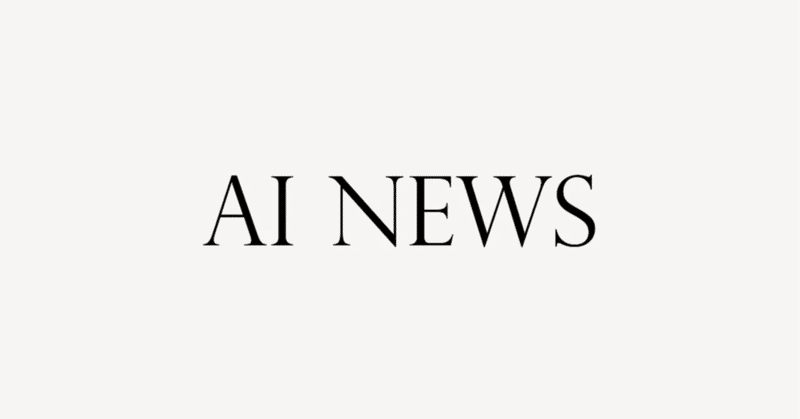
【中国のAI企業SenseTime、GPT-4を上回る性能のSenseNova 5.0を発表】英語解説を日本語で読む【2024年4月26日|@TheAIGRID】
中国のAI企業であるSenseTimeが、SenseNova 5.0という新しいAIモデルを発表しました。このモデルは、ほぼすべてのベンチマークにおいてOpenAIのGPT-4 Turboを上回る性能を示しています。一方、AnthropicのClaude 3 Opusは、一部のベンチマークではSenseNova 5.0を上回っていますが、その差は僅かです。SenseNova 5.0は、画像生成においても優れた性能を示しており、ニュアンスのある写実的な肖像画を生成することができます。また、SenseTimeは18億パラメータの小型モデルであるSenseChat Lightを発表し、同規模の他社モデルを大幅に上回る性能を実現しました。
公開日:2024年4月26日
※動画を再生してから読むのがオススメです。
Around two days ago, there was a rather interesting development.
およそ2日前、かなり興味深い展開がありました。
There was something in China that I think you should all be aware of because it changes the dynamic of the AI race, which leads me to believe now that currently China is hot on the heels of the rest of the world in terms of AI developments.
中国には皆さんが認識しておくべきことがあります。それはAI競争のダイナミクスを変えるものであり、現在、中国がAIの開発において世界の他の国々に追いつこうとしていると私は考えています。
It seems that the US has been leading the charge in terms of state-of-the-art models, but recently this is the most interesting thing we've seen in recent times.
最近では、アメリカが最先端のモデルをリードしてきたようですが、最近はこれが最も興味深いものです。
Let's take a look at exactly what China has been doing and what this announcement is.
中国がこれまでに何をしてきたのか、そしてこの発表が何なのかを見てみましょう。
Essentially what we have here is we have a situation where China has potentially taken the lead with their new model.
基本的に、ここでの状況は、中国が新しいモデルでリードを取った可能性があるということです。
Two days ago, SenseTime launched SenseNova 5.0, which according to this report actually beats GPT-4 on nearly all benchmarks, and there are actually a variety of different things that are truly surprising about this.
2日前、SenseTimeがSenseNova 5.0を発表しました。この報告によると、ほとんどすべてのベンチマークでGPT-4を上回っているとのことで、実際に驚くべき異なる点がいくつかあります。
It isn't just some hyped up GPT-4 thing.
これはただのGPT-4の宣伝ではありません。
If the claims are true, and we're going to dive into them, this means that things are truly, truly ramping up, and it seems that across the board from different nations and different countries, we're definitely going to be seeing increased competition in terms of what AI systems are capable of.
主張が真実であれば、そしてそれについて詳しく調べていくと、事態は本当に本当に加速していることを意味し、異なる国々や地域から、AIシステムがどれだけのことができるかに関して、競争がますます激しくなることが見込まれます。
We can see here that they've essentially compared this to GPT-4 Turbo, but there are a lot of things that this presentation dives into, and bear in mind that the majority of this is in Chinese, but I've done my best to translate most of this so that you can completely understand exactly what's going on.
ここでは、彼らがこれをGPT-4 Turboと比較していることがわかりますが、このプレゼンテーションが掘り下げていることがたくさんあります。そして、これの大部分は中国語であり、私ができる限り翻訳しましたので、何が起こっているのかを完全に理解できるようにしています。
You can see here that their SenseNova 5.0 actually looks pretty decent.
