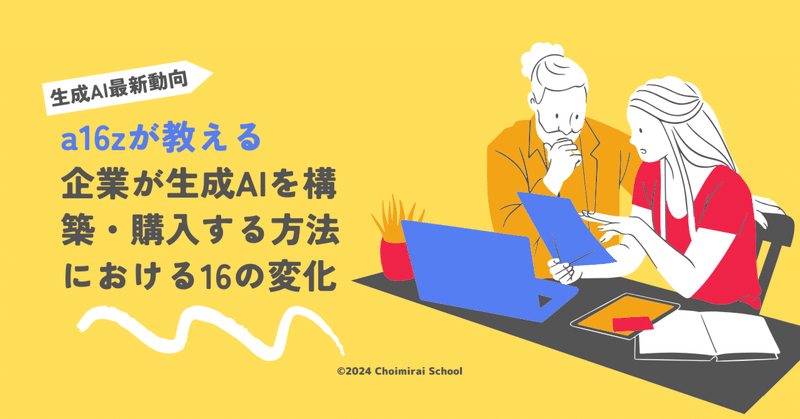
【生成AI最新動向】企業が生成AIを構築・購入する方法における16の変化
こんにちは、Choimirai Schoolのサンミンです。
AI関連企業に対する投資家として圧倒的存在感を示しているのが「a16z」。これは、彼らがAI分野の最新の進歩をつねに追い求め、最先端のテクノロジー企業への資金調達を支援しているからです。そのa16zが、2024年3月21日に「16 Changes to the Way Enterprises Are Building and Buying Generative AI」というタイトルで企業が生成AIをどのように使用・購入し、予算化しているのかを公開。内容がとても参考になったのでClaude 3 Opusに訳してもらいました。まだの方はぜひ参考にしてください。
Generative AI took the consumer landscape by storm in 2023, reaching over a billion dollars of consumer spend1 in record time. In 2024, we believe the revenue opportunity will be multiples larger in the enterprise.
2023年、生成AIは消費者の間で大きな話題となり、記録的な速さで10億ドル以上の消費者支出に達しました。2024年には、エンタープライズ向けの収益機会はさらに大きくなると私たちは考えています。
Last year, while consumers spent hours chatting with new AI companions or making images and videos with diffusion models, most enterprise engagement with genAI seemed limited to a handful of obvious use cases and shipping “GPT-wrapper” products as new SKUs. Some naysayers doubted that genAI could scale into the enterprise at all. Aren’t we stuck with the same 3 use cases? Can these startups actually make any money? Isn’t this all hype?
昨年、消費者が新しいAIとのチャットに時間を費やしたり、ディフュージョンモデルで画像や動画を作成したりしている間、エンタープライズにおける生成AIの取り組みは、ごく一部の明らかなユースケースと、新しいSKUとして提供される「GPTラッパー」製品に限定されているように見えました。生成AIがエンタープライズに拡大できるのかどうか疑問視する人もいました。同じ3つのユースケースに縛られてしまうのでは?これらのスタートアップは本当に収益を上げることができるのか?これは全てハイプなのでは?
Over the past couple months, we’ve spoken with dozens of Fortune 500 and top enterprise leaders,2 and surveyed 70 more, to understand how they’re using, buying, and budgeting for generative AI. We were shocked by how significantly the resourcing and attitudes toward genAI had changed over the last 6 months. Though these leaders still have some reservations about deploying generative AI, they’re also nearly tripling their budgets, expanding the number of use cases that are deployed on smaller open-source models, and transitioning more workloads from early experimentation into production.
ここ数ヶ月の間に、私たちはFortune 500企業やトップのエンタープライズリーダーと数十回にわたって話し合い、さらに70人以上にアンケートを行い、生成AIをどのように使用し、購入し、予算化しているかを理解しました。この6ヶ月間で生成AIに対するリソース配分と態度が大きく変化したことに驚きました。これらのリーダーは生成AIの導入にはまだ懸念を持っていますが、予算をほぼ3倍に増やし、小規模なオープンソースモデルで展開されるユースケースの数を増やし、より多くのワークロードを初期の実験段階から本番環境に移行させています。
This is a massive opportunity for founders. We believe that AI startups who 1) build for enterprises’ AI-centric strategic initiatives while anticipating their pain points, and 2) move from a services-heavy approach to building scalable products will capture this new wave of investment and carve out significant market share.
これはスタートアップにとって大きなチャンスです。1) エンタープライズのAI中心の戦略的イニシアチブに対応しつつ、その課題を予測し、2) サービス中心のアプローチからスケーラブルな製品の構築へと移行するAIスタートアップが、この新しい投資の波を捉え、大きな市場シェアを獲得すると私たちは考えています。
As always, building and selling any product for the enterprise requires a deep understanding of customers’ budgets, concerns, and roadmaps. To clue founders into how enterprise leaders are making decisions about deploying generative AI—and to give AI executives a handle on how other leaders in the space are approaching the same problems they have—we’ve outlined 16 top-of-mind considerations about resourcing, models, and use cases from our recent conversations with those leaders below.
常にエンタープライズ向けの製品を構築し、販売するには、顧客の予算、懸念事項、ロードマップを深く理解する必要があります。エンタープライズのリーダーが生成AIの導入についてどのように意思決定を行っているかを創業者に知ってもらうため、また、同じ問題に取り組んでいるAI幹部に、他のリーダーがどのようなアプローチをとっているかを把握してもらうために、最近のリーダーとの会話から、リソース、モデル、ユースケースに関する16の重要な考慮事項を以下に示します。
Resourcing: budgets are growing dramatically and here to stay
リソース:予算は劇的に増加し、その傾向は続く
1. Budgets for generative AI are skyrocketing.
1. 生成AIの予算は急増しています。
In 2023, the average spend across foundation model APIs, self-hosting, and fine-tuning models was $7M across the dozens of companies we spoke to. Moreover, nearly every single enterprise we spoke with saw promising early results of genAI experiments and planned to increase their spend anywhere from 2x to 5x in 2024 to support deploying more workloads to production.
