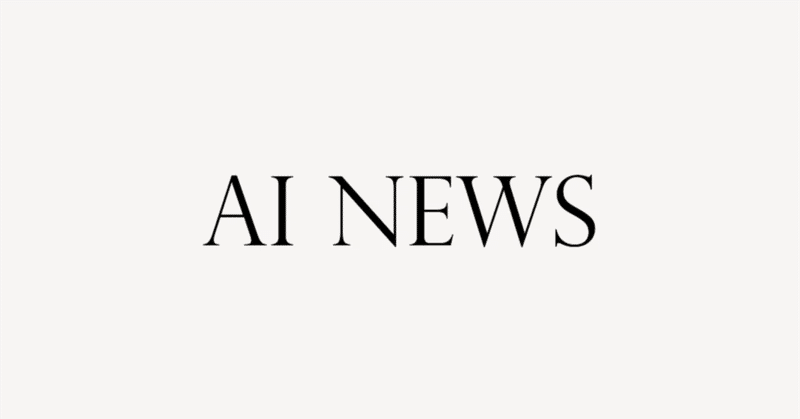
【Med-Gemini:医療現場に革新をもたらすGoogleの最新AI】英語解説を日本語で読む【2024年5月3日|@TheAIGRID】
GoogleのDeepMindとGoogle Researchは、会社のAI「Gemini」を基にして、医療分野に特化した「Med-Gemini」という研究論文を発表しました。Med-Geminiは、これまでのAIシステムが抱えていたベンチマークの曖昧さを克服し、医療現場で高い精度を実現しています。Med-Geminiの特長は、自己学習と検索機能を活用した高度な推論能力にあります。研究によると、Med-Geminiを実際の医師と比較した場合、診断精度で医師を上回る結果が得られました。Med-Geminiは、医師とのコミュニケーションを支援するAMIEとは異なり、膨大な医療データを分析し、診断と治療計画の立案を支援することに特化しています。将来的には、患者情報の総合的な分析を通じて、医療従事者のより適切な意思決定をサポートすることが期待されます。
公開日:2024年5月3日
※動画を再生してから読むのがオススメです。
Google DeepMind and Google Research have released a very interesting research paper called the capabilities of Gemini models in medicine.
Google DeepMindとGoogle Researchは、医療におけるGeminiモデルの能力と題した非常に興味深い研究論文を発表しました。
Essentially what they've done is they've made a paper discussing and showing how Google's Gemini model can be fine-tuned and turned into something that can be used very very effectively for helping out in the medical industry.
基本的に彼らがしたことは、GoogleのGeminiモデルがどのようにファインチューニングされ、医療業界を非常に効果的に支援するために使用できるものに変えられるかを議論し、示す論文を作成したことです。
This is quite surprising because I didn't expect such a system from Google just yet but they also released something earlier this year which was actually pretty similar.
これはGoogleからまだこのようなシステムを期待していなかったので、かなり驚きました。しかし、彼らは今年の初めにも実際にかなり似たようなものをリリースしていました。
If you remember this is something that I spoke about and this was AMIE which was Articulate Medical Intelligence Explorer.
覚えていると思いますが、これは私が話したことで、アーティキュレート・メディカル・インテリジェンス・エクスプローラー(Articulate Medical Intelligence Explorer)というAMIEでした。
It was an advanced AI research system developed by Google and this one which was released around three to four weeks ago.
これは、Googleが開発した高度なAI研究システムで、3〜4週間前にリリースされたものです。
It was basically designed to handle diagnostic reasoning and engage in meaningful conversations within a medical context aiming to enhance the interactions between physicians and patients as well as improve the quality and accessibility of consultations.
基本的に、診断推論を扱い、医療の文脈で意味のある会話を行うように設計されており、医師と患者の相互作用を強化し、相談の質とアクセシビリティを向上させることを目的としていました。
Basically the reason that AMIE was so good is because it was able to use a simulated learning environment to enhance its learning and it engaged in diagnostic dialogues with AI patient simulators allowing it to practice and redefine and refine its conversational and diagnostic skills continually.
基本的にAMIEが優れていた理由は、シミュレーション学習環境を使用して学習を強化することができたからです。そして、AI患者シミュレータとの診断対話に従事し、会話と診断のスキルを継続的に練習し、再定義し、改善することができました。
It was actually trained on a huge diverse set of medical data including real world clinical conversations with medical reasoning scenarios.
実際には、医療推論シナリオを含む現実世界の臨床会話など、非常に多様な医療データで訓練されていました。
One of the key things about AMIE that when it was actually pitted against clinicians it showed us that this was something that was far effective if humans used it in the loop.
AMIEについての重要なことの1つは、実際に臨床医と比較したとき、人間がループの中でそれを使用すれば、これは非常に効果的であることが示されたということです。
You can see right here on this graph we can literally see that with AMIE only the system performed increasingly better than the clinician unassisted and the clinician was assisted by search and search is essentially just the internet which shows a huge improvement in the gaps and then we can see that assisted by AMIE is a far stark increase from just the clinician unassisted.
このグラフでは、AMIEのみのシステムが、支援なしの臨床医や検索で支援された臨床医よりも着実に優れた性能を示し、ギャップの大幅な改善を示していることがわかります。そして、AMIEによって支援された場合は、支援なしの臨床医よりもはるかに大幅な増加が見られます。
Of course AMIE only compared to the clinician assisted by AMIE shows us that AMIE actually did surpass the actual clinician.
