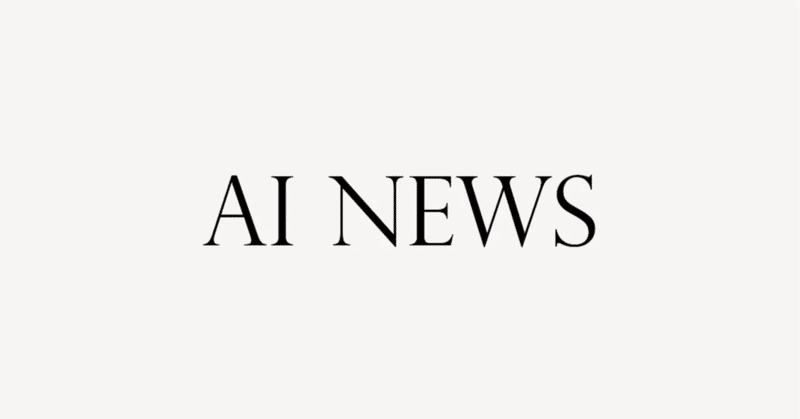
【あなたのビジネスを変革するOpenAIのカスタムAIモデル】英語解説を日本語で読む【2024年4月5日|@Wes Roth】
OpenAIが、科学、チャットアプリ、医療など様々な業界向けにAI技術を提供し、ビジネスの革新を目指しています。中心には、GPTやDALL·Eなどの基盤AIモデルがあり、これを用いて企業や開発者は特定のニーズに合わせたアプリやサービスを開発できます。最近では、外部の企業がOpenAIの技術を活用しやすくなり、カスタムモデルプログラムの拡張やファインチューニングツールが提供されています。例えば、IndeedはOpenAIの技術を利用して仕事推薦の精度を向上させ、コストを削減しました。このような動きは、将来的に企業が自社に特化したAIを活用することが一般的になることを示しています。OpenAIの取り組みにより、AI技術のカスタマイズが新たな標準となりつつあり、ビジネスのあり方に革命をもたらしています。
公開日:2024年4月5日
※動画を再生してから読むのがオススメです。
Sam Altman and the team behind OpenAI have been kind of quiet for a while.
サム・アルトマンとOpenAIのチームはしばらく静かでした。
A calm before the storm, if you will.
もし許していただけるなら、嵐の前の静けさとでも言いましょう。
But in the last few days, we've been getting some glimpses into what potentially is coming next.
しかし、ここ数日で、次に何が来るかについて少し垣間見ることができるようになりました。
Let's take a look at what they've been cooking up.
彼らが何を考えているのか見てみましょう。
Interestingly enough, this seems to be a nod back to their original goal, their original stated mission of being the AI base layer.
興味深いことに、これは彼らの元々の目標、AIの基礎レイヤーであるという元の声明された使命に戻るようです。
Here I think is a great illustration of what they meant by that.
ここで、彼らがそれを意味したと思われる素晴らしい例があります。
Imagine this sort of big block here.
ここに大きなブロックがあると想像してください。
That's layer one.
それがレイヤー1です。
That's the base layer AI.
それが基礎レイヤーAIです。
That's the GPT.
それがGPTです。
That's the Sora.
それがSoraです。
That's the DALL·E.
それがDALL·Eです。
And on top of that, we build the various companies, various apps, various software that takes advantage of this base layer of this AI to build specific functionality.
そしてその上に、このAIの基盤を活用して特定の機能を構築するためのさまざまな企業、さまざまなアプリ、さまざまなソフトウェアを構築しています。
For example, for science, for chat applications, for medical AI, etc.
例えば、科学、チャットアプリケーション、医療AIなどです。
When ChatGPT exploded onto the scene, OpenAI did say that this was kind of the plan for the future.
ChatGPTが登場すると、OpenAIはこれが将来の計画の一環だと述べました。
They were going to be the base layer and people could build on top of it.
彼らは基盤となり、人々がそれに上乗せして構築できると言いました。
Tons of people and apps did build on top of it.
たくさんの人々やアプリがそれに上乗せして構築しました。
But then OpenAI was like, I am death and just killed them all by building their own sort of versions of it, their own applications.
しかし、その後OpenAIは、「私は死神だ」と言って、それらすべてを自分たちのバージョンやアプリケーションを構築することで排除しました。
That's probably not 100% fair because a lot of these startups, they were trying to build technology to fill in the gaps that OpenAI was missing.
これはおそらく100%公平ではないでしょう、なぜならこれらの多くのスタートアップは、OpenAIが欠けている部分を埋める技術を構築しようとしていました。
For example, things like whisper, things that were similar to Code Interpreter or advanced data analytics that it's called.
例えば、ささやきのようなもの、コードインタプリターに似たもの、それを呼ばれる高度なデータ分析などがあります。
In other words, a lot of these startups, they were building kind of these obvious apps that OpenAI itself probably had in the works and they kind of got rolled over by OpenAI, by the base layer, if you will.
言い換えると、これらの多くのスタートアップは、OpenAI自体がおそらく進行中だった明らかなアプリを構築していたが、OpenAIによって転覆された、基盤となるレイヤーによって。
But it seems that now the game has changed.
しかし、今では状況が変わったようです。
OpenAI seemingly, now that it has all the pieces in place, it has the Sora, the whisper, the voice engine, it has advanced data analytics, it has DALL·E with inpainting and editing and all that stuff.
OpenAIは、今や全ての要素が整っているようで、Sora、ささやき、音声エンジン、高度なデータ分析、DALL·E(修復と編集を含む)などを持っています。
Like it's built all of the different pieces of the base layer, their full functionality.
まるで、基盤となるレイヤーの異なる部分をすべて構築して、その全機能を持っているかのようです。
You can talk back and forth to ChatGPT.
ChatGPTとやり取りすることができます。
It's able to understand your speech.
あなたの話し言葉を理解することができます。
It's able to talk back to you.
あなたに話し返すことができます。
The base layer, a lot of it is complete.
基盤となるレイヤーの多くは完成しています。
All the companies that try to plug missing pieces of the base layer are dead.
基盤となるレイヤーの欠けている部分を補う企業は全滅しています。
Seemingly OpenAI is saying, okay guys, we've got the foundation, we've got the base layer, build stuff on top of it.
OpenAIは、基盤を持っているし、ベースレイヤーも持っているから、それに上に何かを構築してほしいと言っているようです。
That's my opinion, but let me show you a few key pieces of news that came out just in the last few days that I think support this viewpoint.
私の意見ですが、ここ数日で出てきたいくつかの重要なニュースを見せて、この視点を支持すると思うものです。
As you look at this, ask yourself this question, is OpenAI rolling out the red carpet for the layer two startups to build on top of it, on top of OpenAI's base layer.
これを見て、自分自身にこの質問をしてみてください。OpenAIは、レイヤー2のスタートアップがOpenAIのベースレイヤーの上に何かを構築するために赤いカーペットを敷いているのでしょうか。
Let's get started.
さあ始めましょう。
This drop today, introducing improvements to the fine tuning API and expanding our custom models program.
今日のこの新機能の導入は、ファインチューニングAPIの改善とカスタムモデルプログラムの拡大を紹介しています。
Developers get more control over fine tuning and new ways to build custom models with OpenAI.
開発者は、ファインチューニングに対するより多くの制御権を得て、OpenAIと新しい方法でカスタムモデルを構築する方法を得ます。
It begins, there are a variety of techniques that developers can use to increase model performance in an effort to reduce latency, improve accuracy, reduce costs.
始まります。開発者がモデルのパフォーマンスを向上させるために使用できるさまざまな技術があり、レイテンシを減らし、精度を向上させ、コストを削減することを目指しています。
Whether it's extending model knowledge with RAG, retrieval, augmented generation.
RAG、リトリーバル、拡張生成など、モデルの知識を拡張するかどうか。
Basically having that model as example would be to search the internet for information like Perplexity, it returns to you the various links it finds.
基本的に、そのモデルを例として、Perplexityのような情報をインターネットで検索して、見つけたさまざまなリンクを返します。
And then kind of summarize them.
そして、それらを要約します。
Or if you've ever done the chat with a PDF thing, right, the PDF is your sort of database, extra data, extra info that you have in there.
もしあなたがPDFを使ったチャットをしたことがある場合、PDFはあなたのデータベース、追加データ、追加情報です。
The model, instead of kind of making up an answer, it retrieves information from that PDF and then answers with that information in mind various custom models, fine tuning, custom trained models with new domain specific knowledge, and they're launching new features to give developers more control over fine tuning.
そのモデルは、答えをでっち上げる代わりに、そのPDFから情報を取得し、その情報を考慮してさまざまなカスタムモデル、微調整、新しいドメイン固有の知識を持つカスタムトレーニングされたモデルで回答します。そして、開発者が微調整により多くの制御を持つための新機能を提供しています。
And they're introducing more ways to work with our team of AI experts and researchers to build custom models.
そして、AIの専門家や研究者のチームと協力してカスタムモデルを構築するためのさらなる方法を導入しています。
And they're not kidding.
そして、彼らは冗談を言っているわけではありません。
As you'll see in a second, they've partnered with quite a number of startups of different companies that are outside of OpenAI to bring some pretty interesting features.
すぐに見るように、OpenAIの外のさまざまなスタートアップ企業と提携して、いくつかの非常に興味深い機能を提供しています。
We'll cover that right after this.
