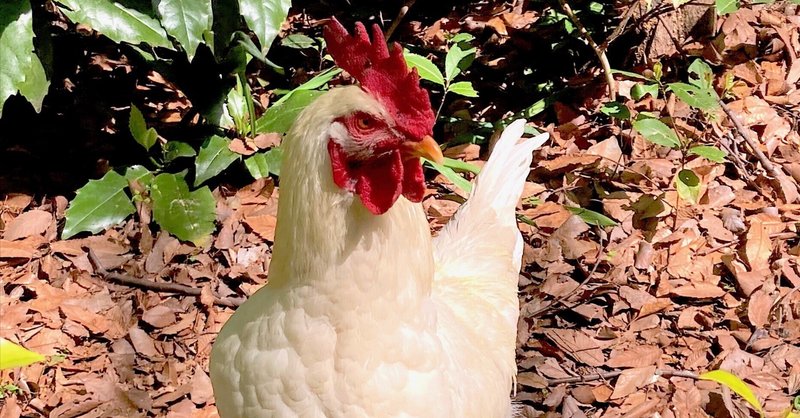
Tabula Gallusへの道
2022年1月にIJMS誌に発表した総説「Towards Tabula Gallus」の日本語翻訳です。DeepLを用いたのち、手動で修正しましたので、不完全な箇所が沢山あります。
Towards Tabula Gallus Masahito Yamagata
Int. J. Mol. Sci. 2022, 23(2), 613
© 2022 by the author. Licensee MDPI, Basel, Switzerland. This article is an open access article distributed under the terms and conditions of the Creative Commons Attribution (CC BY) license (https://creativecommons.org/licenses/by/4.0/).
-------------------------------------------------------------------------
Tabula Gallusに向かって
ハーバード大学 山形方人
原文(オープンアクセス)
https://www.mdpi.com/1422-0067/23/2/613/htm
概要
Tabula Gallusは、ニワトリの体内およびニワトリ胚のすべての細胞タイプのマップを作成することを目的としたプロジェクトの提案である。ニワトリ(Gallus gallus)は、哺乳類の発生と生理を再現するモデル動物として最も認知されている動物の一つである。Tabula Gallusは、現在進行中の他の細胞アトラスプロジェクト(マウスはTabula Muris、ヒトはTabula Sapiens/Human Cell Atlas)と同様に、Gallus gallusの単一細胞トランスクリプトームデータを作成し、各細胞タイプの特徴を明らかにし、この種の生物学研究のためのツールを提供する。Tabula Gallusは、多くの研究者による国際的な共同研究となる可能性がある。このプロジェクトは、Gallus gallusや他の鳥類の基礎科学的研究(細胞生物学、分子生物学、発生生物学、神経科学、生理学、腫瘍学、ウイルス学、行動学、生態学、進化学など)に役立つと思われる。いずれは人間の健康や病気の理解にも有益なものとなるだろう。
キーワード:ニワトリ、Gallus gallus、鳥類、単一細胞RNAシーケンス、トランスクリプトーム、セルアトラス、発生学、バイオイメージング、抗体、進化
1. はじめに
ニワトリ(Gallus gallus)は、西南アジアのセキショクヤケイを起源とする最も一般的な家畜である[1,2]。ニワトリは卵や肉として広く消費されるだけでなく、一部の地域では、羽毛、観賞用ペット、鳴き声コンテスト、闘鶏に利用されている。また、ニワトリは、生きた卵の尿膜液でウイルス粒子を増殖させることにより、ワクチン製造に欠かせない存在となっている。しかし、最も重要なことは、ニワトリは生物学的および医学的研究のための最も貴重なモデル動物の1つであるということだ[3,4]。本総説の目的は、ニワトリの基礎科学の重要性を提唱し、シングルセルRNAシーケンス(scRNA-seq)データを含むマルチモーダル情報のすべてを統合した細胞アトラス「Tabula Gallus」(Tabula:tablet、地図)を提案することである。
ニワトリに関する基礎知識は、鳥類学の全般において必要不可欠である。ハト、ウズラ、カモ、ゼブラフィンチ、ダチョウ、エミュー、フクロウ、カラス、オウムなど他の実験動物種も、生化学、生理学、解剖学、バードソングや知能などの行動の理解を深めるための動物として利用されてきた。さらに、渡り鳥をはじめとする数多くの野生動物が生態学的、鳥類学的研究に貢献してきた。他の鳥類種も特定の問題を研究するための適切なモデルとして選択することができるが[5]、ニワトリと卵は年間を通じて、また多くの場所で容易に大量に入手することができるという入手性の良さから、ニワトリが最良の鳥類モデルであるということが広く受け入れられている。
2. 背景
2.1. パスツール、ダーウィン、カハール
読者は、ヒトの理解につながるいくつかの主要な生物学的概念が、鳥類とその体の一部を見ることによって始まったことを思い出すかもしれない。19世紀後半、ルイ・パスツール Louis Pasteur(1822-1895)は、鶏コレラに対する最初の弱毒性ワクチンを偶然発見することで、その後、彼や他の人々が他の感染症に対するワクチン接種を行うきっかけとなった [6]。また、ダーウィンCharles Darwin(1809-1882)による進化論の着想には、ガラパゴス諸島のモッキンバードやフィンチが重要な役割を果たした[7]。19世紀から20世紀にかけては、カハール Santiago Ramón y Cajal(1852-1934)が鳥類の脳や網膜をゴルジ法の試料に多用し、神経細胞説を打ち立てた[8]。最近では、ローレンツ Konrad Lorentz(1903-1989)が、ガチョウの刷り込みを発見して神経行動学を確立し、動物の行動からヒトの理解の可能性を強調した[9]。
生化学と分子生物学におけるいくつかの画期的な発見も、鳥類を使って行われた。20世紀直前、Christiaan Eijkman(1858-1930)は、ニワトリに玄米を食べさせると脚気の症状が回復することに気づき、抗脚気因子(現在「ビタミンB1」と呼ばれている)の発見につながった[10]。Szent-Györgyi Albert(1893-1986)は、鳥の筋肉をミンチにして細胞呼吸を研究し、フマル酸やその他のステップ(現在ではTCAサイクルとして知られている)を特定した[11]。ラウス Peyton Rous(1879-1970)は、ニワトリの肉腫から、現在ではRous肉腫ウイルスと呼ばれている伝達性レトロウイルスを発見した[12]。このウイルスの研究は、後に逆転写酵素の発見[13]や、正常細胞におけるsrc癌遺伝子の発見[14]につながった。セントルイスのワシントン大学のViktor Hamburger(1900-2001)の研究室に所属していたRita Levi-Montalcini(1909-2012)は、マウス肉腫細胞の凝集塊を発育中のニワトリ胚に移植し、その腫瘍が近傍の感覚・交感神経節の成長を刺激する因子を分泌しているのを発見した[15]。彼女の共同研究者であるStanley Cohen(1942-2013)は、後に神経成長因子(NGF)と呼ばれるこの因子を単離し、これが最も早く発見された成長因子と考えられている[16]。ニューロンドクトリン、刷り込み、ビタミン、TCAサイクル、オンコウイルス、成長因子など、これらのパラダイムシフトを起こす概念は、20世紀中にノーベル生理学・医学賞を受賞した。
NGFに明らかなように、ニワトリとニワトリ胚から影響を受けた最も重要な分野は、おそらく発生生物学の分野であろう[3,17,18]。古代ギリシャ(紀元前330年頃)では、アリストテレスがニワトリの胚の発生を初めて観察したことを記録している [19]。近代科学の黎明期には,ウィリアム・ハーヴェイWilliam Harvey(1578-1657)とマルチェロ・マルピーギMarcello Malpighi(1628-1694)がニワトリ胚を観察し,血管の解剖と発生を研究した [20]。19世紀末には,ヴィルヘルム・ルーWilhelm Roux(1850-1924)が提唱したEntwicklungsmechanikがニワトリ胚の利用を推進した [21,22]。C. H. Waddington(1905-1975)もニワトリ胚を用い、胚軸や左右非対称性が成立するメカニズムを解析している[23]。
その後,ニワトリ胚を用いた研究は,20 世紀半ば以降の発生生物学に大きな影響を与え,発生における基本プロセスの理解のみならず,ヒトの発生や障害をモデル化する機能においても,いくつかの重要な論文や総説[25,26,27,28,29,30,31,32,33,34,35,36,37,38,39,40,41,42,43,44,45,46,47,48] において既にまとめられているとおり,大きな影響を与えている。
2.2. 多くの利点
鳥類は哺乳類と同様、空気を吸う内温動物であり、他の外温モデル動物と比較して有利である。例えば、酵素、結合体、構造タンパク質は、暖かい温度に適応している。また、鳥の体や細胞は、異種タンパク質や合成薬剤の活性をin vivoおよびin vitroで調べることができるプラットフォームとなる。ニワトリ胚からの摘出物や様々な解離細胞や維持培養することで、細胞生物学上の重要な問題に取り組むことができる[49,50,51,52,53,54].また、他の内熱動物から細胞や組織を移植してキメラを作製することも有用である(例:ニワトリ-ウズラキメラ)[28]。
ヒヨコ、ニワトリ胚、卵が生物活性物質の抽出や単離のためのスケーラブルなソースであることは見落とされがちである。たとえば、いくつかの機能性タンパク質は、何千ものニワトリ胚から生化学的に精製され、特性評価された(たとえば、参考文献[55、56、57] )。さらに、ニワトリのタンパク質の配列は、おそらく哺乳類のタンパク質の配列とは異なり、哺乳類に有利な抗原性を有していることは言及に値するはずである(下記を参照)。哺乳類IgGに相当するニワトリの免疫グロブリン(IgY)は、卵黄に移行し、容易に精製され、様々な用途に利用されている[58]。
それにもかかわらず、2004年にClaudio Sternは、「より最近のことでは、新しい若い教員や助成金申請の審査、さらには投稿原稿の一部の審査、
受理には『アンチ・ニワトリ』レイシズムの兆候が見られる」と指摘している[59]。この辛辣な見方は、今でも有効かもしれない。一般に、Jessica Bolkerが最近議論したように、我々はモデル動物の範囲を広げてより多くの種を含める必要がある[60,61]。幸いなことに、ニワトリはヒトの発生や生理をよく再現できるため、現在では有力なモデル動物の一つであると経験的に認識されている [62]。
ニワトリ生物学の数十年にわたる進歩の集大成として、ニワトリのゲノム配列決定が2004年に完了した[63]。比較的コンパクトなゲノムサイズ(〜1.1Gb)は、全ゲノムに基づく遺伝子関連付けや遺伝子発現に基づく解析に理想的な種となる[64]。さらに、鳥類ゲノムB10Kの配列決定に向けた大規模な取り組みが現在進行中である[65,66]。
近年の生殖細胞や体細胞への遺伝子導入の進歩により、遺伝子、分子、細胞種、およびそれらの関係性をさらに詳細に理解することができるようになった。例えば、遺伝子の付加やサイレンシングの効果を研究するためのエレクトロポレーションは、様々な目的で使用されている[67]。その他にも、ニワトリ胚性幹細胞や始原生殖細胞 [68,69,70,71]、piggyBacやTol2トランスポゾン [72,73,74]、各種ウイルスベクター [71,75,76,77,78,79,80] などが遺伝子構造を研究したり操作するためのツールとして用いられている。さらに最近では、CRISPRを用いたゲノム編集技術により、鳥類の特定の染色体座にレポーター遺伝子を挿入することで、単一の遺伝子の発現を直接細胞に正確に結びつけることができるようになった[81,82,83,84]。これらの分子技術と組み合わせることで、超解像顕微鏡や空間トランスクリプトミクスなどのバイオイメージングのブレークスルーにより、発生中の複雑な時空間変化に対する深い洞察が得られる可能性がある[85,86]。
単細胞プロファイリング技術の目覚しい進歩により、多細胞生物を前例のない分解能で研究できるようになり、その能力は大きく向上した[87]。したがって、これらの実証概念の進歩を利用して、Gallus gallusを細胞レベルの分解能で網羅的にアトラス化することは、ゲノムプロジェクトと同様のスケールで、野心的かつ時宜を得た試みといえる。
3. Tabula Gallus
3.1. ニワトリ細胞アトラス
Tabula Gallusは、他の進行中の細胞アトラスプロジェクト(マウスはTabula Muris、ヒトはTabula Sapiens/Human Cell Atlas)と同様に、Gallus gallusの単一細胞トランスクリプトームデータの大要を生成し、各細胞タイプを特徴付け、この種の生物学を研究するツールを提供する
[88,89,90,91]( https://tabula-muris.ds.czbiohub.org ; https://www.humancellatlas.org ;https://tabula-sapiens-portal.ds.czbiohub.org )。Tabula Gallusは、多くの研究者による国際的な共同研究となる可能性がある。このプロジェクトは、Gallus gallusや他の鳥類の基礎科学(細胞生物学、分子生物学、発生生物学、神経科学、生理学、腫瘍学、ウイルス学、行動学、生態学、進化学など)を理解するのに役立つと思われる。さらに、いずれは人間の健康や病気に関する知識にも有益なものとなるでしょう。ここで、Tabula Gallusの全体像についてまとめておく(図1)。
