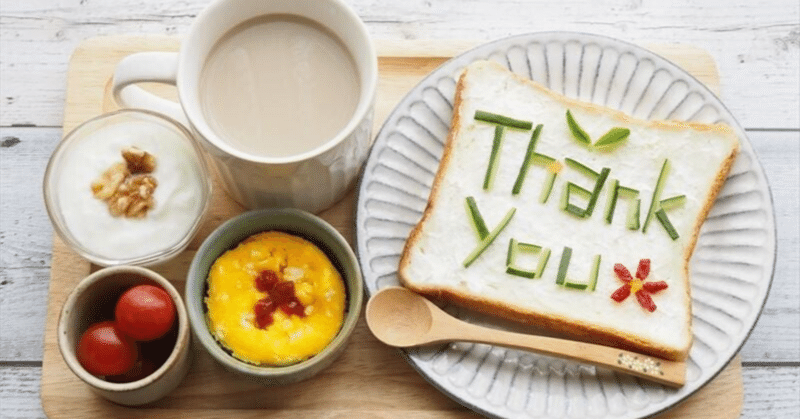
【構造変化のポイント💐】「The Effects of Japanese Foreign Exchange Intervention: GARCH Estimation and Change Point Detection」:先行研究解説 No.12 2023/09/27
Introduction:卒業論文は早めに仕上げたい💛
私もいよいよ卒業論文の執筆に
取りかかる時期がやって参りました👍
何事もアウトプット前提のインプットが
大事であると、noteで毎日発信してきました
これは、どのような内容で
あっても当てはまります👍
論文を一概に読んでも
記憶に残っていなかったり
大切な観点を忘れてしまっていたりしたら
卒業論文の進捗は滞ってしまうと思います
だからこそ、この「note」をフル活用して
卒業論文を1%でも
完成に向けて進めていきたいと思います
私の卒論執筆への軌跡を
どうぞご愛読ください📖
今回の参考文献🔥
今回、読み進めていく論文は
こちらのURLになります👍
『The Effects of Japanese Foreign Exchange Intervention: GARCH Estimation and Change Point Detection』
Eric Hillebrand Gunther Schnabl Discussion
Paper No.6 October 2003
読み終えた先行研究📚
『日本の為替介入の分析』 伊藤隆敏・著
経済研究 Vol.54 No.2 Apr. 2003
『Effects of the Bank of Japan’s intervention on yen/dollar exchange rate volatility』21 November 2004
Toshiaki Watanabe (a), Kimie Harada(b)
前回のお復習い🔖
The Effects of Japanese Foreign Exchange Intervention: GARCH Estimation and Change Point Detection
Eric Hillebrand Department of Economics, Louisiana State University
Gunther Schnabl Department of Economics and Business Administration, Tuebingen
5. Change Point Detection
Although the sub-divided GARCH estimations give a more precise view of changing parameter regimes in comparison to the global model, the segmentations might still be criticized as being arbitrary.
We use a change point detector for ARCH models as proposed by Kokoszka and Leipus (2000) to identify sub-periods on a more systematic basis.
Further, the change point detector provides us with an alternative approach to match increased volatility with intervention periods.
より細分化されたGARCHモデルを使用した推定値は、グローバルな推計モデルと比較して、パラメーター・レジームの変化をより正確に把握できますが、それでもなお、その分割は恣意的であると批判される可能性があります💦
Kokoszka と Leipus (2000) によって提案されたARCHモデルの変化構造転換点の検知方法(the change point detector)を使用して、より体系的にサブ期間を特定します
さらに、この構造転換点の検出手法(the change point detector)は、ボラティリティの増加と介入期間を一致させるための代替アプローチを提供します
5.1. Specification
The change point detector identifies changes in the data-generating parameters of a time series.
Change point detection has a long-standing tradition in the quality control of production processes (Lai 1995) and has also been applied to financial time series (Kokoszka and Leipus 2000).