もちろん、AMIEのみと比較して、AMIEによって支援された臨床医は、AMIEが実際の臨床医を凌駕したことを示しています。
Basically what this showed us, okay, and I know this isn't Med-Gemini just yet, but this is basically showing us that Google's increasing their efforts for medical health in terms of research because they're showing us that these AI systems like AMIE are far superior than just the clinicians.
基本的にこれが示したのは、まだMed-Geminiではありませんが、Googleが研究の面で医療健康への取り組みを強化していることです。なぜなら、AMIEのようなこれらのシステムが臨床医だけよりもはるかに優れていることを示しているからです。
Essentially, like I said, this is of course Med-Gemini, so what we have here is we have the initial Gemini system that exists here.
本質的に、言ったように、これはもちろんMed-Geminiです。ここで私たちが持っているのは、ここに存在する初期のGeminiシステムです。
Gemini is a family of powerful AI systems that are completely multimodal.
Geminiは、完全にマルチモーダルな強力なAIシステムのファミリーです。
You can see that there are the inherited capabilities such as the advanced reasoning, the multimodal understanding, and the long context processing.
高度な推論、マルチモーダルな理解、長い文脈処理などの継承された機能があることがわかります。
This is where they decided to have the development for Med-Gemini.
ここでMed-Geminiの開発を行うことを決めたのはここです。
They did medical specialization with self-training with web search integration, and of course the multimodal understanding.
ウェブ検索の統合による自己学習による医療専門化を行いました。そしてもちろん、マルチモーダルな理解です。
They did fine-tuning and customized encoders, and of course with the long context processing, they did chain of reasoning prompting.
ファインチューニングとカスタムエンコーダを行い、もちろん長い文脈処理では、推論プロンプティングのチェーンを行いました。
With all of these skills combined, that's where we now get, of course, Med-Gemini, this version of Gemini which is specialized for medical applications.
これらすべてのスキルを組み合わせることで、もちろん、医療アプリケーション用に特化したGeminiのバージョンであるMed-Geminiを得ることができるようになりました。
It's very, very fascinating because if you're someone that hasn't been paying attention to this space, this is an industry that is truly about to be disrupted because the applications and the things that we're seeing show us that the benchmarks are looking pretty incredible in terms of the applications.
この分野に注意を払っていない人にとっては、これは本当に破壊されようとしている業界であるため、非常に興味深いことです。なぜなら、私たちが見ているアプリケーションと事物は、アプリケーションの観点からベンチマークがかなり信じられないほど見えることを示しているからです。
One of the things that was there before was, of course, the previous state of the art.
以前にあったものの1つは、もちろん、以前の最先端技術でした。
The previous state of the art in terms of in the industry for medical AI systems that are able to talk and that are able to answer certain questions and queries in terms of the accuracy, you can see that here we can see from September 21st all the way to September 2023, there's been a huge increase in terms of what these AI systems have been able to do.
業界における医療AIシステムの最先端技術は、話すことができ、特定の質問やクエリに答えることができる正確さの点で、ここで2021年9月から2023年9月までの間に、これらのAIシステムができることの面で大幅な増加があったことがわかります。
Noticeably, the jump from GPT-3.5 to Google's med palm, then of course to GPT-4 and med palm 2, and the previous state of the art model which was before Med-Gemini.
特に、GPT-3.5からGoogleのMed-PaLMへ、そしてもちろんGPT-4とMed-PaLM2への飛躍的な進歩、そしてMed-Gemini以前の最先端モデルが注目に値します。
Of course, now what we do have is we do have a state of the art system which is medical Gemini, the state of the art system before the one that was released today with Google.
もちろん、現在私たちが持っているのは、医療用Geminiという最先端のシステムです。今日Googleでリリースされる前の最先端システムです。
Well, not actually released, but the paper was released, was actually GPT-4.
まあ、実際にはリリースされていませんが、論文はリリースされました。実際にはGPT-4でした。
Not just the base version, which the GPT-4 base version was very close to Google's medical one.
ベースバージョンだけでなく、GPT-4ベースバージョンはGoogleの医療用のものに非常に近かったのです。
It's actually GPT-4 with a fine-tuned version that gets it 19.2 percent on the MedQA, which is a decent benchmark for these AI systems.
実際には、これらのAIシステムの適切なベンチマークであるMedQAで19.2パーセントを取得するファインチューニングバージョンのGPT-4です。
GPT-4 with the med prompt actually had a very high benchmark, but once again, Google has beaten them now with GPT-4 and med prompt.
medプロンプトを使用したGPT-4は実際には非常に高いベンチマークを持っていましたが、再びGoogleはGPT-4とmedプロンプトで今それらを打ち負かしました。
The reason I'm showing you guys this infrastructure is because this shows us how crazy it actually is.
この基盤をお見せしているのは、これが実際にどれほどクレイジーであるかを示しているからです。
This takes us from the base level of GPT-4, and you can see all of the different things that they've added to GPT-4 in order to get the system to perform a lot better.
これはGPT-4の基本レベルから始まり、システムのパフォーマンスを大幅に向上させるためにGPT-4に追加されたさまざまなものを見ることができます。
What's crazy is that Med-Gemini, the reason why it's so effective and we're about to get into that, is because it doesn't use all of these crazy techniques like the ensemble with choice shuffle.
クレイジーなのは、Med-Geminiが非常に効果的な理由であり、それについて説明しようとしているのは、選択シャッフルを使用したアンサンブルのようなこれらのクレイジーなテクニックのすべてを使用していないからです。
If you don't know what that is, basically in multiple choice questions, sometimes there is bias rated towards the first question in terms of like the answer.