これについては、この後で詳しく説明します。
They start by talking about some fine tuning of API features, or rather fine tuning of these models using an API.
彼らはAPI機能の微調整、あるいはAPIを使用してこれらのモデルを微調整することについて話し始めます。
For those that are not familiar, fine tuning is basically if you think of the GPT-4 model as this kind of big blob that can do anything, but maybe it doesn't do everything well, right?
馴染みのない人のために、微調整とは、GPT-4モデルをこのような何でもできる大きな塊と考えると、すべてをうまく行うわけではないかもしれません。
What we can do is let's say we wanted to do exclusively coding or customer service with it.
私たちができることは、たとえば、それをコーディングやカスタマーサービスに専念させたいと思った場合です。
We can fine tune this model to do customer service.
私たちはこのモデルをカスタマーサービスを行うように微調整することができます。
I'm going to say support because that's easier to write.
お手紙を書くのが簡単だから、サポートと言います。
We can take this big huge model and fine tune it to be great at support customer service to answer questions that's specific to our company, our business, training how to answer questions correctly in the style that we want.
この大きなモデルを取り上げ、私たちの会社やビジネスに特有の質問に答えるために、サポートカスタマーサービスを素晴らしいものに微調整することができます。私たちが望むスタイルで質問に正しく答える方法をトレーニングします。
Of course, there's going to be tons of other areas where it's going to get worse because this support model is going to be almost a brand new model, right?
もちろん、このサポートモデルはほとんど全く新しいモデルになるので、他の多くの分野で悪化することが予想されますね。
It's a fine tuned GPT-4.
それは微調整されたGPT-4です。
It's a custom model for support.
それはサポート用のカスタムモデルです。
And chances are it might get a lot worse in various other things, like it might not be that good at coding anymore.
そして、他のさまざまなことでかなり悪化する可能性があります。たとえば、もはやコーディングが得意ではなくなるかもしれません。
It might get bad at poetry, but it gets good at support.
詩が下手になるかもしれませんが、サポートは得意になります。
Again, our sort of like little demonstration here, this little representation here, right?
再び、私たちの小さなデモンストレーション、この小さな表現ですね。
The base layer, right?
基本層、そうですね。
That's let's say GPT-4, right?
それは、GPT-4と言いましょう、そうですね。
We take that, we pull it out and we fine tune it to be a science GPT-4 or a medical GPT-4.
それを取り出して、科学的なGPT-4や医学的なGPT-4に微調整します。
All the stuff that GPT-4 already has comes with it, right?
GPT-4がすでに持っているすべてのものが付属していますね?
It understands the language.
それは言語を理解します。
It can reason, right?
それは推論できますね?
It has certain knowledge.
それは特定の知識を持っています。
It has certain skills.
それは特定のスキルを持っています。
We're just taking it and shaping it into this specific task.
私たちはそれを取って、この特定のタスクに形作っています。
And the other really big thing about fine tuning is you can achieve higher quality results while reducing cost and latency.
そして、微調整のもう1つの大きな利点は、コストとレイテンシーを削減しながらより高品質な結果を得ることができることです。
Since we're pulling out just what we need for our customer support agent, for example, there's tons of things that are still in this big GPT model that we don't need, that we kind of leave behind.
たとえば、カスタマーサポートエージェントに必要なものだけを取り出しているので、この大きなGPTモデルにはまだ必要のない多くのものがあり、それらを置いていくことがあります。
This model could be cheaper to run, much faster, etc.
このモデルは、より安価に運用でき、はるかに高速になる可能性があります。
As an example, they give indeed a global job matching and hiring platform, wanted to send personalized recommendations to job seekers, highlighting relevant jobs based on their skills, experience, etc.
お手本として、彼らは実際にはグローバルな仕事のマッチングと採用プラットフォームを提供し、求職者に個別の推薦を送りたいと考えていました。スキルや経験に基づいて関連する仕事を強調しました。
And they were able to fine tune GPT-3.5 Turbo to generate higher quality and more accurate recommendations.
そして、彼らはGPT-3.5 Turboを微調整して、より高品質で正確な推薦を生成することができました。
They were able to cut their token costs by 80%. So it was able to improve cost and latency, how fast it was doing at cutting the number of tokens, like the worst that were run through the model by 80%. This allowed them to scale from less than one million recommendations to job seekers per month to roughly 20 million.
彼らはトークンコストを80%削減することができました。そのため、トークンの数を削減する速度、つまりモデルを通過する最悪のトークンを80%削減することができました。これにより、求職者への推薦件数が1か月あたり100万未満から約2000万件にスケールアップすることができました。
By the way, as a quick aside, so on this channel, we cover a lot of research from companies like Apple, Microsoft, Meta, OpenAI, Google, DeepMind, etc.
ちなみに、ちょっとした余談ですが、このチャンネルでは、Apple、Microsoft、Meta、OpenAI、Google、DeepMindなどの企業からの多くの研究を取り上げています。
And I'm beginning to notice a pattern here that usually we see the research papers come out let's say three to six months before sort of the things that are talked about in those papers start hitting the market.
そして、ここでパターンを見始めているのですが、通常、研究論文が発表されるのは、その論文で話題になっていることが市場に出始める3〜6か月前だということです。
The Orca 2 paper from Microsoft Research kind of showed that teaching small language models had a reason that this was extremely effective.
Microsoft ResearchのOrca 2論文は、小さな言語モデルを教えることが非常に効果的である理由を示していました。
Orca 2 models match or surpass all other models, including models five to ten times larger.
Orca 2モデルは、他のすべてのモデル、つまり5〜10倍大きなモデルを含むすべてのモデルと同等かそれを上回る性能を発揮します。
Smaller fine tune models built for specific tasks are incredibly effective, incredibly fast, and incredibly cheap.
特定のタスク向けに構築された小さな微調整モデルは、非常に効果的で、非常に高速で、非常に安価です。
We've covered Apple's research into vision models, you could say.
私たちは、Appleのビジョンモデルに関する研究を取り上げました、と言えます。
We covered this a few days ago.
数日前にこれを取り上げました。
They had this model that was 80 million parameters that was in some cases beating GPT-4, getting close in other cases, but 80 million parameters is a tiny, tiny model.
80億のパラメータを持つこのモデルは、場合によってはGPT-4を上回り、他の場合には接近していますが、80億のパラメータは非常に小さなモデルです。
GPT-4 is gargantuan, clocking in at we think 1.7 trillion or you know, that's what people are guessing.
GPT-4は巨大で、1.7兆と推定されているか、それとも、それが人々の推測であると思われます。
It's probably somewhere in the low trillions.
おそらく、数兆の範囲にあるでしょう。
Here's a model that is, if I did my math correctly, this is like a thousand of a percent the size of GPT-4 that is getting close to it for performance for certain specific tasks.
もし私が計算を正しく行ったならば、これはGPT-4のサイズの千分の1程度であり、特定のタスクにおいてそれに近づいています。
Not for everything obviously, but for a specific task, this microscopic model is as good as GPT-4.
明らかにすべてのことには当てはまりませんが、特定のタスクにおいて、この微小なモデルはGPT-4と同じくらい優れています。
If I had to guess, I would guess that we're going to be seeing a lot more things like this, where small fast models, GPT-3.5 turbo and the like, get fine tuned to do pretty incredible things in specific domains for pennies, for very cheap and they'll do it very, very fast.
もし推測しなければならないとしたら、私は、小さくて高速なモデル、GPT-3.5 turboなどが、特定のドメインで非常に信じられないことを行うために微調整され、非常に安価で、非常に速くそれを行うのを見ることになるだろうと思います。
And the people that will be building this for businesses, those people will be printing money.
そして、これをビジネスのために構築する人々、その人々はお金を稼ぐことになるでしょう。
That's my guess.
それが私の推測です。
And OpenAI is building services to make that easier.
そして、OpenAIはそれをより簡単にするためのサービスを構築しています。
They're going to be working with some companies on their own.
彼らはいくつかの企業と協力する予定です。
It's going to be an OpenAI plus, this is a company partnership, but I know a percentage of you listening right now are builders in the space, are developers.
おそらく、これは会社のパートナーシップであるOpenAIプラスになるでしょうが、今聞いている中には、この分野でビルダーや開発者である方の割合がいくつかいると思います。
I mean, these B2B solutions, if you're looking to make money, seem like a very, very lucrative thing to approach.
つまり、これらのB2Bソリューションは、お金を稼ぎたいと考えているのであれば、非常に非常に利益を上げるアプローチのように思えます。
OpenAI is launching new features to give developers even more control over their fine tuning jobs.
OpenAIは、開発者がさらに細かい調整作業を行うための新機能を導入しています。
By the way, I've said this before, but just let me reiterate because I don't think I've said it recently, for the next, let's say five years to a decade, one place where people are going to make a lot of money and one place where we're going to see a lot of progress is this idea of shoving LLMs into everything.
ところで、以前にも言いましたが、最近言っていないと思うので、次の、たとえば5年から10年の間、お金をたくさん稼ぐ場所と、進歩がたくさん見られる場所の1つは、大規模言語モデルをすべてに詰め込むという考え方です。
In other words, for any digital task running, there's probably some solution where you take a custom, fine-tuned Large Language Model, or maybe some custom small model, something like Orca 2, which Microsoft, by the way, was kind enough to open source, so you're able to see exactly how they built it.