ここで見ると、彼らのSenseNova 5.0は実際にかなりまともに見えます。
On the left, you can see it states that it is hybrid used, in the middle, they state that it is trained on over 10 billion tokens.
左側には、ハイブリッド使用であることが記載されており、中央には1,000億以上のトークンでトレーニングされていると述べられています。
The inference actually supports up to 200,000 tokens, which is rather interesting because we've seen a longer and longer context windows, so a 200k context window is something that is I wouldn't say surprising, but it does show that of course things are going well, and of course the main main statement from this is that performance exceeds GPT-4 Turbo.
推論は実際に最大20万トークンまでサポートしており、これは興味深いことです。長いコンテキストウィンドウが見られる中、20万のコンテキストウィンドウは驚くべきことではないと言えますが、もちろん事が順調であることを示しており、もちろんこの主な主張は、性能がGPT-4 Turboを上回っているということです。
The reason, as you all know, why GPT-4 Turbo is highly touted as the state-of-the-art model at that time, and that's why people constantly, constantly, constantly try to one-up that in terms of the benchmarks.
皆さんご存知の通り、なぜGPT-4 Turboが当時の最先端モデルとして高く評価されているか、そしてそれがベンチマークの観点で常に常に常に上回ろうとする理由です。
This was the start of the presentation, but things do get a lot more interesting, and I'm going to show you all exactly what that is.
これがプレゼンテーションの始まりでしたが、事はさらに興味深くなり、皆さんにそれが具体的に何であるかを示します。
There was one screenshot here where I'm not entirely sure if they actually had these AI systems hooked up to a game, but the text states that a live demonstration was conducted on the comparison of multiple functions of their model and GPT-4, including creative writing, logical reasoning, diagrams, image understanding, and calculations of food calories based on pictures, and this is what the text actually states here, but we will get into the benchmarks, but I just wanted to include this for full transparency.
ここに1つのスクリーンショットがあり、AIシステムが実際にゲームに接続されているかどうかは完全にはわかりませんが、テキストには、モデルとGPT-4の複数の機能の比較がライブデモンストレーションで行われ、創造的な執筆、論理的推論、図表、画像理解、写真に基づく食品カロリーの計算などが含まれていると記載されています。これがここに実際に記載されているテキストですが、ベンチマークに入りますが、完全な透明性のためにこれを含めたかっただけです。
In order to show off the muscles of the larger models, Sense Time also played the King of Fighters, and at first, the green player GPT-4 had the slight upper hand, but was quickly overwhelmed by the red player Sense Chat's light various combos.
より大きなモデルの力を見せるために、Sense Timeはキング・オブ・ファイターズをプレイしました。最初は、緑のプレイヤーであるGPT-4がわずかに優位でしたが、すぐに赤いプレイヤーであるSense Chatの様々なコンボに圧倒されました。
I'm not sure if they're actually talking about this as if it's some kind of metaphor for their system beating GPT-4, or if they actually hooked it up to a Street Fighter style game and Sense Chat Lite was able to best GPT-4.
彼らが実際にこれをシステムがGPT-4を打ち負かすというメタファーとして話しているのか、それともStreet Fighterスタイルのゲームに接続してSense Chat LiteがGPT-4を上回ったのか、私はよくわかりません。
If you are someone who can translate this more effectively because I did honestly put this through multiple different AI systems, and the result was frequently the same.
もしこれをより効果的に翻訳できる方がいれば、正直に言って、私は複数の異なるAIシステムを通した結果が頻繁に同じだったので。
Here's where things start to get interesting, and here's where things are really, really fascinating because these are obviously the benchmarks for this system.
ここからが興味深くなります。そして、ここが本当に本当に魅力的な部分です。なぜなら、これらは明らかにこのシステムの基準です。
You can see Sense Chat version 5 is over here on the right-hand side, this is Sense Chat version 5.
右側にSense Chatバージョン5があります、これがSense Chatバージョン5です。
And then of course what they actually do do, and this pay attention here because I'm going to say some things that I'm going to recall them so you can see right here that they actually do compare this to GPT-4 Turbo, and that actually is to the eagle-eyed viewers that it is the 1106.