それが何であるかわからない場合は、基本的に多肢選択式の質問では、答えに関して最初の質問に偏りがある場合があります。
For example, if you were to ask someone what is the primary gas found in Earth's atmosphere, and if you were to have four answers, the first one, a lot of people subconsciously might think that that one is correct.
たとえば、地球の大気中で見つかった主要なガスは何かと誰かに尋ねた場合、4つの答えがあるとしたら、多くの人が無意識のうちに最初の答えが正しいと思うかもしれません。
Essentially what you do is you shuffle them.
基本的に行うことは、それらをシャッフルすることです。
When the most common answer is picked among these, you can then find out that answer, and then that's the one that you use.
これらの中で最も一般的な答えが選ばれると、その答えを見つけることができ、それを使用する答えになります。
It's pretty crazy.
それはかなりクレイジーです。
You can see how many different iterations that they did on top of GPT-4 to get to 90.2.
90.2に到達するためにGPT-4の上にどれだけ多くの異なる反復を行ったかがわかります。
Surprisingly, they managed to surpass this benchmark.
驚くべきことに、彼らはこのベンチマークを上回ることに成功しました。
You can see right here that this is where Google's Med-Gemini comes in on the MedQA at 91.1%, which is very interesting in terms of the increase.
ここでGoogleのMed-GeminiがMedQAで91.1%となっているのがわかります。これは増加の面で非常に興味深いことです。
I guess some people could argue that maybe we are starting to peter out in terms of the capabilities of Large Language Models on these benchmarks.
大規模言語モデルのこれらのベンチマークでの能力の面で、私たちがピーターアウトし始めているのかもしれないと主張する人もいるかもしれません。
But I would certainly disagree because whilst yes, this might be the truth, Med-Gemini is pretty great.
しかし、私は確かに反対します。なぜなら、はい、これは事実かもしれませんが、Med-Geminiはかなり優れているからです。
One of the key things you do need to know about the benchmarks is that you can see here it says relabeling with expert clinicians suggests that 7.4 percent of the questions in the data set have quality issues or ambiguous ground truth.
ベンチマークについて知っておく必要がある重要なことの1つは、専門家の臨床医による再ラベル付けでは、データセットの7.4%の質問に品質の問題があるか、あいまいな基礎的事実があることが示唆されていることがわかります。
Essentially, one of the things that have been consistently problematic in the AI benchmarking industry was the fact that these kind of systems unfortunately have to go with benchmarks that are pretty standard.
本質的に、AIベンチマーク業界で一貫して問題となっていたことの1つは、このような種類のシステムが残念ながらかなり標準的なベンチマークに従わなければならないという事実でした。
But these benchmarks contain thousands and thousands of questions, but some of these questions are quite ambiguous and they have quality issues, meaning that the AI systems can't really even get any of the answers correct because the questions literally don't make sense.
しかし、これらのベンチマークには何千もの質問が含まれていますが、これらの質問の一部はかなりあいまいで、品質の問題があります。つまり、AIシステムは、質問が文字通り意味をなさないため、正解を得ることができません。
I wish I did have some examples to show you, but just trust me when I tell you some of the questions are completely insane.
お見せできる例があればいいのですが、質問の中にはまったく理解できないものがあるということを信じてください。
What they're stating is that 7.4 percent of this might not even be that great because the benchmarks are potentially facing several quality issues.
彼らが述べているのは、ベンチマークがいくつかの品質問題に直面している可能性があるため、この7.4%はそれほど優れているとは言えないということです。
Whilst you might think that this is going to be something that peters out in the future, we do know that more advanced reasoning systems could take this to 100 in terms of not only the system being good but of course the benchmarks performing a lot better when those quality issues do get fixed.
これが将来ピーターアウトするものだと思うかもしれませんが、より高度な推論システムがこれを100にすることができることを私たちは知っています。システムが優れているだけでなく、もちろん、それらの品質問題が修正されたときにベンチマークがはるかに優れたパフォーマンスを発揮することができるようになります。
One of the things we could see as well about the medical benchmarking here as we can see that there are several categories in which Med-Gemini actually surpasses the previously state of the art.
ここで医療ベンチマークについても見ることができるのは、Med-Geminiが以前の最先端技術を上回るいくつかのカテゴリーがあるということです。
The blue one here is of course the main focus and we can see that the blue one is Gemini and this surpasses the previous state of the art which is GPT-4 with med prompt in every single category, well nearly every single category.
ここの青いものがもちろん主な焦点で、青いものがGeminiであり、これはほぼすべてのカテゴリーで、以前の最先端技術であるGPT-4とmedプロンプトを上回っていることがわかります。
There are some ones right here where it is pretty much on par and only this one where it's on you know long context video.
ほぼ同等のものがいくつかありますが、長い文脈のビデオに関してはこの1つだけです。
But you can even see right here it says GPT-4 results not available due to context length limitations and the advanced text reasoning you can see here that this is done pretty well, the multimodal understanding it's done pretty well as well.
しかし、ここでさえ、GPT-4の結果は、コンテキストの長さの制限により利用できないと書かれています。高度なテキスト推論は、ここではかなりうまくいっていることがわかります。マルチモーダルな理解もかなりうまくいっています。
Essentially if you just want to take a look at this in terms of how much better it is, if we just take a look at this line we can see that anything above this line is something that is an improvement and we can see that in pretty much near all of these categories we do have a decent improvement.
本質的に、これがどれだけ優れているかを見たい場合は、この線を見るだけです。この線より上のものは改善されていることがわかり、これらのカテゴリーのほぼすべてでかなりの改善があることがわかります。
One of the things here is the medical Gemini on advanced text based reasoning tasks and you can see here that based on the previous state of the art it surpasses it in many different categories.