2023年、私たちが話をした数十社の平均支出は、基盤モデルAPI、自己ホスティング、モデルのファインチューニングで700万ドルでした。さらに、私たちが話をしたほぼすべてのエンタープライズが、生成AIの実験で有望な初期結果を得ており、より多くのワークロードを本番環境に展開するために、2024年には2倍から5倍の支出増加を計画していました。
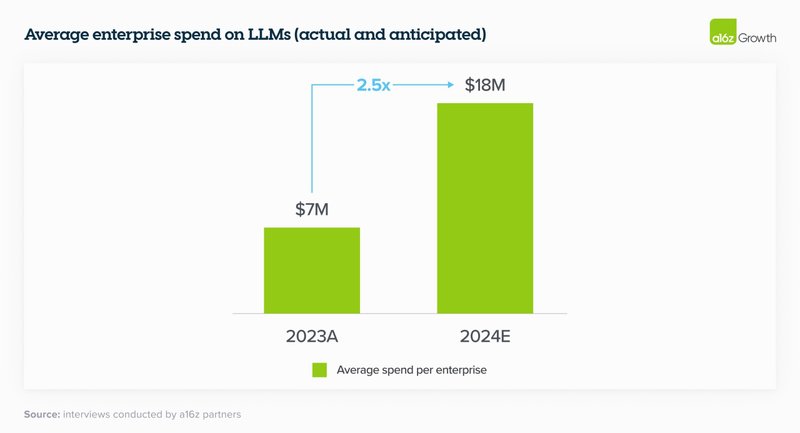
2. Leaders are starting to reallocate AI investments to recurring software budget lines.
2. リーダーたちは、AI投資を継続的なソフトウェア予算項目に再配分し始めています。
Last year, much of enterprise genAI spend unsurprisingly came from “innovation” budgets and other typically one-time pools of funding. In 2024, however, many leaders are reallocating that spend to more permanent software line items; fewer than a quarter reported that genAI spend will come from innovation budgets this year. On a much smaller scale, we’ve also started to see some leaders deploying their genAI budget against headcount savings, particularly in customer service. We see this as a harbinger of significantly higher future spend on genAI if the trend continues. One company cited saving ~$6 for each call served by their LLM-powered customer service—for a total of ~90% cost savings—as a reason to increase their investment in genAI eightfold.
昨年、エンタープライズの生成AI支出の多くは、当然ながら「イノベーション」予算やその他の通常は一時的な資金プールから来ていました。しかし、2024年には、多くのリーダーがその支出をより恒久的なソフトウェアの予算項目に再配分しています。生成AI支出が今年のイノベーション予算から来ると報告したのは4分の1未満でした。はるかに小さな規模ではありますが、特にカスタマーサービスの分野で、生成AI予算を人件費の削減に充てているリーダーも出てきています。この傾向が続けば、将来的に生成AIへの支出が大幅に増加する兆しであると私たちは考えています。ある企業は、LLMを活用したカスタマーサービスで1件あたり約6ドルの節約(合計で約90%のコスト削減)ができたことを理由に、生成AIへの投資を8倍に増やしたと述べています。
Here’s the overall breakdown of how orgs are allocating their LLM spend:
以下は、組織がLLM支出をどのように配分しているかの全体的な内訳です。
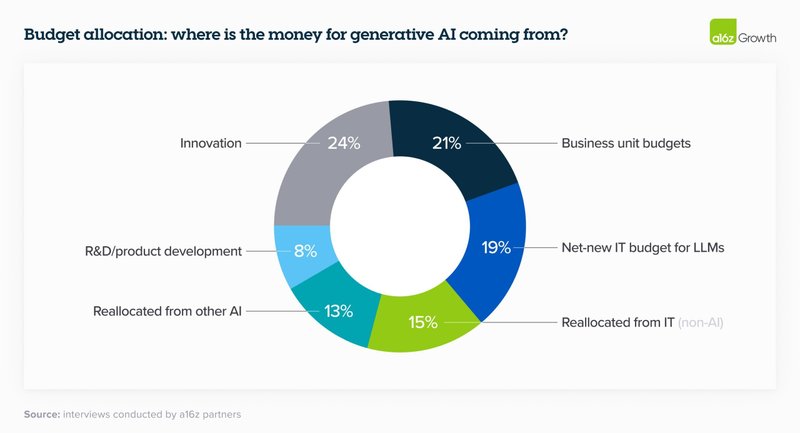
3. Measuring ROI is still an art and a science.
3. ROIの測定はまだ芸術であり科学です。
Enterprise leaders are currently mostly measuring ROI by increased productivity generated by AI. While they are relying on NPS and customer satisfaction as good proxy metrics, they’re also looking for more tangible ways to measure returns, such as revenue generation, savings, efficiency, and accuracy gains, depending on their use case. In the near term, leaders are still rolling out this tech and figuring out the best metrics to use to quantify returns, but over the next 2 to 3 years ROI will be increasingly important. While leaders are figuring out the answer to this question, many are taking it on faith when their employees say they’re making better use of their time.