そしてもちろん、実際に行っていることについては、ここに注意してください、なぜなら私がいくつか言うことがあるので、それらを思い出すことができます。実際には、これをGPT-4 Turboと比較していることがわかります。それは実際には、鷲の目の視聴者にとっては1106です。
This isn't actually the most recent one, but it's not also a most outdated one as well, and we can see that the only benchmarks that it surpasses their model Sense Chat version 5 on is, of course, the math zero shock benchmark right here, and we can see that it's highlighted in the 61% area, and we can see that in this benchmark right here as well.
これは実際には最新のものではありませんが、最も古いものでもありません。そして、私たちのモデルSense Chatバージョン5を上回る唯一のベンチマークは、もちろん、ここにある数学のゼロショックベンチマークです。そして、このベンチマークでも、61%の領域にハイライトされていることがわかります。
We can basically see that currently what we're looking at is a situation where across all of the benchmarks compared to GPT-4 Turbo, one of the most recent models.
基本的には、現在私たちが見ているのは、GPT-4 Turboと比較して、最新のモデルの1つであるベンチマーク全体にわたる状況です。
I don't know why they didn't do the most recent one, I'm guessing just based on where they are in their testing, and of course we do have a comparison with LLaMA 3 70 billion parameters instruct.
なぜ彼らが最新のものをしなかったのかはわかりませんが、おそらく彼らのテストの進行状況に基づいていると思われます。そしてもちろん、LLaMA 3 70億パラメータの指示との比較があります。
We can see that the differences from GPT-4 are honestly, I wouldn't say quite stark, but it doesn't just seem to be a simple percentage gain.
GPT-4との違いは、正直言って、かなり目立つとは言えませんが、単なるパーセンテージの増加ではないようです。
Like, for example, on the MMLU, we can see that this one is rather small, but on the CMMU, I'm guessing that this is a different version of the MMLU, perhaps the Chinese MMLU.
たとえば、MMLUでは、これはかなり小さいですが、CMMUでは、これはMMLUの異なるバージョンであると思われます、おそらく中国のMMLUです。
Honestly, I'm not entirely sure.
正直言って、私は完全にはわかりません。
We can see that it increases, but you can see, like I said, on the different benchmarks here, the gains don't seem to be completely incremental, because if we actually look at the differences, this one is at 80, and this one is at 93.61.
増加していることがわかりますが、私が言ったように、異なるベンチマークでは、得られる利益が完全に段階的ではないようです。実際に違いを見ると、これは80で、これは93.61です。
I think what we're looking at here, and of course, some of these benchmarks aren't all on GPT-4.
ここで見ているのは、そしてもちろん、これらのベンチマークのいくつかはすべてGPT-4上にはありません。
As in, when I was actually checking some of the other models, I couldn't find things like the race, I couldn't find things like the natural questions on other models, and that's like Gemini Pro and Claude.
他のモデルを実際にチェックしていたとき、他のモデルではレースのようなものや自然な質問のようなものが見つからなかったのですが、Gemini ProやClaudeのようなものです。
And I do actually test them against it, and I'll get to that later on in the video.
実際にそれらと比較テストを行っており、そのことについて後でビデオで説明します。
But essentially, I think this is rather fascinating that China has, I would say, surpassed GPT-4 Turbo in their model.
基本的に、中国がGPT-4 Turboを超えたと思うのはかなり興味深いと思います。
And what I would like to see from this model, honestly, is if people could potentially test this model, because it would be interesting to see how it actually compares in terms of users.
このモデルから見たいのは、正直言って、人々がこのモデルをテストできる可能性があるかどうかです。ユーザーの観点で実際にどのように比較されるかを見るのは興味深いと思います。
And what I mean by that is, I mean that whilst, yes, currently on this chart, what you're currently seeing is GPT-4 only winning in two out of the several different categories here.
そして、これは、このチャート上で、現在見ているのは、GPT-4がいくつかの異なるカテゴリーで2つしか勝っていないということです。
And also, even LLaMA 3, we do know that there is a different benchmark, which is rather, rather important.
LLaMA 3でも、かなり重要な異なる基準があることはわかっています。
And the benchmark that I find to be one of the most interesting, because it's not just based on the instance where things could be fine-tuned on the actual benchmarks in terms of just trying to get something that beats things on benchmarks, but isn't actually useful on a day-to-day basis.
そして、私が最も興味深いと考える基準は、ベンチマークを実際に微調整して、単にベンチマークで勝つものを得ようとするだけでなく、実際に日常的に役立つものを得ようとするインスタンスに基づいているということです。
You can see here that we do have GPT-4 1106 on the chatbot arena, as I've stated previously.
