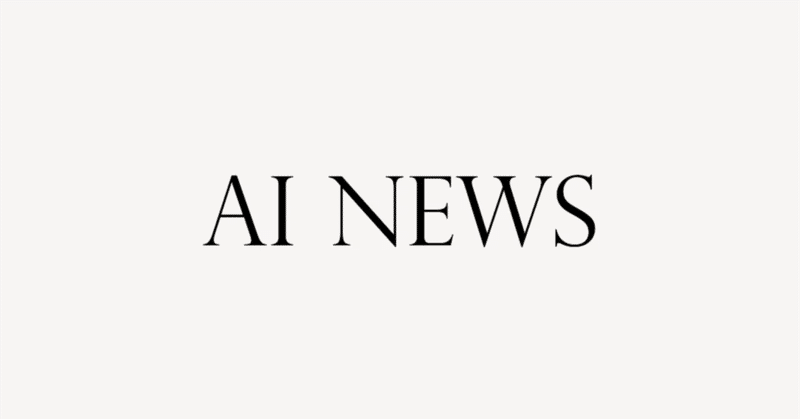
【AlphaFold 3:生命の仕組みを解明する次世代AIの登場】英語解説を日本語で読む【2024年5月9日|@TheAIGRID】
Google傘下のDeepMindとIsomorphic Labsが、AI技術を用いた革新的な生体分子構造予測モデル「AlphaFold 3」を発表した。AlphaFold 3は、タンパク質、DNA、RNA、リガンドなどの構造と相互作用を高精度で予測することができる。この技術は、生物学的世界の理解と創薬の分野に大きな変革をもたらすと期待されている。生命を構成する分子は、単独では機能せず、何百万通りもの方法で相互作用し、組み合わさっている。AlphaFold 3は、これらの分子がどのように組み合わさっているのか、なぜそのような振る舞いをするのかを明らかにし、病気の理解から植物の成長、問題解決、新たな可能性の創出に至るまで、あらゆる分野でその力を発揮する。実際の応用例として、一般的な風邪ウイルスのスパイクタンパク質と抗体・糖との相互作用や、がん治療のターゲットとなるTim 3タンパク質と薬物様分子の結合を正確に予測することに成功している。従来、タンパク質の立体構造を実験的に決定するには数ヶ月から数年を要していたが、AlphaFold 3はわずか数時間から数日で予測を可能にする。これにより、科学者は最も有望な創薬ターゲットや生物学的課題に集中できるようになる。Googleは、AlphaFold 3を無料で利用できるツール「AlphaFold Server」を公開し、あらゆる生物学者がタンパク質、DNA、RNAなどの重要な分子のモデル化を容易に行えるようにした。
公開日:2024年5月9日
※動画を再生してから読むのがオススメです。
Google DeepMind and IsoMorphic Labs have just surprised the industry by releasing AlphaFold 3 and announcing how good it truly is.
Google DeepMindとIsoMorphic Labsが業界を驚かせ、AlphaFold 3をリリースし、その真の性能の高さを発表しました。
It says AlphaFold 3 predicts the structure and interaction of all of life's molecules.
AlphaFold 3は生命を構成する全ての分子の構造と相互作用を予測すると述べています。
You can see here it states introducing AlphaFold 3 a new AI model developed by Google DeepMind and IsoMorphic Labs by accurately predicting the structure of proteins, DNA, RNA, ligands and more and how they interact.
ここでは、Google DeepMindとIsoMorphic Labsによって開発された新しいAIモデルであるAlphaFold 3が、タンパク質、DNA、RNA、リガンドなどの構造とそれらの相互作用を正確に予測することで紹介されています。
We hope it will transform our understanding of the biological world and drug discovery.
私たちは、それが生物学的世界と創薬の理解を変革することを期待しています。
You have to think about it like this, in every cell of every living thing, plant, animals, even us, there are literally billions of these microscopic machines.
このように考えなければなりません。植物、動物、そして私たち人間を含むあらゆる生物の一つ一つの細胞の中には、文字通り何十億もの微小な機械が存在しているのです。
These machines are made up of proteins, DNA and other funky molecules.
これらの機械はタンパク質、DNA、その他の特殊な分子で構成されています。
The thing is, is that none of these pieces work alone.