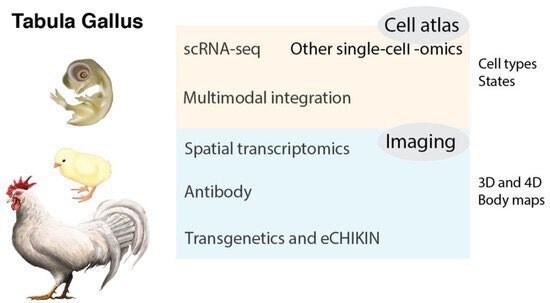
3.2. 細胞の種類と状態
細胞は、生物の構造と機能の基本単位であり、動物は、特殊な役割を持つ非常に多様な種類の細胞を進化させてきた。scRNA-seq 技術は、単一細胞の分解能で遺伝子発現を検出することを可能にし、トランスクリプトーム解析に革命をもたらしている[87,92]。scRNA-seq は、膨大な数の細胞をプロファイリングすることで、細胞の種類や状態を分解し、より高い粒度で情報を提供する。シングルセルテクノロジーには、ニワトリの利点がいくつかある。サイズが比較的大きいため(例えば、ショウジョウバエ(Drosophila melanogaster)やゼブラフィッシュ(Dario rerio)と比較して)、動物全体から器官や組織を解剖することが比較的容易である。また、多数の卵や動物を使って、多くの細胞を採取することも現実的である。したがって、手頃なコストでより多くの細胞をプロファイルし、希少な細胞タイプや胚の段階での微妙な状態を捉えることができるのは強力である。ヒトや希少動物では、新鮮な組織が利用できないため、scRNA-seqではなく、単核RNAシーケンス(snRNA-seq)が標準となっている[93]。snRNA-seqは場合によってはscRNA-seqよりも利点があるが、ニワトリの場合は両方の技術を使用することが可能である。
1つの前例としては、20の臓器および組織からのほぼ100,000個の細胞を含む実験モデル生物Mus musculusからの単一細胞トランスクリプトームデータの大要を作成したTabula Murisプロジェクトがある[88]。 このプロジェクトからのデータは、ほとんど特徴付けられていない細胞集団における遺伝子発現や多くの組織における新しいタイプの細胞の特徴付けを含む、細胞生物学の多くの新しい側面を明らかにしている。
Tabula Gallusは現在初期段階にある(2022年初頭の時点でニワトリを用いた〜20のscRNA-seq研究)[89,90,91,92,93,94,95,96,97,98,99,100,101,102,103,104,105,106,107,108]。それでも、ニワトリの肢芽[96,102]、原始線条期[95,103]、神経堤[94,100]、神経網膜[98,107]における多様な細胞型とその状態を明らかにしたいくつかの新しい研究がこのプロジェクトの実行可能性を支えている。驚くべきことに、発達中の神経網膜では、136の細胞タイプに加えて、14の位置または発達中間体が同定され、中枢神経系における多様な神経細胞タイプの研究の基礎となり[107]、鳥類の視覚系のユニークな機能を裏付けている[109]。さらに、網膜ミュラーグリア細胞のscRNA-seqにより、網膜再生を制御する可能性のある遺伝子[99]や、位置や発生状態も明らかになった[107]。
多くの単一細胞研究の生データと文献は、それぞれNCBI GEO(https://www.ncbi.nlm.nih.gov/geo/)といくつかの単一細胞文献サイト(例えば、https://panglaodb.se/papers.html、https://www.nxn.se/single-cell-studies/()で検索可能である。大規模で複雑なデータセットを探索するために、一般的にはシングルセルデータ用のインタラクティブビューア(Single Cell Portal, https://singlecell.broadinstitute.org; UCSC Cell Browser, https://cells.ucsc.edu ; EMBL-EBI, https://www.ebi.ac.uk/gxa/sc/home への入力が推奨されている。) しかし、それらのデータセットは分離している。したがって、「Tabula Gallus」への第一歩は、これらのデータセットをカタログ化し、多くの研究者の国際共同研究を促進するための統合ウェブサイトを作成することであろう。先例としては、Tabula Muris や Tabula Sapiens/Human Cell Atlas [88,89,90,91] が挙げられるだろう。さらに、検証された遺伝子発現データや画像(下記参照)などのさらなる情報を組み合わせるために、他のモデル動物の場合と同様に、GEISHA(http://geisha.arizona.edu)などの種固有のコミュニティサイトが、ニワトリ生物学のリソースとして優れた出発点となるかもしれない(例:https://flybase.org、https://wormbase.org/)。
3.3. より多くのモダリティ :エピゲノム、タンパク質、糖鎖、コネクトーム
トランスクリプトーム、アクセス可能なクロマチンシーケンシング、DNAメチローム、または選択的なタンパク質発現パターンに基づく最近の単一細胞分子プロファイリングにより、細胞タイプの微妙な区別が可能になった[87,110,111]。これらの複数のモダリティは、細胞タイプや状態の解像度をさらに促進し、より多くの情報を提供する。エピゲノム機能を多様なオミックスデータセットと統合することで、マルチモーダルオミックス解析が推進され、様々な生物学的事象を新しい視点から探索するチャンスがもたらされる。例えば、メチル化による遺伝的DNA塩基修飾やクロマチンの化学的・構造的修飾を検出するための一連のシングルセルシーケンス法は、ゲノム規模でのエピジェネティックな変化を解明することを可能にしている。特に、トランスポザーゼアクセス可能クロマチンのシングルセルシーケンスアッセイ(scATAC-seq)は、単一細胞におけるエピジェネティックなランドスケープの研究に最も広く用いられている技術である[89,112]。エピゲノムの動的な変化は、器官形成やホメオスタシスにおける正常な発達や、疾患において極めて重要な役割を担っている。
タンパク質発現シグネチャーもまた、細胞の種類や状態を定義するのに役立つ。タンパク質の発現を表すには、トランスクリプトームがよく用いられるが、転写後/翻訳後の複雑な制御やタンパク質の分解のために、mRNAの存在量とタンパク質の存在量は相関がないことが長い間注目されてきました。CITE-seq (Cellular Indexing of Transcriptomes and Epitopes by Sequencing), REAP-seq (RNA Expression and Protein Sequencing assay), Ab-seq などの最新のアプローチのいくつかは、オリゴタグ付き免疫グロブリン抗体で解離細胞をマークすることによって単一細胞のトランスクリプトームを補完して同時にタンパク質の評価をする[113,114,115, 116]。SUGAR-seqは、代わりにオリゴヌクレオチド標識レクチンを用いて、糖鎖とRNAを同時に分析する[117]。このように、これらのアプローチにより、単一細胞レベルでの遺伝子発現と他のモダリティとの定量的な相関を明らかにすることができる。
これらの分子モダリティの他に、細胞の空間情報や形態も、細胞の種類や状態を評価するのに有効な要素である(後述)。神経細胞では、電気生理学やコネクトームなどの他のモダリティが、細胞タイプの分類や遷移状態の評価に重要である[86,118,119,120,121]。
3.4. 時間的ならびに空間的トランスクリプトミクス
一般的に細胞タイプは、一般的な生物や成体の細胞プロファイルから得られる既存の情報によって分類・定義されており、発生時や分化の中間段階にのみ存在する胚性細胞タイプや一過性の細胞タイプを正確に反映していない場合がある。この問題に対処するためには、それぞれの仮の細胞型における重要なマーカー遺伝子の発現を検証することが不可欠である。従来の方法の一つは、合成RNAプローブを用いたin situハイブリダイゼーションやRNAScope™アッセイを実施することであった。これらの実験は、典型的には組織切片で、時にはホールマウントで行われることもある。さらに、評価を向上させるためには、多くの時間点を観察する必要があり、一過性の細胞状態はしばしば生体内の3次元空間にマッピングされる必要がある。さらに、同じ組織切片や細胞で複数の遺伝子の発現を同時に観察し、不均質な細胞集団を明らかにすることも有用である。例えば、Multiplexed error-robust fluorescence in situ hybridization(MERFISH)、Seq-FISH、CARTANA™テクノロジーなどの最先端のイメージング手法では、単一細胞や切片における多数の転写産物のコピー数や空間分布を同時に測定することができる[86, 120, 121, 122].これと並行して、ハイスループットなゲノム技術をin situで組織切片に適用することにより、驚くべき解像度のデータが得られ始めている。例えば、Slide-seq技術やVisium™技術では、新鮮な凍結組織切片からRNAを、それぞれDNAバーコード付きビーズの単層や、位置が分かっているバーコード付きスポットのスライドガラスに移し替える[123]。組織切片からのこのような空間トランスクリプトミクスからのデータは、解離した細胞からのscRNA-seqプロファイルと統合でき、scRNA-seqと空間トランスクリプトミクスに基づく細胞の種類と状態の解釈を容易にすることができる [87]。
Tabula Gallusは、ホモサピエンス、Tabula Muris、およびそれらの胚に関する現在進行中の多量の細胞アトラスプロジェクトで使用されている様々な標準的な方法を利用することができる。例えば、このような細胞アトラスプロジェクトでは、動物体内の位置に対する標準的な座標系(共通座標フレームワーク(CCF))が極めて重要である[124,125]。
3.5. 抗体とバイオイメージング
抗体、特に免疫組織化学に用いる抗体は、遺伝子産物やそれを発現する細胞の局在や機能を理解する上で最も強力なツールの一つである。鳥類のタンパク質に対する抗体を、免疫性の高い哺乳類(マウスやウサギなど)で作製することは、一般的に認識されていないとはいえ、通常は困難なことではない。なぜなら、すべてではないにせよ、ほとんどの鳥類由来のタンパク質配列は、哺乳類の対応する配列と離れているからである。ニワトリのタンパク質を注射すると、マウスやウサギに抗原性がある可能性が高い。各抗原は、典型的には細菌発現融合タンパク質または合成されたペプチドとして得ることができる。また、ファージや微生物表面ディスプレイ、核酸免疫など、一連の分子ディスプレイプラットフォームを適用することも可能である[126,127,128]。哺乳類細胞ディスプレイ法は、ニワトリのタンパク質に対する一連のマウス抗体の生成に成功した[107]。発生学ハイブリドーマバンクでは、ニワトリの発生学研究用のモノクローナル抗体を多数提供している(https://dshb.biology.uiowa.edu/)。
ホールマウント免疫染色やin situハイブリダイゼーションと組み合わせて、臓器や組織を透明化する組織清拭法の改良は、細胞や細胞下の解像度での分子プロファイリングを提供し、3Dや4Dのボディ発現マップの作成に役立つ[129]。さらに、画像解析の問題に対処するために、人工知能や機械学習アルゴリズムを適用することも行われる[130]。
3.6. CRISPRを介したノックインとホモロジーによるノックイン
分子的に定義された細胞タイプの構造と機能、および遺伝子の生物学的機能を明らかにするために、最もよく使われる動物(マウス、ゼブラフィッシュ、ショウジョウバエ、線虫)では生殖細胞への遺伝子導入が確立されている。例えば、目的の細胞種で特異的に発現する遺伝子の制御エレメントが、GFPなどのレポーターの発現を制御するトランスジェニック動物を作製することができる[131]。この強力な戦略はヒトには適用することはできないが、細胞の形態を観察するための様々なレポーターラインが開発されている。これらのレポーターラインは、外来DNAを生殖細胞に導入するか、相同組換えを用いてDNA配列を生殖細胞に選択的にノックインすることによって作製される。ニワトリの生殖細胞への遺伝子導入は依然として困難な方法であるが、特別な施設での遺伝子導入ニワトリ系統の作出と維持は可能である[132]。eCHIKIN (electroporation- and CRISPR-mediated Homology-Instructed Knock-IN)は、体細胞におけるCRISPRベースのゲノム編集法で、scRNA-seqデータで細胞種選択的と同定した遺伝子にGFPレポーターやCreリコンビナーゼを挿入する方法[84]である。この方法では、発育中のニワトリ胚のin ovoエレクトロポレーションが行われる。ニワトリの網膜を用いた最初の研究で、eCHIKINは再現性が高く、多くの遺伝子に使用できることが実証された[107]。このような分子遺伝学的手法は、形態学的・生理学的データを単細胞技術に相関させるものである。APEX2やHRPのような他の遺伝子レポーターも、電子顕微鏡で個々の細胞の詳細な構造をさらに研究するために使用することができる[133]。
