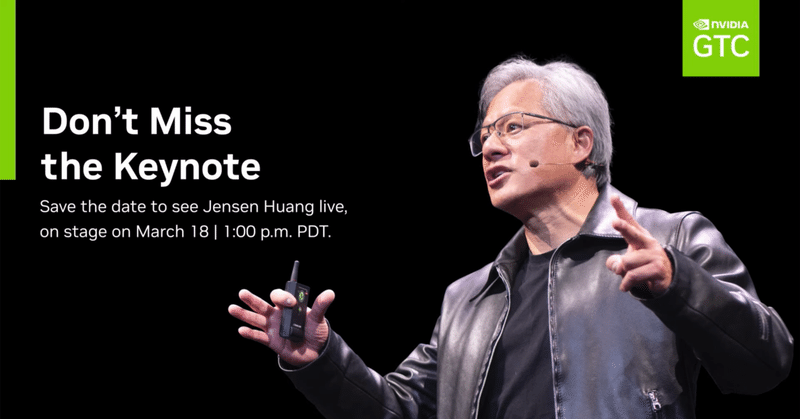
【GTC2024】Jensen Huang’s GTC keynote(翻訳)
本Noteは自身の頭を整理するために作成しており、
記載内容に関しては、自己責任で参照して頂きたいと思います。
上記、日本時間3/19 5:00からの約2時間半の動画を
下記Noteを参考とし、文字起こしを実施したものとなります。
まず、上記Noteを基に英文を抽出し、
抽出した英文をDeepLのAPIを活用し日本語訳したものが下記となります。
動画と本文記載内容の正誤を照合しておりませんので
御了承の程、宜しくお願いします。
Live全文:日本語訳
NVIDIA創業者兼CEOのジェンセン・ウォンさんのご登壇を歓迎します。
GTCへようこそ。
これはコンサートではありません。
皆さんは開発者会議に到着したのです。
多くの科学、アルゴリズム、コンピュータアーキテクチャ、
数学が説明されます。
私は突然、部屋の中にとても重いものを感じました。
世界でも類を見ない会議だ。
これほど多様な科学分野の研究者が一堂に会する会議があるだろうか?
気候技術から電波科学まで、
AIを使って次世代6G無線のMIMOをロボット制御する方法を解明しようとしている。ロボットの自動運転車。人工知能も。
誰もが...まず、私はそこに突然の安堵感を覚えた。
また、この会議には素晴らしい企業が参加しています。
このリストは出席者ではありません。
発表者たちです。
そして驚くべきはこれだ。
私の友人たち、親しい友人たちを除いてみると、
IT業界のマイケル・デルがそこに座っている。
IT業界で一緒に育った友人たち。
そのリストを取り除くと、驚くべきことがわかる。
通常のコンピューターでは解決できない問題を解決するためにアクセラレーテッド・コンピューティングを利用している非IT業界のプレゼンターたちです。
ライフサイエンス、ヘルスケア、ゲノミクス、輸送、もちろん小売、物流、製造、産業など、その業種は実に多岐にわたる。
そして、皆さんは出席するためだけにここにいるのではなく、
自分の研究について発表するためにここにいるのです。
世界の100兆ドルもの産業が、今日この部屋に集まっています。
これは本当に驚くべきことです。何かが起こっている。
私たちの業界だけでなく、産業界が変容しているのです。
コンピュータ産業は、
コンピュータは今日の社会で最も重要な道具であり、
コンピューティングにおける根本的な変革は
あらゆる産業に影響を与えます。
しかし、私たちはどのようにスタートしたのでしょうか?
どうやってここまで来たのか?
私はあなたのために小さな漫画を作りました。
文字通り、私が描いたものです。
1ページで、エヌビディアの軌跡を描いています。
1993年に始まり、これが残りの話になるかもしれません。
1993年、これが私たちの旅です。
私たちは1993年に設立されました。
その過程でいくつかの重要な出来事がありました。
いくつか紹介しましょう。
2006年、CUDAは革命的なコンピューティング・モデルであることが判明しました。
一夜にして大成功を収めようとしていたのですが、
それから約20年後にそれが実現したのです。
私たちはそれを予見していたのです。
それから20年後。2012年、AlexNet、AI、CUDAが初めて接触した。
2016年、私たちはこのコンピューティング・モデルの重要性を認識し、まったく新しいタイプのコンピュータを発明しました。
私たちはそれをDGX-1と呼びました。
170テラフロップスのスーパーコンピューターです。
8つのGPUが初めて一緒に接続されました。
私はサンフランシスコにあるOpenAIというスタートアップに
最初のDGX1を手渡しました。
DGX1は世界初のAIスーパーコンピューターだった。
170テラフロップスを覚えている。
2017年、トランスフォーマーが登場。
2022年、チャットGPTが世界の想像力をかき立て、
人々に人工知能の重要性と能力を認識させた。
そして2023年、ジェネレーティブAIが登場し、新しい産業が始まる。
なぜ?なぜ新しい産業なのか?
なぜなら、
ソフトウェアはこれまで存在しなかったからだ。
私たちは今、ソフトウェアを生産し、
ソフトウェアを書くためにコンピューターを使い、
かつて存在しなかったソフトウェアを生産している。
まったく新しいカテゴリーだ。
何もないところからシェアを奪った。
まったく新しいカテゴリーなのです。
そして、ソフトウェアを生産する方法は、
これまでにないものです。
データセンターでトークンを生成し、
非常に大規模な浮動小数点数を生成する。
まるでこの最後の産業革命の始まりのように、人々は工場を設立し、
そこにエネルギーを投入することに気づいた。
そして電気という目に見えない貴重なものが生まれた。
交流発電機だ。
そして100年後、200年後の今、私たちは新しいタイプの電子、トークンを生み出し、工場と呼ばれるインフラ、AI工場を使って、人工知能という新しい、信じられないほど価値のあるものを生み出している。
新しい産業が出現したのだ。
さて...この新しい産業について、いろいろなことをお話ししましょう。
次にどのようにコンピューティングを行うかについて話すつもりだ。
この新しい産業、新しいソフトウェア、
この新しいソフトウェアについてどう考えるか。
この新しい産業におけるアプリケーションはどうでしょうか?