現在、エンタープライズのリーダーたちは、主にAIによって生み出される生産性の向上によってROIを測定しています。NPS(Net Promoter Scoreの略で、企業や商品・サービスへの愛着度がどの程度あるかを数値化し、顧客ロイヤルティを測る指標)や顧客満足度を良い代理指標として頼りにしていますが、ユースケースに応じて、収益の創出、節約、効率性、精度の向上など、より具体的な収益の測定方法も模索しています。短期的には、リーダーたちはこの技術を展開し、収益を定量化するために最適な指標を見つけ出そうとしていますが、今後2〜3年の間に、ROIはますます重要になってくるでしょう。リーダーたちがこの問題の答えを見つけ出そうとしている間、多くの人は、従業員が時間を有効に使っていると言うことを信じています。
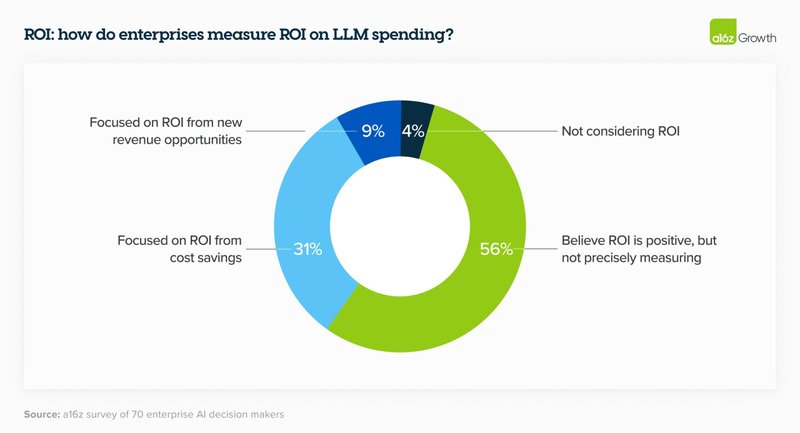
4. Implementing and scaling generative AI requires the right technical talent, which currently isn’t in-house for many enterprises.
4. 生成AIの実装とスケーリングには適切な技術人材が必要ですが、現在、多くの企業では社内にいません。
Simply having an API to a model provider isn’t enough to build and deploy generative AI solutions at scale. It takes highly specialized talent to implement, maintain, and scale the requisite computing infrastructure. Implementation alone accounted for one of the biggest areas of AI spend in 2023 and was, in some cases, the largest. One executive mentioned that “LLMs are probably a quarter of the cost of building use cases,” with development costs accounting for the majority of the budget.
単にモデルプロバイダーへのAPIを持っているだけでは、生成AIソリューションを大規模に構築し、展開するには不十分です。必要なコンピューティングインフラストラクチャを実装、維持、スケーリングするには、高度に専門化された人材が必要です。実装だけでも2023年のAI支出の最大の領域の1つを占めており、場合によっては最大のものでした。あるエグゼクティブは、「LLMはユースケースを構築するコストの4分の1程度で、開発コストが予算の大部分を占めている」と述べています。
In order to help enterprises get up and running on their models, foundation model providers offered and are still providing professional services, typically related to custom model development. We estimate that this made up a sizable portion of revenue for these companies in 2023 and, in addition to performance, is one of the key reasons enterprises selected certain model providers. Because it’s so difficult to get the right genAI talent in the enterprise, startups who offer tooling to make it easier to bring genAI development in house will likely see faster adoption.
エンタープライズがモデルを稼働させるのを助けるために、ファウンデーションモデルプロバイダーは、通常はカスタムモデル開発に関連するプロフェッショナルサービスを提供し、現在も提供し続けています。これは2023年のこれらの企業の収益のかなりの部分を占めており、パフォーマンスに加えて、エンタープライズが特定のモデルプロバイダーを選択する主な理由の1つであると私たちは推測しています。エンタープライズで適切なgenAI人材を確保することが非常に難しいため、genAI開発を社内に持ち込むことを容易にするツールを提供するスタートアップは、より早く採用されるでしょう。
Models: enterprises are trending toward a multi-model, open source world
モデル:エンタープライズはマルチモデル、オープンソースの世界に向かっている
5. A multi-model future.
5. マルチモデルの未来。
Just over 6 months ago, the vast majority of enterprises were experimenting with 1 model (usually OpenAI’s) or 2 at most. When we talked to enterprise leaders today, they’re are all testing—and in some cases, even using in production—multiple models, which allows them to 1) tailor to use cases based on performance, size, and cost, 2) avoid lock-in, and 3) quickly tap into advancements in a rapidly moving field. This third point was especially important to leaders, since the model leaderboard is dynamic and companies are excited to incorporate both current state-of-the-art models and open-source models to get the best results.
わずか6ヶ月前、大多数のエンタープライズは1つのモデル(通常はOpenAIの)、または最大でも2つのモデルを試していました。今日、エンタープライズのリーダーと話をしたところ、彼らは皆、複数のモデルをテストしており、場合によっては本番環境でも使用しています。これにより、1) パフォーマンス、サイズ、コストに基づいてユースケースに合わせてカスタマイズし、2) ロックインを避け、3) 急速に変化する分野の進歩に素早く対応することができます。モデルのリーダーボードは動的であり、企業は最良の結果を得るために、現在の最先端のモデルとオープンソースのモデルの両方を取り入れることに意欲的であるため、この3番目の点はリーダーにとって特に重要でした。
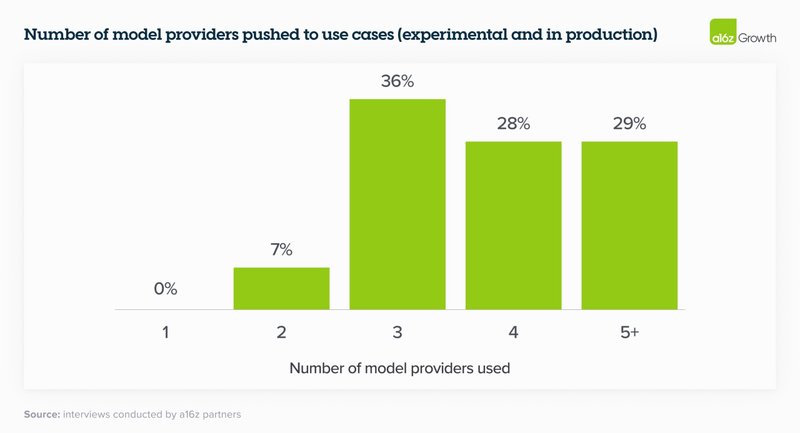
We’ll likely see even more models proliferate. In the table below drawn from survey data, enterprise leaders reported a number of models in testing, which is a leading indicator of the models that will be used to push workloads to production. For production use cases, OpenAI still has dominant market share, as expected.