3.7. マルチモーダル統合とコンピュータ生物学
上述のように、Tabula Gallusはすべてのモダリティを統合し、トランスクリプトーム、エピゲノム、プロテオーム、グライコーム、形態学、電気生理学、コネクトームなどの単一細胞実験データの大要を作り上げる。実験的な発生生物学は、胚性細胞と、成体で定義された細胞タイプとの関係づけに基づいている。このプロジェクトでは、ニワトリや他のモデル動物の胚性細胞に関するマルチモーダルなシングルセルデータと経験的知識を統合したin silico細胞オントロジーへの利用が期待される。ニワトリ胚は、細胞系譜、分化、細胞移動、細胞間相互作用などの基本的な問題を扱う「ウェット」な発生生物学の枠組みとして用いられてきたため、ビッグデータや機械学習などの計算機的アプローチが有望視される。
単一細胞データの最も身近な解析は、組織、器官、完全な生物の形成につながる多様な細胞種や状態、細胞系統の間の系統関係の決定であろう。歴史的に、系統関係の解析は、無傷の胚や操作された胚、あるいは試験管内で培養された細胞を顕微鏡で観察することによって行われてきた[134]。その後,様々な遺伝性レポーターが,レトロスペクティブな方法で系統の追跡のために用いられてきた[135,136].しかし、このような研究は、複製欠損レトロウイルスベクター、トランスポーザブルエレメント、リコンビナーゼなどの実験試薬を導入する必要があった。単細胞データを用いたin silicoでの潜在的遷移(擬似時間)解析は、細胞分化、細胞周期、様々な刺激に対する動的応答など、様々なプロセスにおける細胞の遷移を推定する新しい方法を切り開いてきている[137,138,139]。同様に、RNA velocityは、ある瞬間のある遺伝子の発現率が、そのスプライシングされたmRNAとされていないmRNAの比率で変化することに基づく高度なバイオインフォマティクス手法であり、細胞の遷移を推測することができる[140]。
これと並行して、単一細胞のトランスクリプトームデータと遺伝的系統マーカーを同一細胞から同時に取得する新しい技術も開発されている[139]。例えば、scGESTALTとScarTraceは、CRISPR-Cas9ベースのスカーリングと単一細胞のトランスクリプトームを組み合わせて、細胞のクローナリティとアイデンティティの両方を取得する [141,142]。intMEMOIRは、同じ組織で細胞系統、トランスクリプトーム、空間情報の単一細胞解析が可能である[143]。これらの高度な方法は、ニワトリ胚において、胚性細胞アトラスから成熟細胞アトラスがどのように構築されるかを単一細胞レベルで理解するために応用できる可能性がある。
バイオインフォマティクスは、空間層での細胞間相互作用の予測にも用いることができる。リガンドと受容体の対合を介した細胞間相互作用は、発生や成体において重要である。例えば、リガンド、受容体、及びそれらの相互作用のリポジトリであるCellPhoneDBは、単一細胞データから推測される2つの細胞タイプ間の細胞間相互作用を予測する統計的フレームワークを組み合わせている[144]。
3.8. 比較トランスクリプトミクスと細胞タイプの進化
個々の種内の細胞の種類を説明するために、単一細胞のトランスクリプトームおよびマルチモーダルアトラスの作製が大きく進展している。Charles Darwinは、1859年に出版した「種の起源」[7]において、進化は「生命の樹」のようなものであると推測している。それ以来、生物学者は生命の樹を描こうと試みてきた。強力なDNA配列解析技術と系統樹解析は、このプロジェクトに革命をもたらし、遺伝子にコード化された種間の関係を見出した。生物とその遺伝子そのものに加えて、その細胞や器官もまた進化を遂げている。原始的な動物は単純な神経系を持っているが、進化の究極の産物はヒトの脳であろう。したがって、脳内の神経細胞の種類も進化の過程で劇的に変化した[145,146,147,148]。多くのニューロンタイプと同様に、一般細胞のタイプも多細胞化が始まって以来、構造と機能が多様化した進化上の単位である [149,150,151,152]。
正常な発生過程において、細胞タイプは細胞系統や周囲の環境との関連において、転写因子やシグナル伝達経路などの制御分子で構成される遺伝子制御ネットワークによって確定される[153]。同様に、進化においても、細胞はネットワークを変化させることで多様化し、一連の新しい細胞タイプが生み出される[149,154]。細胞型の生成のメカニズムとしては、細胞タイプの複製が最も妥当であり、発生系統を変更することによって新しい細胞タイプが出現するが、細胞タイプの進化的系統と発生的系統は必ずしも同じとは限らない。一方で、ある種の細胞タイプは、膨大な進化時間の中で同じ制御ネットワークが維持されることによって維持されている。このように、scRNA-seqデータの種を超えた細胞タイプ比較の利用は、細胞タイプの進化的起源や種特異的な細胞タイプに関する疑問への答えを約束するものである。このような非常に長い進化スパンや系統(哺乳類 vs 鳥類 vs 魚類)にわたる細胞タイプの系統解析は、一般に困難と考えられてきた。しかし、近年、このような解析により、ヒトの組織においても新規の細胞タイプの機能を推定できるようになったことが示されてきている[107,147,150,151,152,155]。
4. Tabula Gallusを越えて
Tabula Gallusの蓄積されたリソースは、Gallus gallusや他の鳥類の基礎科学研究を促進するトランスクリプトーム生物学の包括的なコレクションの基盤を提供するものである。この恩恵を受ける分野は、細胞生物学、分子生物学、発生生物学、神経科学、生理学、腫瘍学、ウイルス学、行動学、生態学、進化学、養鶏/食肉産業、医学などであろう。例えば、単純な孵化卵だけでなく、様々な細胞培養法が、薬物を理解しスクリーニングするための薬理学的アッセイシステムを開発するきっかけになるかもしれない [38]。最後に、鳥類の脳の構造と機能を細胞・分子レベルで理解することは、鳥類の驚異的な認知能力と自然知能を生み出すロジスティックと遺伝プログラムを解明する新しい道を開く可能性がある[109,156,157,158]。
文献
Fumihito, A.; Miyake, T.; Sumi, S.; Takada, M.; Ohno, S.; Kondo, N. One subspecies of the red junglefowl (Gallus gallus gallus) suffices as the matriarchic ancestor of all domestic breeds. Proc. Natl. Acad. Sci. USA1994, 91, 12505–12509. [Google Scholar] [CrossRef] [PubMed]
Lawal, R.A.; Hanotte, O. Domestic chicken diversity: Origin, distribution, and adaptation. Anim. Genet. 2021, 52, 385–394. [Google Scholar] [CrossRef]
Stern, C.D. The chick: A great model system becomes even greater. Dev. Cell 2005, 8, 9–17. [Google Scholar] [CrossRef]
Burt, D.W. Emergence of the Chicken as a Model Organism: Implications for Agriculture and Biology. Poult. Sci. 2007, 86, 1460–1471. [Google Scholar] [CrossRef] [PubMed]
Flores-Santin, J.; Burggren, W.W. Beyond the Chicken: Alternative Avian Models for Developmental Physiological Research. Front. Physiol. 2021, 12, 712633. [Google Scholar] [CrossRef]
Smith, K.A. Louis Pasteur, the father of immunology? Front. Immunol. 2012, 3, 68. [Google Scholar] [CrossRef]
Darwin, C. On the Origin of Species; Harvard University Press: Cambridge, MA, USA, 1859. [Google Scholar]
Ramón y Cajal, S. Histologie du Système Nerveux de l’Homme et des Vertébrés; Maloine: Paris, France, 1911. [Google Scholar] [CrossRef]
Burkhardt, R.W. Patterns of Behavior: Konrad Lorenz, Niko Tinbergen, and the Founding of Ethology; University of Chicago Press: Chicago, IL, USA, 2005; ISBN 9780226080901. [Google Scholar]
Pietrzak, K. Christiaan Eijkman (1856–1930). J. Neurol. 2019, 266, 2893–2895. [Google Scholar] [CrossRef] [PubMed]
Ranek, M.J.; Cotten, S.W.; Willis, M.S. Albert Szent-Györgyi, MD, PhD: Discoverer of Vitamin C and a Pioneer of Cellular Respiration, Muscle Physiology, and Cancer Development. Lab. Med. 2011, 42, 694–698. [Google Scholar] [CrossRef]
Van Epps, H.L. Peyton Rous: Father of the tumor virus. J. Exp. Med. 2005, 201, 320. [Google Scholar] [CrossRef] [PubMed]
Coffin, J.M.; Fan, H. The discovery of reverse transcriptase. Annu. Rev. Virol. 2016, 3, 29–51. [Google Scholar] [CrossRef] [PubMed]
Martin, G. The road to Src. Oncogene 2004, 23, 7910–7917. [Google Scholar] [CrossRef]
Zeliadt, N. Classic Profile of Rita Levi-Montalcini. Proc. Natl. Acad. Sci. USA 2013, 110, 4873–4876. [Google Scholar] [CrossRef]
Cohen, S. Origins of growth factors: NGF and EGF. J. Biol. Chem. 2008, 283, 33793–33797. [Google Scholar] [CrossRef]
Bellairs, R.; Osmons, M. The Atlas of Chick Development, 3rd ed.; Academic Press: London, UK, 2014; ISBN 978-0-12-384951-9. [Google Scholar]
Wolpert, L. Much more from the chicken’s egg than breakfast—A wonderful model system. Mech. Dev. 2004, 121, 1015–1017. [Google Scholar] [CrossRef]
Mason, I. The Avian Embryo. Methods Mol. Biol. 2008, 461, 223–230. [Google Scholar] [CrossRef] [PubMed]