We will base the change point detection on a standard GARCH(1,1) model with constant mean return:
構造転換点の検出手法は、時系列のデータ生成パラメーターの変化を識別します👏
変化点検出には、生産プロセスの品質管理において長年の伝統があり (Lai 1995)、この伝統的な手法が、金融時系列にも適用されています (Kokoszka および Leipus 2000)
変化点の検出は、平均リターンが一定の標準的なGARCH(1,1)モデルに基づいて実施されます👍
$$
\\
The Change Point Detection\\
r_t = \mu +\epsilon_t \cdot\cdot\cdot(7)\\ \\\epsilon_t|_{\Omega_{t-1}} \backsim N(0,h_t) \cdot\cdot\cdot(8)\\ \\h_t = \omega + \alpha \epsilon_{t-1}^2+\beta h_{t-1}\cdot\cdot\cdot(9)
$$
In equation (7), rt are the daily returns of the yen/dollar exchange rate, µ is the constant mean. The disturbances εt are assumed to be normally distributed conditional on the information Ωt-1 available at the time t-1 (8).
The mean of the disturbances is assumed to be zero and the variance ht depends on the square of the lagged disturbance of the previous period εt-1 and the variance of the previous period ht-1 (9).
式(7)において、rt は円/ドル為替レートの1日当たりの収益、μ は定数平均になります
誤差項εtは、時間 t-1 で利用可能な情報 Ωt-1 を条件として正規分布すると仮定されます (8)
誤差項の平均は、ゼロであると仮定され、分散 ht は前の期間の遅れた誤差項 εt-1 と前の期間の分散 ht-1 の二乗に依存するとします (9)
Let k* denote a single change point in our time series generated by the GARCH model of equation (7) to (9).
At k* the data generating parameter vector changes from θ1 =(ω1,α1, β1) to θ2 =(ω2,α2,β2).
In other words, the change point detector identifies segments of sufficiently different volatility means.28
The change point detector is the estimator k^of k* defined by
28) In the stationary GARCH(1,1) model, the volatility mean is given by Eht= Eεt^2 = ω /(1−α−β) (Bollerslev 1986).
k*を式(7)~(9)から構成されるGARCHモデルによって生成された時系列内の単一の変化点を表すものとします👍
k* では、データ生成パラメータベクトルが θ1 =(ω1,α1, β1) から θ2 =(ω2,α2,β2) に変化します
換言すれば、変化点の検出は、大いに異なるボラティリティ変動率平均のセグメントを識別するということです
なお、注28より、定常的なGARCH(1,1) モデルでは、ボラティリティ平均は Eht= Eεt^2 = ω /(1−α−β) で与えられます (Bollerslev 1986)
そして、構造変化点の検出は、次のように定義される推定量 k^の k*となります
$$
\\The Definition of Change Point Detector\\ \\\hat{k}\equiv min \{ k:|R_k| =\displaystyle\max_{1 \le j \le n}|R_j|\}\cdot\cdot\cdot (10)
$$
where k and j are indices for time, and the statistic Rk is given by
ここで、k と j は時間のインデックスであり、統計量(R_k)は次の式で与えられます✨
$$
\\
The Statistic R_k\\ \\{\large R_k} = {\large\frac{k(n-k)}{n^2}} \Big( {\large\frac{1}{k}} \displaystyle\sum_{j=1}^k r^2_j-\frac{1}{n-k}\displaystyle\sum_{j=k+1}^n r^2_j\Big)\cdot\cdot\cdot(11)
$$
Intuitively, the detector measures the distance Rk between the means of the two segments that are induced by the hypothetical change point k.
The estimated change point is set where this distance becomes maximal. For the rare case that more than one maximum exists, the first one is chosen.
Kokoszka and Leipus (2000) show that this estimator is consistent and converges in probability to the true change point k* with rate 1/n.
直感的に考えてみても、構造転換点の検出は、仮想の変化点kによって引き起こされる2つのセグメントの平均間の距離Rkを測定しているということです👍
また、この点同士の距離が最大となる点を推定変化点とすることにしましょう
まれに複数の最大値が存在する場合は、最初の最大値が選択されることになります📝
なお、KokoszkaとLeipus(2000)は、この推定量が一貫しており、ト1/nの比率で真の変化点 k*に確率的に収束することを示しています
We approach the multi-change point problem of finding a segmentation of the yen/dollar exchange rate series as a sequential single-change point detection problem.