今後、さらに多くのモデルが普及していくことでしょう。以下の表は、アンケートデータから作成したものですが、エンタープライズのリーダーたちは、多くのモデルをテストしていると報告しています。これは、ワークロードを本番環境に移行するために使用されるモデルの先行指標となります。本番環境のユースケースでは、予想通りOpenAIがまだ圧倒的なシェアを持っています。
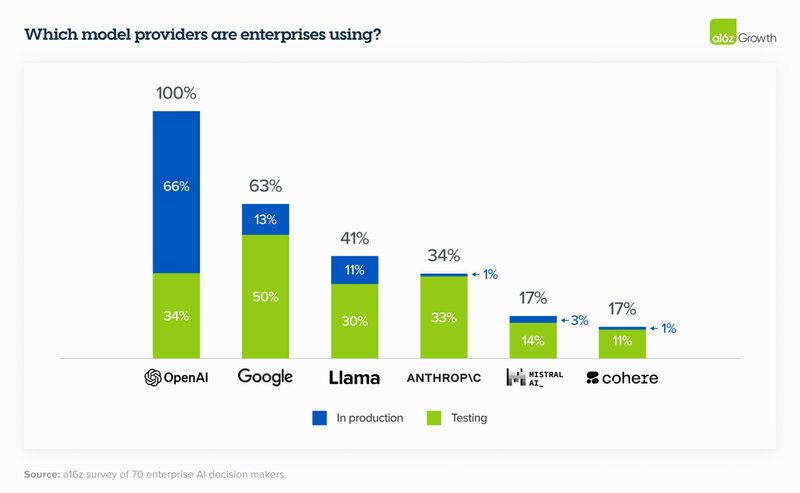
6. Open source is booming.
6. オープンソースが急成長しています。
This is one of the most surprising changes in the landscape over the past 6 months. We estimate the market share in 2023 was 80%–90% closed source, with the majority of share going to OpenAI. However, 46% of survey respondents mentioned that they prefer or strongly prefer open source models going into 2024. In interviews, nearly 60% of AI leaders noted that they were interested in increasing open source usage or switching when fine-tuned open source models roughly matched performance of closed-source models. In 2024 and onwards, then, enterprises expect a significant shift of usage towards open source, with some expressly targeting a 50/50 split—up from the 80% closed/20% open split in 2023.
これは、過去6ヶ月間で最も驚くべき変化の1つです。2023年の市場シェアは80〜90%がクローズドソースで、そのほとんどがOpenAIに行っていたと私たちは推定しています。しかし、アンケートの回答者の46%が、2024年に向けてオープンソースのモデルを好むか、強く好むと述べています。インタビューでは、AIリーダーのほぼ60%が、ファインチューニングされたオープンソースモデルのパフォーマンスがクローズドソースモデルとほぼ同等になった場合、オープンソースの使用を増やすか、切り替えることに興味があると述べています。したがって、2024年以降、エンタープライズはオープンソースへの使用の大幅なシフトを予想しており、一部では2023年の80%クローズド/20%オープンの割合から、50/50の割合を明示的に目標としています。
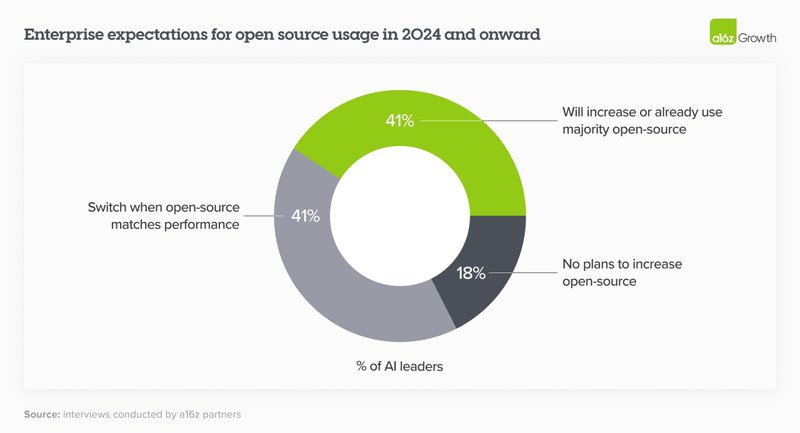
7. While cost factored into open source appeal, it ranked below control and customization as key selection criteria.
7. オープンソースの魅力にコストが影響していましたが、主要な選択基準としては、コストより制御とカスタマイズの優位性が高いです。
Control (security of proprietary data and understanding why models produce certain outputs) and customization (ability to effectively fine-tune for a given use case) far outweighed cost as the primary reasons to adopt open source. We were surprised that cost wasn’t top of mind, but it reflects the leadership’s current conviction that the excess value created by generative AI will likely far outweigh its price. As one executive explained: “getting an accurate answer is worth the money.”