Kain, K.H.; Miller, J.W.; Jones-Paris, C.R.; Thomason, R.T.; Lewis, J.D.; Bader, D.M.; Barnett, J.V.; Zijlstra, A. The chick embryo as an expanding experimental model for cancer and cardiovascular research. Dev. Dyn.2014, 243, 216–228. [Google Scholar] [CrossRef] [PubMed]
Lillie, F.R. The Development of the Chick; Holt: New York, NY, USA, 1908. [Google Scholar] [CrossRef]
Schoenwolf, G. Cutting, pasting and painting: Experimental embryology and neural development. Nat. Rev. Neurosci. 2001, 2, 763–771. [Google Scholar] [CrossRef] [PubMed]
Stern, C.D.; Conrad, H. Waddington’s contributions to avian and mammalian development, 1930–1940. Int. J. Dev. Biol. 2000, 44, 15–23. [Google Scholar]
Hamburger, V.; Hamilton, H.L. A series of normal stages in the development of the chick embryo. J. Morphol.1951, 88, 49–92. [Google Scholar] [CrossRef] [PubMed]
Lumsden, A.; Keynes, R. Segmental patterns of neuronal development in the chick hindbrain. Nature 1989, 337, 424–428. [Google Scholar] [CrossRef]
Jessell, T.M. Neuronal specification in the spinal cord: Inductive signals and transcriptional codes. Nat. Rev. Genet. 2000, 1, 20–92. [Google Scholar] [CrossRef]
Sonoda, E.; Morrison, C.; Yamashita, Y.M.; Takata, M.; Takeda, S. Reverse genetic studies of homologous DNA recombination using the chicken B-lymphocyte line, DT40. Philos. Trans. R. Soc. Lond. B Biol. Sci.2001, 356, 111–117. [Google Scholar] [CrossRef] [PubMed]
Le Douarin, N. The avian embryo as a model to study the development of the neural crest: A long and still ongoing story. Mech. Dev. 2004, 121, 1089–1102. [Google Scholar] [CrossRef]
Pourquie, O. The chick embryo: A leading model in somitogenesis studies. Mech. Dev. 2004, 121, 1069–1079. [Google Scholar] [CrossRef] [PubMed]
Yokouchi, Y. Establishment of a chick embryo model for analyzing liver development and a search for candidate genes. Dev. Growth Differ. 2005, 47, 357–366. [Google Scholar] [CrossRef]
Kohonen, P.; Nera, K.P.; Lassila, O. Avian model for B-cell immunology—New genomes and phylotranscriptomics. Scand. J. Immunol. 2007, 66, 113–121. [Google Scholar] [CrossRef]
Coleman, C.M. Chicken embryo as a model for regenerative medicine. Birth Defects Res. Part C Embryo Today 2008, 84, 245–256. [Google Scholar] [CrossRef]
Rashidi, H.; Sottile, V. The chick embryo: Hatching a model for contemporary biomedical research. BioEssays2009, 31, 459–465. [Google Scholar] [CrossRef]
Swanberg, S.E.; O’Hare, T.H.; Robb, E.A.; Robinson, C.M.; Chang, H.; Delany, M.E. Telomere biology of the chicken: A model for aging research. Exp. Gerontol. 2010, 45, 647–654. [Google Scholar] [CrossRef]
Datar, S.P.; Bhonde, R.R. Modeling chick to assess diabetes pathogenesis and treatment. Rev. Diabet. Stud.2011, 8, 245–253. [Google Scholar] [CrossRef] [PubMed]
Vergara, M.N.; Canto-Soler, M.V. Rediscovering the chick embryo as a model to study retinal development. Neural Dev. 2012, 7, 22. [Google Scholar] [CrossRef]
Nowak-Sliwinska, P.; Segura, T.; Iruela-Arispe, M.L. The chicken chorioallantoic membrane model in biology, medicine and bioengineering. Angiogenesis 2014, 17, 779–804. [Google Scholar] [CrossRef] [PubMed]
Bjørnstad, S.; Austdal, L.P.; Roald, B.; Glover, J.C.; Paulsen, R.E. Cracking the Egg: Potential of the Developing Chicken as a Model System for Nonclinical Safety Studies of Pharmaceuticals. J. Pharmacol. Exp. Ther. 2015, 355, 386–396. [Google Scholar] [CrossRef]
Cooper, M.D. The early history of B cells. Nat. Rev. Immunol. 2015, 15, 191–197. [Google Scholar] [CrossRef]
Bronner, M.E.; Simões-Costa, M. The Neural Crest Migrating into the Twenty-First Century. Curr. Top. Dev. Biol. 2016, 116, 115–134. [Google Scholar] [CrossRef] [PubMed]
Wisely, C.E.; Sayed, J.A.; Tamez, H.; Zelinka, C.; Abdel-Rahman, M.H.; Fischer, A.J.; Cebulla, C.M. The chick eye in vision research: An excellent model for the study of ocular disease. Prog. Retin. Eye Res. 2017, 61, 72–97. [Google Scholar] [CrossRef] [PubMed]
Davey, M.G.; Towers, M.; Vargesson, N.; Tickle, C. The chick limb: Embryology, genetics and teratology. Int. J. Dev. Biol. 2018, 62, 85–95. [Google Scholar] [CrossRef]
Janesick, A.S.; Heller, S. Stem Cells and the Bird Cochlea-Where Is Everybody? Cold Spring Harb. Perspect. Med. 2019, 9, a033183. [Google Scholar] [CrossRef]
Vilches-Moure, J.G. Embryonic Chicken (Gallus gallus domesticus) as a Model of Cardiac Biology and Development. Comp. Med. 2019, 69, 184–203. [Google Scholar] [CrossRef]
Bednarczyk, M.; Dunislawska, A.; Stadnicka, K.; Grochowska, E. Chicken embryo as a model in epigenetic research. Poult. Sci. 2021, 100, 101164. [Google Scholar] [CrossRef] [PubMed]
Wachholz, G.E.; Rengel, B.D.; Vargesson, N.; Fraga, L.R. From the Farm to the Lab: How Chicken Embryos Contribute to the Field of Teratology. Front. Genet. 2021, 12, 666726. [Google Scholar] [CrossRef]
Krumlauf, R.; Wilkinson, D.G. Segmentation and patterning of the vertebrate hindbrain. Development 2021, 148, dev186460. [Google Scholar] [CrossRef]
Tregaskes, C.A.; Kaufman, J. Chickens as a simple system for scientific discovery: The example of the MHC. Mol. Immunol. 2021, 135, 12–20. [Google Scholar] [CrossRef]
Hammarback, J.A.; Palm, S.L.; Furcht, L.T.; Letourneau, P.C. Guidance of neurite outgrowth by pathways of substratum-adsorbed laminin. J. Neurosci. Res. 1985, 13, 213–220. [Google Scholar] [CrossRef]
Walter, J.; Kern-Veits, B.; Huf, J.; Stolze, B.; Bonhoeffer, F. Recognition of position-specific properties of tectal cell membranes by retinal axons in vitro. Development 1987, 101, 685–696. [Google Scholar] [CrossRef]