つまり、任意のデジタルタスクを実行するために、おそらく、カスタムで微調整された大規模言語モデル、またはおそらくOrca 2のようなカスタムの小さなモデルを取り入れる解決策があるでしょう。ちなみに、Microsoftが親切にもオープンソース化したものを見ることができるので、彼らがそれをどのように構築したかがわかります。
And then apply to something like this.
そして、このようなものに適用してみてください。
Think about how much, indeed, a global job matching firm.
実際、グローバルなジョブマッチング企業がどれだけの金額を使っていたか考えてみてください。
How much were they spending on these customized personalizations?
それらのカスタマイズされた個人用の設定がどれだけ重要か。
How important it is to their business model?
それが彼らのビジネスモデルにとってどれだけ重要か。
It probably has a very high importance and probably had a high cost, right?
おそらく非常に重要で、おそらく高額だったでしょうね?
If you're able to build this custom model that cuts down how much it costs them to do and how fast it's done by 80%, how many millions are you saving them per year?
もしこのカスタムモデルを作成できれば、それによってコストが80%削減され、作業時間も80%短縮されるとしたら、1年間で彼らは何百万ドル節約できるでしょうか?
Certainly, you can probably charge a pretty penny for doing something like that.
もちろん、そういうことをすると高額な料金を請求できるかもしれませんね。
And I would guess that the talent pool for people that know how to do this isn't massive.
そして、このようなことを知っている人材プールは大規模ではないと思います。
Like, it's not, like, I doubt that we have an overwhelming amount of people that are highly skilled in something like this.
まあ、あの、私たちにはこのようなことに高度に熟練した多くの人々がいるとは疑っていません。
And in just a second, I'll show you examples of how this is applied.
そして、すぐに、これがどのように適用されるかの例をお見せします。
But here's the new features that OpenAI is rolling out.
しかし、OpenAIが導入している新機能はこちらです。
It's adding a sort of model checkpoints.
ある種のモデルチェックポイントが追加されています。
During each training epoch to reduce the need for various retraining, etc.
各トレーニングエポック中に、さまざまな再トレーニングの必要性を減らすために。
Kind of like a version control, side-by-side Playground UI for comparing model quality and performance.
モデルの品質とパフォーマンスを比較するためのバージョンコントロール、並行してのPlayground UIのようなものです。
Apparently, somebody made two lems fight each other in Street Fighter 2, so they trained them to output what it should do, fireball, kick, move closer, etc., to see which LLM is the best Street Fighter 2 player.
どうやら、誰かがストリートファイター2で2匹のレムを戦わせたそうで、それらを訓練して何をすべきか出力するようにしました。ファイアボール、キック、近づくなど、どちらの大規模言語モデルが最高のストリートファイター2プレイヤーかを見るためです。
That's kind of what I'm imagining here.
それが私がここで想像していることの種類です。
I doubt it's that cool.
それほどクールではないと思います。
And it also supports integration with third-party platforms, starting with weights and biases.
そして、それは重みとバイアスから始まり、サードパーティープラットフォームとの統合もサポートしています。
That's this platform where you're able to track experiments, evaluate model performance, etc., where you have the model registry, the added OpenAI.
それは実験を追跡し、モデルのパフォーマンスを評価することができるプラットフォームであり、モデルレジストリや追加のOpenAIがある場所です。
OpenAI uses weights and bias models to track all their model versions across 2,000-plus projects, millions of experiments, and hundreds of team members.
OpenAIは、2,000以上のプロジェクト、何百万もの実験、数百のチームメンバーを超えるすべてのモデルバージョンを追跡するために、重みとバイアスモデルを使用しています。
You have visibility into model development process with just a few lines of code.
わずか数行のコードでモデル開発プロセスに対する可視性を持つことができます。
You know, you're able to answer questions like what exact version of the dataset was this model trained on?
このモデルがトレーニングされたデータセットの正確なバージョンは何ですか?といった質問に答えることができます。
I'm going to skip some of these parts.
これらの一部をスキップします。
I will leave a link.
リンクを残します。
If you're really interested in this, if you're in this space, I highly encourage to read this, obviously.
本当に興味がある場合、この分野にいる場合は、この記事を読むことを強くお勧めします。
But for the rest of us, I think it might be more interesting to quickly go over the big points.
しかし、私たちの残りの人々にとっては、大まかなポイントを素早く repaso する方が興味深いと思います。
And then let's dive into exactly what this looks like.
そして、それから、これが具体的にどのように見えるかを掘り下げてみましょう。
What are the actual final outputs of this OpenAI as a base layer thing that's happening?
この OpenAI の基本レイヤーとしての最終的な出力は何ですか?
First of all, they are talking about something called assistant fine-tuning.
まず第一に、彼らはアシスタントの fine-tuning と呼ばれるものについて話しています。
This is where OpenAI helps train models for a specific domain in partnership with a dedicated group of OpenAI researchers.
これは、OpenAI が専用のグループの研究者と協力して、特定のドメインのモデルをトレーニングするのを手伝うところです。
Since then, we've met with dozens of customers to assess their custom model needs and evolve their program to further maximize performance.
その後、私たちは数十の顧客と会って、彼らのカスタムモデルのニーズを評価し、パフォーマンスをさらに最大化するためにプログラムを進化させてきました。
And they've published this.
そして、彼らはこれを公開しています。
We're going to take a look at that next.
次にそれを見てみましょう。
But before we look into those specific examples, here's kind of the end of this blog post where OpenAI is saying, well, here's what's next for model customizations.
しかし、それらの具体的な例を見る前に、ここにこのブログ投稿の終わりがあります。OpenAI は、モデルのカスタマイズについて次に何が来るかを述べています。
They're saying that we believe that in the future, the vast majority of organizations will develop customized models that are personalized to their industry, business or use case.
彼らは、将来、ほとんどの組織が、自分たちの業界、ビジネス、またはユースケースに合わせて個別にカスタマイズされたモデルを開発すると信じています。
I think this is very important, especially if you're if you're in this for the money.
私はこれが非常に重要だと思います、特にお金のために取り組んでいる場合は。
Not everybody listening to this channel is approaching this from kind of a business centric perspective.
このチャンネルを聞いている全員がビジネス中心の視点からアプローチしているわけではありません。
Some of us are just interested in the research and kind of personal use of AI, how can we take advantage of it for work, for our careers.
私たちの中には、研究やAIの個人的な利用に興味を持っている人もいます。仕事やキャリアでどのように活用できるのか。
I understand that this might not apply to everybody, but I still think this is very important to understand because this gives you a glimpse into what's going to happen over the next decade.
私は、これが全ての人に当てはまるわけではないと理解していますが、それでもこれを理解することが非常に重要だと思います。なぜなら、これによって次の10年間に何が起こるかを垣間見ることができるからです。
Here's 0% and here's 100%. This is a line, these are all the tasks that can be either automated or significantly improved or maybe made cheaper by applying AI.
ここが0%で、ここが100%です。これが線で、これらはAIを適用することで自動化されるか、大幅に改善されるか、または安くなる可能性があるすべてのタスクです。
From taking either a biological neural net, like a human being, an employee, or even taking code, like a lot of stuff runs on code, on something that a smart software developer sat down and typed out on their keyboard. And now that code runs to complete a certain task, like in accounting or stocks or, I mean, nowadays pretty much everything, right?
生物学的なニューラルネット、つまり人間や従業員、またはコードを取ることから始めて、多くのものがコードで動いています。賢いソフトウェア開発者がキーボードで入力したものです。そしてそのコードは、会計や株式などの特定のタスクを完了するために実行されます。今日ではほとんどすべてのことですよね?
Not that long ago, close to zero of those business operations ran on neural nets.
それほど昔ではないですが、これらのビジネスオペレーションのほとんどがニューラルネット上で実行されていませんでした。
Neural nets, that's the AI that we're talking about.
ニューラルネット、それが私たちが話しているAIです。
That's ChatGPT, that's Sora, that's all the AI music, all the AI images that you're seeing.
それがChatGPT、それがSora、それが見ているすべてのAI音楽、すべてのAI画像です。
A lot of Google stuff is machine learning how they run their ad serving platform, content recommendations, stuff like that.
Googleの多くのものは機械学習です。広告配信プラットフォーム、コンテンツの推薦など、そのようなものをどのように運営しているか。
But you go back a few years and maybe the number of those things were like 1%, right?
1つ数年前に戻ると、それらのものの数はおそらく1%だったでしょうね。
That could be automated neural nets or improved in neural nets in some way.
それは自動化されたニューラルネットワークか、あるいは何らかの方法で改善されたニューラルネットワークかもしれません。
That was like 1%. Since ChatGPT came out and all that stuff started coming out in the last few years, maybe we're at like, I don't know, 1.5%, 2%, I don't know.
それは1%だったんです。ChatGPTが登場して、ここ数年でそれらのものが出始めてから、おそらく、私たちは、知りませんが、1.5%、2%、わかりません。
This might be even like a horrible overestimation. Now that I think about it, it's probably some fraction of 1%. Over the next decade plus, all of those processes will be replaced by AI, by neural nets.
これは実際にはひどく過大評価かもしれません。考えてみると、おそらく1%の一部です。次の10年以上で、それらのプロセスはすべてAI、ニューラルネットによって置き換えられるでしょう。
Some of them will be a one model to rule them all.