制御(独自のデータのセキュリティと、モデルが特定の出力を生成する理由の理解)とカスタマイズ(特定のユースケースに効果的にファインチューニングする能力)は、オープンソースを採用する主な理由として、コストをはるかに上回りました。コストが最優先事項ではなかったことに驚きましたが、これは、生成AIによって生み出される過剰な価値が、そのコストをはるかに上回る可能性が高いという現在のリーダーシップの確信を反映しています。あるエグゼクティブは次のように説明しています。「正確な答えを得ることはお金に値する」と。
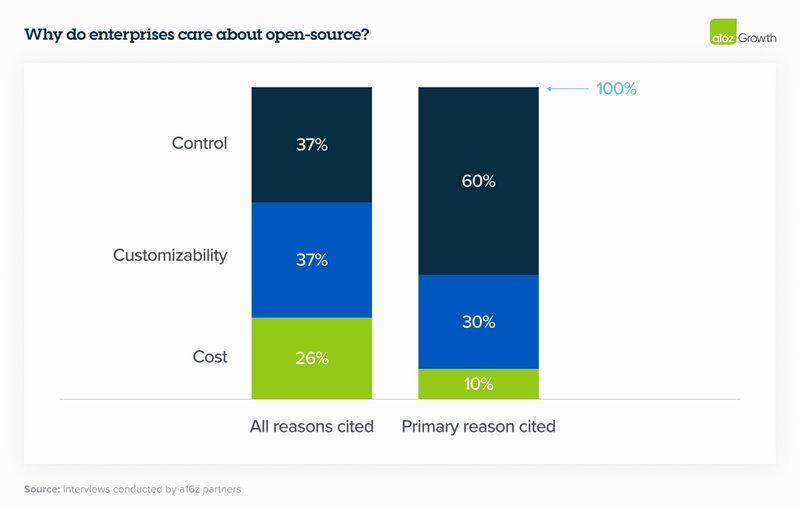
8. Desire for control stems from sensitive use cases and enterprise data security concerns.
8. 制御への欲求は、機密性の高いユースケースとエンタープライズのデータセキュリティの懸念に起因しています。
Enterprises still aren’t comfortable sharing their proprietary data with closed-source model providers out of regulatory or data security concerns—and unsurprisingly, companies whose IP is central to their business model are especially conservative. While some leaders addressed this concern by hosting open source models themselves, others noted that they were prioritizing models with virtual private cloud (VPC) integrations.
エンタープライズは、規制上またはデータセキュリティ上の懸念から、独自のデータをクローズドソースのモデルプロバイダーと共有することにまだ抵抗があります。当然ながら、知的財産が事業モデルの中心である企業は特に保守的です。一部のリーダーは、オープンソースモデルを自社でホスティングすることでこの懸念に対処しましたが、他のリーダーは、仮想プライベートクラウド(VPC)との統合を備えたモデルを優先していると指摘しました。
9. Leaders generally customize models through fine-tuning instead of building models from scratch.
9. リーダーは一般的に、ゼロからモデルを構築するのではなく、ファインチューニングを通じてモデルをカスタマイズします。
In 2023, there was a lot of discussion around building custom models like BloombergGPT. In 2024, enterprises are still interested in customizing models, but with the rise of high-quality open source models, most are opting not to train their own LLM from scratch and instead use retrieval-augmented generation (RAG) or fine-tune an open source model for their specific needs.
2023年には、BloombergGPTのようなカスタムモデルの構築に関する議論が多くありました。2024年には、エンタープライズはまだモデルのカスタマイズに興味を持っていますが、高品質のオープンソースモデルの台頭により、ほとんどがゼロからLLMを独自にトレーニングすることを選択せず、代わりにRAG(検索により強化した文章生成)を使用するか、特定のニーズに合わせてオープンソースモデルをファインチューニングしています。
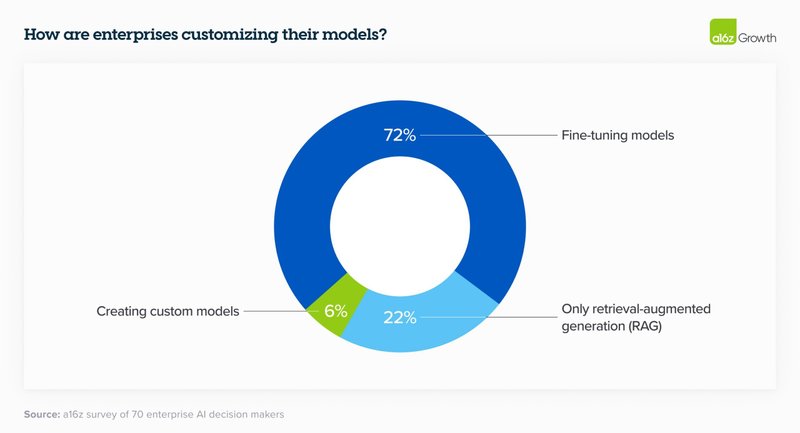
10. Cloud is still highly influential in model purchasing decisions.
10. クラウドはモデル購入の意思決定に依然として大きな影響を与えています。
In 2023, many enterprises bought models through their existing cloud service provider (CSP) for security reasons—leaders were more concerned about closed-source models mishandling their data than their CSPs—and to avoid lengthy procurement processes. This is still the case in 2024, which means that the correlation between CSP and preferred model is fairly high: Azure users generally preferred OpenAI, while Amazon users preferred Anthropic or Cohere. As we can see in the chart below, of the 72% of enterprises who use an API to access their model, over half used the model hosted by their CSP. (Note that over a quarter of respondents did self-host, likely in order to run open source models.)