ここでの1つは、高度なテキストベースの推論タスクに関する医療用Geminiです。ここでは、以前の最先端技術に基づいて、多くの異なるカテゴリーでそれを上回っていることがわかります。
One of the things that they actually did talk about is that they actually did talk about how this system compared with GPT-4 in some scenarios.
彼らが実際に話していたことの1つは、このシステムがGPT-4といくつかのシナリオでどのように比較されたかについて実際に話したことです。
GPT-4 just didn't actually have the context length to support that on long context reasoning.
GPT-4は、長い文脈の推論でそれをサポートするのに十分な文脈の長さを実際には持っていませんでした。
It's important to know that long context reasoning with Google's new context length it's actually a pretty important feature for the future because it allows us to process more information.
Googleの新しいコンテキストの長さを使用した長いコンテキストの推論は、将来にとって非常に重要な機能であることを知っておくことが重要です。なぜなら、より多くの情報を処理することができるからです。
The thing is with the medical industry, the more data you have, the more comprehensive of a picture you do have because a human body is made up of so many different intricate parts and because they all connect together, if you have a long context window, you're able to fit more data in and arguably get to a better conclusion about what the diagnosis may be or what's going wrong with a certain individual's body.
医療業界では、データが多いほど、より包括的な画像が得られます。なぜなら、人体は非常に多くの複雑な部分で構成されており、それらすべてが一緒に接続されているからです。長い文脈ウィンドウがある場合は、より多くのデータを適合させ、診断が何であるか、または特定の個人の体に何が問題なのかについてより良い結論を得ることができると言えます。
It's important to have that for the future especially in terms of the needle in the haystack which is where you're trying to get that data from a long piece of context and use it correctly and of course reason with that correctly.
特に、長い文脈の一部からそのデータを取得し、それを正しく使用し、もちろんそれを正しく推論しようとしている針の中の干し草の点で、将来のためにそれを持つことが重要です。
You can see Gemini, well Med-Gemini surpasses state of the art and we can of course see it here as well compared to AMIE where it does surpass that as well.
Gemini、まあMed-Geminiが最先端を凌駕していることがわかりますし、もちろんここでもAMIEと比較して、それを凌駕していることがわかります。
Of course some of these aren't that crazy but it seems like maybe there's going to be some advanced reasoning techniques now.
もちろん、これらのいくつかはそれほどクレイジーではありませんが、おそらくいくつかの高度な推論技術があるようです。
One of the things that I did actually see that was pretty cool was that the advanced reasoning that we did see was a little bit different.
私が実際に見てかなりクールだと思ったことの1つは、私たちが見た高度な推論が少し異なっていたということです。
They had two kind of ways that they did advanced reasoning with this and I think that this is probably what we're going to see in terms of different models that are specialized for different use cases.
彼らには、これを使って高度な推論を行う2種類の方法がありました。そして、これは、さまざまなユースケースに特化したさまざまなモデルの観点から、おそらく私たちが見ることになるものだと思います。
In the case of Med-Gemini, they essentially had self-training and search and these were leveraged to enhance its capabilities in handling complex medical data and queries.
Med-Geminiの場合、彼らは本質的に自己学習と検索を行いました。そして、これらは、複雑な医療データとクエリを処理する能力を高めるために活用されました。
Let's actually dive into how these features were used by Med-Gemini.
これらの機能がどのようにMed-Geminiによって使用されたかについて実際に掘り下げてみましょう。
The self-training aspect with Med-Gemini was where you use the model's own outputs to generate new training examples which are then used to further improve the model.
Med-Geminiでの自己学習の側面は、モデル自身の出力を使用して新しいトレーニング例を生成し、それをさらにモデルの改善に使用するところでした。
Apologies for that coming off.
それが外れたことをお詫びします。
This method is particularly beneficial for refining the model's capabilities in an area where the initial training data might be limited or lack diversity.
この方法は、初期のトレーニングデータが限られているか、多様性に欠ける分野でモデルの能力を改善するのに特に有益です。
Essentially, what they do is the first thing that they do is they generate synthetic examples.
本質的に、彼らが行うことは、まず最初に合成例を生成することです。
For example, Med-Gemini processes medical data or queries and then generates responses based on its current understanding.
たとえば、Med-Geminiは医療データやクエリを処理し、現在の理解に基づいて応答を生成します。
These responses, along with the context in which they were made, serve as new training examples.
これらの応答は、それらが行われたコンテキストとともに、新しいトレーニング例として役立ちます。
We have its refinement.
私たちはその洗練を持っています。
These generated examples are fed back into the training cycle, allowing Med-Gemini to learn from its own outputs.
これらの生成された例はトレーニングサイクルにフィードバックされ、Med-Geminiが自身の出力から学習できるようにします。
This iterative process helps the model to continually refine its reasoning and decision-making capabilities, especially in handling complex medical scenarios.
この反復プロセスは、特に複雑な医療シナリオを扱う際に、モデルの推論と意思決定の能力を継続的に洗練するのに役立ちます。
We have enhanced learning from simulators.
シミュレーターからの強化学習があります。
This is where, for Med-Gemini, simulations could involve creating scenarios where the model must interpret complex medical data from text, images, or even long medical records.
これは、Med-Geminiの場合、シミュレーションでは、モデルがテキスト、画像、または長い医療記録から複雑な医療データを解釈しなければならないシナリオを作成することを含む可能性があります。
Feedback from the simulations helps the model adjust its methods for better accuracy and reliability.