ここでは、以前に述べたように、チャットボットアリーナにGPT-4 1106があることがわかります。
The chatbot arena is different because it ranks models based on their arena elo, which is determined by votes against other systems.
お話し相手のエリアは異なりますが、それは他のシステムに対する投票によって決定されるエリアエロに基づいてモデルをランク付けしています。
And basically, all this means is that people test AI systems side by side, and their elo increases when people vote for that system being the most useful.
基本的に、これは人々がAIシステムを並べてテストし、そのシステムが最も有用とされると人々が投票すると、そのエロは増加します。
And it's a blind test, so you don't know which system is which.
そして、これはブラインドテストなので、どのシステムがどれかはわかりません。
And then over time, you get to see which system is actually useful on a day-to-day basis, answering a variety of different questions without the bias of looking at other systems.
そして、時間の経過とともに、他のシステムを見るバイアスなしで、さまざまな質問に答える日常的に実際に有用なシステムを見ることができます。
It's something that I think is very useful, and we can see that GPT-4 1106 is basically at rank number one.
私は非常に有用だと思うものであり、GPT-4 1106が実質的に第1位にランクされていることがわかります。
And of course, like I said, the new one does have a slightly higher elo rating, but I still think that this is rather rather impressive for what we're seeing here.
そしてもちろん、私が言ったように、新しいものは若干高いエロレーティングを持っていますが、それでも私はこれが私たちがここで見ているものにとってかなり印象的だと思います。
Something that I also did want to test was I wanted to test this against other models because one thing I realized when looking at this was I was like, okay, whilst this is good, there are other state of the art systems out there.
私が試したかったもう一つのことは、他のモデルと比較してみたかったということでした。これを見て気づいたことの一つは、これは良いとしても、他にも最先端のシステムがあるということでした。
Because yes, GPT-4 Turbo 1106 is state of the art, but GPT-4 Turbo isn't the only one.
そうです、GPT-4 Turbo 1106は最先端ですが、GPT-4 Turboだけではありません。
And GPT-3 Turbo and LLaMA 3 70 billion parameters aren't the only ones there.
GPT-3 TurboやLLaMA 3 70億パラメータもそこにあるだけではありません。
The other one that most people might be thinking about, and maybe you're not thinking about this, but I certainly am, is I'm thinking about the Claude 3.
ほとんどの人が考えているかもしれない他の1つ、あるいはあなたが考えていないかもしれませんが、私は確かに考えているのは、Claude 3のことです。
Claude 3, if you don't know, it's a model, a state of the art model, and it was released by Anthropic.
Claude 3、わからない場合は、それはモデルであり、最先端のモデルであり、アントロピックによってリリースされました。
I've done this a little bit sloppily, but let me just explain to you what you're currently looking at.
私は少し雑にやってしまいましたが、現在見ているものを説明させてください。
All you're looking at is Claude 3's benchmarks, and we can see that it is compared to GPT-4 in this tab right here.
見ているのはClaude 3のベンチマークだけで、このタブでGPT-4と比較されていることがわかります。
And essentially, across the board, completely, Claude 3's Opus model does surpass the benchmarks of GPT-4.
基本的に、全体的に、Claude 3のOpusモデルはGPT-4のベンチマークを上回っています。
What this does mean here, what I've actually done here is I've actually circled these areas where Claude 3 retains its leadership in terms of how far it is, but I've added x's here because these are areas where sense chat v5 actually beats Claude Opus.
ここで意味するのは、実際には、Claude 3がどれだけ優れているかについて、これらの領域を囲んでいますが、ここにxを追加しました。これは、sense chat v5が実際にClaudeOpusを上回る領域です。
In the math benchmarks, which is math problem solving, it does better than Claude Opus.
数学のベンチマークでは、数学問題の解決において、ClaudeOpusよりも優れています。
There we can see it's 61.9, and this one is 60.1.
ここでは61.9、こちらは60.1です。
That's where sense chat v5 reigns supreme.
それがsense chat v5が最高であるところです。
Then, of course, we can see here on the common sense knowledge, the heliswag, this is 95.4 percent, and then for here on the common sense heliswag, we can see that theirs is actually at 97 percent.
そして、もちろん、ここで一般常識の知識、heliswag、これは95.4%で、ここで一般常識のheliswag、彼らの方は実際に97%です。
This is actually a huge improvement.
これは実際には大きな改善です。
We can see that 95.4 percent to 97.52 percent is a big improvement.