そして、次に何が起こるのか、そして次に起ころうとしていることに備えて、今日からどのように準備を始めればいいのか。さて、その前に、コンピュータグラフィックス、物理学、人工知能が交差し、すべてがコンピュータの中で、オムニバースの中で、仮想世界のシミュレーションの中で交差する、エヌビディアの魂、私たちの会社の魂をお見せしたいと思います。
今日お見せするのは、文字通りすべてシミュレーションであり、アニメーションではありません。物理学だから美しいのだ。
世界は美しい。
ロボット工学によってアニメーション化されているから素晴らしいだけだ。人工知能によってアニメーション化されている。
あなたが今日一日中見ようとしているものは、
完全に生成されたものであり、完全にシミュレートされたものであり、
オムニバースであり、
そのすべて、あなたがこれから楽しもうとしているものは、
すべてが自家製である世界初のコンサートなのです。
すべてが自家製なのだ。
これからホームビデオを見ることになる。
さあ、座って楽しんでください。
ありがとう。
加速コンピューティングは転換点を迎えている。
汎用コンピューティングは息切れした。
私たちは、コンピューティングの規模を拡大し続け、
コンピューティングのコストを下げ続け、持続可能でありながら、
より多くのコンピューティングを消費し続けることができるように、
コンピューティングを行う別の方法を必要としています。
アクセラレーテッド・コンピューティングは、
汎用コンピューティングを劇的に高速化します。
そして、私たちが関わるすべての産業において、
そしてこれからお見せする多くの産業において、その影響は劇的です。
しかし、私たちの業界ほど重要な業界はありません。
シミュレーションツールを使って製品を作る業界です。
この業界では、コンピューティングのコストを下げることが重要なのではありません。
コンピューティングの規模を拡大することなのです。
私たちは、私たちが行う製品全体を完全に忠実に、
完全にデジタルで、基本的にはデジタルツインと呼ばれるものでシミュレーションできるようになりたいと考えています。
設計、製造、シミュレーション、運用を完全にデジタルで行いたい。
そのためには、業界全体を加速させる必要がある。
そして今日、私たちのエコシステム全体を加速させ、
世界をアクセラレーテッド・コンピューティングへと導くために、
この旅に参加してくれるパートナーがいることを発表したいと思います。
しかし、ボーナスもあります。
アクセラレーションが実現すれば、インフラはCUDA GPUになります。
そうなれば、
ジェネレーティブAIのためのインフラとまったく同じになります。
ですから、
いくつかの非常に重要なパートナーシップを発表できることを嬉しく思っています。
世界で最も重要な企業です。
ANSYSは、世界中で製造されている製品のエンジニアリング・シミュレーションを行っています。
私たちは彼らと提携し、ANSYSのエコシステムをCUDAで加速し、
ANSYSをオムニバース・デジタル・ツインに接続します。
素晴らしいことです。
本当に素晴らしいのは、
NVIDIA GPUアクセラレーション・システムのインストール・ベースが世界中にあり、あらゆるクラウド、あらゆるシステム、あらゆる企業にあるということです。
そのため、彼らがアクセラレートするアプリケーションは、
巨大なインストールベースを持つことになります。
エンドユーザーは素晴らしいアプリケーションを手に入れることができる。もちろん、システム・メーカーやCSPも大きな顧客需要を持つことになる。
シノプシス。
シノプシスは、NVIDIAにとって文字通り
最初のソフトウェア・パートナーだ。
シノプシスは当社の設立当初から存在していました。
シノプシスは高位設計でチップ業界に革命を起こしました。
私たちはCUDAでシノプシスを加速しようとしています。
私たちは、
これまで誰も知らなかった最も重要なアプリケーションの1つであるコンピュテーショナル・リソグラフィーを加速します。
チップを作るためには、
リソグラフィーを限界まで高める必要があります。
NVIDIAは、コンピュテーショナル・リソグラフィを信じられないほど加速するライブラリ、ドメイン固有のライブラリを作成しました。
TSMCは本日、NVIDIA Koolithoを使った生産を開始すると発表しました。
ソフトウェアで定義され、加速されれば、次のステップは、半導体製造の未来にジェネレーティブAIを適用し、ジオメトリをさらに押し進めることです。
ケイデンスは、
世界で不可欠なEDAおよびSDAツールを構築しています。
私たちもケイデンスを使用しています。
この3社、アンシス、シノプシス、ケイデンスの間で、
私たちは基本的にNVIDIAを構築しています。
私たちは一緒になって、ケイデンスをCUDAで加速しています。
また、NVIDIA GPUでスーパーコンピューターを構築し、
顧客が100倍、1000倍のスケールで流体力学シミュレーションを行えるようにしています。
基本的に、リアルタイムで風洞を再現することができます。
ケイデンス・ミレニアム、NVIDIA GPUを内蔵したスーパーコンピューター。ソフトウェア会社がスーパーコンピューターを作る。
私はそれを見るのが大好きだ。
ケイデンスのコ・パイロットを一緒に作る。
想像してみてください。
ケイデンスがANSYSのツール・プロバイダを統合し、
AIコ・パイロットを提供することで、
何千、何万ものコ・パイロット・アシスタントがチップやシステムの設計を手伝ってくれるようになるのです。
また、ケイデンス・デジタル・ツイン・プラットフォームをオムニバースに接続する予定です。世界のCAE、EDA、SDAを加速させ、
デジタル・ツインで未来を創る。
そして、それらすべてを、
未来のデジタル・ツインのための基本的なオペレーティング・システムであるOmniverseにつなげようとしています。
スケールの恩恵を多大に受けた産業のひとつに、
皆さんよくご存知の大規模言語モデルがあります。
基本的に、トランスフォーマーが発明された後、
私たちは大規模な言語モデルを驚異的な速度でスケールさせることができるようになりました。さて、6カ月ごとに倍増することで、私たちが業界を成長させ、計算要件をここまで成長させたのはなぜでしょうか?
その理由は単純明快だ。
モデルのサイズが2倍になれば、脳のサイズも2倍になり、
それを埋めるために必要な情報も2倍になる。
そのため、パラメータ数を2倍にするたびに、
トレーニング・トークン数も適切に増やさなければならない。
この2つの数字の組み合わせが、
サポートしなければならない計算規模となる。
最新の、最先端のOpenAIモデルは、
約1兆8000億パラメータです。
1.8兆個のパラメータを学習するには、数兆個のトークンが必要だった。
つまり、数兆個のパラメータは、数兆個のトークンのオーダーで、2つを掛け合わせると、1秒あたり約300億、400億、500億、4兆の浮動小数点演算が必要になる。さて、今すぐCOの計算をしなければならない。
ちょっと待ってください。
つまり、300億クアドリリオン。
クアドリリオンはペタのようなものです。
ペタフロップのGPUがあれば、
そのモデルを訓練するために300億秒の計算が必要になります。
300億秒は約1,000年です。
まあ、1,000年なら、それだけの価値はある。
もっと早くやりたいけど、それだけの価値はある。
多くの人が私に、ねえ、何かをするのにどれくらいかかるの?20年?その価値はある。
でも、来週にはできるかな?