重要なのは、これらの部品が単独で機能しないことです。
They're all kind of interacting, combining in millions of ways.
それらは全て何らかの形で相互作用し、何百万通りもの方法で組み合わさっているのです。
Only by seeing that interaction can we actually understand how life works.
その相互作用を見ることによってのみ、私たちは生命がどのように機能するのかを実際に理解することができるのです。
This is where AlphaFold 3 comes in.
ここでAlphaFold 3の出番となります。
They literally just published a picture in Nature Magazine, which we'll get into later, in which they talk about this amazing new AI model.
彼らは後で触れるNature誌に、この驚くべき新しいAIモデルについて述べた写真を文字通り掲載したばかりなのです。
This model can literally predict the structure of life's very molecules and even how they interact with crazy good accuracy.
このモデルは、生命の分子の構造や、それらがどのように相互作用するのかを、信じられないほど高い精度で予測することができるのです。
We're actually talking a significant leap on what we could do before.
私たちは実際に、以前にできたことからの大きな飛躍について話しているのです。
The crazy thing is, is that this is probably about to show us how all those crazy, crazy machines fit together, why they behave the way they do.
驚くべきことに、これはおそらくすべてのクレイジーな機械がどのように組み合わさっているのか、なぜそのような振る舞いをするのかを示そうとしているのです。
This is the key to understanding everything from diseases to how plants grow and how to fix problems or build new awesome stuff.
これは、病気から植物の成長の仕方まで、そして問題を修正したり新しい素晴らしいものを構築したりする方法まで、あらゆることを理解するための鍵なのです。
The details of this are pretty, pretty crazy.
この詳細はかなりクレイジーです。
It's pretty incredible that they've managed to build upon the success of AlphaFold 2, which was the one that changed the game in understanding proteins.
タンパク質の理解においてゲームを変えたAlphaFold 2の成功の上に彼らが築いたことは本当に信じられないことです。
Researchers everywhere actually do use it.
世界中の研究者が実際にそれを使っています。
Think malaria vaccines, cancer research, the entire whole deal.
マラリアワクチン、がん研究、まさに全てのことを考えてみてください。
AlphaFold's already been such a game changer.
AlphaFoldはすでにゲームチェンジャーとなっています。
It's even won major science awards.
それは主要な科学賞さえも受賞しています。
AlphaFold 3, it actually goes way beyond just proteins to all sorts of biomolecules.
AlphaFold 3は、タンパク質だけでなく、あらゆる種類の生体分子にまで及んでいます。
Think eco-friendly materials, stronger crops, supercharged medicine.
環境に優しい材料、より強力な作物、超強力な医薬品などを考えてみてください。
This is pretty much next level science with the power to change the world.
これはまさに世界を変える力を持つ次世代の科学なのです。
How does this actually work?
これは実際にどのように機能するのでしょうか?
Given a list of input molecules, AlphaFold 3 generates their joint 3D structure, revealing how they all fit together.
入力分子のリストが与えられると、AlphaFold 3はそれらの共同3D構造を生成し、それらがどのように組み合わさっているかを明らかにします。
It models large biomolecules such as proteins, DNA and RNA, as well as small molecules, also known as ligands, a category encompassing many different drugs.
タンパク質、DNA、RNAなどの大きな生体分子だけでなく、リガンドとして知られる多くの異なる薬物を含むカテゴリーの小さな分子もモデル化します。
They can model chemical modifications to these molecules, which control the healthy functioning of cells that when disrupted can lead to the disease.
これらの分子の化学修飾をモデル化することができますが、それは細胞の健全な機能を制御し、破壊されると病気につながる可能性があります。
We also see that AlphaFold 3's capabilities come from its next generation architecture and the training that now covers all of life's molecules.
また、AlphaFold 3の機能は、次世代のアーキテクチャと、生命のすべての分子をカバーするようになったトレーニングに由来していることもわかります。
At the core of the model is an improved version of the EVO former module, which is essentially a module that kind of learns the grammar of protein folding by studying evolutionary examples.