Luo, Y.; Raible, D.; Raper, J.A. Collapsin: A protein in brain that induces the collapse and paralysis of neuronal growth cones. Cell 1993, 75, 217–227. [Google Scholar] [CrossRef]
Etchevers, H. Primary culture of chick, mouse or human neural crest cells. Nat. Protoc. 2011, 6, 1568–1577. [Google Scholar] [CrossRef] [PubMed]
Roycroft, A.; Mayor, R. Michael Abercrombie: Contact inhibition of locomotion and more. Int. J. Dev. Biol.2018, 62, 5–13. [Google Scholar] [CrossRef]
Costa, M.L.; Jurberg, A.D.; Mermelstein, C. The Role of Embryonic Chick Muscle Cell Culture in the Study of Skeletal Myogenesis. Front. Physiol. 2021, 12, 668600. [Google Scholar] [CrossRef] [PubMed]
Hoffman, S.; Sorkin, B.C.; White, P.C.; Brackenbury, R.; Mailhammer, R.; Rutishauser, U.; Cunningham, B.A.; Edelman, G.M. Chemical characterization of a neural cell adhesion molecule purified from embryonic brain membranes. J. Biol. Chem. 1982, 257, 7720–7729. [Google Scholar] [CrossRef]
Serafini, T.; Kennedy, T.E.; Galko, M.J.; Mirzayan, C.; Jessell, T.M.; Tessier-Lavigne, M. The netrins define a family of axon outgrowth-promoting proteins homologous to C. elegans UNC-6. Cell 1994, 78, 409–424. [Google Scholar] [CrossRef]
Drescher, U.; Kremoser, C.; Handwerker, C.; Löschinger, J.; Noda, M.; Bonhoeffer, F. In vitro guidance of retinal ganglion cell axons by RAGS, a 25 kDa tectal protein related to ligands for Eph receptor tyrosine kinases. Cell 1995, 82, 359–370. [Google Scholar] [CrossRef]
Schade, R.; Calzado, E.G.; Sarmiento, R.; Chacana, P.A.; Porankiewicz-Asplund, J.; Terzolo, H.R. Chicken egg yolk antibodies (IgY-technology): A review of progress in production and use in research and human and veterinary medicine. Altern. Lab. Anim. 2005, 33, 129–154. [Google Scholar] [CrossRef]
Stern, C.D. The chick embryo—Past, present and future as a model system in developmental biology. Mech. Dev. 2004, 121, 1011–1013. [Google Scholar] [CrossRef]
Bolker, J. Model organisms: There’s more to life than rats and flies. Nature 2012, 491, 31–33. [Google Scholar] [CrossRef] [PubMed]
Bolker, J.A. Animal Models in Translational Research: Rosetta Stone or Stumbling Block? Bioessays 2017, 39, 1700089. [Google Scholar] [CrossRef]
Haniffa, M.; Taylor, D.; Linnarsson, S.; Aronow, B.J.; Bader, G.D.; Barker, R.A.; Camara, P.G.; Gray Camp, J.; Chédotal, A.; Copp, A.; et al. A roadmap for the Human Developmental Cell Atlas. Nature 2021, 597, 196–205. [Google Scholar] [CrossRef] [PubMed]
International Chicken Genome Sequencing Consortium. Sequence and comparative analysis of the chicken genome provide unique perspectives on vertebrate evolution. Nature 2004, 432, 695–716. [Google Scholar] [CrossRef] [PubMed]
Cheng, Y.; Burt, D.W. Chicken genomics. Int. J. Dev. Biol. 2018, 62, 265–271. [Google Scholar] [CrossRef] [PubMed]
Jarvis, E.D. Perspectives from the Avian Phylogenomics Project: Questions that Can Be Answered with Sequencing All Genomes of a Vertebrate Class. Annu. Rev. Anim. Biosci. 2016, 4, 45–59. [Google Scholar] [CrossRef]
Feng, S.; Stiller, J.; Deng, Y.; Armstrong, J.; Fang, Q.; Hart Reeve, A.; Xie, D.; Chen, G.; Guo, C.; Faircloth, B.C.; et al. Dense sampling of bird diversity increases power of comparative genomics. Nature 2020, 587, 252–257. [Google Scholar] [CrossRef] [PubMed]
Nakamura, H.; Funahashi, J. Electroporation: Past, present and future. Dev. Growth Differ. 2013, 55, 15–19. [Google Scholar] [CrossRef]
Farzaneh, M.; Attari, F.; Mozdziak, P.E.; Khoshnam, S.E. The evolution of chicken stem cell culture methods. Br. Poult. Sci. 2017, 58, 681–686. [Google Scholar] [CrossRef] [PubMed]
Han, J.Y.; Park, Y.H. Primordial germ cell-mediated transgenesis and genome editing in birds. J. Anim. Sci. Biotechnol. 2018, 9, 19. [Google Scholar] [CrossRef] [PubMed]
Xiong, C.; Wang, M.; Ling, W.; Xie, D.; Chu, X.; Li, Y.; Huang, Y.; Li, T.; Otieno, E.; Qiu, X.; et al. Advances in Isolation and Culture of Chicken Embryonic Stem Cells in vitro. Cell Reprogram. 2020, 22, 43–54. [Google Scholar] [CrossRef]
Lee, J.; Kim, D.H.; Lee, K. Current Approaches and Applications in Avian Genome Editing. Int. J. Mol. Sci.2020, 21, 3937. [Google Scholar] [CrossRef]
Yamagata, M.; Sanes, J.R. Dscam and Sidekick proteins direct lamina-specific synaptic connections in vertebrate retina. Nature 2008, 451, 465–469. [Google Scholar] [CrossRef]
Takahashi, Y.; Watanabe, T.; Nakagawa, S.; Kawakami, K.; Sato, Y. Transposon-mediated stable integration and tetracycline-inducible expression of electroporated transgenes in chicken embryos. Methods Cell Biol.2008, 87, 271–280. [Google Scholar] [CrossRef]
Macdonald, J.; Taylor, L.; Sherman, A.; Kawakami, K.; Takahashi, Y.; Sang, H.M.; McGrew, M.J. Efficient genetic modification and germ-line transmission of primordial germ cells using piggyBac and Tol2 transposons. Proc. Natl. Acad. Sci. USA 2012, 109, 1466–1472. [Google Scholar] [CrossRef]
Morgan, B.A.; Fekete, D.M. Manipulating gene expression with replication-competent retroviruses. Methods Cell Biol. 1996, 51, 185–218. [Google Scholar] [CrossRef]
Leber, S.M.; Yamagata, M.; Sanes, J.R. Gene transfer using replication-defective retroviral and adenoviral vectors. Methods Cell Biol. 1996, 51, 161–183. [Google Scholar] [CrossRef] [PubMed]
Ishii, Y.; Reese, D.E.; Mikawa, T. Somatic transgenesis using retroviral vectors in the chicken embryo. Dev. Dyn. 2004, 229, 630–642. [Google Scholar] [CrossRef]
Hen, G.; Yosefi, S.; Shinder, D.; Or, A.; Mygdal, S.; Condiotti, R.; Galun, E.; Bor, A.; Sela-Donenfeld, D.; Friedman-Einat, M. Gene transfer to chicks using lentiviral vectors administered via the embryonic chorioallantoic membrane. PLoS ONE 2012, 7, e36531. [Google Scholar] [CrossRef]