それらの中には、すべてを支配する1つのモデルもあるでしょう。
For example, some people will just use ChatGPT for whatever task they need, right?
たとえば、一部の人々は、必要なタスクに対して単にChatGPTを使用するだけですね。
They'll just use the base layer, kind of like that non-custom, non-fine-tuned version of GPT-4 to ask questions.
彼らは基本層を使うだけで、GPT-4のカスタムされていない、微調整されていないバージョンのようなものを使って質問します。
One interesting thing about Perplexity, for example, that's having incredible success and progress, it's not really doing that much on top of the base layer.
例えば、Perplexityについて興味深いことは、信じられないほどの成功と進歩を遂げているが、実際には基本層の上であまり多くのことをしていないということです。
It's taking all the language models that already exist.
既に存在するすべての言語モデルを取り入れています。
You have your GPT-4 Turbo, Claude3 Sonnet, Claude3 Opus, Mistral Large.
GPT-4 Turbo、Claude 3 Sonnet、Claude 3 Opus、Mistral Largeがあります。
They have a few that you can play around in the Playground with.
お客様がプレイグラウンドで遊べるいくつかのものがあります。
Then you have their own fast ones that they've kind of fine-tuned.
そして、彼ら自身が微調整した高速なものがあります。
But for the pro users, they're just using the base layer, whether that's Claude3 or GPT-4, with heaps of software on top of it.
しかし、プロのユーザーにとっては、Claude 3やGPT-4などの基本レイヤーを使用しており、その上にたくさんのソフトウェアを追加しています。
They're taking GPT-4, they're building a little Perplexity, little search engine on top of it, and they're adding heaps of software to search the internet, pull back answers.
GPT-4を取り、その上に少しPerplexity、少し検索エンジンを構築し、インターネットを検索し、回答を取得するためにたくさんのソフトウェアを追加しています。
Literally in this image, let's say this is GPT-4, this is Perplexity, and the heaps of software is the features of that Perplexity search engine.
このイメージでは、これがGPT-4で、これがPerplexityであり、たくさんのソフトウェアはそのPerplexity検索エンジンの機能です。
And they're valued at billions of dollars.
そして、それらは数十億ドルで評価されています。
Bezos is investing in it, tons of other people are investing in it.
ベゾスが投資しており、他の多くの人々も投資しています。
And rumor is Apple is in talks of potentially buying them.
そして噂によると、Appleは彼らを買収する可能性について話し合っているとのことです。
That's one example where literally billions of dollars are created just with this.
これは、文字通り数十億ドルがこのだけで生み出される1つの例です。
But these Large Language Models or other things like vision models and speech models and image generation models, they're coming for everything.
しかし、これらの大規模言語モデルやビジョンモデル、音声モデル、画像生成モデルなど、すべてに向けて進んでいます。
They're going to be in your thermostat.
おそらくそれらはあなたのサーモスタットに入ることになります。
They're going to be in the food photography, right?
おそらくそれらは食品写真に使われることになりますね?
Some sort of an enhancement.
ある種の向上ですね。
You know, this is a Magnific AI or Magnifique AI, right?
これはマグニフィックAIまたはマニフィックAIですよね?
That upscaler.
そのアップスケーラーですね。
I've featured it on a channel.
私はそれをチャンネルで紹介しました。
Some people use it to upscale their food photography, right?
一部の人々はそれを使って食品写真を拡大するのに使いますね?
Look at that thing.
あのものを見てください。
You can see every little grain of pesto, every pine nut.
ペストのすべての小さな粒子、すべての松の実が見えます。
I'm guessing this is cracked black pepper.
おそらくこれはひび割れた黒胡椒ですね。
I mean, that looks delicious.
それは本当に美味しそうですね。
I mean, take a look at this burger.
このバーガーを見てくださいよ。
Like, they're going to use this in product photography.
例えば、彼らはこれを製品写真で使用する予定です。
The left is sort of the un-upscaled.
左側はアップスケールされていない感じです。
The right is the upscaled.
右側はアップスケールされています。
I mean, most product images will use this in one way or another.
多くの商品画像は、何らかの形でこれを使用することになるでしょう。
We've covered Google Shopping AI with their new thing they're rolling out where you're basically able to use AI to create product visuals, to put them into different environments, to create virtual sort of those models, right?
Google Shopping AIを取り上げましたが、彼らが新しく導入しているもので、AIを使用して製品のビジュアルを作成し、異なる環境に配置し、仮想的なモデルを作成することができるようになりますね。
That kind of showcase your product.
それはあなたの製品をショーケースにするようなものです。
It's able to translate everything into different languages.
すべてを異なる言語に翻訳することができます。
You can basically have an international store selling to the entire world in their own native language.
基本的には、世界中の人々に自国語で販売する国際ストアを持つことができます。
You don't have to do product photography, right?
製品の写真を撮る必要はありませんよね?
You can pull out your phone.
携帯電話を取り出してもいいですよ。
If you have a prototype, take a picture of it and the AI will create 3D images and variations and put them in the hands of models and just do all of that for you.
プロトタイプがあれば、それを撮影してAIが3D画像やバリエーションを作成し、モデルの手に持たせてくれます。
As you'll see in a second, OpenAI is partnering up with people in the legal field to create custom trained models for them, another company that does health insurance, another company that does education, JetBrains for coding, email assistance, Salesforce, health and weight loss, productivity, clinical trials, creativity for farmers to increase their income, personalized fitness and health coaching, content creation.
すぐにご覧いただく通り、OpenAIは法律分野の人々と提携して、彼らのためにカスタムトレーニングされたモデルを作成しています。健康保険を提供する別の会社、教育を提供する別の会社、コーディングのためのJetBrains、メールアシスタント、Salesforce、健康と体重管理、生産性、臨床試験、農家の収入を増やすための創造性、パーソナライズされたフィットネスと健康コーチング、コンテンツ作成。
Like, I can sit here all day and just name use cases, but I think this kind of chart, I hope, represents it a little bit better, right?
ここに座って一日中ユースケースを挙げることができますが、この種のチャートが少しでもそれをよりよく表現していると思います。
We're at close to zero right now of penetration of this technology in the market that's going to change everything.
現在、この技術の市場への浸透はほぼゼロに近い状況ですが、これはすべてを変えるでしょう。
It's going to reduce costs, improve automations, create new use cases that we haven't even thought of, right?
コストを削減し、自動化を改善し、私たちが考えもしなかった新しいユースケースを作り出すでしょう。
Everybody's going to want it.
誰もがそれを欲しがるでしょう。
Everybody's going to need it.
誰もがそれを必要とするでしょう。
And over the next however many years, five, 10, 20, it's going to get rolled out from almost zero to eventually approaching 100.
そして、次の5年、10年、20年の間に、ほぼゼロから徐々に100に近づくまで展開されていくでしょう。
And for every model that gets rolled out, people pay money and this money, it won't be an issue.
モデルが導入されるたびに、人々はお金を支払いますが、このお金は問題にはなりません。
It won't be hard to sell these services because they're going to be saving money.
これらのサービスを売るのは難しくないでしょう、なぜならお金が節約されるからです。
They're going to be making things faster.
物事を速くすることになります。
It's hot.
それはホットです。
It's sexy.
それはセクシーです。
Everybody wants it from your local mom and pop store.
誰もが地元の小売店からそれを欲しがります。
That's local.
それは地元のものです。
That's brick and mortar.
それは実店舗です。
That's selling, I don't know, quilts.
それは、知らない、キルトを売ることです。
I've been doing e-commerce for the last 10 years.
私は過去10年間、eコマースを行ってきました。
I'm telling you, there's like off the top of my head, I can think of a number of places where if I could get a custom trained fine-tuned model to just do that task for me, it would probably be able to do it faster, better, cheaper than I could from inventory management to customer service, to a lot of aspects of marketing.
私は言っているんだ、頭の中で即座に思いつく限り、在庫管理から顧客サービス、マーケティングの多くの側面まで、カスタムトレーニングされたモデルを使えば、私よりも速く、より良く、より安くその仕事をこなせる場所がいくつかあると。
What I'm saying is this may be a good opportunity for those of you who like money.
私が言いたいのは、お金が好きな人にとってこれは良い機会かもしれないということです。
Opening it continues saying with a variety of techniques available to build a custom model, organizations of all sizes, and this is important.
それを開くと、利用可能なさまざまな技術を使ってカスタムモデルを構築するための組織が、すべてのサイズで、これが重要です。
This is kind of like the big thing too of all sizes because with enterprise level software, like software that you sell to hospitals and government organizations, it was hard to build.
これは、すべての規模の組織にとって大きな課題でもあるのです。なぜなら、病院や政府機関に販売するようなエンタープライズレベルのソフトウェアでは、構築が難しかったからです。
You had to have a massive team to even approach projects like that.
そうしたプロジェクトに取り組むには、膨大なチームが必要でした。
There's really no such thing as like artisan software, how you can go to a farmer's market and buy some artisan loaves of bread.
農家の市場に行って、アーティザンのパンを買うように、アーティザンソフトウェアなんて実際には存在しないんです。
That's really delicious.
それは本当においしいですね。
With software, you wanted a certain amount of scale because you had to build that software.
ソフトウェアでは、ある程度のスケールが必要でした。なぜなら、そのソフトウェアを構築する必要があったからです。
The bigger of a customer size that it served, the better the economics of that software would be.