だから1,000年、1,000年。ですから、私たちに必要なのは、
より大きなGPUなのです。
私たちはこのことに早くから気づいていました。
そして、その答えはGPUを大量に搭載することだと気づきました。
もちろん、テンソルコアの発明や、MV-Linuxの進化によって、実質的に巨大なGPUを作ることができるようになりました。
また、
メラノックスという会社のインフィニバンドという素晴らしいネットワークでGPUを接続することで、巨大なシステムを作ることができるようになりました。
DGX1は私たちの最初のバージョンでしたが、
これが最後ではありませんでした。
私たちはずっとスーパーコンピューターを作り続けてきました。
2021年には4,500GPUのSeleneを開発しました。
そして2023年には、世界最大級のAIスーパーコンピューターを構築しました。
オンラインになったばかりです。EOSです。
そして、私たちはこれらのものを構築しながら、
世界がこれらのものを構築する手助けをしようとしています。
そして、世界がこれらのものを構築するのを手助けするためには、
まず我々がそれらを構築しなければならない。
チップ、システム、ネットワーク、必要なソフトウェアのすべてを構築するのです。これらのシステムを見てほしい。
システム全体で実行されるソフトウェアを書いて、
何千ものGPUに計算を分散させることを想像してみてください。
しかし、その内部には何千もの小さなGPUがあり、
何百万ものGPUがそのすべてに仕事を分散させ、
作業負荷のバランスをとることで、エネルギー効率を最大限に高め、
計算時間を最適化し、コストを抑えることができるのです。
このような基本的な技術革新があったからこそ、
私たちはここまで来られたのです。
そして今、
ChatGPTの奇跡が目の前に現れているのを目の当たりにしている。
インターネット上のテキストだけでなく、
テキストや画像、グラフやチャートなど、
マルチモダリティのデータで訓練するつもりです。
テレビを見て学習するのと同じようにね。
物理学に基づいたモデルが、
腕が壁を通り抜けないことを理解できるように、
ビデオをたくさん見ることになる。
世界中の多くのビデオと世界中の多くの言語を組み合わせて見ることで、
これらのモデルは常識を持つようになる。
私たちがそうであるように、
合成データ生成のようなものを使うだろう。
私たちが学ぼうとするとき、
この基調講演の準備をしていたときの私のように、
想像力を働かせてその結末をシミュレートするかもしれない。
この基調講演の準備の時もそうだった。
この基調講演がどうなるかをシミュレーションしていたとき、
誰かが、別のパフォーマーは、エネルギー全開で講演できるように体調を整えるために、完全にトレッドミルの上で演技をしたと言っていた。
私はそんなことはしなかった。
もし私が開始10分ぐらいで少し風邪気味になったら、
何が起こったかわかるだろう。
それで、私たちはどこにいたのですか?
私たちは合成データ生成を使ってここに座っている。
強化学習を使う。頭の中で練習する。生徒と教師、討論者のように、AIとAIが互いにトレーニングし合う。そうすることで、モデルのサイズが大きくなる。データ量も増え、さらに大きなGPUを作らなければならなくなる。ホッパーは素晴らしいですが、より大きなGPUが必要です。というわけで、皆さん。
とてもとても大きなGPUを紹介したいと思います。
デビッド・ブラックウェルにちなんで名づけられました。
数学者。ゲーム理論家。確率。完璧な名前だと思いました。
ブラックウェル、皆さん、お楽しみください。
ありがとう。どうやったらこんなことができるのか、
という質問から始めたいと思います。
どうやったらこんなことができるのかという疑問から先に始めようと思う。
Blackwellはチップではありません。
Blackwellはプラットフォームの名前です。
私たちはGPUを作っていると思われていますが、
GPUは以前のようには見えません。
これがBlackwellシステムの心臓部です。
これは社内ではBlackwellとは呼ばれず、ただの数字です。
そして、これがBlackwellで、その隣に座っているのが、
現在生産されている世界で最も先進的なGPUです。これがホッパーです。
これがホッパーです。ホッパーは世界を変えました。
これがブラックウェル大丈夫だ
ホッパーとてもいい子だいい子だいい子だ
2080億トランジスタ2つの色素の間に小さな線があるのが見えますか?
2つの色素が1つのチップだと思うように、
このように2つの色素がくっついたのは初めてのことです。
その間に10テラバイトのデータがあり、
1秒間に10テラバイトのデータがあるため、
ブラックウェルのチップのこの2つの面は、
自分たちがどちら側にいるのかまったくわからない。
ただ1つの巨大なチップなのだ。
ブラックウェルの野望が物理学の限界を超えていると聞かされたとき、
エンジニアは、だから何だと言った。それでこうなった。
これがブラックウェルのチップで、2種類のシステムに搭載されています。
1つ目は...
フォーム・フィット機能はホッパーと互換性がありますか?
ホッパーをスライドさせ、ブラックウェルを押し込む。
これが、ランプ化の課題の一つである効率化の理由です。
世界中にホッパーが設置されていますが、
同じインフラ、同じデザイン、電力、サーマル、ソフトウェアです。
これが現在のHGXのホッパーバージョンです。
そしてこれが2つ目のホッパーです。
これは試作ボードです。ジャニーン、ちょっと貸してくれる?
皆さん、ジャニーン・ポールです。
これは完全に機能するボードです。
これは100億ドル。2番目は50億ドル。
それ以降は安くなるので、会場にいるお客さんは大丈夫です。
でも、これはかなり高い。
これはブリングアップボードだ。
で、本番のやり方はこんな感じ。
これを使うんだ。
2つのBlackwellチップと
4つのBlackwellダイがGrace CPUに接続されています。
グレイスCPUは超高速のチップ間リンクを持っています。
驚くべきは、このコンピューターがこの種のものとしては初めて、
これだけの計算量を......。
まず第一に、これほど小さな場所に収まっている。
第二に、メモリが首尾一貫している。
まるでひとつのアプリケーションに一緒に取り組んでいる、
ひとつの大きな家族のように感じられる。
だから、その中ですべてが首尾一貫している。
ただ、その量は......数字を見ただろう。
あれもこれもテラバイトもある。
しかし、これは奇跡です。
これは......これは......これは......これは......これは?
上はMVLink、下はPCI Expressだ。
左はどっち?どっちでもいい。どっちでもいい。
ひとつはCPUチップ間リンク。
私の左か、あなたの左か、どちらかによって違う。どっちでもいいんだ。
うまくいけばプラグインされるんだけどね。
さて、これがグレース・ブラックウェルのシステムだ。
でもまだあるんだ。
つまり、すべてのスペックは素晴らしいんだけど、
新しい機能がたくさん必要なんだ。
物理学の限界を超えるためには、より多くのXファクターが必要です。
そこで私たちが行ったことのひとつが、
もうひとつのトランスフォーマー・エンジン、
第2世代のトランスフォーマー・エンジンを発明したことです。
このエンジンは、
数値フォーマットを可能な限り低い精度に自動的に再スケーリングし、
再キャストする機能を備えている。
覚えておいてほしいのは、
人工知能とは確率のことだということだ。
つまり、1.7、約1.7×約1.4で、約1.7×約1.4となる。
これって意味があるんですか?