Matsui, R.; Tanabe, Y.; Watanabe, D. Avian adeno-associated virus vector efficiently transduces neurons in the embryonic and post-embryonic chicken brain. PLoS ONE 2012, 7, e48730. [Google Scholar] [CrossRef] [PubMed]
Ito, T.; Ono, M.; Matsui, R.; Watanabe, D.; Ohmori, H. Avian adeno-associated virus as an anterograde transsynaptic vector. J. Neurosci. Methods 2021, 359, 109221. [Google Scholar] [CrossRef]
Sid, H.; Schusser, B. Applications of Gene Editing in Chickens: A New Era Is on the Horizon. Front. Genet.2018, 9, 456. [Google Scholar] [CrossRef]
Chojnacka-Puchta, L.; Sawicka, D. CRISPR/Cas9 gene editing in a chicken model: Current approaches and applications. J. Appl. Genet. 2020, 61, 221–229. [Google Scholar] [CrossRef]
Park, J.S.; Lee, K.Y.; Han, J.Y. Precise Genome Editing in Poultry and Its Application to Industries. Genes2020, 11, 1182. [Google Scholar] [CrossRef]
Yamagata, M.; Sanes, J.R. CRISPR-mediated Labeling of Cells in Chick Embryos Based on Selectively Expressed Genes. Bio-Protocol 2021, 11, e4105. [Google Scholar] [CrossRef] [PubMed]
Schermelleh, L.; Ferrand, A.; Huser, T.; Eggeling, C.; Sauer, M.; Biehlmaier, O.; Drummen, G.P.C. Super-resolution microscopy demystified. Nat. Cell Biol. 2019, 21, 72–84. [Google Scholar] [CrossRef]
Zhuang, X. Spatially resolved single-cell genomics and transcriptomics by imaging. Nat. Methods 2021, 18, 18–22. [Google Scholar] [CrossRef]
Stuart, T.; Satija, R. Integrative single-cell analysis. Nat. Rev. Genet. 2019, 20, 257–272. [Google Scholar] [CrossRef] [PubMed]
Tabula Muris Consortium. Single-cell transcriptomics of 20 mouse organs creates a Tabula Muris. Nature2018, 562, 367–372. [Google Scholar] [CrossRef] [PubMed]
Regev, A.; Teichmann, S.A.; Lander, E.S.; Amit, I.; Benoist, C.; Birney, E.; Bodenmiller, B.; Campbell, P.; Carninci, P.; Clatworthy, M.; et al. The Human Cell Atlas. eLife 2017, 6, e27041. [Google Scholar] [CrossRef] [PubMed]
Osumi-Sutherland, D.; Xu, C.; Keays, M.; Levine, A.P.; Kharchenko, P.V.; Regev, A.; Lein, E.; Teichmann, S.A. Cell type ontologies of the Human Cell Atlas. Nat. Cell Biol. 2021, 23, 1129–1135. [Google Scholar] [CrossRef]
The Tabula Sapiens Consortium. Tabula Sapiens: An Atlas of Single-Cell Gene Expression. Am. J. Med. Genet. A 2021, 185, 2857–2858. [Google Scholar] [CrossRef] [PubMed]
Hwang, B.; Lee, J.H.; Bang, D. Single-cell RNA sequencing technologies and bioinformatics pipelines. Exp. Mol. Med. 2018, 50, 1–14. [Google Scholar] [CrossRef]
Ding, J.; Adiconis, X.; Simmons, S.K.; Kowalczyk, M.S.; Hession, C.C.; Marjanovic, N.D.; Hughes, T.K.; Wadsworth, M.H.; Burks, T.; Nguyen, L.T.; et al. Systematic comparison of single-cell and single-nucleus RNA-sequencing methods. Nat. Biotechnol. 2020, 38, 737–746. [Google Scholar] [CrossRef] [PubMed]
Morrison, J.A.; McLennan, R.; Wolfe, L.A.; Gogol, M.M.; Meier, S.; McKinney, M.C.; Teddy, J.M.; Holmes, L.; Semerad, C.L.; Box, A.C.; et al. Single-cell transcriptome analysis of avian neural crest migration reveals signatures of invasion and molecular transitions. eLife 2017, 6, e28415. [Google Scholar] [CrossRef] [PubMed]
Vermillion, K.L.; Bacher, R.; Tannenbaum, A.P.; Swanson, S.; Jiang, P.; Chu, L.F.; Stewart, R.; Thomson, J.A.; Vereide, D.T. Spatial patterns of gene expression are unveiled in the chick primitive streak by ordering single-cell transcriptomes. Dev. Biol. 2018, 439, 30–41. [Google Scholar] [CrossRef]
Feregrino, C.; Sacher, F.; Parnas, O.; Tschopp, P. A single-cell transcriptomic atlas of the developing chicken limb. BMC Genom. 2019, 20, 401. [Google Scholar] [CrossRef]
Li, J.; Xing, S.; Zhao, G.; Zheng, M.; Yang, X.; Sun, J.; Wen, J.; Liu, R. Identification of diverse cell populations in skeletal muscles and biomarkers for intramuscular fat of chicken by single-cell RNA sequencing. BMC Genom. 2020, 21, 752. [Google Scholar] [CrossRef] [PubMed]
Tegla, M.G.G.; Buenaventura, D.F.; Kim, D.Y.; Thakurdin, C.; Gonzalez, K.C.; Emerson, M.M. OTX2 represses sister cell fate choices in the developing retina to promote photoreceptor specification. eLife 2020, 9, e54279. [Google Scholar] [CrossRef]
Hoang, T.; Wang, J.; Boyd, P.; Wang, F.; Santiago, C.; Jiang, L.; Yoo, S.; Lahne, M.; Todd, L.J.; Jia, M.; et al. Gene regulatory networks controlling vertebrate retinal regeneration. Science 2020, 370, eabb8598. [Google Scholar] [CrossRef]
Gandhi, S.; Hutchins, E.J.; Maruszko, K.; Park, J.H.; Thomson, M.; Bronner, M.E. Bimodal function of chromatin remodeler Hmga1 in neural crest induction and Wnt-dependent emigration. eLife 2020, 9, e57779. [Google Scholar] [CrossRef] [PubMed]
Estermann, M.A.; Williams, S.; Hirst, C.E.; Roly, Z.Y.; Serralbo, O.; Adhikari, D.; Powell, D.; Major, A.T.; Smith, C.A. Insights into Gonadal Sex Differentiation Provided by Single-Cell Transcriptomics in the Chicken Embryo. Cell Rep. 2020, 31, 107491. [Google Scholar] [CrossRef] [PubMed]
De Lima, J.E.; Blavet, C.; Bonnin, M.A.; Hirsinger, E.; Comai, G.; Yvernogeau, L.; Delfini, M.C.; Bellenger, L.; Mella, S.; Nassari, S.; et al. Unexpected contribution of fibroblasts to muscle lineage as a mechanism for limb muscle patterning. Nat. Commun. 2021, 12, 3851. [Google Scholar] [CrossRef]
Guillot, C.; Djeffal, Y.; Michaut, A.; Rabe, B.; Pourquié, O. Dynamics of primitive streak regression controls the fate of neuromesodermal progenitors in the chicken embryo. eLife 2021, 10, e64819. [Google Scholar] [CrossRef]
Janesick, A.; Scheibinger, M.; Benkafadar, N.; Kirti, S.; Ellwanger, D.C.; Heller, S. Cell-type identity of the avian cochlea. Cell Rep. 2021, 34, 108900. [Google Scholar] [CrossRef]