それがサービスする顧客の規模が大きいほど、そのソフトウェアの経済性が向上します。
It's a little bit different here.
ここは少し違います。
We covered this blog post from Semi Analysis a while back.
私たちは以前、Semi Analysisのブログ記事を取り上げました。
Semi Analysis is a website that talks about all the news and events and does analysis of semiconductor and AI industries.
Semi Analysisは、半導体およびAI産業のニュースやイベントについて話し、分析を行うウェブサイトです。
Sort of the hardware, the chips behind AI, all that stuff, NVIDIA and TSMC and all of that.
ハードウェア、AIの背後にあるチップ、NVIDIAやTSMCなどのすべてのものです。
And they dropped this little lie that I thought was fascinating.
そして、私が魅了されたと思った小さな嘘をついていると言っています。
They're saying we're training a C and N. So CNN is a convolutional neural network to accelerate the frequent satellite imaging of data centers to expand our tracking to every data center across every country.
私たちはCとNを訓練していると言っています。つまり、CNNは畳み込みニューラルネットワークであり、データセンターの頻繁な衛星画像の加速を行い、すべての国のすべてのデータセンターへの追跡を拡大しています。
This is kind of like a publisher.
これは出版社のようなものです。
They have a website, a newsletter, they sell their reports, and they have this really specific use case.
彼らはウェブサイトを持ち、ニュースレターを出し、レポートを販売し、非常に具体的なユースケースを持っています。
They have access to satellite images.
おそらく、その情報の多くは公開されていると思います。
I'm sure a lot of that stuff is public.
彼らは衛星画像にアクセスできます。
They track 1,100 data centers and their deployments with publicly available information, including but not limited to property records, power usage.
彼らは、不動産記録や電力使用量を含む公開情報を使用して、1,100のデータセンターとその展開を追跡しています。
This is Freedom of Information Act requests and satellite images.
これは情報公開法の要求と衛星画像です。
They have these very specific use cases where it would help them to have a neural net, a CNN in this case.
彼らには、この場合にニューラルネット、CNNが役立つ非常に具体的なユースケースがあります。
It's not a Large Language Model.
これは大規模言語モデルではありません。
It's not a transformer-based architecture, a convolutional neural network in this case.
これは、トランスフォーマーベースのアーキテクチャでもありません。この場合は畳み込みニューラルネットワークです。
It kind of like labels and is trained on images.
それは画像にラベルを付け、画像で訓練されています。
But they're building their own custom AI model to help them analyze images of these data centers, looking at the satellite images and Figure out what's happening there.
しかし、彼らは自分たちのカスタムAIモデルを構築して、これらのデータセンターの画像を分析し、衛星画像を見てそこで何が起こっているかを把握するのに役立てています。
They might do the same thing for these other things, for property records, power usage, right?
彼らは同じことを、不動産記録や電力使用量についても行うかもしれませんね。
All this stuff.
このすべてのもの。
How many other people in the world might be interested in doing something like this to analyze satellite images for this specific AI accelerator data centers?
世界中でこのようなことに興味を持つ他の人はどれくらいいるでしょうか?この特定のAIアクセラレータデータセンターの衛星画像を分析するために。
Probably not that many.
おそらくそれほど多くはありません。
Maybe a handful.
たぶん数人。
The point being that there's going to be a great demand for these custom small AI models for very specific use cases for organizations of all sizes that can develop personalized models to realize more meaningful, specific impact from their AI implementations.
重要なのは、すべてのサイズの組織が、より意味のある、具体的な影響を実現するために、パーソナライズされたモデルを開発できるように、非常に特定のユースケースのためにこれらのカスタム小規模AIモデルに大きな需要があるということです。
The key is to clearly scope the use case, design and implement evaluation systems, choose the right techniques, and be prepared to iterate over time for the model to reach optimal performance.
鍵は、ユースケースを明確に範囲を定め、評価システムを設計および実装し、適切な技術を選択し、モデルが最適なパフォーマンスに達するまでの時間をかけて反復する準備をすることです。
Again, the people doing this will be making lots of money.
再び、これを行う人々はたくさんのお金を稼ぐことになります。
With OpenAI, most organizations can see meaningful results quickly with the self-serve fine-tuning API for any organization that needs to more deeply fine-tune their models or imbue new domain-specific knowledge into the model.
OpenAIでは、ほとんどの組織が、自己サービスの微調整APIを使用して迅速に意味のある結果を見ることができます。自分のモデルをより深く微調整する必要がある組織や、モデルに新しいドメイン固有の知識を注入する必要がある組織にとって。
Our custom model programs can help.
私たちのカスタムモデルプログラムが役立ちます。
One, this is where they have their own in-house AI people.
第一に、これは彼らが自社内でAIの専門家を持っている場所です。
Two is where OpenAI helps them develop it, probably for bigger companies with specific use cases that can afford to pay for that.
二つは、OpenAIがそれらを開発するのを手伝う場所であり、おそらくそれを支払う余裕のある特定の用途を持つ大企業向けです。
But I think there's another obvious sort of category, and that's people that are outside of a corporation, right?
しかし、もう1つ明らかなカテゴリがあると思いますが、それは企業の外にいる人々ですね?
Like a third party that just goes to businesses and says, hey, I can create this for you and charge them a certain amount of money.
企業に行って、「ねえ、私がこれを作成できますよ」と言って、ある金額を請求する第三者のような存在です。
A consultant or whatever, AI consultant, automation consultant, whatever you want to call that.
コンサルタントまたはAIコンサルタント、自動化コンサルタント、何でも呼んでください。
And again, we're at close to 0% now and it's heading to 100.
そして、再び、今はほぼ0%に近づいており、100に向かっています。
The early movers will probably have a massive advantage.
初期の動き手はおそらく大きな利点を持つでしょう。
But you might be saying, okay, but like, what are some of the use cases that make sense?
しかし、あなたは言っているかもしれませんが、「では、どのようなユースケースが理にかなっているのでしょうか?」
Is all this just hype?
これはすべてただの宣伝なのでしょうか?
Is all this just like marketing hype?
これはただのマーケティングの宣伝なのでしょうか?
People are getting overexcited for nothing.
人々は何もないことに興奮しすぎているのです。
By the way, Demi-Sasabi said an interesting thing, right?
お話変わりますが、デミ・ササビが興味深いことを言いましたね。
Here's AI Breakfast saying, in the words of Demi-Sasabi, the AI landscape is overhyped.
こちらがAI Breakfastが言うところによると、AIの景色は過大評価されています。
Yes, it is overhyped, but underestimated.
はい、過大評価されていますが、過小評価されています。
It's funny how both can be true, but it makes sense.
両方が真実であるのは面白いですが、それは理にかなっています。
There's a lot of hype, but people aren't fully grasping the massiveness of everything that's happening here.
たくさんの興奮がありますが、人々はここで起こっているすべての巨大さを完全に理解していません。
Because what we're talking about is just a tiny sliver of it, like these fine-tuned models for specific business cases.
なぜなら、私たちが話しているのは、特定のビジネスケース向けのこれらの微調整されたモデルのごく一部だからです。
That's just a tiny, tiny part of it.
それはただのごくわずかな部分です。
But let's see what these use cases are.
しかし、これらのユースケースが何であるかを見てみましょう。
These are customer stories from OpenAI.
これらはOpenAIからの顧客のストーリーです。
This is kind of what they're talking about where they work together with companies to create custom fine-tuned models for their specific solutions.
これは、彼らが企業と協力して、特定のソリューションのためにカスタムで微調整されたモデルを作成するという話です。
Here's one that kind of jumped out at me.
これは、私にとって目を引くものです。
This is Super Human.
これはスーパーヒューマンです。
That's the company name.
それが会社名です。
And they're introducing a new era of email with OpenAI.
そして彼らはOpenAIと共に新しいメールの時代を紹介しています。
They're building a suite of next generation AI email products that are saving users time, driving value, and increasing engagement.
彼らは、ユーザーの時間を節約し、価値を生み出し、エンゲージメントを高める次世代のAIメール製品スイートを構築しています。
We've all struggled with the overflowing inbox, right?
私たちはみんな、溢れかえる受信トレイに苦労してきたでしょう?
There's too many things, too many appointments, too many meeting minutes to keep up on.
やるべきことが多すぎて、約束が多すぎて、会議の議事録が多すぎて、追いつくのが大変です。
Super Human is reimagining how that could be using AI to help you write emails, to rewrite certain ideas in your voice.
スーパーヒューマンは、AIを使ってメールの書き方を手伝ったり、あなたの声で特定のアイデアを書き直したりすることで、それがどのように再構築されるかを想像しています。
Or for example, just say it out loud, kind of dictate it and have the AI kind of fill the gaps, write it in your voice, make sure everything's correct.
お手本を読み上げるか、音声で言ってみるだけでもいいです。AIが空白を埋めてくれます。自分の声で書いて、すべてが正しいことを確認してください。
And then send it.
そしてそれを送信します。
I'll summarize a quick summary of each email that's arriving.
私は到着した各メールの簡単な要約をします。
Instant reply allows you to reply from certain contextual options, similar to how a text message, you have those little options to reply.
インスタントリプライ機能を使用すると、特定の文脈オプションから返信できます。テキストメッセージのように、返信するための小さなオプションがあるような感じです。
It's important to understand that yes, so GPT, ChatGPT can do a lot of this, but this we're talking about custom solutions.