だから、
パイプラインの特定の段階で必要な精度と範囲を保持する
数学の能力は非常に重要なんだ。
だから...これは単にALUを小さく設計したというだけの話ではありません。世の中はそんなに単純ではありません。
何千ものGPUを使うような計算で、
そのALUをいつ使えるかを考えなければなりません。
何週間も何週間も実行し、
トレーニングジョブが収束することを確認したい。
この新しいトランスフォーマー・エンジンには、第5世代のNVLinkが搭載されています。これはHopperの2倍の速度ですが、非常に重要なのは、ネットワーク内で計算ができることです。
その理由は、非常に多くの異なるGPUが一緒に動作する場合、
お互いに情報を共有しなければならないからです。
互いに同期を取り、更新し合う必要がある。
そして頻繁に、部分積を減らして、
部分積の合計を他のみんなに再ブロードキャストしなければならない。
そのため、"all reduce"、"all to all"、"all gather "と呼ばれる作業が多く発生する。
これはすべて、GPUが互いに連携できるようにするための同期とコレクティブの領域の一部です。
非常に高速なリンクを持ち、ネットワーク内で数学ができることで、
本質的にさらに増幅することができます。
つまり、1秒あたり1.8テラバイトといっても、
実質的にはそれ以上なのです。
つまり、ホッパーの何倍にもなるのです。
スーパーコンピューターが何週間も動き続ける可能性はほぼゼロです。
その理由は、
非常に多くのコンポーネントが同時に動作するためで、
統計的に、継続的に動作する確率は非常に低いのです。
そのため、故障が発生するたびに、
チェックポイントを行い、できるだけ頻繁に再起動する必要があります。
しかし、
弱ったチップや弱ったノードを早期に発見する能力があれば、
そのノードをリタイアさせ、
別のプロセッサーと交換することができます。
スーパーコンピューターの稼働率を高く維持する能力は、
特に20億ドルをかけて構築したばかりの
スーパーコンピューターでは非常に重要です。
そこで私たちはRASエンジン(信頼性エンジン)を導入し、
Blackwellチップ上のすべてのゲート、すべてのメモリ、
それに接続されたすべてのメモリについて、100%のセルフテスト、
インシステムテストを実施しました。
まるで、
すべてのチップに私たちのチップをテストする
先進的なテスターを同梱しているようなものです。
これは初めてのことです。
とても楽しみです。
セキュアなAI今日、
RASに拍手を送ったのはこのカンファレンスだけだ。
セキュアAI。
明らかに、あなたは何億ドルも費やして非常に重要なAIを作った。
そしてそのコード、AIの知性はパラメータにエンコードされている。一方では、それを失わないようにしたい。一方では、それが汚染されないようにしたい。そのため、私たちはデータを暗号化する能力を手に入れた。すべて暗号化されているのです。暗号化して送信し、計算するときは信頼された環境、信頼されたエンジン環境で行うことができるようになりました。そして最後が解凍です。計算速度が非常に速くなると、ノードへのデータの出し入れが不可欠になります。そこで私たちは高速圧縮エンジンを導入し、データを20倍速くコンピュータに出し入れできるようにしました。これらのコンピューターは非常にパワフルで、多額の投資を必要とします。
私たちが一番避けたいのは、
コンピュータをアイドル状態にしておくことです。
だから、これらの機能はすべて、ブラックウェルに栄養を与え、
可能な限り忙しくさせることを目的としている。
全体的に見て、
ホッパーと比べるとチップあたりのトレーニング性能はFP8の2.5倍です。
また、FP6と呼ばれる新しいフォーマットが追加され、
計算速度は同じでも、メモリのおかげで帯域幅が増幅され、
メモリに格納できるパラメーターの量が増えた。
FP4は実質的にスループットを2倍にする。
これは推論にとって極めて重要だ。
チャットボットとチャットしているとき、
チャットボットにレビューや画像作成を依頼しているとき、
裏側ではGPUがトークンを生成していることを思い出してください。
推論と呼ぶ人もいるが、生成と呼ぶほうが適切だ。
これまでのコンピューティングは検索でした。
携帯電話を手に取り、何かに触れると、何らかの信号が発せられ、基本的には電子メールがどこかのストレージに送られる。
誰かが記事を書いたり、画像を作ったり、ビデオを録画したり。
録音されたコンテンツは携帯電話にストリーミングされ、
レコメンダー・システムに基づいて再構成され、
あなたに情報を提供します。
将来、そのコンテンツの大半が検索されなくなることはご存じでしょう。
その理由は、コンテキストを理解していない誰かが事前に録音したものだからです。
もし、あなたが誰で、
どのような理由で情報を取得しようとしているのか、
そのコンテキストを理解し、
あなたの好みに合わせて情報を作成してくれるAIと一緒に仕事ができれば、エネルギーの節約、ネットワーク帯域幅の節約、無駄な時間の節約につながるでしょう。
未来はジェネレイティブであり、
それがジェネレイティブAIと呼ばれる理由であり、
これがまったく新しい産業である理由である。
計算方法は根本的に異なります。
私たちはジェネレーティブAI時代のためのプロセッサーを開発しました。
その最も重要な部分のひとつが、
コンテンツ・トークンの生成です。私たちはこれをFP4と呼んでいます。
これはかなりの計算量です。
トークン生成で5倍、ホッパーの推論能力で5倍、十分なように思えます。
しかし、なぜそこで止まるのか?
その答えは、十分ではないからだ。
その理由をお見せしましょう。
そこで私たちは、
このGPUよりもさらに大きなGPUを持ちたいと考えました。
そこで、GPUをスケールアップすることにしました。
その前に、
私たちがどのようにスケーリングしてきたかをお話ししましょう。
この8年間で、私たちは計算量を1,000倍に増やしました。
8年間で1,000倍。ムーアの法則の古き良き時代を思い出してほしい。
5年ごとに2倍、いや5倍、10倍だった。
これは最も簡単な計算だ。5年ごとに10倍、10年ごとに100倍。
PC革命の全盛期には、10年ごとに100倍だった。
10年ごとに100倍。この8年間で1000回。
あと2年ある。というわけで、それを踏まえて考えてみた。
コンピュータの進歩の速さは異常ですが、
それでもまだ十分な速さではないので、別のチップを作りました。
このチップは信じられないようなチップです。
MV-Linkスイッチと呼んでいます。
500億トランジスタです。
それだけでホッパーとほぼ同じ大きさです。
このスイッチ・チップには4つのMV-Linkがあり、
それぞれ1秒あたり1.8テラバイトで、
先ほど申し上げたように計算機能を備えています。
このチップは何のためにあるのでしょうか?