2023年、多くのエンタープライズは、セキュリティ上の理由から既存のクラウドサービスプロバイダー(CSP)を通じてモデルを購入しました。リーダーは、クローズドソースモデルがデータを誤って処理することよりも、CSPを懸念していました。また、長い調達プロセスを避けるためでもありました。これは2024年でもまだ当てはまります。つまり、CSPと優先モデルの相関関係はかなり高いということです。Azureユーザーは一般的にOpenAIを好み、Amazonユーザーは人類学やCohereを好みました。下の図からわかるように、モデルにアクセスするためにAPIを使用しているエンタープライズの72%のうち、半数以上がCSPがホストするモデルを使用しています。(回答者の4分の1以上が自己ホスティングを行っていますが、これはオープンソースモデルを実行するためと思われます)。
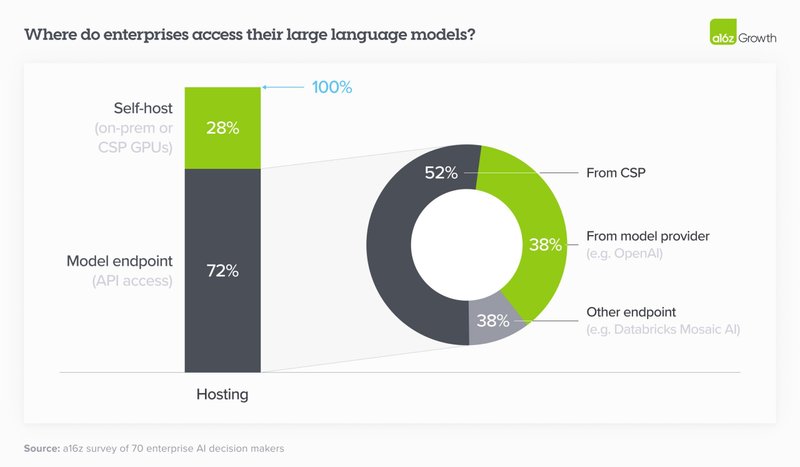
11. Customers still care about early-to-market features.
11. 顧客はまだ早期市場投入の機能を重視しています。
While leaders cited reasoning capability, reliability, and ease of access (e.g., on their CSP) as the top reasons for adopting a given model, leaders also gravitated toward models with other differentiated features. Multiple leaders cited the prior 200K context window as a key reason for adopting Anthropic, for instance, while others adopted Cohere because of their early-to-market, easy-to-use fine-tuning offering.
リーダーたちは、ある特定のモデルを採用する最大の理由として、推論能力、信頼性、アクセスのしやすさ(例えば、CSP上で)を挙げましたが、他の差別化された機能を持つモデルにも引き寄せられました。例えば、複数のリーダーがAnthropicを採用した主な理由として、以前の200Kのコンテキストウィンドウを挙げました。また、早期市場投入で使いやすいファインチューニングを提供しているため、Cohereを採用したリーダーもいました。
12. That said, most enterprises think model performance is converging.
12. とはいえ、ほとんどのエンタープライズはモデルのパフォーマンスが収束していると考えています。
While large swathes of the tech community focus on comparing model performance to public benchmarks, enterprise leaders are more focused on comparing the performance of fine-tuned open-source models and fine-tuned closed-source models against their own internal sets of benchmarks. Interestingly, despite closed-source models typically performing better on external benchmarking tests, enterprise leaders still gave open-source models relatively high NPS (and in some cases higher) because they’re easier to fine-tune to specific use cases. One company found that “after fine-tuning, Mistral and Llama perform almost as well as OpenAI but at much lower cost.” By these standards, model performance is converging even more quickly than we anticipated, which gives leaders a broader range of very capable models to choose from.
技術コミュニティの大部分がモデルのパフォーマンスを公開ベンチマークと比較することに注目している一方で、エンタープライズのリーダーは、ファインチューニングされたオープンソースモデルとファインチューニングされたクローズドソースモデルのパフォーマンスを、自社の内部ベンチマークセットと比較することに注力しています。興味深いことに、クローズドソースモデルは通常、外部ベンチマークテストでより良いパフォーマンスを示すにもかかわらず、エンタープライズのリーダーは、特定のユースケースにファインチューニングしやすいため、オープンソースモデルに比較的高いNPS(場合によってはより高い)を与えています。ある企業は、「ファインチューニング後、MistralとLlamaはOpenAIとほぼ同等のパフォーマンスを発揮するが、コストははるかに低い」ことがわかりました。これらの基準によれば、モデルのパフォーマンスは私たちが予想していたよりもさらに速く収束しており、リーダーに非常に有能な幅広いモデルの選択肢を与えています。
