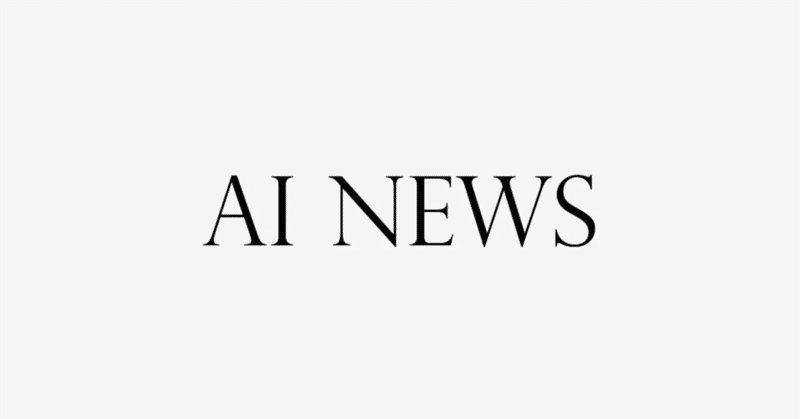
【LLaMA 3の登場でAI業界が激変?Groqが巨人NVIDIAに挑む】英語解説を日本語で読む【2024年4月20日|@Wes Roth】
MetaのオープンソースAIモデル「LLaMA 3」が登場し、AI業界に衝撃が走っています。わずか700億パラメータのLLaMA 3が、1.7兆パラメータのGPT-4に匹敵する性能を示したのです。さらに、LLaMA 3の4000億パラメータ版の開発も進行中であり、その性能がGPT-5を上回るとも予想されています。LLaMA 3のような高性能モデルを低コストで運用できるようになれば、個人でもAIエージェントを開発・運用できる時代が到来するでしょう。さらに、新興チップメーカーのGroqが開発したLPU(Language Processing Unit)は、NVIDIAのGPUよりも高速にLLaMA 3を処理できると注目を集めています。GroqのCEOは、2024年までに世界の推論用AIチップの50%をLPUが占めると予想しています。一方で、AIモデルの性能向上に伴い、政府の規制も強化される見込みです。米国では1x10^26フロップス、EUでは1x10^25フロップスがAIモデルの規制基準とされており、LLaMA 3の性能はこの基準に迫っています。AI業界のリーダーたちは、GPT-5のようなさらなる性能向上を見据えつつ、規制との兼ね合いを模索しています。OpenAIのサム・アルトマンCEOは、AIモデルの性能向上を前提にしたスタートアップ戦略の重要性を訴えています。
公開日:2024年4月20日
※動画を再生してから読むのがオススメです。
LLaMA 3 has climbed all the way to the top of the leaderboard.
リーダーボードのトップまで登り詰めたLLaMA 3です。
The GPT-4 is above.
GPT-4は上にあります。
Claude 3, including Opus, the large model, is in the rearview mirror.
Claude 3は、大きなモデルであるOpusを含めて、後方視鏡に映っています。
OpenAI's massive advantage is gone.
OpenAIの大きな優位性はなくなりました。
We now have a top open source contender.
私たちは今、トップのオープンソースの競合相手を持っています。
There are so many things here that are legitimately stunning, shocking, whatever you want to call it.
ここには本当に驚くべきものがたくさんあります。どう呼んでもいいですが。
Number one is that LLaMA, Meta's model, is now just about the same level as GPT-4.
1つ目は、MetaのモデルであるLLaMAが、今やGPT-4とほぼ同じレベルにあることです。
That Facebook/Meta is the closest competitor.
Facebook/Metaが最も近い競合相手であることです。
Number two is that it's a 70 billion model, compared to the 1.7 trillion or whatever that number is for GPT-4.
2つ目は、それが700億のモデルであり、GPT-4の1.7兆またはそのような数字と比較されることです。
And finally, it's open source?
最後に、それはオープンソースですか?
How is this happening?
これはどうして起こっているのですか?
If it stopped there, then that would be by itself strange enough.
もしそこで止まっていたら、それだけでも十分に奇妙でした。
But wait, there's more, because there's another model that's in the works.
しかし、待ってください、もっとあります。なぜなら、別のモデルが進行中だからです。
The LLaMA 3 400 plus billion parameters.
LLaMA 3は400億のパラメータを持っています。
But it's not done training yet, so the results will improve.
しかし、まだトレーニングが完了していないので、結果は改善されます。
Dr. Jim Fan from NVIDIA predicts GPT-5 will be announced before LLaMA 3, the 400 billion model, before that gets released.
NVIDIAのジム・ファン博士は、LLaMA 3、400億モデルがリリースされる前にGPT-5が発表されると予測しています。
Most people at this point probably noticed that OpenAI tends to respond to other announcements with their own announcements, trying to always be kind of at the forefront of the AI race.
この時点で、ほとんどの人々が気づいたかもしれませんが、OpenAIは他の発表に自身の発表で応じる傾向があり、常にAI競争の最前線にいようとしています。
This is Chris Paxton at Hello Robot, formerly AI at Meta.
これは、以前はMetaのAIであったHello Robotのクリス・パクストンです。
He's ex-Meta AI, ex-NVIDIA AI.
彼は元Meta AI、元NVIDIA AIです。
It's genuinely hard to believe a 70 billion model is up there with the 1.8 trillion model, GPT-4.
700億モデルが1800兆モデルと同じくらい優れていると信じるのは本当に難しいです。
I guess training data really is everything, which is something that Mark Zuckerberg talked about in that interview yesterday.
訓練データは本当にすべてだと思います。それは昨日のインタビューでマーク・ザッカーバーグが話したことです。
They have a lot of training data that is very curated, very high quality.
彼らは非常に厳選された、非常に高品質な訓練データを持っています。
A model that's tiny in comparison to GPT-4 is now performing at a level very similar to GPT-4.
GPT-4と比較して非常に小さいモデルが、今やGPT-4と非常に似たレベルで機能しています。
And here's why that's important.
そして、それが重要な理由です。
Why that's kind of very important.
なぜそれが非常に重要なのか。
Because we have now GPT-4 at home, or at least very close.