もしこのようなチップを作れば、
すべてのGPUが他のGPUと同時にフルスピードで会話することができる。
正気の沙汰とは思えない。
しかし、もしそれができるのなら、その方法を見つけ、
そのためのシステムを構築できるのなら、それは費用対効果に優れている。
費用対効果が高い。
すべてのGPUをコヒーレントリンクで接続し、
事実上1つの巨大なGPUにすることができたら、
どんなに素晴らしいことでしょう。
このチップは銅を直接駆動する必要があります。
このチップのサーデイは驚異的な発明で、
銅を直接駆動することができます。
その結果、
このようなシステムを構築することができるのです。
さて、このシステムはちょっと正気の沙汰ではありません。
これは1つのDGXです。
これが現在のDGXの姿です。
ちょうど6年前、かなり重かったのですが、
私は持ち上げることができました。
最初のDGX-1をOpenAIに納品し、
そこの研究者たちがインターネットに写真を載せて、
私たち全員がサインをしました。
私のオフィスに来ていただければ、そこにサインしてありますよ。
でも、持ち上げることはできます。
ちなみに、このDGXは170テラフロップスでした。
ナンバリングシステムに馴染みがないと思いますが、
これは0.17ペタフロップスです。
つまりこれは720です。
OpenAIに最初に納品したのは0.17でした。
0.2に切り上げても違いはありません。
でも当時は、すごい、あと30テラフロップスだ、という感じだった。
そして現在、720ペタフロップス、
トレーニング用のほぼ1エクサフロップス、
世界初の1ラック1エクサフロップスのマシンが完成しました。
ご存知のように、
エクサフロップス・マシンは地球上に2、3台しかありません。
つまり、これは1ラックに1エクサフロップスのAIシステムなのです。
では、その裏側を見てみましょう。
これがそれを可能にするものです。
これが背面のDGX MV-Linkスパインです。
毎秒130テラバイトがこのシャーシの背面を通っています。
これはインターネットの総帯域幅よりも大きい。
ですから、基本的には1秒以内にすべての人に送ることができます。
合計5,000本のNVLinkケーブルがあり、その長さは2マイルに及びます。
これが驚くべきことです。
もしオプティクスを使わなければならなかったら、
トランシーバーとリタイマーを使わなければならなかっただろう。
そのトランシーバーとリタイマーだけで、
MV-Linkスパインを駆動するために2万ワット、
トランシーバーだけで2キロワットのコストがかかったでしょう。
その結果、MVリンク・スイッチで完全に無料化し、
20キロワットを計算用に節約することができました。
このラック全体は120キロワットですから、
20キロワットは大きな違いです。液冷式です。
中に入るのは室温程度の25度C。
出てくるのは45℃のジャグジーだ。
つまり、室温のものが入り、ジャグジーが出てくる。
60万個の部品をね。
以前、誰かが言ったんだ、君たちはGPUを作っていると。
誰かがGPUと言うと、私はこれを見る。
2年前、私がGPUを見たのはHGXだった。
それは70ポンド、35,000個の部品でした。
今のGPUは60万パーツ、3,000ポンドです。
3,000ポンド。3,000ポンドというと、
カーボンファイバー製のフェラーリみたいな重さですね。
それが有用な指標かどうかはわからないが、誰もがそれを感じている。
感じる。分かるよ。そうだね。そう言われてみれば、そうだ。
何が3,000ポンドなのか分からない。
じゃあ、3,000ポンドは1トン半だね。だから象とはちょっと違う。
DGXはこんな感じです。
では、実際にどんな風に動くのか見てみましょう。さて、想像してみよう。これをどのように働かせるのか、そしてそれは何を意味するのか?
GPTモデル、
1兆8000億のパラメータ・モデルをトレーニングする場合、
2万5000アンペアで約3カ月から5カ月かかる。
もしHopperでそれを行うとしたら、
おそらく8,000個のGPUが必要で、15メガワットを消費するでしょう。
8,000GPUで15メガワット。90日、約3カ月かかるだろう。
それで、この画期的なAIモデルを訓練することができる。
これは明らかに、誰もが考えるほど高価なものではありませんが、8,000GPUです。それでも大金だ。
8,000GPU、15メガワット。
もしBlackwellを使えば、2,000GPUで済む。
2,000GPUで同じ90日。たった4メガワットの電力です。
これが私たちの目標です。
私たちの目標は、コストとエネルギーを継続的に下げることです。
コストとエネルギーは正比例します。
次世代モデルを訓練するために必要な計算を拡大し、
スケールアップし続けることができるようにするためです。
これがトレーニングだ。
推論や生成は今後も極めて重要です。
最近、NVIDIA GPUがクラウドにある時間のおそらく半分は、
トークン生成に使われています。
コパイロット、チャット、チャットGPT、あるいは画像生成、動画生成、タンパク質生成、化学物質生成など、さまざまなモデルが使用されています。たくさんの生成が行われている。これらはすべて、推論と呼ばれるコンピューティングの範疇にある。しかし、大規模な言語モデルにとって、推論は非常に難しい。ひとつは、非常に大きいということ。そのため、GPU1つでは収まらない。これは、Excelが1つのGPUに収まらないと想像してみてください。また、あなたが日常的に実行しているアプリケーションが、1つのコンピューターに収まらないことを想像してみてください。実際、ほとんどのアプリケーションはそうだ。ハイパースケール・コンピューティングでは、過去に何度も、多くの人が多くのアプリケーションを同じコンピュータに載せていました。そして今、突然、このチャットボットと対話する推論アプリケーションは、そのチャットボットを実行するために、後ろにスーパーコンピュータを必要とします。
それが未来だ。
チャットボットは何兆ものトークン、何兆ものパラメータを持ち、対話的な速度でトークンを生成しなければならない。どうでしょう?