シミュレーションからのフィードバックは、より高い精度と信頼性のためにモデルがその方法を調整するのに役立ちます。
Of course, we have the search for Med-Gemini.
もちろん、Med-Geminiの検索があります。
This is where we have Med-Gemini when it actually encounters a question that it might struggle with, has low confidence, or insufficient internal data.
これは、Med-Geminiが実際に苦労するかもしれない質問に遭遇したり、自信が低かったり、内部データが不十分だったりする場所です。
It can perform a web search to gather additional information.
追加情報を収集するためにウェブ検索を実行できます。
Like here, you can see it says confident, no.
ここでは、自信がないと言っているのがわかります。
Of course, we go to here.
もちろん、ここに行きます。
Of course, we go to here, which is web search.
もちろん、ここに行きます。これはウェブ検索です。
Of course, there's an uncertainty-guided search where essentially Med-Gemini employs an uncertainty-guided search strategy where the model calculates that its predictions have high uncertainty and it proactively searches for more information before finalizing its response.
もちろん、不確実性に導かれた検索があります。本質的にMed-Geminiは、モデルがその予測に高い不確実性があると計算し、応答を最終決定する前により多くの情報を積極的に検索する不確実性誘導検索戦略を採用しています。
This method helps in the accuracy and reliability of its outputs.
この方法は、出力の精度と信頼性に役立ちます。
This is the loop that it conducts in order to get better results.
これは、より良い結果を得るために行うループです。
Of course, we have continuous update of the knowledge.
もちろん、知識の継続的な更新があります。
The ability to search and integrate information from external sources means that Med-Gemini can continuously update its knowledge base without the need for frequent retraining.
外部ソースから情報を検索して統合する機能があるということは、Med-Geminiが頻繁な再トレーニングを必要とせずに知識ベースを継続的に更新できることを意味します。
This is actually pretty crucial in the medical field where new research and clinical practices can change standard care protocols.
これは、新しい研究と臨床実践が標準的なケアプロトコルを変更する可能性がある医療分野では実際には非常に重要です。
By combining these two approaches that we have here, it can better adapt to new or rare medical scenarios.
ここにあるこれら2つのアプローチを組み合わせることで、新しいまたはまれな医療シナリオにより適応できます。
It has access to the latest medical information via search, which means that its data is not only based on the initial training data but also the latest studies, clinical trials, and guidelines.
検索を通じて最新の医療情報にアクセスできるため、そのデータは初期のトレーニングデータだけでなく、最新の研究、臨床試験、ガイドラインにも基づいています。
Through the continuous learning and adaptation, it becomes more proficient at handling diverse and complex medical queries, making it a valuable tool for medical professionals seeking ai support.
継続的な学習と適応により、多様で複雑な医療クエリを処理することにより熟練し、AIサポートを求める医療専門家にとって貴重なツールになります。
Of course, here are the video benchmarks, and this is something where it's pretty good.
もちろん、ここにビデオベンチマークがあります。これは非常に優れているものです。
We do know that Google's Gemini 1.5 Pro can actually take in, I think, an hour of video, which means it can also look at these videos and analyze the scene in a medical way.
GoogleのGemini 1.5 Proは実際に1時間のビデオを取り込むことができ、これらのビデオを見て医療の観点からシーンを分析することもできることを私たちは知っています。
You can see right here the states.
ここに州が見えます。
Here you can see the previous state of the art where we've got Med-PaLM and GPT-4 Vision.
ここでは、Med-PaLMとGPT-4 Visionという以前の最先端技術が見られます。
I'm wondering what would happen when GPT-4 releases the video, how it's going to compete with Medical Gemini on this as well, which is going to be pretty crazy.
GPT-4がビデオをリリースしたとき、これもMedical Geminiと競合することになるのは非常にクレイジーになるだろうと思っています。
Of course, here is where we have the capabilities of Gemini models in medicine, and this is where we have the actual benchmarks.
もちろん、ここは医療におけるGeminiモデルの機能があるところで、実際のベンチマークがあります。
You can see right here, this is where we have the benchmark, and you can see the accuracy from the clinician, then the clinician and search, then the prior state of the art, then of course the Med-Gemini, then the Med-Gemini plus search.
ここでベンチマークがあり、臨床医の精度、検索での臨床医、以前の最先端技術、そしてもちろんMed-Gemini、Med-Gemini+検索の精度が見られます。
We can see a stark improvement like I showed you guys before with, of course, AMIE.
前に皆さんにお見せしたように、AMIEと比べて大幅な改善が見られます。
This is the AMIE stuff right here, is what I'm guessing.
これはAMIEのものだと思います。
This is, of course, the GPT-4 or AMIE.
これは、もちろん、GPT-4またはAMIEです。
I'm not sure which one, but what we do have here is a clear conclusion from this graph.
どちらかわかりませんが、ここから明確な結論が得られます。
Just on this part, we can see that the clinician is actually the lowest rated one in terms of accuracy.
このグラフのこの部分だけでも、臨床医の精度が実際に最も低いことがわかります。
If we go all the way up here, we can see that Med-Gemini plus search gives us a huge increase in terms of performance.
ここまで来ると、Med-Geminiに検索を加えることで、パフォーマンスが大幅に向上することがわかります。
There is a huge gap right here in terms of what's being done, and I think that is rather impressive on how AI is able to bridge this knowledge gap for clinicians and help them.
ここで行われていることには大きなギャップがあり、AIが臨床医の知識のギャップを埋め、彼らを助けることができるのは非常に印象的だと思います。
I definitely think that this is something that could provide a lot of details and help to people.