これは重要ですが、GPT、ChatGPTはこれらの多くを行うことができますが、ここではカスタムソリューションについて話しています。
That's somebody sitting there and specifically trying to make this as good as possible for the specific use cases.
それは誰かが座って、特定のユースケースにできるだけ良くするように努めているということです。
But okay, so maybe this isn't as sexy as I made it sound out to be, but here's what caught my attention.
でも、まあ、私が言ったように魅力的ではないかもしれませんが、私の注意を引いたのはこちらです。
They're saying here, okay, so what's next?
ここで言っているのは、では次は何かということですか?
Super Humanist Company imagines a world where GPT-based agents will soon be able to filter, triage, and respond to email automatically, scheduling meetings and appointments, and taking basic actions online and in the real world.
Super Humanist Companyは、GPTベースのエージェントがメールを自動的にフィルタリング、トリアージ、返信し、会議や予約をスケジュールし、オンラインや現実世界で基本的なアクションを取ることができるようになる世界を想像しています。
Again, I mean, they're talking about building an email agent or a scheduling agent.
再び、つまり、彼らはメールエージェントやスケジューリングエージェントを構築することについて話しています。
Kind of like an executive assistant for you that has access to your emails, to your calendar, and you can kind of like manage and stay on top of it based on what you tell it to do.
あなたのメールやカレンダーにアクセスできる、あなたのためのエグゼクティブアシスタントのようなもので、あなたが指示したことに基づいて管理し、最新の状況を把握することができます。
The idea of opening up an email inbox and then reading the first email and then the second email, the third email, or only doing triage kind of thinking, okay, like what can I get away with not answering today?
メールの受信トレイを開いて、最初のメール、2番目のメール、3番目のメールを読む、またはトリアージを行うという考え方は、今日は何に返信しなくてもいいかを考えるようなものです。
Let me see what's the most important things.
最も重要なことを見てみましょう。
Like the idea of you doing that yourself with your brain, your eyes, and you clicking the mouse or whatever.
あなた自身がその作業を脳と目とマウスを使って行うという考え方は、どうでしょうか。
Like in five years, that's going to be laughable.
5年後には、それは笑い話になるでしょう。
That's going to be on par with manually adjusting the heat in your house every 10 minutes instead of using a thermostat.
それは、家の暖房を10分ごとに手動で調整することと同じくらい古臭くなるでしょう。
How many people have that now?
今、それを使っている人はどれくらいいますか?
Close to zero, right?
ほとんどゼロですよね。
Maybe some fraction of 1% of the people in the world are using that in their inbox, right?
世界中の人々のうち、そのようなものを使っているのは1%未満ですよね。
It's not 1%. It's less for sure.
1%ではありません。確実にそれよりも少ないです。
If you look at the entire world, even the United States, it's less.
世界全体を見ると、アメリカでさえも、それは少ないです。
In five years, it'll be 100% or close, right?
5年後には、100%に近いか、そうでしょう?
Somewhere getting closer to 100%. Next, we have Harvey.
どこかで100%に近づいています。次に、ハービーがいます。
It's a custom trained model for legal professionals.
それは法律関係者向けのカスタムトレーニングされたモデルです。
Of course, lawyers and technology, the combination of the two is hilarious, right?
もちろん、弁護士とテクノロジーの組み合わせは、おかしいですよね?
The I am not a cat, your honor video is perhaps the funniest video I've ever seen in my life.
「私は猫ではありません、裁判官さま」というビデオは、おそらく私が人生で見た中で最も面白いビデオです。
I think the first time I heard myself laughing, I'm not even exaggerating.
私が自分の笑い声を初めて聞いたとき、本当に大げさではありません。
Then of course, you have the lawyers that use Chagmit to, I don't know what they were trying to do, but it basically made up a bunch of court cases to cite their arguments, and it got them into a lot of trouble.
もちろん、Chagmitを使う弁護士もいますが、彼らが何をしようとしていたのかはわかりませんが、基本的には彼らの主張を引用するために裁判例を捏造し、それが多くのトラブルを引き起こしました。
At the time, a lot of the clueless reporters that cover AI wrote articles saying their conclusion was AI bad.
当時、AIを取り上げる無知な記者たちが、彼らの結論はAIが悪いと書いた記事を書きました。
The reality was they shouldn't have used GPT-4 kind of that base model, right?
実際のところ、彼らはGPT-4という基本モデルを使うべきではなかったのですね。
They needed something that was custom trained, that had some architecture, that had some rag, that retrieval augmented generation, right?
お客様が必要としていたのは、カスタムトレーニングされたもので、ある程度のアーキテクチャを持ち、いくつかの参照を持ち、検索を補完する生成を持っているものでしたね?
As you can see here, this is the model that was trained.
こちらでご覧の通り、これがトレーニングされたモデルです。
It's custom trained.
それはカスタムトレーニングされています。
And as you can see here, it's giving you little citations of the case law.
そしてこちらでご覧の通り、それは判例の少しの引用を提供しています。
For everything that it writes, it supports it by giving you a little, okay, so I'm referencing this specific case law, right?
書いたすべてのことについて、少しの引用をして、この特定の判例を参照していることをサポートしていますね。
Stone versus writer or whatever, right?
ストーン対ライターとか、そういう感じですね?
To support its claims.
その主張をサポートするために。
It's not guessing, it's not hallucinating, it's not taking the best shot at it.
それは推測しているわけではなく、幻覚を見ているわけでもなく、最善の方法を取っているわけでもありません。
It's using actual databases to support those claims.
それは実際のデータベースを使用して、それらの主張をサポートしています。
It's custom trained not to take a stab at it or whatever.
それは、それを試みることなくカスタムトレーニングされています。
It's custom trained to answer just as accurately as possible.
それはできるだけ正確に答えるようにカスタムトレーニングされています。
That company that's doing it, they're called Harvey, and they've worked with 10 of the largest law firms to test this model.
それを行っている会社はハービーと呼ばれ、このモデルをテストするために最大の10つの法律事務所と協力しています。
And they were surprised by how strong the reaction was from those law firms.
そして、それらの法律事務所からの反応の強さに驚かれました。
97% of the time, the lawyers prefer the output from the case law model from this custom trained model, because it was longer, more complete answer.
97%の時間、弁護士たちはこのカスタムトレーニングされたモデルからの出力を好みました。それはより長く、より完全な回答でした。
It went into the nuance of what the question was asking and covered more relevant case law.
質問が何を尋ねているかのニュアンスに入り、より関連性のある判例をカバーしました。
Hallucination reduction was one of Harvey's motivations for building a custom model.
幻覚の軽減は、ハービーがカスタムモデルを構築する動機の1つでした。
Again, the problem wasn't quote unquote AI.
再び、問題は「AI」という言葉ではありませんでした。
The problem was it just didn't have the correct architecture needed to provide the correct answers and the people using it didn't have the correct training to understand what it was good at, where it could fail.
問題は、正しい答えを提供するために必要な正しいアーキテクチャを持っていなかったこと、それを使用している人々がそれが得意で、どこで失敗する可能性があるかを理解するための正しいトレーニングを受けていなかったことでした。
Again, that's another part of this whole thing is training the staff, the people using it to kind of understand its limitations, etc.
この全体の一部として、スタッフやそれを使用する人々にトレーニングを行い、その限界などを理解するようにすることも重要です。
Having ChadGPT represent you in a legal case is shockingly not a good idea.
ChadGPTに法的な問題を代表させるのは、驚くほど良い考えではありません。
Where are they seeing this whole thing going next?
彼らはこの全体の次にどこに進むと考えているのでしょうか?
Well, they're saying this, don't build for the current capabilities of these models today.
今日のこれらのモデルの現在の能力に合わせて構築しないでください、と彼らは言っています。
Because remember, when you do that, what happens?
なぜなら、そうすると何が起こるか覚えているでしょうか?
OpenAI comes in, it's like, I am deaf.
OpenAIが登場し、私は聾者だと言います。
And it just like reaps everybody, right?
そして、それはまるで誰もが収穫されるようですね。
Build for where the models are going to be.
モデルがどこに向かっているかに合わせて構築してください。
Tackle more complex versions of problems so that when better versions of the model come out, they aren't solved as a side effect.
より複雑な問題のバージョンに取り組んで、より良いモデルのバージョンが出ても、それが副作用として解決されないようにしてください。
And what's Harvey working on next?
そして、ハービーは次に何に取り組んでいますか?
Let's see.
さて、どうしましょうか。
One of their main focuses is agents.
彼らの主な焦点の1つはエージェントです。
Again, surprise, surprise.
また、驚きですが。
AI autonomous agents are once again, the next frontier.
AI自律エージェントは再び、次のフロンティアです。
Here's yet another company that's like, well, that's the very next step.
ここには、まさにその次のステップとなる会社がさらにあります。
It's in this case, how to combine multiple model calls together in a single working output.
この場合は、複数のモデル呼び出しを1つの作業出力に組み合わせる方法です。
This would simplify the user experience and reduce the amount of prompt engineering and typing users needed to do.
これにより、ユーザーエクスペリエンスが簡素化され、ユーザーが行う必要があるプロンプトエンジニアリングやタイピングの量が減少します。
Another one is Oscar, bringing AI to health insurance.