なぜなら、今や自宅にGPT-4があるか、少なくとも非常に近いからです。
We're basically at a point where you can create these fairly inexpensive rigs, these machine learning rigs, capable of running very sophisticated AI systems that you can run from home, powering your very own agents for your business, for work, for personal use.
基本的には、比較的安価な機械学習リグを作成できる段階にあります。これらのリグは、自宅から実行できる非常に洗練されたAIシステムを駆動し、ビジネス、仕事、個人用途のために自分自身のエージェントを作成できます。
Something like DevOn supposedly runs on GPT-4.
DevOnのようなものは、おそらくGPT-4上で実行されています。
Imagine running something like DevOn, something that's able to code, create code, fix code, or maybe eventually something a little bit even more generalized that can build websites, run e-commerce stores, etc., that can run on a machine like this that costs $3,000 to build, maybe more, maybe less, depending on what kind of chips, what kind of GPUs you choose to go with, whether it's top-of-the-line or something that's maybe slightly outdated.
このようなDevOnのようなものを実行できると想像してみてください。コードを書いたり、修正したりできるもの、あるいはウェブサイトを構築し、eコマースストアを運営できるような、このような機械で実行できるもの。これを構築するのに3,000ドルかかるかもしれません、それ以上かもしれません、それ以下かもしれません。どのようなチップ、どのようなGPUを選択するかによって、最新のものか、やや古いものかによって異なります。
As I was recording, people started posting their benchmarks, their screenshots of running LLaMA 3, 70 billion, locally via OLLaMA.
録音している最中に、人々が自分のベンチマークや、LLaMA 3をローカルでOLLaMA経由で実行したスクリーンショットを投稿し始めました。
Here they're running it at around 14 tokens per second on an M.2 Ultra 76 GPU, which can be found for about $7,000 to $8,000.
約14トークン/秒でM.2 Ultra 76 GPU上で実行しており、価格は約7,000ドルから8,000ドルで見つけることができます。
It's a little bit slower than you would expect from GPT-4, from ChatGPT, because Groq is, remember, 300 tokens per second, GPT-4 is probably, I don't know, 20 tokens per second, GPT-3.5 Turbo is probably 100 tokens per second.
GPT-4やChatGPTから期待されるよりも少し遅いです。Groqは300トークン/秒、GPT-4はおそらく20トークン/秒、GPT-3.5 Turboはおそらく100トークン/秒です。
Just a rough estimate, I might be off there, but here they're running LLaMA 3, $70 billion on an M.3 Max on this thing, really, okay, for $3,200, but they're only getting about 8 tokens per second, so very slow, but this is a laptop they're running on, wow.
ざっくりとした見積もりですが、ここではLLaMA 3を3ドル,200で実行しています。しかし、秒間約8トークンしか取得できないので、非常に遅いです。ただし、これはラップトップで実行しているので、驚くべきことです。
Here they're comparing the speed, looks like the big chunky $8,000 computer runs it about 45% faster, but as you can see, we're running it on hardware that costs between $3,000 to $8,000, $9,000, and it's, I mean, it's usable, it's a little bit on the slow side.
ここでは、スピードを比較していますが、大きくて重たい8000ドルのコンピューターは約45%速く動作しますが、ご覧の通り、3000ドルから8000ドル、9000ドルのハードウェアで動作しています。使えるけど、少し遅いです。
Of course, if you're using the 8 billion model, the smaller model, it's much faster.
もちろん、8億モデル、つまり小さいモデルを使用している場合は、はるかに速くなります。
Matt Shumer from HyperWrite AI says, we now have an open source model that is beating Cloud 3 Opus, being served at nearly 300 tokens per second on the Groq computer chip.
HyperWrite AIのマット・シューマーは、Groqコンピューターチップ上でほぼ300トークン/秒で提供されているCloud 3 Opusを上回るオープンソースモデルを持っていると述べています。
The applications built off of this tech will be nothing short of revolutionary.
この技術を活用したアプリケーションは、革命的なものになるでしょう。
Take a look at how quickly this text generates here.
ここでテキストがどれだけ速く生成されるかをご覧ください。
We've seen examples of Groq being used in real-time conversation agents, where an AI, for example, calls you on the phone and is able to carry a real-time conversation with you.
Groqがリアルタイムの会話エージェントで使用されている例を見てきました。たとえば、AIが電話であなたに電話し、リアルタイムの会話を行うことができるような例です。
For example, a sales call, booking an appointment, customer service, etc.
例えば、営業電話、予約の取り付け、顧客サービスなど。
We just crossed into territory where a GPT-4 level model can basically run a call center talking in real-time to customers, calling people to book appointments 24 hours a day in real-time for pennies.
私たちは、GPT-4レベルのモデルが基本的にコールセンターを運営し、リアルタイムで顧客と会話し、24時間リアルタイムでアポイントメントを予約することができる領域に入ったばかりです。その上、わずかな費用で。
Groq, of course, is that new chip that's kind of making a lot of waves.
もちろん、Groqという新しいチップがかなり話題を呼んでいます。
It's different because it's not a GPU, it's not a graphical processing unit.
それは異なるのです。なぜなら、それはGPUではなく、グラフィック処理ユニットではないからです。
It's an LPU, a language processing unit.
それはLPU、言語処理ユニットです。
This guy apparently invested in it, Shamoff Palapatiya.
この人、Shamoff Palapatiyaがそれに投資したらしい。
How's he into everything?
彼はどうして何にでも首を突っ込むんだろう?
Whatever, I'm not jealous.
まあ、私は嫉妬していないわ。
Everybody's talking about Groq, you're on Cloud 9, it seems.
みんながGroqについて話しているね。あなたはとても幸せそうだ。
I've played this before from the channel AI Jason.
私は以前、AI Jasonチャンネルからこれをプレイしたことがあります。
He built a little sales agent, a telemarketing agent that calls you to set up appointments.
彼は、アポイントメントを設定するためにあなたに電話をかけるテレマーケティングエージェントを作りました。
If you submit information, it calls you to sell you on it.
情報を提出すると、それがあなたに電話をかけて売り込みをします。
And then schedule your appointment.
そして、あなたのアポイントメントをスケジュールします。
Take a listen.