Dai, M.; Feng, M.; Li, Z.; Chen, W.; Liao, M. Chicken peripheral blood lymphocyte response to ALV-J infection assessed by single-cell RNA sequencing. bioRxiv 2021. [Google Scholar] [CrossRef]
Mantri, M.; Scuderi, G.J.; Abedini-Nassab, R.; Wang, M.F.Z.; McKellar, D.; Shi, H.; Grodner, B.; Butcher, J.T.; De Vlaminck, I. Spatiotemporal single-cell RNA sequencing of developing chicken hearts identifies interplay between cellular differentiation and morphogenesis. Nat. Commun. 2021, 12, 1771. [Google Scholar] [CrossRef]
Yamagata, M.; Yan, W.; Sanes, J.R. A cell atlas of the chick retina based on single-cell transcriptomics. eLife2021, 10, e63907. [Google Scholar] [CrossRef]
Zhang, J.; Lv, C.; Mo, C.; Liu, M.; Wan, Y.; Li, J.; Wang, Y. Single-cell RNA sequencing analysis of chicken anterior pituitary: A bird’s-eye view on vertebrate pituitary. Front. Physiol. 2021, 12, 562817. [Google Scholar] [CrossRef]
Cook, R.G. The comparative psychology of avian visual cognition. Curr. Dir. Psychol. Sci. 2000, 9, 83–89. [Google Scholar] [CrossRef]
Zhu, C.; Preissl, S.; Ren, B. Single-cell multimodal omics: The power of many. Nat. Methods 2020, 17, 11–14. [Google Scholar] [CrossRef]
Hao, Y.; Hao, S.; Andersen-Nissen, E.; Mauck, W.M., 3rd; Zheng, S.; Butler, A.; Lee, M.J.; Wilk, A.J.; Darby, C.; Zager, M.; et al. Integrated analysis of multimodal single-cell data. Cell 2021, 184, 3573–3587. [Google Scholar] [CrossRef] [PubMed]
Lareau, C.A.; Duarte, F.M.; Chew, J.G.; Kartha, V.K.; Burkett, Z.D.; Kohlway, A.S.; Pokholok, D.; Aryee, M.J.; Steemers, F.J.; Lebofsky, R.; et al. Droplet-based combinatorial indexing for massive-scale single-cell chromatin accessibility. Nat. Biotechnol. 2019, 37, 916–924. [Google Scholar] [CrossRef] [PubMed]
Stoeckius, M.; Hafemeister, C.; Stephenson, W.; Houck-Loomis, B.; Chattopadhyay, P.K.; Swerdlow, H.; Satija, R.; Smibert, P. Simultaneous epitope and transcriptome measurement in single cells. Nat. Methods2017, 14, 865–868. [Google Scholar] [CrossRef]
Peterson, V.M.; Zhang, K.X.; Kumar, N.; Wong, J.; Li, L.; Wilson, D.C.; Moore, R.; McClanahan, T.K.; Sadekova, S.; Klappenbach, J.A. Multiplexed quantification of proteins and transcripts in single cells. Nat. Biotechnol. 2017, 35, 936–939. [Google Scholar] [CrossRef] [PubMed]
Stoeckius, M.; Zheng, S.; Houck-Loomis, B.; Hao, S.; Yeung, B.Z.; Mauck, W.M., 3rd; Smibert, P.; Satija, R. Cell Hashing with barcoded antibodies enables multiplexing and doublet detection for single cell genomics. Genome Biol. 2018, 19, 224. [Google Scholar] [CrossRef] [PubMed]
Mair, F.; Erickson, J.R.; Voillet, V.; Simoni, Y.; Bi, T.; Tyznik, A.J.; Martin, J.; Gottardo, R.; Newell, E.W.; Prlic, M. A Targeted Multi-omic Analysis Approach Measures Protein Expression and Low-Abundance Transcripts on the Single-Cell Level. Cell Rep. 2020, 31, 107499. [Google Scholar] [CrossRef]
Kearney, C.J.; Vervoort, S.J.; Ramsbottom, K.M.; Todorovski, I.; Lelliott, E.J.; Zethoven, M.; Pijpers, L.; Martin, B.P.; Semple, T.; Martelotto, L.; et al. SUGAR-seq enables simultaneous detection of glycans, epitopes, and the transcriptome in single cells. Sci. Adv. 2021, 7, eabe3610. [Google Scholar] [CrossRef] [PubMed]
Lipovsek, M.; Bardy, C.; Cadwell, C.R.; Hadley, K.; Kobak, D.; Tripathy, S.J. Patch-seq: Past, Present, and Future. J. Neurosci. 2021, 41, 937–946. [Google Scholar] [CrossRef]
Sun, Y.C.; Chen, X.; Fischer, S.; Lu, S.; Zhan, H.; Gillis, J.; Zador, A.M. Integrating barcoded neuroanatomy with spatial transcriptional profiling enables identification of gene correlates of projections. Nat. Neurosci.2021, 24, 873–885. [Google Scholar] [CrossRef] [PubMed]
Larsson, L.; Frisén, J.; Lundeberg, J. Spatially resolved transcriptomics adds a new dimension to genomics. Nat. Methods 2021, 18, 15–18. [Google Scholar] [CrossRef] [PubMed]
Zhang, M.; Eichhorn, S.W.; Zingg, B.; Yao, Z.; Cotter, K.; Zeng, H.; Dong, H.; Zhuang, X. Spatially resolved cell atlas of the mouse primary motor cortex by MERFISH. Nature 2021, 598, 137–143. [Google Scholar] [CrossRef]
Eng, C.L.; Lawson, M.; Zhu, Q.; Dries, R.; Koulena, N.; Takei, Y.; Yun, J.; Cronin, C.; Karp, C.; Yuan, G.C.; et al. Transcriptome-scale super-resolved imaging in tissues by RNA seqFISH. Nature 2019, 568, 235–239. [Google Scholar] [CrossRef]
Rodriques, S.G.; Stickels, R.R.; Goeva, A.; Martin, C.A.; Murray, E.; Vanderburg, C.R.; Welch, J.; Chen, L.M.; Chen, F.; Macosko, E.Z. Slide-seq: A scalable technology for measuring genome-wide expression at high spatial resolution. Science 2019, 363, 1463–1467. [Google Scholar] [CrossRef]
Rood, J.E.; Stuart, T.; Ghazanfar, S.; Biancalani, T.; Fisher, E.; Butler, A.; Hupalowska, A.; Gaffney, L.; Mauck, W.; Eraslan, G.; et al. Toward a Common Coordinate Framework for the Human Body. Cell 2019, 179, 1455–1467. [Google Scholar] [CrossRef]
Wang, Q.; Ding, S.L.; Li, Y.; Royall, J.; Feng, D.; Lesnar, P.; Graddis, N.; Naeemi, M.; Facer, B.; Ho, A.; et al. The Allen Mouse Brain Common Coordinate Framework: A 3D Reference Atlas. Cell 2020, 181, 936–953. [Google Scholar] [CrossRef]
Cherf, G.M.; Cochran, J.R. Applications of Yeast Surface Display for Protein Engineering. Methods Mol. Biol.2015, 1319, 155–175. [Google Scholar] [CrossRef]
Almagro, J.C.; Pedraza-Escalona, M.; Arrieta, H.I.; Pérez-Tapia, S.M. Phage Display Libraries for Antibody Therapeutic Discovery and Development. Antibodies 2019, 8, 44. [Google Scholar] [CrossRef] [PubMed]
Tombácz, I.; Weissman, D.; Pardi, N. Vaccination with Messenger RNA: A Promising Alternative to DNA Vaccination. Methods Mol. Biol. 2021, 2197, 13–31. [Google Scholar] [CrossRef] [PubMed]
Vieites-Prado, A.; Renier, N. Tissue clearing and 3D imaging in developmental biology. Development 2021, 148, dev199369. [Google Scholar] [CrossRef]