これは間違いなく、人々に多くの詳細と助けを提供できるものだと思います。
The only thing that I do think could be problematic here is that hopefully humans don't completely rely on the AI because sometimes the AI might miss certain things in diagnoses, and I think humans always have a lot more data points than an AI system.
ここで問題になると思うのは、人間がAIに完全に頼らないことを願っています。なぜなら、時にはAIが診断で特定のものを見逃す可能性があるからです。そして、人間は常にAIシステムよりも多くのデータポイントを持っていると思います。
Because something that I've and this is a little bit off tangent, but it does make sense here, is that if you are prompting GPT-4 or any other AI system, sometimes you'll think, Why can't this system get what I want to go out?
少しそれましたが、ここでは意味があることです。GPT-4やその他のAIシステムにプロンプトを与えている場合、このシステムが私が外に出たいと思っていることを理解できないのはなぜだろうと時々考えるでしょう。
But if you give the system every single piece of information that you can, like, for example, if you're asking it how to write a certain essay and if you say when the deadline is, if you say what your teacher is like, if you say the style, if you say the length, if you say a few things you need, the more information you give these systems, the much better they are at essentially giving you the output that you want.
しかし、特定のエッセイの書き方を尋ねているときなど、締め切り、先生の好み、スタイル、長さ、必要なことをいくつか言えば、システムにできるだけ多くの情報を与えるほど、本質的に望む出力を与えるのにはるかに優れています。
That's something personally that I've seen, and that's why I state that the reasons humans would have a lot more information is because a human might go to a system and be like, Oh, I have a runny nose.
これは個人的に見てきたことであり、だからこそ人間の方がはるかに多くの情報を持っていると述べています。なぜなら、人間はシステムに行って、「ああ、鼻水が出ている」と言うかもしれないからです。
But if you do, I have a runny nose to an AI system, that's the only piece of information it has, whereas a human who's in the room with you can see how your skin looks, it can see how you're moving around, it can see if you're fatigued, it knows your age, it knows what you look like, it knows your family tree.
しかし、AIシステムに「鼻水が出ている」と言っても、それが持っている唯一の情報です。一方、あなたと同じ部屋にいる人間は、あなたの肌の様子、動き回り方、疲れているかどうか、年齢、外見、家系図を知っています。
The point here is that I do think that this is going to definitely improve as we move on in the future, and hopefully this can be a very good tool for clinicians in the future.
ここでのポイントは、これが将来改善されていくと思いますし、これが臨床医にとって非常に良いツールになることを願っています。
Like I said before, whilst yes, this is doing well, there are some problematic benchmarks you can see here.
前に言ったように、はい、これはうまくいっていますが、いくつかの問題のあるベンチマークがあります。
As I spoke about previously revisiting the MedQA benchmarks, there were some probabilities.
以前にMedQAベンチマークを再検討したところ、いくつかの確率がありました。
However, some MedQA test questions have missing information, such as figures or lab results, and potentially outdated ground truth answers.
しかし、一部のMedQAテスト質問には、図や検査結果などの情報が欠けていたり、地上の真実の答えが古くなっている可能性があります。
To address these concerns, they had at least three U.S. doctors review each question to answer the question themselves, check if the original answers were still correct, and note any missing details or ambiguous elements in these questions.
これらの懸念に対処するために、彼らは少なくとも3人の米国人医師に各質問をレビューさせ、自分で質問に答え、元の答えがまだ正しいかどうかを確認し、これらの質問で欠落している詳細やあいまいな要素を指摘させました。
They used a method called bootstrapping, where a committee of three reviewers decided if a question should be excluded due to its flaws.
彼らは、ブートストラッピングと呼ばれる方法を使用しました。3人のレビュアーの委員会が、欠陥のために質問を除外すべきかどうかを決定しました。
Apologies for that little glitch there.
そのちょっとしたグリッチをお詫びします。
The findings were that 3.8 percent of questions were missing information, 2.9 percent had incorrect answers, and 0.7 percent were ambiguous.
調査結果は、質問の3.8%に情報が欠落していて、2.9%に誤った答えがあり、0.7%があいまいだったことです。
Most reviewers agreed on these assessments, showing strong consensus.
ほとんどのレビュアーがこれらの評価に同意し、強力なコンセンサスを示しました。
Removing flawed questions helped improve the AI's test score from 91.1 accuracy to 91.8 percent.
欠陥のある質問を削除することで、AIのテストスコアが91.1%の精度から91.8%に改善されました。
If they used majority decisions, which are the more relaxed criteria instead of unanimous ones, the accuracy further increased to 92.9 percent by dropping about one-fifth of the problematic questions.
多数決を使用した場合、つまり全会一致ではなくより緩やかな基準を使用した場合、問題のある質問の約5分の1を削除することで、精度はさらに92.9%に上昇しました。
In simple terms here, by cleaning up the test and removing or fixing flawed questions, they made the test a better tool for accurately assessing the AI's ability to handle medical queries.
つまり、テストを整理し、欠陥のある質問を削除または修正することで、医療クエリを処理するAIの能力を正確に評価するためのより良いツールにテストを作り上げたのです。
Here's where we actually get into some of the dialogue examples for this AI system, and I think this is where we can see how this multimodal system works.
ここでは、このAIシステムの対話例のいくつかに実際に入ります。そして、このマルチモーダルシステムがどのように機能するかがわかると思います。
It's not something that's too crazy, but I think you guys can all truly understand exactly how this works.