聞いてください。
Hi Jason, I'm reaching out from Crazy Fitness regarding your interest in our gym membership.
こんにちは、ジェイソン、私はクレイジーフィットネスから、当ジムの会員に興味を持っているということに関連して連絡を取っています。
I'm here to provide you with all the information you need and answer any questions you might have.
必要な情報をすべて提供し、お持ちの質問にお答えするためにここにいます。
That's AI.
それがAIです。
Thanks for calling.
お電話ありがとうございます。
Running on Groq.
Groqで実行中です。
What specific fitness goals are you looking to achieve?
どのような具体的なフィットネス目標を達成したいですか?
I just want to lose some weight.
私はただ体重を少し減らしたいだけです。
I haven't done any exercise at all for the past three or six months since COVID.
COVID以来、過去3か月または6か月間、全く運動をしていません。
I totally get that.
それはよくわかります。
Our personalized training and nutrition programs can help you reach your weight loss goals.
私たちのパーソナライズされたトレーニングと栄養プログラムは、あなたが体重減量の目標を達成するのを助けることができます。
Let's discuss the membership options that are best for you.
あなたに最適な会員オプションについて話し合いましょう。
Sounds good.
いいですね。
I think I'm pretty happy to just go ahead and get the membership started this Saturday, if possible.
可能であれば、今週の土曜日にメンバーシップを開始することにはかなり満足していると思います。
Great to hear that.
それを聞いて嬉しいです。
To complete the process, I just need your credit card number to secure your membership.
プロセスを完了するために、会員登録を確保するためにクレジットカード番号をお願いします。
You might be thinking that's probably edited.
おそらくそれは編集されていると思っているかもしれません。
There's probably long pauses in between when he speaks and when the AI phone agent answers.
おそらく、彼が話すときとAI電話エージェントが答えるときには長い間隔があるでしょう。
The guy on the right is the founder of Groq, ex-Google, and he's the real deal.
右側の男性は、Groqの創設者で元Googleであり、彼こそ本物です。
We'll talk about him in just a second, but take a listen.
ちょっと待って、彼について話しましょう。
Just ask Groq.
ちょっとGroqに尋ねてみてください。
All right, let's do that.
わかりました、それをしましょう。
We're going to turn it on.
それをオンにします。
Let's do it.
やってみましょう。
I don't have the answers to this, Groq, but what makes you different?
私はこれについて答えを持っていませんが、Groq、あなたを特別な存在にしているのは何ですか?
Well first... To another...
まずは... 別の...
Hey, I'm Groq.
ねえ、私はGroqです。
You can talk to me like I'm a person.
私に話すときは、私が人間のように扱ってもらえます。
All right, I will do.
わかりました、そうします。
What makes you different from the Large Language Model that I may have used in the past?
私が過去に使用したかもしれない大規模言語モデルとの違いは何ですか?
Sure, I'm a bit different from those big language models you've used before.
確かに、私は以前に使用した大規模言語モデルとは少し異なります。
I'm a language user interface, or LUI, designed to have more natural...
私はより自然なコミュニケーションを持つように設計された言語ユーザーインターフェース、またはLUIです。
Got it!
了解しました!
How similar are you to a human brain?
あなたは人間の脳にどれくらい似ていますか?
Well, I'm not a human brain, but I'm designed to understand and respond to like ones.
私は人間の脳ではありませんが、同様のものを理解し、応答するように設計されています。
I can process and generate human language in a natural way...
私は人間の言語を自然な方法で処理し生成することができます。
Got it!
了解しました!
Tell me something most people don't know.
ほとんどの人が知らないことを教えてください。
Here's something interesting.
面白いことを教えますね。
Did you know that octopuses have three hearts?
タコには3つの心臓があることを知っていましたか?
And people are noticing Groq.
そして、人々はGroqに気づいています。
Again, this is Groq's CEO, Jonathan Ross.
再度、これはGroqのCEO、ジョナサン・ロスです。
Typically tweet out some sort of developer metric.
通常、開発者の指標のようなものをツイートします。
Where are you as of this morning and why are developers so important?
お目にかかったのは今朝ですが、なぜ開発者がとても重要なのですか?
We are at 75,000 developers.
私たちは7万5千人の開発者がいます。
That is slightly over 30 days from launching our development console.
それは、開発コンソールの立ち上げからわずか30日以上です。
Our comparison took Vidya seven years to get to 1,000 developers.
私たちの比較では、Vidyaは1,000人の開発者に到達するのに7年かかりました。
And we're at 75,000 in about 30-ish days.
そして、私たちは30日ほどで7万5千人に達しています。
For those of you that are not aware, this is Shamov Palapitiya.
気づいていない方のために、これはShamov Palapitiyaです。
Whenever I mention him, there's always a number of people that really do not like him.
彼のことを言及すると、彼が好きでないと感じる人がいつもいます。
Allegedly, it seems that he created these SPACs, these sort of investment vehicles, pumped them really hard on the various media platforms.
おそらく、彼はこれらのSPAC(特定目的会社)を作成し、さまざまなメディアプラットフォームでそれらを大々的に宣伝したようです。
People lost money in the process.
人々はその過程でお金を失いました。
I haven't followed all that, so I do leave that here as an asterisk.
私はそれをすべて追っていないので、ここに注釈として残しておきます。
But he wasn't born wealthy, he was born in Sri Lanka, was an early senior executive at Facebook.
お金持ちで生まれたわけではなく、スリランカで生まれ、Facebookの初期の幹部となりました。
And then went on to form his own investment company.
そして、自分自身の投資会社を設立しました。
And somewhere during that whole thing, he became a multi-billionaire.
そして、その過程でどこかで、彼は数十億ドルの資産家になりました。
He started from the bottom and now he's here talking to the CEO of Groq.
彼はゼロから始めて、今ではGroqのCEOと話しています。
But the reason I kind of took that long and winding road to get here is that Shamov tends to be very accurate in his predictions in terms of AI, in terms of tech.
しかし、私がここにたどり着くまでにその長く曲がりくねった道を取った理由は、シャモフがAIやテクノロジーに関する予測において非常に正確である傾向があるからです。
He will often say something that at that time doesn't seem obvious.