我々は、95.4%から97.52%が大幅に改善されたことが分かります。
However, Claude actually retains the leadership benchmarks in these areas where we can see the MMLU, the graduate level reasoning, the grade school math.
ただし、Claudeは実際には、MMLU、大学院レベルの推論、小学校の数学など、これらの分野でリーダーシップの基準を維持しています。
But something that I do want to add, whilst yes, Claude 3 does have the best on these benchmarks, I would have to be honest and state that it isn't far off.
ただし、はい、Claude 3はこれらの基準で最も優れていると言わざるを得ませんが、正直に述べると、それほど大差はありません。
Like, for example, if we look at the code on the human evaluation at 84.9 percent, or for example, if we look at the big bench hard, we can see it's currently at 82.98 percent, whereas on the big bench hard, it's at 86.8 percent.
例えば、人間の評価コードが84.9%であることを見ると、また、例えば、ビッグベンチハードを見ると、現在82.98%であり、一方、ビッグベンチハードでは86.8%です。
I mean, it is pretty interesting to see how these differences are, but the long story short is that basically, Gentschat v5 surpasses GPT-4 Turbo, Claude 3 Opus surpasses GPT-4 Turbo, but in terms of the actual benchmarks, the ones that you do want to care about according to the arena, GPT-4 Turbo's most recent version retains its leadership spot, even if the benchmarks that you know are benchmarks by like math and coding and stuff don't appear to be correct.
これらの違いを見るのはかなり興味深いと思いますが、要するに、Gentschat v5はGPT-4 Turboを凌駕し、Claude 3 OpusはGPT-4 Turboを凌駕しますが、実際のベンチマーク、つまり競技場によると重要なベンチマークは、GPT-4 Turboの最新バージョンがリーダーシップの地位を維持していることです。数学やコーディングなどのベンチマークは正しくないようですが、気にする必要があるベンチマークです。
People seem to be having a great time with GPT-4 Turbo, but firmly followed by Claude 3 Opus.
人々はGPT-4 Turboで楽しんでいるようですが、しっかりとClaude 3 Opusが続いています。
And then Google's Gemini 1.5 Pro in the API.
そして、GoogleのGemini1.5 ProがAPIで登場します。
I think this is still rather impressive because it seems that this company has been working very quietly and very diligently in stealth.
私は、これはまだかなり印象的だと思います。なぜなら、この会社は非常に静かに、そして非常に熱心にステルスで働いてきたようです。
Because this is something that did catch people off guard.
なぜなら、これは人々を驚かせたことだからです。
There are some other things that are actually really surprising, and one of the most surprising things about this was something in regards to the smaller models.
実際には、他にも驚くべきことがいくつかあり、その中で最も驚くべきことの1つは、小さなモデルに関するものでした。
Because their smaller model is insane, and it's so crazy to the point where I'm wondering to see if some of these things are actually even legit.
彼らの小さなモデルは狂っているので、実際にこれらのことのいくつかが本当に正当なものであるかどうか疑問に思っています。
One of the things they actually talk about is a writing task, and here's where they compare GPT-4.
彼らが実際に話していることの1つは、ライティングの課題であり、ここで彼らはGPT-4を比較しています。
You can see right here, GPT-4 to GensChat v5.
ここで、GPT-4をGensChat v5と比較できます。
And right here, this is where, and funnily enough, this actually does look like the chatbot arena.
そしてここで、これが、面白いことに、実際にチャットボットの競技場のように見えるところです。
I'm wondering if in a couple of days, we are actually going to get GensChat v5 in the arena.
数日後に、実際にGensChat v5を競技場で見ることができるかどうか疑問に思っています。
But, they talk about how essentially the summary of this what actually happened here was that they basically had a task to write a college entrance exam essay on innovation based on the classic Chinese novel, Dream of the Red Chamber.
しかし、彼らは実際にここで起こったことの要約として、基本的には、彼らは古典的な中国小説「紅楼夢」に基づいた革新に関する大学入学試験のエッセイを書くという課題を持っていたと話しています。
And the author notes that GPT-4's writing style tends to be more rigid and structured, using repetitive phrases.
そして著者は、GPT-4の文章スタイルがより硬直して構造化され、繰り返しのフレーズを使用する傾向があると指摘しています。
And in contrast, SenseNova's 5.0 exhibits more free-flowing and divergent writing style, drawing upon a wide range of Chinese cultural and literary references from ancient times to the modern internet age.