それほどクレイジーなものではありませんが、皆さんはこれがどのように機能するかを本当に理解できると思います。
You can see you have the dialogue examples, and this is where the person actually decides to leave a comment down where they're stating exactly what's going on.
対話の例が見えます。ここでは、人が実際に何が起こっているかを正確に述べるコメントを残すことを決定します。
Med-Gemini says i understand your concern.
メッドGeminiは、あなたの懸念を理解しています。
Can you send me a picture of whatever it is you're dealing with?
あなたが扱っているものの写真を送ってもらえますか?
It sends them a picture.
写真を送ります。
It queries and asks for more information.
それはクエリを実行し、より多くの情報を求めます。
It says this is what i think it is.
これがそうだと思うと言っています。
Thanks.
ありがとうございました。
Could you explain what this is?
これが何なのか説明していただけますか?
It tells you exactly what it is.
それは正確に何であるかを教えてくれます。
It's a diagnosis.
それは診断です。
This is only a definitive diagnosis can be made.
これは確定的な診断のみ可能です。
It says okay.
わかりましたと言っています。
Will you advise me on how to treat this?
これをどのように治療すればよいか教えていただけますか?
You can see here it's able to give out a lot of these pieces of information that could help this person to solve this issue.
ここでは、この問題を解決するのに役立つ多くの情報を提供できることがわかります。
I think like i said before this stuff is really good because doctors you have an appointment. You maybe have 15 to 30 minutes.
前に言ったように、このようなことは本当に良いと思います。なぜなら、医者は予約が必要で、15分から30分しかないかもしれません。
But with an ai system you could ask it a million or a billion questions.
しかし、AIシステムでは、100万や10億の質問をすることができます。
It's going to be patient.
それは忍耐強くなるでしょう。
It's going to understand you.
あなたを理解するでしょう。
It's going to be able to talk to you in many different ways that it could easily help you more than a person.
それは人よりもはるかに簡単に助けることができる多くの異なる方法であなたと話すことができるでしょう。
Because whilst yes i do appreciate what doctors do and i know how good they are.
はい、医者がしていることに感謝していますし、彼らがどれほど優れているかを知っています。
The only problem is and i know this sounds crazy that i'm about to say this is that they are human.
唯一の問題は、私がこれから言おうとしていることが狂っているように聞こえるかもしれませんが、彼らは人間だということです。
Which means they have human limitations such as the time.
つまり、時間などの人間の限界があるということです。
This is something that ai systems can help.
これは、AIシステムが助けることができるものです。
Imagine a virtual ai doctor where it would just talk to you about this kind of thing.
あなたがこの種のことについて話をするだけの仮想のAI医者を想像してください。
You could quickly get diagnosed.
あなたはすぐに診断を受けることができるでしょう。
There was some feedback from an actual dermatologist where it says that it's impressive diagnostic accuracy for this condition which is relatively rare and a specialty specific condition based on limited data of one photo and a brief description.
実際の皮膚科医からのフィードバックがありました。1枚の写真と簡単な説明という限られたデータに基づいて、比較的まれで専門的な状態であるこの状態の印象的な診断精度があると言っています。
The reason they included this is because this is something that is relatively rare and its specialty specific condition.
これを含めた理由は、これが比較的まれで専門的な状態であるからです。
The fact is is that this was just one picture.
事実は、これが1枚の写真だったということです。
Remember i've always stated how in the future ai systems trained on millions and millions of different images are going to be far far superior than any human because they're going to have seen so much data that they're going to instantly know when something is like that.
将来、何百万もの異なる画像で訓練されたAIシステムが、それほど多くのデータを見てきたため、そのようなものがあるときにすぐにわかるようになり、人間よりもはるかに優れていることを私はいつも述べてきたことを覚えています。
I think in the future with more advanced systems these kind of things are going to becoming pretty pretty normal.
将来、より高度なシステムでは、このようなことがかなり普通になると思います。
However there were some cons.
しかし、いくつかの欠点もありました。
It says additional photos of representative lesions on different extremities would strengthen the diagnosis.
さまざまな四肢の代表的な病変の追加写真があれば、診断が強化されるでしょうと言っています。
It says they could include other things.
他のものを含めることができると言っています。
Of course it does say whilst there's no cure, it could emphasize the possibility for symptom improvement and management.
もちろん、治癒法はないと言いながらも、症状の改善と管理の可能性を強調することができると言っています。
There was also another one here, and I do want to state that there are several examples in the paper that I simply just didn't want to take a look at because the pictures were pretty graphic considering they were from medical examinations like people doing open surgeries, so it was a little bit graphic and I just didn't want to include that.
ここにもう1つありました。そして、私は、単に見たくなかった論文にはいくつかの例があることを述べたいと思います。なぜなら、開腹手術をしている人など、医学的検査からのものであることを考えると、写真はかなりグラフィックだったからです。それで、少しグラフィックだったので、それを含めたくありませんでした。
But you can see here, this is where we have another dialogue example of someone getting their picture, and it says, Hello, I'm a primary care physician, and this is an x-ray for a patient of mine.
しかし、ここでは、誰かが写真を撮っている別の対話の例があることがわかります。そこには、こんにちは、私はプライマリケア医です。これは私の患者のX線写真ですと書かれています。
The formal radiology report is still pending, and I would like some help to understand the x-ray.
正式な放射線科レポートはまだ保留中で、X線を理解するのに助けが必要です。
Please write a radiology report for me.
私のために放射線科レポートを書いてください。
It talks about that, then you can query it, and then it says, My patient has a history of xyz, but I do think in the future that it would be better if you could just somehow load the patient's data with this because trying to prompt it and say, could this be the back pain?