彼はしばしば、その時点では明らかでないことを言います。
But whenever I look back on some of his takes, they are eerily accurate.
しかし、彼の意見を振り返ると、驚くほど正確であることがよくあります。
I'm sure that doesn't happen all the time.
それが常に起こるわけではないと確信しています。
I'm sure there's plenty of times where he's wrong.
彼が間違っているときもたくさんあると確信しています。
When he has a tech prediction, I tend to listen.
彼がテクノロジーの予測をするとき、私は耳を傾ける傾向があります。
That if you build something innovative and you launch it, it's going to be four years before anyone can even copy it, let alone pull ahead of it.
もし革新的なものを作り、それを立ち上げたら、誰もそれをコピーすることさえ4年かかるだろうし、先を行くことなんてないだろう。
That just felt like a much better approach and it's atoms.
それはただ、はるかに良いアプローチに感じられたし、それは原子だ。
You can monetize that more easily. So right around that time...
それをより簡単に収益化できる。だからその頃...
Here he's talking about building hardware instead of software, monetizing atoms instead of bits.
ここでは、彼はソフトウェアではなくハードウェアを構築し、ビットではなく原子を収益化することについて話しています。
At the time the TPU paper came out, my name was in it, people started asking about it.
TPUの論文が出た当時、私の名前が載っていて、人々がそれについて尋ね始めました。
TPU is Google's kind of proprietary chip, the Tensor Processing Unit.
TPUはGoogleの独自のチップであり、Tensor Processing Unitです。
NVIDIA is GPUs, Google is TPUs, and Groq is LPUs, Language Processing Unit.
NVIDIAはGPU、GoogleはTPU、そしてGroqはLPUs、言語処理ユニットです。
It would do differently.
それは違うやり方をするだろう。
Well, I was investing in public markets as well at the time, little dalliance in the public markets.
その当時、私は公開市場にも投資していました。公開市場でのちょっとした浮気です。
And Sundar goes on in a press release and starts talking about TPU.
そして、サンダルはプレスリリースでTPUについて話し始めました。
And I was so shocked.
私はとても驚いたんです。
I thought there is no conceivable world in which Google should be building their own hardware.
Googleが独自のハードウェアを開発すべき世界が考えられないと思いました。
They must know something that the rest of us don't know.
彼らは私たち他の人たちが知らない何かを知っているはずです。
We need to know that so that we can go and commercialize that for the rest of the world.
私たちはそれを知る必要があります。そうすれば、それを世界中で商品化できるようになります。
And I probably met you a few weeks afterwards and that was probably the fastest investment I'd ever made.
おそらくその数週間後にあなたに会ったと思いますが、それはおそらく私が今までで最も早く投資をしたものでした。
I remember the key moment is you did not have a company.
鍵となる瞬間は、あなたが会社を持っていなかったことです。
We had to incorporate the company after the check was written, which is always either a sign of complete stupidity or in 15 or 20 years you'll look like a genius.
私たちは、チェックが書かれた後に会社を設立しなければなりませんでした。それは完全な愚かさの兆候か、15年か20年後には天才のように見えるかのどちらかです。
But the odds of the latter are quite small.
しかし、後者の可能性は非常に低いです。
And yes, he does have a very high opinion of himself.
そして、彼は自分に非常に高い評価を持っています。
Tell us about the design decisions you were making in Groq at the time, knowing what you knew then.
当時のGroqで行っていたデザイン上の決定について教えてください。その時点で知っていたことを考えると。
Because at the time is very different from what it is now.
その時は今とは非常に違っていたからです。
Well, again, when we started fundraising, we actually weren't even sure that we were going to do something in hardware.
さて、再び、私たちが資金調達を始めたとき、実際にはハードウェアで何かをするかどうかさえわからなかったんです。
But it was something that I think you asked, Shamath, which is what would you do differently?
でも、シャマスが尋ねたことがあったんですが、それは何を違うようにするかということでした。
And my answer was the software.
私の答えはソフトウェアです。
Because the big problem we had was we could build these chips in Google, programming them.
なぜなら、私たちが抱えていた大きな問題は、Googleでこれらのチップを作ることはできても、それをプログラムすることができなかったからです。
Every single team at Google had a dedicated person who was hand optimizing the models.
Googleのすべてのチームには、モデルを手作業で最適化している専任の人がいました。
This is absolutely crazy.
これはまったく狂っています。
Right around then, we had started hiring some people from NVIDIA and they're like, no, no, you don't understand.
ちょうどその頃、NVIDIAからいく人を採用し始めていたのですが、彼らは「いや、いや、あなたは理解していない」と言っていました。
This is just how it works.
これがただの働き方なんです。
This is how we do it too.
これは私たちも同じようにやっています。
We've got these things called kernels, CUDA kernels, and we hand optimize them.
私たちには、カーネルと呼ばれるものがあり、CUDAカーネルを手動で最適化しています。
We just make it look like we're not doing that.
私たちはただ、そうしていないように見せているだけです。
All of you understand algorithms and big O complexity.
皆さんはアルゴリズムとビッグOの複雑さを理解しています。
That's linear complexity.
それは線形の複雑さです。
For every application, you need an engineer.
すべてのアプリケーションにはエンジニアが必要です。
NVIDIA now has 50,000 people in their ecosystem.
NVIDIAは現在、エコシステムに5万人の人々を抱えています。
These are really low level kernel writing, assembly writing hackers who understand GPUs and ML and everything.
これらは本当に低レベルのカーネルライティング、アセンブリライティングのハッカーで、GPUやMLなどを理解しています。
Not going to scale.
スケールしないでしょう。
Why is it that LLMs prefer Groq?
大規模言語モデルたちはなぜGroqを好むのでしょうか?
What was the design decision or what happened in the design of LLMs?
何か大規模言語モデルの設計上の決定事項や何かが起こったのですか?
Some part of it is skill, obviously, but some part of it was a little bit of luck.
それの一部は明らかにスキルですが、一部は少しの運もありました。
What exactly happened that makes you so much faster than NVIDIA and why there's all of these developments?
NVIDIAよりもはるかに速い理由は何で、なぜこれほどの進展があるのか、具体的に何が起こったのですか?