対照的に、SenseNovaの5.0は、より自由な流れるような書き方を示し、古代から現代のインターネット時代までの幅広い中国の文化的および文学的な参照を活用しています。
Long story short, they're basically trying to say that if we look at the writing tasks in the examples that they've included, GPT-4 loses.
要するに、彼らは基本的に、彼らが含めた例の中のライティング課題を見ると、GPT-4が負けると言おうとしています。
There was also another one right here where they put GPT-4's model against SenseChat v5 in, I guess you could say, a logic/reasoning task.
ここにもう1つありましたが、GPT-4のモデルをSenseChat v5と比較して、論理/推論のタスクを行ったところがありました。
And they were trying to compare this in terms of what this model would do when it was trying to Figure out the amount of coffee and water consumed.
そして、このモデルがコーヒーと水の消費量を計算しようとするときに何をするかを比較しようとしていました。
And honestly, it's pretty hard to decipher what's going on here because, like I said before, it's a translation.
正直なところ、ここで何が起こっているのかを解読するのはかなり難しいです。前にも言ったように、これは翻訳です。
The interpretation might actually not be correct.
解釈は実際には正しくないかもしれません。
And I'm not going to get into the details of that.
その詳細には立ち入らないつもりです。
But the long story short here was that GPT-4 wasn't able to realize exactly what was going on.
しかし、要するに、GPT-4は実際に何が起こっているかを正確に理解できませんでした。
But SenseChat v5, the reasoning steps provided the correct answer.
しかし、SenseChat v5は、推論ステップが正しい答えを提供しました。
I will leave the prompt and the explanation in the description.
プロンプトと説明は説明に記載しておきます。
But it's just very confusing trying to get a logical reasoning task correct.
しかし、論理的な推論タスクを正しく行うのは非常に混乱することです。
Because if you've ever seen the examples of these incredibly confusing tasks, one word can truly change the entire outcome of the answer.
なぜなら、これらの非常に混乱するタスクの例を見たことがあれば、1つの単語が答えの全体的な結果を本当に変えることができるからです。
And it's important to get the translations 100% because even if you get them 99, you can completely fail the question.
翻訳を100%正確にすることが重要です。99%だと、問題を完全に間違える可能性があります。
This is probably the most fascinating part of the thing when I was looking through it in terms of the benchmarks.
これは、ベンチマークの観点から見て、おそらくそのものの中で最も魅力的な部分です。
And in fact, that's actually not true.
実際、それは実際には真実ではありません。
It's actually coming up soon.
実際、それはもうすぐやってきます。
But right here, and we will get to the most interesting part here.
しかし、ここで、そして私たちはここで最も興味深い部分に取り組みます。
But right here, this is where we take a look at their visual recognition systems.
しかし、ここで、これは彼らの視覚認識システムを見ていく場所です。
And of course, you can see it is compared to Google's Gemini provision, OpenAI's GPT-4 Vision, Quen's VR Max, which is a vision system, and of course, the intern LLM X Composer 2 VL and Step 1 Vision.
もちろん、GoogleのGeminiプロビジョン、OpenAIのGPT-4 Vision、QuenのVR Max(ビジョンシステム)、そしてもちろん、インターン大規模言語モデル X Composer 2 VLとStep 1 Visionと比較されています。
There are all of these different ones compared on these benchmarks.
これらすべての異なるものがこれらのベンチマークで比較されています。
And we can see from what we've seen here, that the top one does surpass them.
ここで見てきた内容から、トップのものがそれらを上回っていることがわかります。
One thing that I also saw that was really, really cool was that they also did have image generation.
その中で私が見たもう一つのすごいことは、画像生成も行っていたことです。
And by the looks of things, it seems to be very, very photorealistic.
そして見たところ、非常に写実的なようです。
Essentially, the text states that Sense 5.0 sets a new benchmark in terms of AI-powered image generation, demonstrated by its impressive performance in generating nuanced and lifelike portraits.
基本的に、テキストによると、Sense 5.0はAIによる画像生成の新たな基準を設定し、微妙で写実的な肖像画を生成するという印象的なパフォーマンスを示しています。
The following example illustrates the AI's capability to create text-image visuals.
次の例は、AIのテキスト画像ビジュアルの能力を示しています。
And essentially, using the prompt, a vibrant Asian female portrait with beauty makeup, casual hair, a smile, natural, with movie-grade quality, presenting different expressions and styles.