もう1つは、AIを健康保険にもたらすOscarです。
The OpenAI models together with Oscar help ensure HIPAA compliance, doubling productivity, automated documentation, and claims processing.
OpenAIモデルとOscarを組み合わせることで、HIPAAコンプライアンスを確保し、生産性を2倍にし、自動化された文書作成や請求処理を行います。
Medical care workers cut their time spent documenting various medical care conversations, stuff like that, by 40%. So that's your doctor, that's your nurse, almost half of the time, instead of thinking like what your needs are, they're sitting there filling out this paperwork, draining their mental energy, their physical energy.
医療従事者は、さまざまな医療ケアの会話を文書化する時間を40%削減しました。つまり、あなたの医師や看護師は、あなたのニーズを考える代わりに、その書類作業に座って、精神的エネルギーや身体的エネルギーを消耗しているのです。
Think about how many millions of dollars we spend having them do that instead of something like a Large Language Models taking care of it for pennies.
何百万ドルもかけて彼らにそれをさせる代わりに、数ペニーでそれを処理するような大規模言語モデルを使うことを考えてみてください。
This reduces burnout and allows nurses and clinicians to focus on higher order tasks.
これにより、職業倦怠感が軽減され、看護師や臨床医がより高度な業務に集中することができます。
How many lawyers and doctors and nurses use various tools like this to help them in their work right now?
現在、弁護士や医師、看護師がこのような様々なツールを使って仕事を手伝っているのは何人くらいですか?
Probably close to zero, right?
おそらく、ほとんどゼロですよね?
We're definitely not near 1%, right?
私たちは間違いなく1%にも満たないですよね?
It's some tiny fraction of 1%. How many of them will be using it in five to 10 years?
1%のごくわずかな割合です。5年から10年後にはそれを利用する人はどれくらいいるでしょうか?
Probably a lot closer to 100%, right?
おそらく、100%にかなり近いでしょうね?
Next, they're also talking about claims, improving accuracy, automating 40,000 tickets by the end of the year.
次に、彼らはクレームについても話しており、年末までに4万枚のチケットを自動化して精度を向上させると言っています。
All right, so this is what a claim looks like, right?
では、これがクレームの見た目ですね?
You click this little magic button, help me with this inquiry, and it fills it out, I assume.
この小さな魔法のボタンをクリックして、この問い合わせを手伝ってもらい、それを記入してくれると思います。
Next, they're saying creating an AI flywheel.
次に、彼らはAIフライホイールを作成すると言っています。
A flywheel in business usually refers to kind of like a virtual cycle where something almost kind of like builds on itself to keep improving that business or that habit or whatever.
ビジネスにおけるフライホイールとは、ほとんど自己を築いてビジネスや習慣を改善し続ける仮想サイクルのようなものを指します。
The more users a social network has, the more value there is for existing users and the more users it will attract.
ソーシャルネットワークのユーザーが多ければ多いほど、既存のユーザーにとっての価値が高まり、さらに多くのユーザーを引き寄せることになります。
This is kind of like a direct network effect.
これは、直接的なネットワーク効果の一種です。
They're saying we don't want to just nibble around the edges of administrative use case simplification, right?
彼らは、管理上のユースケースの簡素化の周辺をちょっとずついじるだけではなく、と言っていますね?
Because this is what we're talking about, right?
なぜなら、これが私たちが話していることなのですよね?
It's just simplifying administrative use cases, right?
ただ、管理上のユースケースを簡素化するだけですよね?
Important thing, very valuable thing, will save a lot of money, will save the mental energies of doctors and nurses that need to focus on their patients.
重要なこと、非常に価値のあること、多くのお金を節約し、患者に焦点を合わせる必要がある医師や看護師の精神エネルギーを節約することになります。
It's important, it's critical, but they're saying that's just the beginning.
それは重要であり、重要ですが、彼らはそれが始まりに過ぎないと言っています。
They're looking to bring down the cost of seeing physicians and being in the hospital by a factor of 10 in the next three to five years.
彼らは、次の3〜5年で医師を診察し、入院するコストを10分の1に引き下げることを目指しています。
Our customer was Zelma, who's using GPT-4 to make education data accessible.
お客様は、教育データを利用するためにGPT-4を使用しているZelmaでした。
Our customer success story is Healthify, an app that helps with weight loss.
お客様の成功事例は、体重減少を支援するアプリであるHealthifyです。
This is for a specific population.
これは特定の人口を対象としています。
It looks like they're really focusing on India, it seems, and specifically classifying traditional Indian foods.
彼らはインドに本当に焦点を当てているようですし、具体的には伝統的なインド料理を分類しているようです。
Focusing on certain custom populations can be a really good idea.
特定のカスタム人口に焦点を当てることは、本当に良いアイデアかもしれません。
Here they're saying SNAP achieved around 80% accuracy for single Indian foods, right?
ここでは、SNAPが単一のインド料理について約80%の精度を達成したと言っていますね。
Here's where it gets a little bit interesting if you're looking at it from a business perspective.
ここが少し面白くなるところです、もしビジネスの観点から見ているならば。
Like how important is it for businesses to have something like this?
ビジネスがこのようなものを持つことがどれほど重要か、ということですか?
Well, increased engagement.
まあ、エンゲージメントの増加です。
Because of the fine-tuned models, the AI, the engagement increases.
微調整されたモデルやAIのおかげで、エンゲージメントが増加します。
People are more interested, people tend to use it more.
人々はより興味を持ち、それをより多く使用する傾向があります。
Users track 50% more often with these new models.
これらの新しいモデルでは、ユーザーは50%頻繁にトラッキングします。
Users engage more for nutrition and fitness coaching.
ユーザーは栄養やフィットネスのコーチングにより多く参加します。
Clients engage with AI-supported coaches 18% more.
クライアントはAIサポートのコーチと18%多く関わります。
And, and this is kind of interesting, with users permission, the agents will even be able to order food or book gym classes.
そして、これは興味深いことですが、ユーザーの許可を得て、エージェントは食事を注文したり、ジムのクラスを予約したりすることさえできるようになります。
A brand new industry that's going to emerge that we haven't yet seen, but a few people are beginning to mention it in the future when we have agents that control our lives.
まだ見たことのない新しい産業が登場するでしょうが、数人が将来、私たちの生活をコントロールするエージェントが現れると言及し始めています。
That sounds harsh.
それは厳しいようですね。
That help us run our lives.
私たちの生活を運営するのに役立ちます。
They're kind of like our assistants, but they do connect us to information, to services, to everything.
彼らは私たちのアシスタントのようなものですが、情報やサービス、すべてにつながります。
They're going to recommend certain products, right?
彼らは特定の製品を推奨する予定ですね?
They're going to order food for us, book gym classes, etc.
お客様のために食事を注文したり、ジムのクラスを予約したりする予定です。
A lot of marketing dollars are spent on marketing to the person, right?
多額のマーケティング費が個人に向けて使われているんですよね?
Billboards, ads, it's focused on you, right?
広告看板など、それはあなたに焦点を当てているんですよね?
It's focused on trying to get you, the human, to buy something.
人間であるあなたに何かを購入させようとしているんです。
Slowly over time, we're going to see more and more money going into marketing to AIs, to these agents.
時間の経過とともに、ますます多くのお金がAI、これらのエージェントに向けてマーケティングに投入されることになるでしょう。
What is that going to mean?
それはどういう意味を持つのでしょうか?
Well, I mean, the simplest way is spending ad dollars to get the company behind these agents to recommend your products over the competitors.
まあ、最も単純な方法は、広告費を使って、これらのエージェントの背後にいる企業に、競合他社の製品よりもあなたの製品を推奨させることです。
That's the most obvious one, but there might be things like with search engine optimization, right?
それが最も明らかなものですが、検索エンジン最適化のようなものがあるかもしれませんね?
It's how you optimize your webpage, the links that are pointing to your webpage, that determines how high you appear in Google Search.
あなたのウェブページを最適化する方法、ウェブページにリンクしているリンクが、Google検索でどれだけ高く表示されるかを決定します。
Could there be an SEO, but for autonomous AI agents and AO, if you will, autonomous AI agents optimization, it'll be interesting to see how that unfolds.
SEOがあるかもしれませんが、自律型AIエージェントやAO向けに、自律型AIエージェントの最適化があるかもしれません。それがどのように展開されるか興味深いですね。
But you know, companies will be spending money trying to Figure out how to get autonomous agents to recommend them over their competition.
しかし、企業はお互いの競合他社よりも自律エージェントに自分たちを推薦させる方法を見つけるためにお金を使うことになるでしょう。
Like that's going to happen.
そんなことが起こるわけないでしょう。
100% chance of that.
その確率は100%です。
And gen AI isn't just for us soft first-worlders only.
そして、汎用AIは私たちのようなソフトな第一世界の人々だけのためではありません。
Digital Green uses OpenAI to increase farmer income in countries including India and Kenya.
デジタル・グリーンは、インドやケニアを含む国々で農民の収入を増やすためにOpenAIを使用しています。
It's important for these farmers to be able to teach each other best practice for growing new crops, share various local weather conditions.
これらの農民が新しい作物の栽培のベストプラクティスを教え合い、さまざまな地元の気象条件を共有できることが重要です。
And there's a lot of problems with that, right?
それにはたくさんの問題がありますね。
Not just connecting them, but also having a lot of different languages that are being spoken, right?