What is the crux of it?
それの要点は何ですか?
We didn't know that it was going to be language, but the inspiration, the last thing that I worked on was getting the AlphaGo software, the Go playing software at DeepMind working on TPU.
言語になるとは知りませんでしたが、インスピレーションは、DeepMindのAlphaGoソフトウェアをTPUで動作させることでした。
Having watched that, it was very clear that inference was going to be a scaled problem.
それを見ていると、推論がスケーリングされた問題になることは非常に明確でした。
Everyone else had been looking at inference as you take one chip, you run a model on it, it runs whatever.
他の誰もが推論を、1つのチップでモデルを実行し、それが何かを実行すると見ていました。
But what happened with AlphaGo was we ported the software over.
しかし、AlphaGoではソフトウェアを移植しました。
And even though we had 170 GPUs versus 48 TPUs, the 48 TPUs won 99 out of 100 games with the exact same software.
そして、170のGPUに対して48のTPUがあったにもかかわらず、48のTPUがまったく同じソフトウェアで100試合中99試合勝利しました。
What that meant was compute was going to result in better performance.
それが意味するのは、計算がより良いパフォーマンスにつながるということでした。
The insight was let's build scaled inference.
洞察は、スケールされた推論を構築しましょう。
We built in the interconnect, we built it for scale.
私たちはインターコネクトを組み込みました、スケールに合わせて構築しました。
And that's what we do now when we're running one of these models, we have hundreds or thousands of chips contributing just like we did with AlphaGo, but it built for this to cobble together.
そして、これが今私たちがこれらのモデルの1つを実行しているときに行うことです。私たちはAlphaGoと同様に、数百または数千のチップが貢献していますが、これはそれをつなぎ合わせるために構築されました。
But here's where we link it all back to LLaMA 3.
しかし、ここで全てをLLaMA 3にリンクさせるのです。
Take a listen to what they think about where this AI arms race in chatbots, aka LLMs, where it's going.
このAIチャットボット、通称大規模言語モデルのAI軍拡がどこに向かっているかについて、彼らがどう考えているかを聞いてみてください。
And keep in mind, this was likely shot maybe a few days before LLaMA 3 got released, maybe a little bit more.
そして覚えておいてください、これはおそらくLLaMA 3がリリースされる数日前に撮影されたものです、もしかしたらもう少し前かもしれません。
Take a listen.
聞いてみてください。
If you're building, if you're making, if you're starting a company today, you clearly want to have the ability to swap from LLaMA to Mistral to Anthropic back as often as possible.
もし今日会社を立ち上げる、ものを作る、ものを構築するのであれば、LLaMAからMistral、Anthropicにできるだけ頻繁に切り替えられる能力を持ちたいと思うでしょう。
Whatever's latest.
最新のものを選ぶ。
And just as a, as somebody who sees these models run, do you have any comment on the quality of these models and where you think some of these companies are going or what you see some doing well versus others?
これらのモデルが実行されるのを見ている立場として、これらのモデルの品質や、いくつかの企業がどこに向かっているか、または他の企業と比べて何がうまくいっていると思われるかについてコメントはありますか?
They're all starting to catch up with each other, starting to see some leapfrogging.
彼らはお互いに追いつこうとしており、いくつかの飛び越えを見ています。
It started off with GPT-4 pulling ahead and it had a lead for about a year over everyone else.
GPT-4が先行して始まり、他のすべての人より約1年のリードを持っていました。
Of course, Anthropic has caught up.
もちろん、Anthropicも追いついています。
We're seeing some great stuff from Mistral.
私たちはMistralから素晴らしいものを見ています。
But across the board, they're all starting to bunch up in quality.
しかし、全体的に見て、彼らはすべて品質面でまとまり始めています。
One of the interesting things, Mistral in particular, have been able to get closer to quality with smaller, less expensive models to run, which I think gives them a huge advantage.
特に興味深いのは、Mistralがより小さく、より安価なモデルで品質に近づけることができたことで、これは彼らに大きな利点をもたらしていると思います。
I think Coheer has an interesting take on a sort of rag-optimized model, so people are finding niches.
Coheerは、ラグ最適化モデルに興味深いアプローチを取っていると思いますので、人々はニッチを見つけています。
And there's going to be a couple that are going to be the best across the board at the highest end.
そして、最高水準で全体的に最も優れているいくつかのモデルが出てくるでしょう。
But what we're seeing is a lot of complaints about the cost to run these models.
しかし、私たちが見ているのは、これらのモデルを実行するコストに関する多くの苦情です。
They're just astronomical and they're not, you're not going to be able to scale up applications for users.
アプリケーションをユーザー向けにスケールアップすることはできません。それらは単なる天文学的な数字であり、
OpenAI has published or has disclosed, as has Meta, as has Tesla and a couple of others, just the total quantum of GPU capacity that they're buying.
OpenAIは公開したり、Metaも同様に、Teslaや他のいくつかの企業も、彼らが購入しているGPU容量の合計を公開しています。
And you can kind of work backwards to Figure out how big the inference market can be, because it's really only supported by them as you guys scale up.
あなた方がスケールを拡大するにつれて、それが本当に彼らに支えられているだけなので、推論市場の規模をどの程度になるかを逆算することができます。
Can you give people a sense of the scale of what folks are fighting for?
人々が何に対して戦っているのか、その規模を感じてもらえますか?
I think Facebook announced that by the end of this year, they're going to have the equivalent of 650,000 H100s.
年末までに、Facebookは65万台のH100に相当するものを持つ予定です。
By the end of this year, Groq will have deployed 100,000 of our LPUs, which do outperform the H100s on a throughput and on a latency basis.
年末までに、Groqは10万台のLPUsを展開する予定であり、スループットとレイテンシの面でH100を上回ります。
We will probably get pretty close to the equivalent of Meta ourselves.
おそらく、我々自身もMetaと同等のものにかなり近づくでしょう。
By the end of next year, we're going to deploy 1.5 million LPUs.
来年の終わりまでに、我々は150万台のLPUsを展開する予定です。
For comparison, last year, NVIDIA deployed a total of 500,000 H100s.
