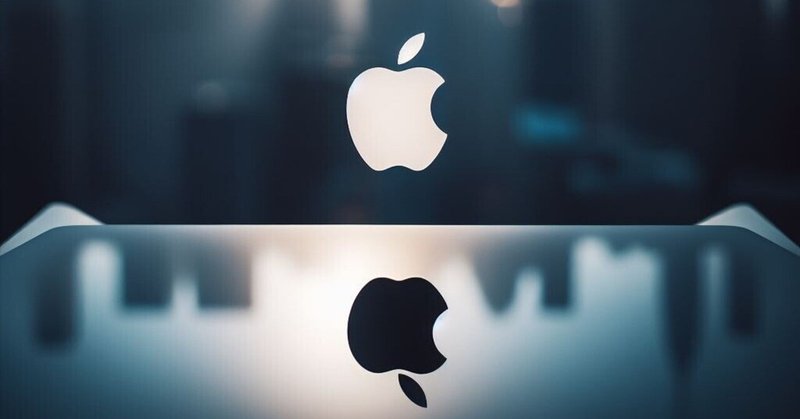
Appleが、LLM in a flashを発表した。
Appleが、限られたメモリ容量における効率的な大規模言語モデルの推論に関する論文をarxivにて発表しました。
本論文は、大規模言語モデル (LLM) が抱えるメモリ不足問題を解決する方法を提案しています。提案手法は、モデルパラメータをフラッシュメモリに格納し、必要に応じて DRAM に読み込むことで、制限された DRAM 容量でも効率的に LLM を実行することを可能にします。
モバイルデバイス等のDRAM 容量が限られたデバイスにおいて、効果的なアプローチとなることが期待されます。
以下は、当該論文のアブストラクトです。
Large language models (LLMs) are central to modern natural language processing, delivering exceptional performance in various tasks. However, their intensive computational and memory requirements present challenges, especially for devices with limited DRAM capacity. This paper tackles the challenge of efficiently running LLMs that exceed the available DRAM capacity by storing the model parameters on flash memory but bringing them on demand to DRAM. Our method involves constructing an inference cost model that harmonizes with the flash memory behavior, guiding us to optimize in two critical areas: reducing the volume of data transferred from flash and reading data in larger, more contiguous chunks. Within this flash memory-informed framework, we introduce two principal techniques. First, “windowing” strategically reduces data transfer by reusing previously activated neurons, and second, “row-column bundling”, tailored to the sequential data access strengths of flash memory, increases the size of data chunks read from flash memory. These methods collectively enable running models up to twice the size of the available DRAM, with a 4-5x and 20-25x increase in inference speed compared to naive loading approaches in CPU and GPU, respectively. Our integration of sparsity awareness, context-adaptive loading, and a hardware-oriented design paves the way for effective inference of LLMs on devices with limited memory.
この記事が気に入ったらサポートをしてみませんか?