Hallou, A.; Yevick, H.G.; Dumitrascu, B.; Uhlmann, V. Deep learning for bioimage analysis in developmental biology. Development 2021, 148, dev199616. [Google Scholar] [CrossRef] [PubMed]
Luo, L.; Callaway, E.M.; Svoboda, K. Genetic Dissection of Neural Circuits: A Decade of Progress. Neuron2018, 98, 256–281. [Google Scholar] [CrossRef]
Davey, M.G.; Balic, A.; Rainger, J.; Sang, H.M.; McGrew, M.J. Illuminating the chicken model through genetic modification. Int. J. Dev. Biol. 2018, 62, 257–264. [Google Scholar] [CrossRef]
Joesch, M.; Mankus, D.; Yamagata, M.; Shahbazi, A.; Schalek, R.; Suissa-Peleg, A.; Meister, M.; Lichtman, J.W.; Scheirer, W.J.; Sanes, J.R. Reconstruction of genetically identified neurons imaged by serial-section electron microscopy. eLife 2016, 5, e15015. [Google Scholar] [CrossRef]
Wolf, S.; Wan, Y.; McDole, K. Current approaches to fate mapping and lineage tracing using image data. Development 2021, 148, dev198994. [Google Scholar] [CrossRef]
Gray, G.E.; Leber, S.M.; Sanes, J.R. Migratory patterns of clonally related cells in the developing central nervous system. Experientia 1990, 46, 929–940. [Google Scholar] [CrossRef]
Woodworth, M.B.; Girskis, K.M.; Walsh, C.A. Building a lineage from single cells: Genetic techniques for cell lineage tracking. Nat. Rev. Genet. 2017, 18, 230–244. [Google Scholar] [CrossRef]
Kester, L.; van Oudenaarden, A. Single-Cell Transcriptomics Meets Lineage Tracing. Cell Stem Cell 2018, 23, 166–179. [Google Scholar] [CrossRef]
Wagner, D.E.; Klein, A.M. Lineage tracing meets single-cell omics: Opportunities and challenges. Nat. Rev. Genet. 2020, 21, 410–427. [Google Scholar] [CrossRef]
VanHorn, S.; Morris, S.A. Next-Generation Lineage Tracing and Fate Mapping to Interrogate Development. Dev. Cell 2021, 56, 7–21. [Google Scholar] [CrossRef] [PubMed]
La Manno, G.; Soldatov, R.; Zeisel, A.; Braun, E.; Hochgerner, H.; Petukhov, V.; Lidschreiber, K.; Kastriti, M.E.; Lönnerberg, P.; Furlan, A.; et al. RNA velocity of single cells. Nature 2018, 560, 494–498. [Google Scholar] [CrossRef] [PubMed]
Alemany, A.; Florescu, M.; Baron, C.S.; Peterson-Maduro, J.; van Oudenaarden, A. Whole-organism clone tracing using single-cell sequencing. Nature 2018, 556, 108–112. [Google Scholar] [CrossRef] [PubMed]
Raj, B.; Wagner, D.E.; McKenna, A.; Pandey, S.; Klein, A.M.; Shendure, J.; Gagnon, J.A.; Schier, A.F. Simultaneous single-cell profiling of lineages and cell types in the vertebrate brain. Nat. Biotechnol. 2018, 36, 442–450. [Google Scholar] [CrossRef]
Chow, K.K.; Budde, M.W.; Granados, A.A.; Cabrera, M.; Yoon, S.; Cho, S.; Huang, T.H.; Koulena, N.; Frieda, K.L.; Cai, L.; et al. Imaging cell lineage with a synthetic digital recording system. Science 2021, 372, eabb3099. [Google Scholar] [CrossRef]
Efremova, M.; Vento-Tormo, M.; Teichmann, S.A.; Vento-Tormo, R. CellPhoneDB: Inferring cell-cell communication from combined expression of multi-subunit ligand-receptor complexes. Nat. Protoc. 2020, 15, 1484–1506. [Google Scholar] [CrossRef]
Arendt, D.; Bertucci, P.Y.; Achim, K.; Musser, J.M. Evolution of neuronal types and families. Curr. Opin. Neurobiol. 2019, 56, 144–152. [Google Scholar] [CrossRef]
Yuste, R.; Hawrylycz, M.; Aalling, N.; Aguilar-Valles, A.; Arendt, D.; Armañanzas, R.; Ascoli, G.A.; Bielza, C.; Bokharaie, V.; Bergmann, T.B.; et al. A community-based transcriptomics classification and nomenclature of neocortical cell types. Nat. Neurosci. 2020, 23, 1456–1468. [Google Scholar] [CrossRef]
Tosches, M.A. From Cell Types to an Integrated Understanding of Brain Evolution: The Case of the Cerebral Cortex. Annu. Rev. Cell Dev. Biol. 2021, 37, 495–517. [Google Scholar] [CrossRef]
BRAIN Initiative Cell Census Network (BICCN). A multimodal cell census and atlas of the mammalian primary motor cortex. Nature 2021, 598, 86–102. [Google Scholar] [CrossRef] [PubMed]
Arendt, D.; Musser, J.M.; Baker, C.V.H.; Bergman, A.; Cepko, C.; Erwin, D.H.; Pavlicev, M.; Schlosser, G.; Widder, S.; Laubichler, M.D.; et al. The origin and evolution of cell types. Nat. Rev. Genet. 2016, 17, 744–757. [Google Scholar] [CrossRef] [PubMed]
Shafer, M.E.R. Cross-Species Analysis of Single-Cell Transcriptomic Data. Front. Cell Dev. Biol. 2019, 7, 175. [Google Scholar] [CrossRef]
Tanay, A.; Sebé-Pedrós, A. Evolutionary cell type mapping with single-cell genomics. Trends Genet. 2021, 37, 919–932. [Google Scholar] [CrossRef]
Tarashansky, A.J.; Musser, J.M.; Khariton, M.; Li, P.; Arendt, D.; Quake, S.R.; Wang, B. Mapping single-cell atlases throughout Metazoa unravels cell type evolution. eLife 2021, 10, e66747. [Google Scholar] [CrossRef]
Levine, M.; Davidson, E.H. Gene regulatory networks for development. Proc. Natl. Acad. Sci. USA 2005, 102, 4936–4942. [Google Scholar] [CrossRef]
Arendt, D. The evolution of cell types in animals: Emerging principles from molecular studies. Nat. Rev. Genet. 2008, 9, 868–882. [Google Scholar] [CrossRef] [PubMed]
Tosches, M.A.; Yamawaki, T.M.; Naumann, R.K.; Jacobi, A.A.; Tushev, G.; Laurent, G. Evolution of pallium, hippocampus, and cortical cell types revealed by single-cell transcriptomics in reptiles. Science 2018, 360, 881–888. [Google Scholar] [CrossRef]
Emery, N.J. Cognitive ornithology: The evolution of avian intelligence. Philos. Trans. R. Soc. Lond. B Biol. Sci.2006, 361, 23–43. [Google Scholar] [CrossRef] [PubMed]
Mooney, R. Neural mechanisms for learned birdsong. Learn. Mem. 2009, 16, 655–669. [Google Scholar] [CrossRef] [PubMed]
Güntürkün, O.; Bugnyar, T. Cognition without Cortex. Trends Cogn. Sci. 2016, 20, 291–303. [Google Scholar] [CrossRef] [PubMed]
この記事が気に入ったらサポートをしてみませんか?