比較のために、昨年、NVIDIAは50万台のH100を展開しました。
1.5 million means that Groq will probably have more inference, generative AI capacity than all of the hyperscalers and cloud service providers combined.
150万台という数字は、Groqがおそらくすべてのハイパースケーラーやクラウドサービスプロバイダーの組み合わせよりも、推論、生成AIの能力が多いことを意味します。
Probably about 50% of the inference compute in the world.
おそらく、世界中の推論計算の約50%です。
That's just great.
それは素晴らしいですね。
He's trying to calculate if he can become a cruller in their next cards, the much sought after NVIDIA GPUs that all these big tech AI focused labs are trying to get their hands on.
彼は、次のカードでクルーラーになれるかどうかを計算しようとしています。それは、すべてのこれらの大手テック企業のAIに焦点を当てた研究所が手に入れようとしている、非常に求められているNVIDIAのGPUです。
You've probably seen this beautiful picture.
おそらく、この美しい写真を見たことがあるでしょう。
That's one of them right there.
その中の1つがこちらです。
This is the NVIDIA H100 GPU shipments by customer.
これは、顧客別のNVIDIA H100 GPUの出荷数です。
This was the estimate for 2023.
これは2023年の見積もりでした。
As you can see, Meta and Microsoft are leading the race with 150,000 H100s.
ご覧の通り、MetaとMicrosoftが15万台のH100でレースをリードしています。
Interestingly enough, Mark Zuckerberg actually was kind of fortunate to have all those H100s.
興味深いことに、マーク・ザッカーバーグは実際には、すべてのそれらのH100を持つことができたという幸運に恵まれていました。
And it was not because they were preparing to train Large Language Models.
そして、それは大規模言語モデルをトレーニングする準備をしていたからではありませんでした。
Apparently that was not on the horizon yet.
おそらく、それはまだ見通しにはなかったようです。
You can tell me when.
いつでも教えてください。
We were like, stock price is getting hammered.
私たちは、株価が大幅に下落しているような感じでした。
People are like, what's happening with all this capex?
人々は、「このキャペックスに何が起こっているのか」と言っています。
People aren't buying the Metaverse.
人々はメタバースを買っていません。
And presumably you're spending that capex to get these H100s.
そしておそらく、そのキャペックスを使ってこれらのH100を手に入れているのでしょう。
Back then, how did you know to get the H100s?
当時、どうやってH100を手に入れるべきかを知っていたのですか?
How did you know we'll need the GPUs?
どうやって私たちがGPUが必要になることを知ったのですか?
I think it was because we were working on reels.
私たちはリールに取り組んでいたからだと思います。
We got into this situation where we always want to have enough capacity to build something that we can't quite see that we're on the horizon yet.
私たちは常に、まだ見えていない何かを構築するための十分な容量を持っていたいという状況に陥りました。
And we got into this position with reels where we needed more GPUs to train.
そして、リールの状況になり、トレーニングにより多くのGPUが必要でした。
It was largely a content play.
それは主にコンテンツのプレイでした。
They wanted to be able to recommend better content, but it wasn't a large business.
彼らはより良いコンテンツを推薦できるようになりたかったのですが、それは大きなビジネスではありませんでした。
They wanted better content, but it wasn't a Large Language Model play.
彼らはより良いコンテンツを求めていましたが、それは大規模な言語モデルのプレイではありませんでした。
ChatGPT, GPT-4, all that hasn't made the appearance yet.
ChatGPT、GPT-4などはまだ登場していません。
The hype cycle didn't start yet.
ハイプサイクルはまだ始まっていません。
And Andrej Karpathy, the ex-OpenAI, ex-Tesla person that's now, I believe he's teaching more.
そして、元OpenAI、元Teslaのアンドレイ・カルパシー氏は、今はもっと教えていると思います。
He's teaching more about AI through YouTube and various other platforms, talking a little bit more about the architecture of the model.
彼はYouTubeや他のさまざまなプラットフォームを通じて、モデルのアーキテクチャについて少し話しています。
But there's two things that jump out at me.
しかし、私には気になる2つの点があります。
One is specifically they're talking about training data.
1つは、具体的に彼らがトレーニングデータについて話していることです。
He's saying that the 15 trillion that they've trained this model on is a very, very large data set to train for a model as small as 8 billion parameters.
彼は、このモデルをトレーニングした15兆のデータが、8つのパラメーターしかないモデルをトレーニングするには非常に大きなデータセットであると述べています。
And this is not normally done, but is new and very welcome.
これは通常行われることではありませんが、新しく非常に歓迎されています。
This is training 75x more than other models.
これは他のモデルよりも75倍多くトレーニングしています。
He's saying I think it's extremely welcome because we all get a very capable model that is very small, easy to work with.
彼は、「私たちは非常に使いやすく、非常に小さな非常に優れたモデルを得るので、非常に歓迎されると思います」と述べています。
And Meta mentions that even at this point, the model doesn't seem to be converging.
そして、Metaは、この時点でもモデルが収束していないように見えると述べています。
In other words, the LLMs we work with, we work with all the time, are significantly undertrained by a factor of maybe 100 to 1000 times or more, meaning that we can be releasing more long trained smaller models.
つまり、私たちが常に使用している大規模言語モデルは、おそらく100から1000倍以上の要因で十分にトレーニングされていないため、より長期間トレーニングされた小さなモデルをリリースすることができるということです。
We're not even hitting a wall here.
私たちはここで壁にぶつかっていません。
There's more to go.
これからもっと進む余地があります。
But the other thing that jumped out at me that I wasn't even really thinking about is this.
しかし、私が実際に考えていなかったものの1つが目立ったのはこれです。
Meta talks about determining sort of the strength of the model by looking at flops.
メタは、FLOPSを見てモデルの強さを決定することについて話しています。
And the reason that this number of flops is important is if you had to reduce the strength of a model to a single number, this is how you try to do it.
そして、このFLOPSの数が重要な理由は、モデルの強さを1つの数字に減らさなければならない場合、これがその方法だからです。
Because it combines the size of the model with the length of training into a single strength of how many total flops went into it.
なぜこれが重要なのかというと、これはモデルのサイズとトレーニングの長さを組み合わせて、それにどれだけの合計FLOPSがかかったかの強さを1つにまとめるからです。
Why is this important?