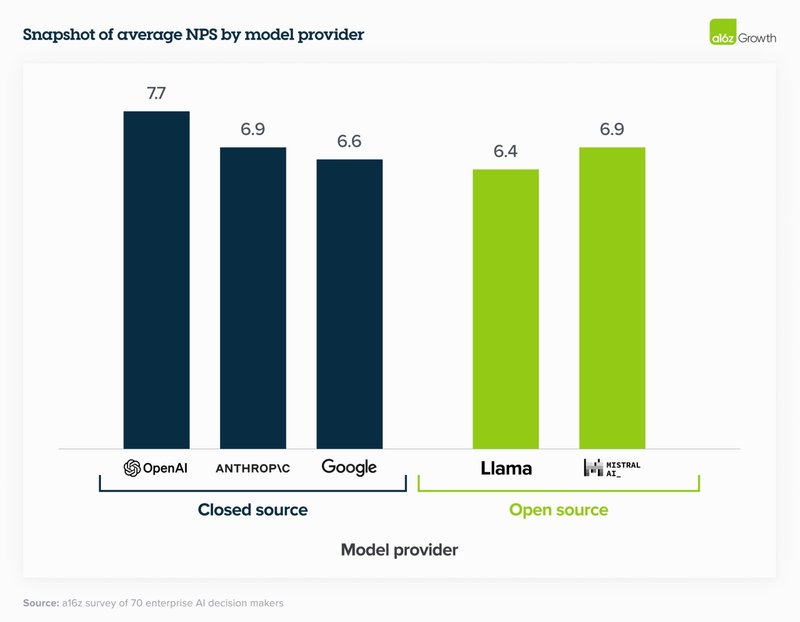
13. Optimizing for optionality.
13. 選択肢の最適化。
Most enterprises are designing their applications so that switching between models requires little more than an API change. Some companies are even pre-testing prompts so the change happens literally at the flick of a switch, while others have built “model gardens” from which they can deploy models to different apps as needed. Companies are taking this approach in part because they’ve learned some hard lessons from the cloud era about the need to reduce dependency on providers, and in part because the market is evolving at such a fast clip that it feels unwise to commit to a single vendor.
ほとんどのエンタープライズは、モデルの切り替えにAPIの変更以上のことを必要としないようにアプリケーションを設計しています。一部の企業では、文字通りスイッチを切り替えるだけで変更が行われるようにプロンプトを事前にテストしています。また、必要に応じて異なるアプリにモデルを展開できる「モデルガーデン」を構築している企業もあります。企業がこのアプローチを取っているのは、一つにはクラウド時代から学んだ、プロバイダーへの依存度を下げる必要性という厳しい教訓があるためであり、もう一つには市場が非常に速いペースで進化しているため、単一のベンダーにコミットするのは賢明ではないと感じているためです。
Use cases: more migrating to production
ユースケース:より多くが本番環境に移行
14. Enterprises are building, not buying, apps—for now.
14. エンタープライズはアプリを購入するのではなく、構築しています(今のところ)。
Enterprises are overwhelmingly focused on building applications in house, citing the lack of battle-tested, category-killing enterprise AI applications as one of the drivers. After all, there aren’t Magic Quadrants for apps like this (yet!). The foundation models have also made it easier than ever for enterprises to build their own AI apps by offering APIs. Enterprises are now building their own versions of familiar use cases—such as customer support and internal chatbots—while also experimenting with more novel use cases, like writing CPG recipes, narrowing the field for molecule discovery, and making sales recommendations. Much has been written about the limited differentiation of “GPT wrappers,” or startups building a familiar interface (e.g., chatbot) for a well-known output of an LLM (e.g., summarizing documents); one reason we believe these will struggle is that AI further reduced the barrier to building similar applications in-house.
エンタープライズは、社内でのアプリケーション構築に圧倒的に注力しており、その理由の1つとして、実戦で試された、カテゴリーを席巻するようなエンタープライズAIアプリケーションが不足していることを挙げています。結局のところ、このようなアプリのマジッククアドラントはまだ存在しないのです。また、ファウンデーションモデルがAPIを提供することで、エンタープライズが独自のAIアプリを構築することがこれまで以上に容易になりました。エンタープライズは現在、カスタマーサポートや社内チャットボットなどのおなじみのユースケースの独自バージョンを構築する一方で、CPGレシピの作成、分子発見の絞り込み、販売推奨などのより新しいユースケースも試しています。「GPTラッパー」、つまりLLMの周知の出力(例:ドキュメントの要約)に使い慣れたインターフェース(例:チャットボット)を構築するスタートアップの差別化が限られていることについては多くが書かれています。私たちがこれらが苦戦すると考える理由の1つは、AIがさらに同様のアプリケーションを社内で構築する障壁を下げたことです。
However, the jury is still out on whether this will shift when more enterprise-focused AI apps come to market. While one leader noted that though they were building many use cases in house, they’re optimistic “there will be new tools coming up” and would prefer to “use the best out there.” Others believe that genAI is an increasingly “strategic tool” that allows companies to bring certain functionalities in-house instead of relying as they traditionally have on external vendors. Given these dynamics, we believe that the apps that innovate beyond the “LLM + UI” formula and significantly rethink the underlying workflows of enterprises or help enterprises better use their own proprietary data stand to perform especially well in this market.
しかし、エンタープライズ向けのAIアプリがより多く市場に出回るようになったときに、この状況が変化するかどうかはまだ分かりません。あるリーダーは、多くのユースケースを社内で構築しているものの、「新しいツールが登場するだろう」と楽観的であり、「最高のものを使いたい」と述べています。他のリーダーは、生成AIは戦略的ツールとしてますます重要になっており、企業が従来のように外部ベンダーに頼るのではなく、特定の機能を社内に取り込むことを可能にすると考えています。これらのダイナミクスを考えると、「LLM + UI」の定型を超えて革新し、エンタープライズの根底にあるワークフローを大幅に見直したり、エンタープライズが独自のデータをより有効に活用できるようにしたりするアプリは、この市場で特に良好なパフォーマンスを発揮すると私たちは考えています。
15. Enterprises are excited about internal use cases but remain more cautious about external ones.
15. エンタープライズは内部のユースケースに興奮していますが、外部のユースケースについてはより慎重です。
That’s because 2 primary concerns about genAI still loom large in the enterprise: 1) potential issues with hallucination and safety, and 2) public relations issues with deploying genAI, particularly into sensitive consumer sectors (e.g., healthcare and financial services). The most popular use cases of the past year were either focused on internal productivity or routed through a human before getting to a customer—like coding copilots, customer support, and marketing. As we can see in the chart below, these use cases are still dominating in the enterprise in 2024, with enterprises pushing totally internal use cases like text summarization and knowledge management (e.g., internal chatbot) to production at far higher rates than sensitive human-in-the-loop use cases like contract review, or customer-facing use cases like external chatbots or recommendation algorithms. Companies are keen to avoid the fallout from generative AI mishaps like the Air Canada customer service debacle. Because these concerns still loom large for most enterprises, startups who build tooling that can help control for these issues could see significant adoption.