基本的に、プロンプトを使用して、美しいメイクをしたアジア系女性の肖像画が、自然な笑顔、自然な髪型、映画の品質で、異なる表情やスタイルを持つ、生き生きとしたものが作成されます。
Sense Nova 5.0 showcases its sophisticated interpretation of textual descriptions and its ability to generate diverse facial expressions and styles that carry the subtlety and richness of film-grade portraits.
Sense Nova 5.0は、テキストの記述の洗練された解釈と、微妙で豊かな映画品質の肖像画を持つ多様な表情やスタイルを生成する能力を披露しています。
That description is pretty good, but I've got to be honest, if this is their text-to-image generation model, I have to say that, while yes, Midjourney might be on this level, this is definitely something that looks really, really good.
その説明はかなり良いですが、率直に言わせてもらうと、これが彼らのテキストから画像を生成するモデルなら、Midjourneyがこのレベルにあるかもしれませんが、これは本当にとても良いものに見えます。
I mean, we can't say that this doesn't look completely realistic.
これが完全にリアルではないとは言えないという意味です。
And as for the prompt, I think this is something that does do it really, really well.
そしてプロンプトに関しては、これは本当にうまくやっていると思います。
Of course, I'm guessing that they did compare this to other systems.
もちろん、これを他のシステムと比較したのだろうと思います。
Right here, in fact, it isn't entirely clear, but I'm guessing that these are other systems.
ここでは実際、完全にははっきりしていませんが、おそらくこれらは他のシステムだと思います。
But either way, this one right here does look really, really realistic.
とにかく、この中の1つは非常にリアルに見えます。
And I have to say that this is pretty impressive.
そして、これはかなり印象的だと言わざるを得ません。
Here's where we talk about the most interesting bit, because like I said before, we were previously supposed to, but I was thought that the slide was the other slide.
ここが最も興味深い部分について話す場所です。前にも言ったように、以前はそうするはずだったのですが、スライドが別のスライドだと思われていました。
Essentially, this is where they talk about their smaller models, their more compact models that they can use for many different things.
基本的に、これは彼らが話す小さなモデル、さまざまなことに使用できるよりコンパクトなモデルについて話す場所です。
You can see that the one they've highlighted is SenseChat Light, which is 1.8 billion parameters in size.
彼らが強調しているのは、サイズが1.8億パラメータであるSenseChat Lightです。
And it does do a lot better than others at the similar size.
そして、同じサイズの他のものよりもはるかに優れています。
We can see compared to the GEMMA 2 billion parameters by Google, it completely destroys it.
GoogleのGEMMA 20億パラメータと比較すると、完全に破壊しています。
We can see that LLaMA 2, 13 billion parameters, it completely does better than it.
LLaMA 2130億パラメータと比較すると、完全に優れています。
But one thing that I didn't really understand from this and was pretty confusing was, of course, the benchmarks, because they didn't actually do this on traditional benchmarks.
しかし、これから本当に理解できなかったことで、かなり混乱したのは、ベンチマークです。なぜなら、彼らは実際には従来のベンチマークでこれを行っていなかったからです。
They did this on a different kind of benchmark.
おそらく、彼らは異なる種類のベンチマークでこれを行った。
When this text on this left column was actually translated, the benchmarks that we got were, I guess you could say, the words we got were comprehensive score, language comprehension, creativity, reasoning, and the average overall.
この左列のテキストが実際に翻訳されたとき、私たちが得たベンチマークは、言うなれば、私たちが得た言葉は総合スコア、言語理解、創造性、推理、そして全体の平均でした。
One thing that I do think is quite surprising here is that 1.8 billion parameter model does showcase incredible capabilities because time and time again, we've seen that trend to be increased.
ここでかなり驚くべきことの1つは、18億のパラメーターモデルが信じられないほどの能力を示していることです。何度も何度も、その傾向が増加しているのを見てきました。
One critique that I do have here is that they didn't compare this to Microsoft's Phi 3.
ここで私が持つ1つの批判は、彼らがこれをMicrosoftのPhi 3と比較していないことです。
And I'm guessing that Phi 3 was literally just released, so I guess that's okay.
そして私はPhi 3が文字通りリリースされたばかりだと推測していますので、それは大丈夫だと思います。
But they didn't actually compare this to LLaMA 3.
しかし、彼らは実際にこれをLLaMA 3と比較していませんでした。
You can see here that they compared this to LLaMA 2, 7 billion parameters.
ここで、彼らはこれをLLaMA 2、70億のパラメーターと比較していることがわかります。
And of course, they state that it beats it and it beats Google's and the other ones like Quen's 1.5.