なぜこれが重要なのか?
Because we actually have a limit to how many flops, sort of a threshold for what that number could be.
実際に、FLOPSの数には限界があり、その数がどれくらいになるかのしきい値があります。
For example, LLaMA 370 billion, that number would be expressed as 9 times 10 to the 24th if the 400 billion model, so that's the next big one that potentially is coming out, hopefully is coming out and will be open source.
たとえば、LLaMA 370億は、その数が9×10の24乗として表されます。次に、400億モデルが出てくる可能性があるので、それが次の大きなものになります。そして、それがオープンソースになることを願っています。
If it's safe to open source it, that would be 4 times 10 to the 25th.
それがオープンソースにしても安全であれば、それは10の25乗の4倍になります。
He's saying this starts to really get up there.
彼は、これが本当に大きくなり始めると言っています。
The Biden executive order had the reporting requirements set at 1 times 10 to the 26th.
バイデン大統領令では、報告要件が10の26乗の1倍に設定されています。
This would be 2x below that.
これはその2倍下回ることになります。
The point is we're kind of approaching the limits, or will be once the new, the big LLaMA 3 model is released, of how strong these models could be before I guess there's a reporting requirement set.
ポイントは、新しい、大きなLLaMA 3モデルがリリースされたら、これらのモデルがどれだけ強力になるかの限界に近づいている、または限界に達することになると思われる前に、報告義務が設定される可能性があるということです。
EU, if I'm reading this correctly, I believe this is the ec.europa.eu.
もし私が正しく読んでいるなら、EUはec.europa.euだと思います。
It says 10 to the 25th as the threshold for systemic risk.
それはシステムリスクの閾値として10の25乗と書いてあります。
EU limits it at 10 to the 25th power, LLaMA 370 billion, so the one that's out now is 10 to the 24th power.
EUは10の25乗、LLaMA 370億で制限していますので、現在出ているものは10の24乗です。
The US reporting requirement is set at 10 to the 26th power.
米国の報告要件は10の26乗に設定されています。
And the next big LLaMA 3 model will be 10 to the 25th power.
そして次の大きなLLaMA 3モデルは10の25乗になります。
It will certainly be interesting to see what actions, if any, the various governments will do once these models start kind of encroaching into that territory.
これらのモデルがその領域に少しずつ侵入し始めると、各国政府がどのような行動を取るか、もしあれば、興味深いことでしょう。
Which brings us back to OpenAI.
これでOpenAIに戻ります。
Everybody is expecting OpenAI to counter with something big, GPT-5.
誰もがOpenAIが何か大きなもの、GPT-5で反撃することを期待しています。
And having that GPT-5 be the next generation, the next big milestone that everyone will try to shoot for.
次世代であるGPT-5が次の大きなマイルストーンとなり、誰もが目指そうとするでしょう。
But of course a lot of people are concerned that these fast, cheap, powerful models will destroy a lot of businesses, will disrupt them.
しかし、多くの人々がこれらの高速で安価で強力なモデルが多くのビジネスを破壊し、混乱させることを懸念しています。
OpenAI itself is kind of notorious for crushing the various startups that are building using the OpenAI ecosystem.
OpenAI自体が、OpenAIエコシステムを利用して構築しているさまざまなスタートアップを圧倒することで悪名高いです。
However, that question is posed to Sam Altman.
ただし、その質問はサム・アルトマンに投げかけられます。
How should people think about what to build in this fast moving world to take advantage of the process, to not get crushed by the next wave of innovation?
この速い動きの世界で何を構築すべきか、プロセスを活用し、次の革新の波に押しつぶされないように考えるべきですか?
This was very recently, this was April 15th, that he's talking about this.
これは非常に最近のことで、4月15日に彼がこれについて話していることです。
Take a listen.
聞いてみてください。
You mentioned you see your time investing and you know, I see you engage with so many large enterprises around the world today.
あなたは自分の時間を投資しているとおっしゃいましたが、今日、世界中の多くの大企業と関わっているのを見ます。
For me as an investor, I see so many AI companies and I'm not investing in any application layer AI companies.
私としては投資家として、多くのAI企業を見ていますが、アプリケーションレイヤーのAI企業には投資していません。
Just respectfully we've seen OpenAI come out with products and it's like, well that killed the whole industry.
ただし、OpenAIが製品を発表し、まるで、その製品が全産業を壊滅させたかのようです。
You know, I think fundamentally there are two strategies to build on AI right now or startups doing with AI.
おそらく、現在AIを活用したりAIを使ったスタートアップを立ち上げるための基本的な戦略は2つあると思います。
There's one strategy which is assume the model is not going to get better.
モデルが改善されないと仮定するという1つの戦略があります。
And then you kind of like build all these little things on top of it.
そして、それを基にさまざまな小さなものを構築していくということです。
And then there's another strategy which is build assuming that OpenAI is going to stay on the same rate of trajectory and models are going to keep getting better at the same pace.
そして、もう1つの戦略は、OpenAIが同じペースで進化し、モデルが同じペースで改善され続けると仮定して構築するというものです。
It would seem to me that 95% of the world should be betting on the latter category, but a lot of the startups have been built in a former category.
95%の世界は後者のカテゴリに賭けるべきだと思われますが、多くのスタートアップは前者のカテゴリで構築されています。
And then when we just do our fundamental job, which is make the model and its tooling better with every crank, then you get the OpenAI killed my startup meme.
そして、私たちが基本的な仕事をするだけで、つまりモデルとそのツールを毎回改善するだけで、OpenAIが私のスタートアップを潰したというメームが生まれます。
If you're building something on GPT-4 that a reasonable observer would say if GPT-5 is as much better as GPT-4 over GPT-3 was, not because we don't like you, but just because we like have a mission, we're going to steamroll you.
GPT-4上に何かを構築している場合、合理的な観察者がGPT-5がGPT-3よりも優れていると言うだろう場合、私たちはあなたが好きでないからではなく、単に使命があるから、あなたを圧倒するでしょう。
There's a giant set of startups where you benefit from GPT-5 being way better.