それは、エンタープライズにおいて、genAIに関する2つの主要な懸念が依然として大きく立ちはだかっているからです。1) 幻覚や安全性の問題の可能性、2) genAIの展開、特に医療や金融サービスなどの機密性の高い消費者セクターへの展開に関する広報上の問題です。昨年最も人気のあったユースケースは、内部の生産性に焦点を当てたものか、顧客に届く前に人間を経由するもの(コーディングコパイロット、カスタマーサポート、マーケティングなど)でした。下の図からわかるように、これらのユースケースは2024年のエンタープライズでも依然として主流となっており、エンタープライズは、テキストの要約やナレッジマネジメント(内部チャットボットなど)などの完全に内部のユースケースを、契約レビューなどの機密性の高いヒューマン・イン・ザ・ループ(人間による継続的なフィードバックを機械学習ライフサイクルのループの中に含めること)のユースケースや、外部チャットボットや推奨アルゴリズムなどの顧客向けのユースケースよりもはるかに高い割合で本番環境に移行させています。企業は、エア・カナダのカスタマーサービスの失敗のような、生成AIの失敗による影響を避けたいと考えています。これらの懸念がほとんどのエンタープライズにとって依然として大きいため、これらの問題を管理するためのツールを構築するスタートアップは、大きな採用を見込めるかもしれません。
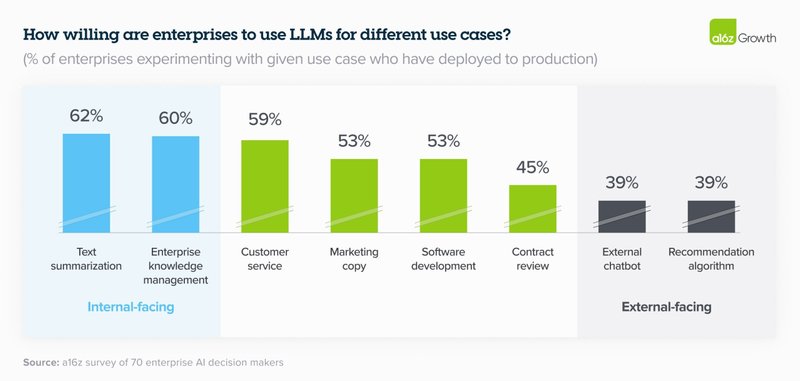
Size of total opportunity: massive and growing quickly
総機会の規模:巨大かつ急速に成長
16. We believe total spend on model APIs and fine-tuning will grow to over $5B run-rate by the end of 2024, and enterprise spend will make up a significant part of that opportunity.
16. モデルAPIとファインチューニングの総支出は、2024年末までに50億ドル以上の売上高に成長し、エンタープライズの支出がその機会の大部分を占めると私たちは考えています。
By our calculations, we estimate that the model API (including fine-tuning) market ended 2023 around $1.5–2B run-rate revenue, including spend on OpenAI models via Azure. Given the anticipated growth in the overall market and concrete indications from enterprises, spend on this area alone will grow to at least $5B run-rate by year end, with significant upside potential. As we’ve discussed, enterprises have prioritized genAI deployment, increased budgets and reallocated them to standard software lines, optimized use cases across different models, and plan to push even more workloads to production in 2024, which means they’ll likely drive a significant chunk of this growth.
私たちの計算では、Azure経由のOpenAIモデルへの支出を含めて、モデルAPI(ファインチューニングを含む)市場は2023年末に約15〜20億ドルの売上高で終わったと推定しています。全体的な市場の予想される成長とエンタープライズからの具体的な兆候を考えると、この分野だけでの支出は、年末までに少なくとも50億ドルの売上高に成長し、大幅な上昇の可能性があります。これまで議論してきたように、エンタープライズはgenAIの導入を優先し、予算を増やして標準的なソフトウェアラインに再配分し、さまざまなモデル間でユースケースを最適化し、2024年にはさらに多くのワークロードを本番環境に移行する計画であるため、この成長の大部分を牽引する可能性が高いです。
Over the past 6 months, enterprises have issued a top-down mandate to find and deploy genAI solutions. Deals that used to take over a year to close are being pushed through in 2 or 3 months, and those deals are much bigger than they’ve been in the past. While this post focuses on the foundation model layer, we also believe this opportunity in the enterprise extends to other parts of the stack—from tooling that helps with fine-tuning, to model serving, to application building, and to purpose-built AI native applications. We’re at an inflection point in genAI in the enterprise, and we’re excited to partner with the next generation of companies serving this dynamic and growing market.
過去6ヶ月間、エンタープライズは生成AIソリューションを見つけて導入するというトップダウンの義務を発行してきました。以前は1年以上かかっていた取引が2〜3ヶ月で成立するようになり、それらの取引は過去よりもはるかに大規模になっています。この記事ではファウンデーションモデルレイヤーに焦点を当てていますが、エンタープライズにおけるこの機会は、ファインチューニングに役立つツーリングから、モデルの提供、アプリケーションの構築、目的別に構築されたAIネイティブアプリケーションまで、スタックの他の部分にも及ぶと私たちは考えています。私たちはエンタープライズにおける生成AIの変曲点にいます。そして、この動的で成長する市場に対応する次世代の企業と提携できることを楽しみにしています。
この記事が気に入ったらサポートをしてみませんか?