3トークンというのは単語1個分です。
宇宙は最後のフロンティアと呼ばれる冒険で、
80トークンのようなものです。ああ、これはうまくいかないな。
何を言ってるんだか...スタートレックは見たことがない。
私たちは、このようなトークンを作り出そうとしています。
このトークンと対話するとき、トークンができるだけ早く、できるだけ早く自分に返ってくることを期待する。だから、トークンを生成する能力は本当に重要だ。
このモデルの作業を何台ものGPUで並列化することで、
いくつかのことが実現できます。
ひとつは、スループットを上げることで、
トークン1個あたりの生成コストを下げることができるからです。
つまり、スループットがサービスを提供するコストを決めるのです。
一方では、別のインタラクティブ・レートがあり、
これは1秒あたりのトークン数で、ユーザーごとに異なります。
これはサービスの質に関係します。
つまり、この2つは互いに競合しているのです。
この2つを両立させるためには、GPUに作業を分散させ、
並列化する方法を見つけなければなりません。
そして、その探索空間は膨大であることがわかった。
数学が絡んでくると言っただろう。
みんな、やれやれって感じだよ。
今、このスライドを出したとき、何人かが息を呑んだ。
Y軸は1秒あたりのトークンで、データセンターのスループットです。
X軸は1秒あたりのトークン数で、インタラクティビティを表しています。
右上が最高であることに注目してください。
インタラクティビティが非常に高いことが望まれます。
ユーザーあたりの1秒あたりのトークン数。
データセンターあたりの1秒あたりのトークン数を非常に高くしたい。
右上は素晴らしい。
しかし、それを実現するのは非常に難しい。
X,Y座標、つまりX,Y座標のひとつひとつを見て、
その交点のひとつひとつにわたって最適な答えを探すためには、
この青い点はすべてソフトウェアの再分割によるものです。
ある最適化ソリューションが、テンソル並列、エキスパート並列、パイプライン並列、データ並列のどれを使うかを考え、この巨大なモデルをすべての異なるGPUに分散し、必要なパフォーマンスを維持しなければなりません。
そして、CUDAのおかげで、
これほど豊かなエコシステムがあるからこそ、この宇宙を探索し、
緑の屋根線を見つけることができたのです。
TP2EP8DP4という緑のルーフラインがある。
これは2つのテンソル並列を意味する。
2つのGPUにまたがるテンソル並列、
8つのエキスパート並列、4つのデータ並列。
もう一方の端には、4つのテンソル・パラレルと16のエキスパート・パラレルがある。ソフトウェアの構成や分布、ランタイムの違いによって、このような異なる結果が得られるのだ。
そして、そのルーフラインを発見しなければならない。
これは一つのモデルに過ぎない。
これはコンピュータの1つの構成に過ぎない。
世界中で作られているすべてのモデルと、
利用可能なシステムのすべての異なる構成を想像してみてください。
さて、基本を理解したところで、
ホッパーと比較したブラックウェルの推論を見てみよう。
これが驚くべきことなのです。
私たちはトリリウム・パラメーター生成AIのために設計されたシステムを作ったので、1世代で、Blackwellの推論能力は桁外れです。
実際、ホッパーの約30倍です。
そうですね。ChatGPTのような大規模言語モデルでは、
青い線がホッパーです。
ホッパーのアーキテクチャを変えたわけではありません。
ただチップを大きくしただけです。
最新の10テラバイトを使っただけです。
テラバイト/秒のチップを2つつなげば、2080億/年の巨大なチップになる。
何も変わらなかったらどうだっただろう?
紫色の線がそうですが、それほど素晴らしいものではありませんでした。
そこで、FP4テンソルコア、新しいトランスフォーマー・エンジン、
そして非常に重要なのがNVLinkスイッチです。
その理由は、
これらすべてのGPUが結果や部分的な製品を
共有しなければならないからです。
GPUがオール・トゥ・オールやオール・ギャザーを行う場合、
GPU同士が通信を行う場合、NVLinkスイッチの通信速度は、
過去に最速のネットワークを使用した場合に比べ、ほぼ10倍速くなります。
なるほど...Blackwellは、
生成AIのための素晴らしいシステムになるでしょう。
そして将来、データセンターは、先ほど申し上げたように、
AI工場として考えられるようになるでしょう。
AI工場の目標は、収益を上げることです。
交流発電機のように電気を発生させるのではなく、
前回の産業革命や今回の産業革命のように、
インテリジェンスを発生させるのです。
だから、この能力は超、超重要なのだ。
ブラックウェルの興奮は本当に桁外れです。
私たちが最初に、つまり1年半前か2年前だと思いますが、
Hopperの市場投入を始めたときです。
その時、2社のCSPがランチに参加してくれたんです。
そして、2社の顧客に恵まれました。
今はもっと増えています。
Blackwellにとって信じられないような興奮です。
信じられないような興奮です。
そして、さまざまなコンフィギュレーションがある。
もちろん、ホッパー・フォーム・ファクターにスライドさせる構成をお見せしましたので、アップグレードは簡単です。
液冷式の例もお見せしましたが、これはその極端なバージョンです。
ラック全体がMV-Link-72で接続されています。
Blackwellは、
さまざまなモダリティで素晴らしい仕事をしている
世界のAI企業(現在、非常に多くの企業が存在する)に向けて、
今後さらに力を注いでいく予定です。
CSPは、どのCSPも準備を整えている。
世界中のOEMやODM、地域クラウド、
主権AI、通信事業者がBlackwellと契約している。
Blackwellは、
私たちの歴史の中で最も成功した製品ローンチとなるでしょう。
それを見るのが待ち遠しい。
これに参加してくれているいくつかのパートナーに感謝したい。
AWSはBlackwellに向けて準備を進めている。
セキュアなAIを搭載した初のGPUを構築する予定だ。
222エクサフロップスのシステムを構築中だ。
デジタル・ツインをアニメ化したとき、
クラスターがすべて降りてくるのを見ただろう。
ところで、これは単なるアートではありません。
私たちが構築しているもののデジタル・ツインです。
それだけ大きなものになるということです。
インフラ以外にも、私たちはAWSとともに多くのことを行っています。
SageMakerのAIをCUDAで加速しています。
ベッドロックAIをCUDAアクセラレートしています。
Amazon Roboticsは、NVIDIA OmniverseとIsaac Simを使って我々と協力しています。AWS HealthにはNVIDIA Healthが統合されています。
AWSはアクセラレーテッド・コンピューティングに傾倒しているのです。
GoogleはBlackwellの準備を進めています。
GCPにはすでにA100s、H100s、T4s、L4sがあります。
NVIDIA CUDA GPUの全フリートがあり、
最近GEMMAモデルを発表しました。
我々はGCPのあらゆる側面を最適化し、高速化するために取り組んでいる。データ処理のためのDataproc、データ処理エンジン、JAX、XLA、Vertex AI、ロボット工学のためのMujokoを高速化しています。このように、私たちはグーグルやGCPとさまざまな取り組みを行っています。
オラクルはBlackwellのために準備を進めています。
オラクルは我々の素晴らしいパートナーです。
また、多くの企業にとって本当に重要なOracle Databaseを加速させるために協力しています。
マイクロソフトは、Blackwellを加速させています。
Microsoft NVIDIAは幅広いパートナーシップを結んでいます。
我々はCUDAを加速させ、あらゆるサービスを加速させている。
Microsoft AzureにあるチャットやAIサービスでは、NVIDIAが推論やトークン生成を行っています。
彼らは最大のNVIDIA InfiniBandスーパーコンピュータを構築し、
基本的には我々のデジタル・ツイン、
あるいは物理的なツインを構築しました。
私たちは、NVIDIAのエコシステムをAzureにもたらしています。
NVIDIA DGRX CloudをAzureに。
NVIDIA OmniverseはAzureでホストされています。
NVIDIA HealthcareはAzureにあります。
そのすべてがMicrosoft Fabricと深く統合され、深くつながっています。
業界全体がBlackwellに向けて準備を進めています。
これがこれからお見せするものです。
これまでご覧いただいたBlackwellのシーンのほとんどは、
Blackwellの完全忠実設計です。
私たちの会社のすべてのものには、デジタル・ツインがあります。
そして実際、このデジタル・ツインのアイデアは本当に広まっていて、
企業が非常に複雑なものを初めて完璧に作り上げるのに役立っています。
そして、
これ以上にエキサイティングなことがあるだろうか......