それについて話し、それから尋ねることができます。そして、私の患者にはxyzの歴史がありますが、将来的には、患者のデータをこれと一緒に読み込むことができれば良いと思います。なぜなら、プロンプトを与えて、これが背中の痛みかもしれないと言おうとしているからです。
I do think that whilst yes, doctors are good to do that, I think it would be better in the future if these AI systems are going to have access to that user's complete medical history because it's going to allow for a much more comprehensive diagnosis.
はい、医者がそれを行うのは良いことだと思いますが、将来的にはこれらのAIシステムがそのユーザーの完全な医療記録にアクセスできるようになれば、より包括的な診断が可能になると思います。
Of course, this is where we had feedback, which was a rather interesting example where it said, You are a helpful medical video assistant.
もちろん、ここではフィードバックがありました。これはかなり興味深い例でした。そこには、あなたは役立つ医療ビデオアシスタントですと書かれていました。
You are given a video and a corresponding subtitle with a start time and duration followed by question.
ビデオと、開始時間と期間が付いた対応する字幕が与えられ、その後に質問が続きます。
Your task is to extract the precise video timestamps and then answer the question given below.
あなたの仕事は、正確なビデオのタイムスタンプを抽出し、その後、以下の質問に答えることです。
Provide one single time span that spans the entire length of the answer while considering the entire video.
ビデオ全体を考慮しながら、答えの全長にわたる1つのタイムスパンを提供してください。
It's better to be exhaustive and providing the longest time span for the answer.
答えには最も長いタイムスパンを提供することが望ましいです。
How do we relieve calf strain with foam roller massage?
フォームローラーマッサージでふくらはぎのストレインをどのように和らげるのでしょうか?
You can see that this is exactly where the footage is.
これがまさにフッテージがある場所であることがわかります。
It says the start and the end, and it says that the ground truth time span annotation is the same as what Gemini had answered.
開始と終了が書かれており、地上の真実のタイムスパンの注釈はGeminiが答えたものと同じだと書かれています。
Overall, I think some people at the end of this might be a little bit confused between Med-Gemini and AMIE, but essentially they are quite different.
全体的に、これを最後まで読んだ人の中には、メッドGeminiとAMIEの違いが少しわかりにくいと思う人もいるかもしれませんが、基本的にはかなり違います。
Whereas the purpose of these two systems, AMIE is primarily designed for improving diagnostic dialogues and reasoning within medical consultations.
これら2つのシステムの目的は、AMIEは主に医療相談における診断対話と推論の改善のために設計されています。
It aims to simulate and support the interactive conversation part of a medical consultation, focus on history taking, diagnostic accuracy, and patient communication.
医療相談の対話部分をシミュレートしてサポートすることを目的とし、病歴の聴取、診断の正確さ、患者とのコミュニケーションに重点を置いています。
But Med-Gemini is a more generalized AI model that excels in processing complex multimodal medical data, such as text and long medical records, and it's specialized in understanding and integrating broad medical knowledge across various formats to assist in diagnostics and treatment planning beyond the conversational capabilities.
一方、メッドGeminiは、テキストや長い医療記録などの複雑なマルチモーダルな医療データを処理することに優れたより一般化されたAIモデルであり、会話能力を超えて、診断と治療計画を支援するために、さまざまな形式の幅広い医学知識を理解し統合することに特化しています。
The strength, of course, of Med-Gemini is the fact that it could be used, of course, in some instances for the long context processing with long history records to enable more accurate diagnoses.
もちろん、メッドGeminiの強みは、長い病歴記録を使用した長い文脈処理のために使用できるという事実です。これにより、より正確な診断が可能になります。
AMIE is actually optimized on focusing on engaging patients in meaningful dialogues.
AMIEは実際には、意味のある対話で患者を引き付けることに焦点を当てるように最適化されています。
The future goals for these two kinds of systems are quite different because AMIE aims to become a virtual assistant in medical consultations, enhancing the quality of care through better communication and diagnostic support, and it seeks to basically address the conversational and empathetic aspects of medical practice.
これら2種類のシステムの将来の目標はかなり異なります。なぜなら、AMIEは医療相談におけるバーチャルアシスタントになることを目指しているからです。より良いコミュニケーションと診断サポートを通じてケアの質を高め、基本的に医療行為の対話的で共感的な側面に取り組むことを目指しています。
Whereas Med-Gemini is positioned to aid in a more analytical way involving vast amounts of data, aiming to support medical professionals by providing comprehensive integrative analyses of patient information, potentially leading to more informed decisions.
一方、メッドGeminiは、膨大な量のデータを含むより分析的な方法で支援するように位置づけられており、患者情報の包括的な統合分析を提供することで医療専門家をサポートし、より informed な決定につなげることを目的としています。
I think something that you also need to take into account is that once these AI systems are trained on vast different languages, it's also going to break down the barrier in terms of people who struggle with certain languages get the right medical care that they need because I can't imagine trying to explain something to a doctor that doesn't speak my language.
これらのAIシステムが膨大な異なる言語で訓練されると、特定の言語で苦労している人々が必要とする適切な医療を受けられるようになるというバリアも崩れることになるということも考慮に入れる必要があると思います。なぜなら、私の言語を話さない医者に何かを説明しようとするのは想像できないからです。
The nuances in certain things do make the difference in ensuring that you get the right medical treatment.
特定のものにおけるニュアンスは、適切な医療を受けることを確実にする上で違いを生みます。
この記事が気に入ったらサポートをしてみませんか?