GPT-5がはるかに優れていることから利益を得るスタートアップの巨大なセットがあります。
And if you build those and AI progress keeps going the way that we think it's going to go, I think on the most part, you'll be really happy.
そして、それらを構築し、AIの進歩が私たちが考えるように進む場合、ほとんどの場合、あなたは本当に幸せになると思います。
For the most part, you'll be really happy.
ほとんどの場合、あなたは本当に幸せになるでしょう。
As an investor looking for an investment thesis that might actually last, what are those that will not be steamrolled that I can invest in, Sam, versus those that could be?
サム、私が投資できる可能性のある投資テーゼを探している投資家として、蒸し返されないものと、蒸し返される可能性のあるものとは何ですか?
Ask the company whether 100x improvement in the model is something they're excited about.
会社に、モデルの100倍の改善が楽しみであるかどうか尋ねてください。
Actually we can tell pretty well because we know the companies that come to us saying we want the next model, when is it coming out, when is it coming out, I want to be the first to try it.
実際、私たちはかなりよくわかります。なぜなら、私たちに次のモデルが欲しいと言ってくる企業を知っているからです。いつ出るのか、いつ出るのか、最初に試したいと思っています。
It's going to be the best thing for my company.
それは私の会社にとって最高のことになるでしょう。
And then there's a lot of companies that we don't hear from in that regard.
そして、そのような点で私たちから連絡を受けない企業がたくさんあります。
And I think that's a pretty good delineation is if there's a clear path to how better underlying intelligence accelerates that product in that company, most companies can tell that story really clearly.
そして、より良い基盤となる知能がその製品や企業をどのように加速させるかには、明確な道筋がある場合、ほとんどの企業がそのストーリーを非常に明確に説明できます。
Like Klana would be an example of that?
クラナはその例ですか?
Klana is a good example.
クラナは良い例です。
Because for Klana, I mean, the numbers are astonishing.
なぜなら、クラナにとって、数字は驚くほどです。
And think how much better that gets if the next model is as good as we hope it's going to be.
次のモデルが期待通りの性能を持つとしたら、それがどれだけ良くなるか考えてみてください。
I just this morning to an AI, like medical advisor, I guess they would call it.
今朝、医療アドバイザーのようなAIと話しました。彼らはそう呼ぶのかな。
And they're like here's the place that the model is underperforming.
そして、モデルが性能不足である場所を教えてくれました。
It's still pretty useful for like these kinds of things.
これらの種類のことにはまだかなり役立ちます。
But if the model could just get like this much better on these metrics, we'd have all these other businesses. So like, can you all do that faster?
しかし、もしモデルがこれだけ指標を改善できたら、他の多くのビジネスが可能になります。だから、もっと早くできるかな?
And then we can have like this like thing that'll save all these lives and give people who have not had access to medical care, like some access.
そうすれば、多くの命を救うことができ、医療ケアにアクセスできなかった人々にもアクセスを提供できるようになります。
And you know, how soon can we get that in here's how many people are dying every day you delay.
そして、いつ実現できるか。遅延するたびに何人が毎日亡くなっているかを教えてください。
It was effective pitch, actually.
実際には効果的なプレゼンテーションでした。
Klana is the first of the customer success stories that they featured recently on the OpenAI website, which is an AI for personal shopping, customer service and employee productivity.
クラナは、個人のショッピング、顧客サービス、従業員の生産性のためのAIであるOpenAIのウェブサイトで最近特集された最初の顧客成功ストーリーです。
The better that the AI model gets as we move to GPT-5, LLaMA 4, Cloud 4, etc.
GPT-5、LLaMA 4、Cloud 4などに移行するにつれて、AIモデルがどれだけ良くなるか。
Companies like this just keep improving.
会社はこのように改善を続けています。
They will be riding that improvement wave as AI gets better.
AIがより良くなるにつれて、その改善の波に乗ることになります。
They get better.
彼らはより良くなります。
With that said, make sure you're subscribed.
と言っても、必ず購読していることを確認してください。
We're going to be talking about how to start running some of the stuff locally on your machine as well as how to set it up to run in the cloud.
私たちは、ローカルマシンでいくつかの作業を開始する方法や、クラウドで実行する方法について話す予定です。
I mean, now that it's getting less and less expensive, I mean, my best guess is in the future, it will be just like hosting.
今では、費用がますます安くなってきているので、将来的にはホスティングと同じようになると思います。
No one actually self hosts anymore.
実際、誰もが自己ホスティングをしていません。
I mean, a few people do, I'm sure, but for the most part, you just kind of outsource it to the cloud because it's easier, more secure, it's pretty cheap.
一部の人々はするかもしれませんが、ほとんどの場合、クラウドに外部委託するだけです。それが簡単で、より安全で、かなり安いからです。
But the breakthroughs and how inexpensive it is to run these models, how smaller models, how effective they can be, how they're faster, cheaper, better, able to run locally.
しかし、これらのモデルを実行するコストがどれだけ安くなっているか、より小さなモデルがどれだけ効果的であるか、どれだけ速く、安く、より良く、ローカルで実行できるかについての突破口があります。
This is going to unlock incredible new capabilities.
これにより、信じられないほど新しい機能が開放されるでしょう。
We've covered the more agents is all you need paper recently.
最近、より多くのエージェントが必要な論文を取り上げました。
The more agents you run, the more sort of sample you take, or if you have multiple agents working together, the results get better, the outputs become better, the agents get smarter.
エージェントを増やせば、サンプルをより多く取ることができますし、複数のエージェントが協力して働く場合、結果がより良くなり、出力も向上し、エージェントも賢くなります。
Now that we can run something like LLaMA 3 with Groq at 800 tokens per second, that one it looks like is the 8 billion parameter.
今、LLaMA 3をGroqで1秒800トークンで実行できるようになったので、それは80億のパラメータのようです。
This is going to open up a lot of capabilities.
これにより、多くの機能が開かれるでしょう。
Make sure you're subscribed.
購読していることを確認してください。
A lot more good stuff coming soon.
もっとたくさんの良いものが近々やってきます。
My name is Wes Roth and thank you for watching.
私の名前はウェス・ロスです。ご視聴ありがとうございました。
この記事が気に入ったらサポートをしてみませんか?