彼らをつなげるだけでなく、さまざまな言語が話されていることもありますね。
They need to connect with their local suppliers and provide market and pricing information.
彼らは地元の仕入先とつながり、市場や価格情報を提供する必要があります。
It's like Eve online, but for farmers, farmer to farmer training videos, increase farmer income by an average of 24%. This was interesting.
それは農民向けのEve onlineのようなもので、農民同士のトレーニングビデオを通じて平均24%の収入増加をもたらします。これは興味深いものでした。
We, I mentioned this in yesterday's video.
昨日のビデオでこれに触れました。
There's some things that governments and various well-funded government like entities can do that may not be necessarily something that large corporations are willing to do where they can use their resources to build something that's useful for the whole country, right?
政府やさまざまな資金力のある政府機関ができることは、大企業がやろうとしないかもしれないことがあります。国全体に役立つものを構築するためにリソースを活用できるという点で、そうですね。
For example, a database for all the countries and that cultural AI to be trained on, right?
たとえば、すべての国のデータベースとその文化的なAIをトレーニングすることができるということですね。
Microsoft probably won't do that.
Microsoftはおそらくそれを行わないでしょう。
Google won't do that, but maybe something like DARPA might consider it.
Googleはそうはしないでしょうが、DARPAのような組織がそれを検討する可能性はあります。
I don't know if that's exactly in their wheelhouse or not, but certainly it's something that would help everybody in that country that's in AI to be able to do something like this here.
それが彼らの得意分野に正確に含まれているかどうかはわかりませんが、確かにAI分野の人々がこうしたことをできるようになるのは誰もが助けられることです。
India's Ministry of Agriculture validates all documents in the knowledge base to ensure accuracy and reliability.
インドの農業省は、知識ベース内のすべての文書を検証して正確性と信頼性を確認しています。
And then with GPT-4, they're using RAG, Retrieval Augmented Generation, to pull the needed stuff from there, various crop research, fact sheets, etc.
そして、GPT-4では、RAG、Retrieval Augmented Generationを使用して、そこから必要な情報、さまざまな作物研究、事実シートなどを引き出しています。
And the cost of this farmer chat, these extension services, they went from $35 per farmer to 35 cents per farmer.
そして、この農家向けチャット、これらのエクステンションサービスのコストは、農家1人あたり35ドルから35セントに下がりました。
Again, massive, massive leap.
再び、膨大な進歩です。
And again, right now this technology is available to close to 0% of the world's population of farmers with those needs, right?
そして、今この技術は、そのようなニーズを持つ世界の農家のほぼ0%に利用可能ですね。
And over the next decade, hopefully it will start approaching 100%. And there's like 20 of these little customer profiles.
そして、次の10年で、それが100%に近づくことを願っています。そして、これらの小さな顧客プロファイルが20あります。
Each one has its own mind-blowing application.
それぞれが驚くべきアプリケーションを持っています。
Like there's not one use case here that isn't kind of revolutionary in its own way, where it provides more features, more use cases, it drops the price of doing something.
ここには革命的なものでないユースケースはなく、それぞれが独自の方法でより多くの機能、より多くのユースケースを提供し、何かを行うコストを下げます。
It frees up the humans, the experts, to focus on what they're supposed to be doing instead of being bogged down in the details.
専門家たちが、細かい詳細に縛られることなく、本来やるべきことに集中できるようになります。
Where this medical group believed they would need specialized medical models to get good results.
この医療グループは、良い結果を得るために専門的な医療モデルが必要だと信じていました。
And they were shocked to find that GPT-4 outperformed a team of highly trained human experts.
そして、彼らはGPT-4が高度に訓練された人間の専門家チームを上回る結果を出したことに驚いた。
They said it, not I. They were shocked.
私が言ったのではなく、彼らが言ったのです。彼らは驚いていました。
I'm sensing that the title of this video is going to have the word shocking in it.
この動画のタイトルに「驚くべき」という言葉が入ると感じています。
What do you think?
あなたはどう思いますか?
Am I correct?
私の直感は正しいでしょうか?
Is my intuition accurate?
私の直感は正確でしょうか?
I'll leave this page down below.
このページは下にリンクを残しておきます。
This is the OpenAI.com.
これはOpenAI.comです。
This is not their blog, which I think a lot of people are familiar with.
これは彼らのブログではなく、多くの人が馴染みがあると思います。
This is/customer -stories.
これは、顧客の物語です。
And I think it just got populated with all of this stuff.
そして、私はこれらすべてのもので満たされたと思います。
There's a whole bunch of them here.
ここにはたくさんのものがあります。
And most of them aren't like fluffy.
そして、ほとんどのものはフワフワしていません。
They're not just whatever nonsense.
それらはただの無駄なものではありません。
They're impactful.
それらは影響力があります。
And they showcase how powerful these custom trained models can be.
そして、これらのカスタムトレーニングされたモデルがどれほど強力かを示しています。
I'll leave it off there.
ここで終わりにします。
I think I've made my point.
私は自分のポイントを述べたと思います。
Back when software was rolling out, that created a lot of wealth, a lot of change in the world.
ソフトウェアが展開されていた頃、それは世界中で多くの富を生み出し、多くの変化をもたらしました。
Software was eating the world, they said.
彼らは「ソフトウェアが世界を飲み込んでいる」と言いました。
This is the next step where AI is doing that thing.
これはAIがその役割を果たしている次の段階です。
We probably shouldn't say AI is eating the world.
AIが世界を飲み込んでいるとは言わない方が良いかもしれません。
We should say AI is helping the world.
AIが世界を助けていると言うべきです。
I think that's the A16Z motto.
私はそれがA16Zのモットーだと思います。
AI is going to save the world.
AIが世界を救うでしょう。
And I agree for a lot of people that are scared of AI, I don't know, becoming the Terminator and turning us into paper clips or whatever that scenario is.
そして、AIに恐れを抱く多くの人々に同意します。ターミネーターになって私たちをペーパークリップに変えるなどのシナリオがあるかどうかはわかりません。
I hope they take a look at this as the ability to make our lives easier to eliminate busy work, to help people understand various medical procedures better, to deal with email, to understand legal cases better, to make education data more accessible, so more people understand what decisions are being made in our school systems.
私は、これを私たちの生活をより簡単にする能力として見てほしいと思います。忙しい仕事を排除し、さまざまな医療手続きをよりよく理解するのを助け、メールの取り扱い、法的事案をよりよく理解するのを助け、教育データをよりアクセスしやすくし、より多くの人々が学校システムで行われている決定を理解できるようにします。
Help people in farming communities that are struggling to a little bit better.
お手伝いするのは、少しでも農村コミュニティで苦労している人々です。
This is where AI is right now.
今、AIはここにあります。
This is what it can do right now.
今、それができることはこれです。
And over the next five years, ten years, it's going to be rolling out from zero to 100% of whatever use cases we can apply it to.
そして、次の5年、10年で、私たちがそれを適用できるあらゆる用途に0%から100%まで展開されるでしょう。
The world will change.
世界は変わるでしょう。
Some people will make a lot of money.
一部の人々はたくさんのお金を稼ぐでしょう。
And it's going to be a heck of a wild ride.
そして、それはかなりの荒々しい乗り物になるでしょう。
I don't know about you, but I'm pretty excited about what's coming.
私はあなたについてはわかりませんが、私は今起こることにかなり興奮しています。
I'm excited about the empowerment that AI is going to bring to education, to business, to creating music, to creating movies with things like SunoAI and Sora.
私はAIが教育、ビジネス、音楽の創造、SunoAIやSoraなどの映画の創造にもたらす力に興奮しています。
It's going to empower people to do more.
それは人々により多くのことをする力を与えるでしょう。
And we'll have some bumps in the road, I'm sure.
そして、私たちは道のりにいくつかの障害があるでしょう。
But wherever you are in life, I would say, get excited.
どんな状況にいるとしても、興奮してください。
If you're in a position to become this AI automation consultant and help people incorporate neural networks into various parts of their business to create these fine-tuned custom models for them, I think that's going to be a millionaire making industry, probably for some people.
もしあなたがこのAI自動化コンサルタントになる立場にいて、人々がビジネスのさまざまな部分にニューラルネットワークを取り入れて、彼らのためにこれらの微調整されたカスタムモデルを作成するのを手助けすることができるなら、それはおそらく何人かにとっては百万長者になる産業になると思います。
If you're running a business or want to think about where things like that could help you in the business, where they could help you do more or do more with less.
もしあなたがビジネスを運営しているか、ビジネスでどこでそのようなものが役立つか考えたいのであれば、それがあなたがより多くを成し遂げたり、より少ない労力でより多くを成し遂げるのを手助けするかもしれない場所を考えてみてください。
And if you're wondering why I keep showing you this wolf from Puss in Boots, I honestly don't know.
そして、なぜ私が「長靴をはいた猫」のオオカミを何度も見せているのか疑問に思っているなら、正直言ってわかりません。
It's just a great character.
それはただ素晴らしいキャラクターなんです。
He just cracks me up.
彼は私を笑わせるんです。
This is the guy that plays them, by the way, if you weren't aware.
ちなみに、これが彼を演じている人物です。もし気づいていなかったら。
Anyways, my name is Wes Roth and thank you for watching.
とにかく、私の名前はウェス・ロスです。ご視聴ありがとうございました。
この記事が気に入ったらサポートをしてみませんか?