors, there's no access to high-quality medical care.
世界の多くの場所で貧困があり、医師がいない、高品質な医療が利用できない場所があります。
What if these trained AI agents could help people for pennies to at least get a correct diagnosis?
これらの訓練されたAIエージェントが、少額で正しい診断を受けるために人々を助けることができたらどうでしょうか?
How's that dystopian?
それがディストピア的なのはどうしてですか?
I almost feel like this ultra dystopian would be if we outlawed this technology, that we didn't allow humanity to benefit from what it could bring to the world, because that would benefit the people that already have everything that they need.
私は、この超ディストピア的なのは、この技術を禁止し、人類がそれがもたらす恩恵を受けることを許さない場合に起こると思います。それはすでに必要なすべてを持っている人々に利益をもたらすでしょう。
If somebody said that would be ultra dystopian, I could agree that would be.
誰かがそれが超ディストピア的だと言ったら、それに同意できるかもしれません。
But this I just don't understand.
しかし、これについては理解できません。
I also notice how, and a lot of these comments have that in common, they do tend to get personal.
ある程度のコメントには共通点があり、それは個人攻撃になりがちだとも感じます。
There's personal attacks.
個人攻撃があります。
Here they're saying, well, you're detached from the world if you didn't see this coming.
ここでは、「これが来るとは思わないなんて、あなたは世間から遠ざかっている」と言っています。
You may be smarter over here, but you're kind of clueless over here.
こちらでは賢いかもしれませんが、こちらでは無知です。
That's what a lot of these comments had in general.
それが多くのコメントに共通していたことです。
They're not saying, I disagree with what you're saying, and here's a respectful breakdown of some points that I disagree with you with.
彼らは、「あなたの言っていることには同意しません。ここにいくつかのポイントを尊重して反対します」とは言っていません。
Most of them were wrong and you're clueless and you're a horrible person.
ほとんどが間違っており、あなたは無知でひどい人間だと言っています。
That was the kind of combination of those things, which again, I have a hard time grasping where that's coming from.
それらの組み合わせがそのようなものでした。再び、それがどこから来ているのか理解するのが難しいです。
We've covered Sam Altman talking yesterday to the kids at MIT because it does seem like he's getting a lot of this from, this is kind of sad, from these college students at these elite universities, or at least some subset of them.
1昨日、MITの学生たちに話をしていたサム・アルトマンについて取り上げました。彼は、これが多くのものを得ているように思われますが、これは少し悲しいことですが、これらのエリート大学の学生たち、または少なくともその一部からです。
He posted this, I think, as a response to that.
これに対する彼の反応として、彼はこれを投稿しました。
Just the timeline fits to where I think he was talking about what he experienced during his college tours, talking to these students at Stanford and MIT, etc.
タイムラインが合致しているだけでなく、彼はおそらく、彼の大学ツアー中に経験したことについて話していたと思います。スタンフォードやMITの学生たちと話していたことについてです。
He's saying, using technology to create abundance, intelligence, energy, longevity, whatever, will not solve all problems, it will not magically make everyone happy.
彼は言っています、技術を使って豊かさ、知性、エネルギー、長寿などを創造することはすべての問題を解決するわけではないと、誰もが幸せになるわけではないと。
But it is an unequivocally great thing to do and expands our option space.
しかし、それは断固として素晴らしいことであり、私たちの選択肢を拡大します。
To me, it feels like a moral imperative.
私にとって、それは道徳的な義務のように感じられます。
At the bottom here, he's saying his most surprising takeaway from the recent college visits that this, what he said above there, is a surprisingly controversial opinion with certain demographics.
ここでは、最近の大学訪問からの最も驚くべき収穫は、上記で述べたことが、特定の人口統計にとって驚くほど論争的な意見であると彼は言っています。
Again, on this channel even, you can go back like a year, see some of those videos I did about GPT-4 reasoning where I talk about specifically this, me dealing with this, where you cover some cool new AI breakthrough that seems to be very beneficial and move science forward.
おまたせしましたが、このチャンネルでも1年前のように遡ることができ、私がGPT-4の推論について行ったビデオを見ることができます。そこでは、この具体的な話題について話している私の姿があります。そこでは、非常に有益で科学を前進させるような新しいAIのブレークスルーについて取り上げています。
It's surprisingly controversial.
驚くべきことに、それは論争の的です。
With certain demographics, it's a minority of people, but they're really incensed about it.
特定の人口層では、少数派の人々ですが、彼らは本当に激怒しています。
He says, prosperity is a good thing, actually.
彼は、「繁栄は実際には良いことだ」と言っています。
D, D, growth.
D、D、成長です。
That's it for me for today.
今日はこれで終わりです。
If you've enjoyed this stuff here on this channel and would like to join me in my quest to build the biggest and most valuable private forum where we can talk about AI, how to build autonomous AI agents, where we can all upskill and learn to use AI to prepare for what's coming in the future.
このチャンネルでこのコンテンツを楽しんでいただけたら、私と一緒に最大かつ最も価値のあるプライベートフォーラムを構築するという私のクエストに参加したいと思うかもしれません。そこでは、AIについて話し合い、自律型AIエージェントを構築する方法、AIを使って未来に備えるためにスキルを向上させ、学ぶことができます。
I launched a natural 20.
自然な20を出しました。
I'll put the links down in the description.
リンクは説明欄に記載します。
We're almost thousand strong.
私たちはほぼ1000人のメンバーがいます。
Join us, won't you?
一緒に参加してくださいね。
Let's build it together.
一緒に作り上げましょう。
My name is Wes Roth, and thank you for watching.
私の名前はウェス・ロスです。ご視聴ありがとうございました。
この記事が気に入ったらサポートをしてみませんか?