モデルの中核には、進化の例を研究することでタンパク質の折りたたみの文法を学習し、言語の文法を学んだ後に新しい文の意味を予測できるのと同じように、その知識を使って新しいアミノ酸配列の3D構造を予測するEVOフォーマーモジュールの改良版があります。
Uses that knowledge to predict the 3D structure of new amino acid sequences, much like how we can kind of predict the meaning of a new sentence after learning the grammar of a language.
タンパク質の折りたたみの文法を進化の例から学習し、その知識を使って新しいアミノ酸配列の3D構造を予測する、言語の文法を学んだ後に新しい文の意味を予測するのと同じような、ディープラーニングアーキテクチャです。
It's a deep learning architecture that underpinned AlphaFold 2's incredible performance.
AlphaFold 2の驚異的なパフォーマンスを支えたものです。
After processing the input, AlphaFold 3 assembles its predictions using a diffusion network akin to those found in AI image generators.
入力を処理した後、AlphaFold 3は、AI画像ジェネレーターに見られるものと同様の拡散ネットワークを使用して予測をアセンブルします。
The diffusion process starts with a cloud of atoms and over many steps converges on its final most accurate molecular structure.
拡散プロセスは原子の雲から始まり、多くのステップを経て最終的に最も正確な分子構造に収束します。
Here's where we have one of the predictions.
ここに予測の一つがあります。
In this, well in this case, what we do have here is we have the ground truth shown in grey and then we have the real one shown in the actual colours.
この場合、グレーで示されているのがグランドトゥルースで、実際の色で示されているのが本物です。
In this example, what we do have here is we have us looking at the spike of a protein of a common cold virus.
この例では、一般的な風邪ウイルスのスパイクタンパク質を見ています。
The spike protein is a part of the virus that helps it infect our cells and the AI model actually accurately predicted how this spike protein interacts with antibodies, which are the immune system's defence proteins that attach the virus and neutralise it and of course simple sugars.
スパイクタンパク質は、ウイルスが私たちの細胞に感染するのを助ける部分で、このAIモデルは、このスパイクタンパク質が抗体(ウイルスに付着してそれを中和する免疫システムの防御タンパク質)や単純な糖とどのように相互作用するかを正確に予測しました。
In this prediction that you're currently seeing on screen, the spike protein is in blue, the antibodies which try to stop the virus are shown in turquoise.
現在画面に表示されているこの予測では、スパイクタンパク質は青、ウイルスを阻止しようとする抗体はターコイズ色で示されています。
The simple sugars are also shown in yellow and these predictions essentially closely match what scientists have observed in real life experiments which are shown in grey.
単純な糖は黄色で示されており、これらの予測は、グレーで示されている実際の実験で科学者たちが観察したものと密接に一致しています。
By using animations of this interaction, scientists can then see exactly how the virus actually interacts with antibodies and sugars.
この相互作用のアニメーションを使用することで、科学者はウイルスが抗体や糖とどのように実際に相互作用するかを正確に知ることができます。
This information is crucial because understanding how these immune system processes helps us Figure out how to fight different viruses including COVID-19 leading to the potential of better treatments and AlphaFold 3 saves so much time by providing accurate predictions that would otherwise require lengthy and expensive laboratory equipments.
これらの免疫システムのプロセスがどのように機能するかを理解することは、COVID-19を含むさまざまなウイルスと戦う方法を解明するのに役立つため、この情報は非常に重要であり、AlphaFold 3は、そうでなければ長くて高価な実験室の機器を必要とする正確な予測を提供することで、多くの時間を節約します。
It does this because determining the 3D structure of proteins using experimental methods like x-ray crystallography or cryo-electron microscopy can take months or even years and AlphaFold 3 can predict these structures in literally hours or days and with predicted structures available, scientists can then focus on the most promising drug targets or biological questions without spending time exploring dead ends.
これは、実験的な方法(X線結晶学やクライオ電子顕微鏡など)を使用してタンパク質の3D構造を決定するのに数ヶ月または数年かかるため、AlphaFold 3はこれらの構造を文字通り数時間または数日で予測することができ、予測された構造が利用可能になると、科学者は時間を無駄にせずに最も有望な薬物標的や生物学的な問題に焦点を当てることができます。
This allows researchers to test hypotheses so there's hypothesis generation where it can generate new hypotheses about how biological molecules function or interact and researchers can then test these hypotheses directly reducing the need for broad exploratory studies.