The observation period is sub-divided into an increasing number of sub-periods, where the change points mark the boundaries.
First, the change point detector is applied to the whole exchange rate series, which yields one change point and two sub-series.
The detector then is applied to both sub-series, increasing the number of change points to three and the segments to four and so on.
円/ドル為替レート系列の分割を見つける複数個の構造変化点問題を、逐次的な単一変化点の検出問題としてアプローチします
観察期間は、増加するサブ期間につれて分割され、変化点が境界を示しています
まず、構造変化点の検出が為替レート系列全体に適用され、1 つの変化点と2つのサブ系列が生成されます
次に、構造変化点の検出が両方のサブシリーズに適用され、変化点の数が3つ、セグメントが4つなどに増加していくことになります
The starting point of our GARCH estimations performed in section 4 was set by the publication of Japanese foreign exchange intervention data starting from April 1, 1991.
To obtain a less arbitrary starting point we extended the observation period to about 20 years starting in October 1983. The change point detection progresses in five steps and thereby theoretically sets 31 change points.
We can then choose the change point closest to April 1991 as starting point of the GARCH estimation and re-estimate our model for the new subperiods.
セクション4で実行されたGARCH推定の開始点は、1991年4月1日から始まる日本の為替介入データの公表によって設定されました📝
より恣意的な開始点を取得するために、観察期間を1983年10 月から約20年間に延長しました
すると、変化点の検出は、5段階に分かれており、理論的には31個の変化点が設定されることになったのです
次に、1991年4月に最も近い変化点をGARCH推定の開始点として選択し、新しいサブ期間のモデルを再推定することができるのです
本日の解説は、ここまでとします
このような歴史や先行研究をしっかり理解した上で、卒業論文執筆に取り組んでいきたいです
今回、私が卒業論文執筆において取り上げる
24年ぶりの「円安是正」介入は本当にレアな経済政策
ということを再認識できたような気がします💖
私の研究テーマについて🔖
私は「為替介入の実証分析」をテーマに
卒業論文を執筆しようと考えています📝
日本経済を考えたときに、為替レートによって
貿易取引や経常収支が変化したり
株や証券、債権といった金融資産の収益率が
変化したりと日本経済と為替レートとは
切っても切れない縁があるのです💝
(円💴だけに・・・)
経済ショックによって
為替レートが変化すると
その影響は私たちの生活に大きく影響します
だからこそ、為替レートの安定性を
担保するような為替介入はマクロ経済政策に
おいても非常に重要な意義を持っていると
推測しています
決して学部生が楽して執筆できる
簡単なテーマを選択しているわけでは無いと信じています
ただ、この卒業論文をやり切ることが
私の学生生活の集大成となることは事実なので
最後までコツコツと取り組んで参ります🔥
本日の解説は、以上とします📝
今後も経済学理論集ならびに
社会課題に対する経済学的視点による説明など
有意義な内容を発信できるように
努めてまいりますので
今後とも宜しくお願いします🥺
マガジンのご紹介🔔
こちらのマガジンにて
卒業論文執筆への軌跡
エッセンシャル経済学理論集、ならびに
【国際経済学🌏】の基礎理論をまとめています
今後、さらにコンテンツを拡充できるように努めて参りますので何卒よろしくお願い申し上げます📚
最後までご愛読いただき誠に有難うございました!
あくまで、私の見解や思ったことを
まとめさせていただいてますが
その点に関しまして、ご了承ください🙏
この投稿をみてくださった方が
ほんの小さな事でも学びがあった!
考え方の引き出しが増えた!
読書から学べることが多い!
などなど、プラスの収穫があったのであれば
大変嬉しく思いますし、投稿作成の冥利に尽きます!!
お気軽にコメント、いいね「スキ」💖
そして、お差し支えなければ
フォロー&シェアをお願いしたいです👍
今後とも何卒よろしくお願いいたします!
この記事が気に入ったらサポートをしてみませんか?