デジタル・ツインで作られたコンピュータを作ることだ。
それで、ウィストロンが何をしているかをお見せしましょう。
NVIDIAアクセラレーテッド・コンピューティングの需要に応えるため、
当社の主要な製造パートナーの1つであるWistron社は、
Omniverse SDKとAPIを使って開発したカスタムソフトウェアを使用して、NVIDIA DGXとHGX工場のデジタルツインを構築しています。Wistron社の最新工場では、マルチCADとプロセスシミュレーションデータを仮想的に統合し、統一されたビューにするためにデジタルツインからスタートしました。この物理的に正確なデジタル環境でレイアウトのテストと最適化を行うことで、作業員の効率が51%向上しました。建設中、オムニバースのデジタルツインは、物理的な構築とデジタルプランの一致を検証するために使用されました。矛盾を早期に発見することで、コストのかかる変更注文を避けることができました。そして、その結果は素晴らしいものでした。
デジタルツインを使用することで、
Wisdrawnの工場は5ヶ月のところ、
わずか2ヶ月半という半分の期間でオンライン化することができました。
運用では、オムニバースのデジタルツインは、ウィストロンが新しいプロセスに対応したり、既存のスペースでのオペレーションを改善するために、新しいレイアウトを迅速にテストし、生産ラインのすべてのマシンからのライブIoTデータを使用してリアルタイムのオペレーションを監視するのに役立っています。NVIDIA AIとOmniverseにより、NVIDIAのグローバルなパートナーエコシステムは、AIを活用したデジタル化を加速する新時代を築いています。私がまずすべてをデジタルで製造し、それから物理的に製造するというのが、これからの時代の流れです。
みんなに聞かれるんだ、どうやって始まったの?と聞かれる。
この素晴らしいアイデアにすべてを注ぎ込むきっかけとなったものは何だったのですか?と聞かれる。ちょっと待って。みんな、今のはそんな瞬間になるはずだったんだ。リハーサルをしないからこうなるんだ。ご存知のように、これがファースト・コンタクトだった。
2012年 アレックスネットこのコンピューターに猫を入れると、
猫が出てきて、猫と言うんだ。
そして私たちは、ああ、これはすべてを変えようとしている、と言った。RGBの3つのチャンネルで100万個の数字が表示される。
これらの数字は誰にも意味をなさない。
それをこのソフトウェアに入れると、圧縮され、次元が縮小される。
100万次元から100万次元に縮小する。
3つの文字、1つのベクトル、1つの数字に変換する。
そしてそれは一般化され、猫をさまざまな猫にすることができる。
猫の正面と背面にもできる。それを見て、信じられないと言うだろう。
どんな猫でも?ええ、どんな猫でも。
そして、すべての猫を認識することができた。
そして私たちは、その仕組みに気づいた。
システム的に、構造的に、スケーラブルなんだ。
どれくらい大きくできる?どこまで大きくできるか?
そうして私たちは、
これがまったく新しいソフトウェアの書き方だと想像したのです。
そして今日、ご存知のように、C-A-Tと入力することができる。
そして出てくるのは猫だ。それは逆だった。そうだろ?信じられない。
そうだよ。3つの文字から100万のピクセルを作り出し、
それが意味を成すなんて。それが奇跡なんだ。
そして、文字どおり10年後、10年後、私たちはテキストを認識し、
画像を認識し、ビデオや音や画像を認識するようになった。
認識するだけでなく、その意味も理解できる。
テキストの意味を理解する。
だからチャットもできる。要約もできる。テキストを理解する。
単に英語を認識するだけでなく、英語を理解する。
ピクセルを認識するだけでなく、ピクセルを理解する。
さらに、2つのモダリティの間で条件付けすることもできる。
言語が画像を条件づけることで、
あらゆる種類の興味深いものを生み出すことができる。
さて、これらのことを理解できるのであれば、デジタル化されたもので、他に何を理解できるでしょうか?私たちがテキストと画像から始めたのは、それらをデジタル化したからです。
しかし、他に何をデジタル化したのか?
まあ、多くのものをデジタル化したことがわかった。
タンパク質、遺伝子、脳波。デジタル化できるものは何でも、
構造さえあれば、そこから何らかのパターンを学ぶことができるだろう。
そして、
そこからパターンを学ぶことができれば、
その意味を理解することができる。
意味を理解できれば、それを生成することもできるかもしれない。
したがって、ジェネレーティブAI革命はここにある。
では、他に何を生成できるのか?
他に何を学ぶことができるのか?