これにより、研究者は仮説を検証することができ、生物分子がどのように機能したり相互作用したりするかについて新しい仮説を生成することができ、研究者はこれらの仮説を直接テストすることができ、広範な探索的研究の必要性が減少します。
There was also another example here in which we can see Tim 3.
ここにはTim 3というもう一つの例がありました。
This is a protein that researchers are studying because it might be useful/a useful target for cancer treatments and scientists found a way to create small molecules that could stick to this protein and potentially block the harmful effects.
これは、がん治療に有用なターゲットになる可能性があるため、研究者が研究しているタンパク質です。科学者たちは、このタンパク質に付着し、有害な影響をブロックできる可能性のある小さな分子を作る方法を見つけました。
Before this study there weren't any clear images showing how these small drug-like molecules would actually fit into the Tim 3 structure and without this information designing effective drugs was pretty challenging.
この研究の前には、これらの小さな薬のような分子がTim 3構造にどのように実際にはまるのかを示す明確な画像はありませんでした。この情報がなければ、効果的な薬を設計することはかなり困難でした。
Essentially AlphaFold 3 comes in.
本質的にAlphaFold 3が登場します。
Scientists use AlphaFold 3 to predict what Tim 3 would look like when these small molecules bind to it and they only provided the AI with the protein sequence which is essentially the recipe and a simple description of the drug-like molecules.
科学者たちは、これらの小さな分子がTim 3に結合したときにどのように見えるかを予測するためにAlphaFold 3を使用しました。AIにはタンパク質配列(本質的にはレシピ)と薬のような分子の単純な記述しか提供しませんでした。
That's where we get to the outcome.
そこで私たちは結果にたどり着きます。
AlphaFold 3 actually predicted how these molecules would fit together perfectly into the pocket of the Tim 3 protein aligning almost exactly with the structures that scientists discovered through experiments.
AlphaFold 3は、これらの分子がTim 3タンパク質のポケットに完璧にはまり、科学者が実験で発見した構造とほぼ完全に一致すると実際に予測しました。
It also showed that without these drug molecules present the pocket actually looked different proving that AlphaFold 3 can actually recognize changes in protein shapes when other molecules are around and this essentially means that AlphaFold 3 can predict how drug molecules will interact with proteins more accurately than ever before and this is going to lead to faster and more efficient drug discovery helping scientists develop better treatments for cancer and other diseases.
また、これらの薬分子が存在しない場合、ポケットは実際には異なって見えることを示し、AlphaFold 3が他の分子が周りにあるときのタンパク質の形状の変化を実際に認識できることを証明しました。これは本質的に、AlphaFold 3が薬の分子がタンパク質とどのように相互作用するかをこれまで以上に正確に予測できることを意味し、がんやその他の病気のためのより良い治療法を開発する科学者を助けることで、より迅速で効率的な創薬につながるでしょう。
Of course it makes sense to talk about isoMorphic labs.
もちろん、アイソモルフィックラボについて話すのは理にかなっています。
One of the things that they talk about leading the drug discovery at isoMorphic labs is that AlphaFold 3 is 50% more accurate than the best traditional methods on the Pose busters benchmark without needing the input of any structural information making AlphaFold 3 the first AI system to surpass physics-based tools for biomolecular structure prediction.
彼らがアイソモルフィックラボでの創薬をリードしていると話している点の一つは、AlphaFold 3がPosebustersベンチマークにおいて、構造情報の入力を必要とせずに、従来の最良の方法よりも50%以上正確であり、AlphaFold 3を生体分子の構造予測のための物理ベースのツールを超える最初のAIシステムにしていることです。
IsoMorphic labs is using AlphaFold 3 to accelerate and improve the success of drug design by helping understand how to approach new disease targets and developing novel ways to pursue existing ones that were previously out of reach.
アイソモルフィックラボは、新しい疾患ターゲットへのアプローチ方法を理解し、以前は手の届かなかった既存のターゲットを追求する新しい方法を開発することで、創薬の成功を加速し改善するためにAlphaFold 3を使用しています。
They also talk about how we can now create and test hypotheses at the atomic level and produce highly accurate structure predictions within seconds standing in stark contrast to the months or even years required to experimentally determine answers to similar questions.