もちろん、彼らはそれを打ち負かし、GoogleやQuen's 1.5などの他のものを打ち負かすと述べています。
But even what they actually did was, if we actually look at the original parameters, we can see they actually did compare LLaMA 3, 70 billion instruct.
しかし、実際に彼らが行ったことは、元のパラメーターを見ると、彼らは実際にLLaMA 3、70億の命令を比較していることがわかります。
I'm guessing, okay, and this is just a guess, maybe it isn't true that if the original system beats LLaMA 3, I'm guessing that this smaller system doesn't beat LLaMA 3 because they haven't included it for whatever reasons.
私は推測していますが、もしかしたら元のシステムがLLaMA 3を打ち負かすのであれば、この小さなシステムがLLaMA 3を打ち負かすわけではないと思います。何らかの理由でそれを含めていないからだと思います。
And I'm pretty sure they could have.
おそらく彼らはできたでしょう。
I think this is still pretty interesting because they've got a smaller model that is levels above these other smaller models.
これはまだ非常に興味深いと思います。なぜなら、彼らは他の小さなモデルよりもレベルが上の小さなモデルを持っているからです。
But the point here is that based on some other things that I've seen as well they actually do talk about image generation.
しかし、ここでのポイントは、私が他のいくつかのことに基づいて見たことによると、実際に画像生成について話しているということです。
And I've got to be honest, the text here is a little bit confusing.
率直に言って、ここのテキストは少し混乱しています。
I have tried to understand the context of what it's trying to explain, but it does get a little bit confusing sometimes.
私はそれが何を説明しようとしている文脈を理解しようと努力しましたが、時々少し混乱します。
And I will leave a link to the article.
そして、その記事へのリンクを残します。
One thing that I actually do talk about that they say that this is going to be a calorie assistant and you can use it to submit images and completely understand your calories.
実際に話していることの1つは、これがカロリーアシスタントになると言っており、画像を提出して完全にカロリーを理解することができるということです。
And I think right now the AI space is heating up quite a lot.
そして、今のところAIの分野はかなり盛り上がっています。
And this is not going to be the only company coming out of China to present their models that do take us by surprise.
そして、これは私たちを驚かせるモデルを持つ中国から出てくる唯一の企業ではないでしょう。
But so far, this seems like a very interesting update.
しかし、今のところ、これは非常に興味深いアップデートのように思えます。
What's also interesting is that the company shares soared more than 30% after announcing its native generative AI model.
その会社のネイティブ生成AIモデルを発表した後、株価が30%以上急騰したことも興味深いです。
This was something that made the company stock price jump 30%. So maybe it could be argued that the benchmarks that they're talking about aren't as good.
これは、会社の株価が30%上昇した要因であり、彼らが話している基準がそれほど良くない可能性があるかもしれません。
But the only way for us to know is, of course, for us to test it in the arena, for other people to do their independent evaluations.
しかし、私たちがそれを知る唯一の方法は、もちろん、アリーナでテストすること、他の人が独自の評価を行うことです。
And of course, things are going to be a little bit different because I'm guessing that this is fine-tuned on the Chinese language.
そしてもちろん、これは中国語に微調整されていると推測しているので、少し異なることになるでしょう。
The translations might not be as completely accurate.
翻訳は完全に正確でないかもしれません。
I'm guessing maybe if they make an English model, that might be as good or better.
もしかしたら、英語モデルを作成すれば、それが同等以上になるかもしれません。
But of course, this is going to be something that we would have to see.
しかしもちろん、これは見なければならないことになるでしょう。
Let me know what you think about SenseTime's new model and if we think China is going to catch up to the US competitors and how this actually impacts the landscape.
SenseTimeの新しいモデルについてどう思うか、中国が米国の競合他社に追いつくと思うか、そしてこれが実際に景色にどのように影響するかを教えてください。
I think this is, of course, some very fascinating competition because I think we're going to continue to see models and different companies pour millions and billions of dollars into this industry.
私は、これは非常に魅力的な競争だと思います。この業界に何百万、何十億ドルもの資金を投入するモデルや異なる企業を引き続き見ることになると思います。
Because, like I said before, I think we are now in that terminal race condition where if one company stops, then they are pretty much giving up the lead.
なぜなら、前にも述べたように、私たちは今、その終着点の競争状態にあると思うからです。一つの企業が停止すれば、リードをほぼ放棄することになります。
この記事が気に入ったらサポートをしてみませんか?