また、原子レベルで仮説を作成してテストし、数秒以内に非常に正確な構造予測を生成できるようになったことについても述べており、これは同様の質問に対する答えを実験的に決定するのに必要とされる数ヶ月あるいは数年とは対照的です。
They also talk about how they're using this.
また、彼らはこれをどのように使用しているかについても述べています。
They state that already we are using AlphaFold 3 day to day.
彼らは、すでに私たちは日々AlphaFold 3を使用していると述べています。
Our scientists have seen that designing small molecules against AlphaFold 3's structural predictions helps create designs that bind effectively to a target protein.
私たちの科学者は、AlphaFold 3の構造予測に対して小分子を設計することが、標的タンパク質に効果的に結合する設計の作成に役立つことを確認しました。
The improved structural accuracy of protein-to-protein interfaces with AlphaFold 3 opens up the possibility of designing new treatment modalities, such as antibodies or other therapeutic proteins.
AlphaFold 3によるタンパク質間インターフェースの構造精度の向上により、抗体やその他の治療用タンパク質などの新しい治療法を設計する可能性が開けます。
A richer understanding of a novel target can be achieved by looking at the structure of targets in their full biological context, in complex with other protein binding partners, DNA, RNA, and ligand cofactors.
新規ターゲットのより豊富な理解は、他のタンパク質結合パートナー、DNA、RNA、リガンド補因子との複合体における、完全な生物学的文脈でのターゲットの構造を見ることで得られます。
Of course, there was a paper that was released with the AlphaFold 3 release.
もちろん、AlphaFold 3のリリースと共に論文が発表されました。
However, this is currently an Accelerated Article Preview (an AAP), which is a version of a scientific article that's published online before it appears in a traditional print journal.
しかし、これは現在、Accelerated Article Preview(AAP)となっており、これは伝統的な印刷ジャーナルに掲載される前にオンラインで公開される科学論文のバージョンです。
It basically just means that it's designed to get important research findings out to scientists and the public as quickly as possible.
基本的には、重要な研究成果を科学者や一般の人々にできるだけ早く伝えることを目的としています。
They kind of skip some of the editing and formatting that can actually slow down a traditional journal publication.
伝統的なジャーナル出版を遅らせる可能性のある編集やフォーマットの一部をスキップしているのです。
That means there is a watermark on each page to remind you that these aren't the final polished versions.
つまり、これらが最終的な洗練されたバージョンではないことを思い出させるために、各ページに透かしが入っているのです。
Here's where we actually talk about the main thing, which is, of course, the AlphaFold server.
ここでは、もちろんAlphaFoldサーバーについて、主なことを実際に話しています。
Google actually launched this tool that helps you do this stuff, so scientists can use it for free.
Googleは実際に、この作業を支援するツールを無料で科学者が使えるようにしました。
There's no fancy subscription needed.
特別な購読は必要ありません。
Basically, with a few clicks, any biologist can use AlphaFold 3 to make models of proteins, DNA, RNA, and other important molecules.
基本的に、数回のクリックで、どんな生物学者でもAlphaFold 3を使ってタンパク質、DNA、RNA、および他の重要な分子のモデルを作成することができます。
This is pretty huge because the AlphaFold server lets scientists quickly come up with new ideas to test out in the lab.
これはかなり大きなことです。なぜなら、AlphaFoldサーバーを使えば、科学者たちは素早く新しいアイデアを考え出して実験室で試すことができるからです。
This cuts down the time wasted on guesswork.
これにより、推測に無駄な時間を費やすことが減ります。
Just picture your science projects going way faster, and remember, this used to take a huge amount of time.
科学プロジェクトがはるかに速く進む様子を想像してみてください。覚えておいてください、これには以前は膨大な時間がかかっていました。
All of that is being saved.
それが全て節約されているのです。
This is the quick trailer I'll leave you with, and if you need any of the links, they'll be either in a top comment or a link in the description.
これが私が最後に残すクイックトレーラーで、リンクが必要な場合は、トップコメントまたは説明のリンクにあります。
この記事が気に入ったらサポートをしてみませんか?