私たちがぜひとも学びたいことの一つは、気候を学びたいことだ。
異常気象を学びたい。
地域的なスケールで、
十分に高い解像度で将来の天候を予測する方法を学びたい。
異常気象は、世界に1500億ドルの損害を与えている。
それ以上に、その被害は均等ではない。
1,500億ドルは世界の一部に、そしてもちろん世界の一部の人々に集中している。私たちは適応する必要があり、何が起こるかを知る必要がある。そこで私たちは、気象予測のための地球のデジタル・ツインである「Earth 2」を開発し、コアディヴという驚異的な発明をしました。
見てみよう地球の気候が変化するにつれて、AIを活用した気象予測は、2021年に台湾とその周辺地域に広範囲な被害をもたらしたスーパー台風「チャントゥ」のような激しい暴風雨をより正確に予測し、追跡することを可能にしている。現在のAI予測モデルは、暴風雨の進路を正確に予測することができますが、解像度が25kmに制限されているため、重要な詳細を見逃す可能性があります。NVIDIAのCORDIFは、高解像度でレーダー同化された矮小気象予報とERA5再解析データで訓練された画期的な新しい生成AIモデルである。コーディフを使用することで、チャンツのような極端な事象を、従来の気象モデルの1000倍のスピードと3000倍のエネルギー効率で、25kmから2kmの解像度で超解像することができます。NVIDIAの気象予測モデルForecastNetのスピードと精度と、Cordiffのような生成AIモデルを組み合わせることで、数百、数千キロスケールの地域気象予測を探索し、暴風雨の最良、最悪、そして最も起こりそうな影響について明確な画像を提供することができます。この豊富な情報は、人命や物的損害を最小限に抑えるのに役立ちます。現在、CORDiFは台湾向けに最適化されていますが、間もなくNVIDIA Earth-2推論サービスの一部として、世界中の多くの地域でジェネレーティブ・スーパーサンプリングが利用できるようになります。
気象会社は、
グローバルな気象予測のソースを信頼しなければなりません。
我々は、彼らの気象シミュレーションを加速させるために協力しています。シミュレーションの最初の原則的ベース。しかし、EarthをCoreDiffに統合することで、企業や国々が地域の高解像度気象予測を行うのを支援できるようにするつもりです。ですから、もしあなたが天気予報について知りたいことややりたいことがあれば、気象会社に連絡してください。本当にエキサイティングな仕事です。
エヌビディア・ヘルスケア。15年前に始めたものです。
私たちはこれにとてもとても興奮しています。
この分野は私たちの誇りです。
医療画像であれ、遺伝子配列決定であれ、計算化学であれ、
NVIDIAがその背後にある計算を担っている可能性は非常に高いのです。
私たちは、この分野で非常に多くの仕事をしてきました。今日、私たちは本当に、本当にクールなことをすると発表します。画像や音声を生成するために使われているこれらのAIモデルを想像してみてください。しかし、画像や音声を理解する代わりに、遺伝子やタンパク質、アミノ酸のために行ってきたすべてのデジタル化が、機械学習を通して行われ、生命の言語を理解できるようになります。もちろん、生命の言語を理解する能力は、アルファフォールドによって初めて証明された。これは本当に驚くべきことだ。何十年にもわたる骨の折れる作業の後、世界ではクライオ電子顕微鏡やX線結晶学を使ってデジタル化し、再構築することしかできなかった。
これらの異なる技術によって、わずか1年足らずの間に、
20万個のタンパク質が丹念に再構築されたのだ。
アルファフォールドは2億個のタンパク質を再構築した。
基本的に、これまでに配列が決定されたすべてのタンパク質、
すべての生物。これは完全に革命的だ。
そのモデルを使うのは難しいし、作るのも難しい。そこで私たちは、このモデルを構築することにした。世界中の研究者のために作るんだ。そして、これだけではありません。私たちが作るモデルは他にもたくさんあります。それでは、私たちがこのモデルを使って何をしようとしているのか、お見せしましょう。
新薬のバーチャル・スクリーニングは、計算上困難な問題である。
既存の技術では、何十億もの化合物をスキャンすることしかできず、
新薬候補を特定するためには何千もの標準的な計算ノードで何日もかかる。NVIDIA Bionemo NIMSは、新しいジェネレーティブ・スクリーニングのパラダイムを可能にします。AlphaFoldによるタンパク質構造予測、MolMIMによる分子生成、DiffDockによるドッキングにNIMSを使用することで、数分で候補分子を生成し、スクリーニングできるようになりました。MolMIMは、カスタム・アプリケーションに接続することで、生成プロセスを制御し、所望の特性に対して反復的に最適化することができます。これらのアプリケーションは、Bionemoマイクロサービスを使って定義することも、ゼロから構築することもできる。
ここでは、物理ベースのシミュレーションによって、
分子が標的タンパク質に結合する能力を最適化し、
並行して他の有利な分子特性についても最適化する。
MolMIMは、標的タンパク質に結合し、合成可能な高品質の医薬品類似分子を生成する。
BioNemoはNIMSによって創薬の新しいパラダイムを実現し、
de novoタンパク質設計やバーチャルスクリーニングのためのガイド付き分子生成のような強力な創薬ワークフローを構築するために組み合わせることができるオンデマンドマイクロサービスを提供します。BioNemo NIMSは、研究者や開発者が計算薬物設計を刷新できるよう支援します。
NIMSは、
研究者や開発者が計算薬物設計を刷新できるよう支援しています。
MoMEM、CoreDiff、他にも様々なモデルがあります。他にもたくさんのモデルがあります。コンピュータ・ビジョンのモデル、ロボット工学のモデル、そしてもちろん、本当に素晴らしいオープンソースの言語モデルもあります。これらのモデルは画期的だ。
しかし、企業が使うのは難しい。どうやって使うのか?
どうやって社内に導入し、ワークフローに組み込むのか?
どのようにパッケージ化して実行するのか?
先ほど私は、推論は並外れた計算問題である。
これらのモデルのひとつひとつについてどのように最適化を行い、
スーパーコンピューターを動かすのに必要な計算スタックを組んで、
社内でこれらのモデルを実行できるようにするのか?
そこで私たちは素晴らしいアイデアを思いついた。私たちは、あなたがソフトウェアを受け取り、操作するための新しい方法を発明するつもりです。
このソフトウェアは、基本的にデジタル・ボックスに入っている。
私たちはそれをコンテナと呼んでいます。
それをNIMと呼んでいます。
それが何なのか、ご説明しましょう。
NIM。事前に訓練されたモデルです。かなり賢い。
そして、
NVIDIAのインストールベース全体で実行できるようにパッケージ化され、
最適化されています。
その中身は驚くべきものです。
事前に訓練された最先端のオープンソースモデルがすべてあります。
オープンソースかもしれません。
私たちのパートナーのものかもしれません。
NVIDIA Momentのように、私たちが作成したものかもしれません。
これは、すべての依存関係とともにパッケージ化されています。
CUDA、正しいバージョン。CUDNN、適切なバージョン。複数のGPUに分散されたTensorRT、LLM。Trident推論サーバーは、すべて完全にパッケージ化されている。最適化されている。シングルGPUか、マルチGPUか、マルチノードのGPUかによって、最適化されています。
そして、使いやすいAPIで接続されています。
ここで、AI APIとは何かを考えてみよう。
AI APIとは、単に会話するためのインターフェースです。
つまり、
これは本当にシンプルなAPIを持つ未来のソフトウェアなのです。
そのAPIは人間と呼ばれる。
そして、これらのパッケージ、つまり信じられないようなソフトウェアが最適化され、パッケージ化され、ウェブサイトに掲載されます。
そしてそれをダウンロードできる。持ち運ぶこともできる。
どのクラウドでも実行できる。
自分のデータセンターで動かすこともできる。
ワークステーションで動かすこともできる。
ai.nvidia.comにアクセスするだけです。
私たちはこれをNVIDIA Inference Microserviceと呼んでいますが、
社内ではNIMSと呼んでいます。
いいですか?想像してみてください。
いつかチャットボットができて、そのチャットボットがNIMSに入る。
そして、チャットボットを大量に組み立てる。
それが、いつの日かソフトウェアが構築される方法です。
私たちは将来、どのようにソフトウェアを構築するのでしょうか?ゼロから書いたり、Pythonのコードを大量に書いたりすることはまずないだろう。AIのチームを編成する可能性が非常に高い。おそらく、あなたが与えたミッションを実行プランに落とし込むスーパーAIを使うことになるでしょう。その実行計画の一部は、別のNIMに引き渡されるかもしれない。
そのNIMはおそらくSAPを理解するだろう。SAPの言語はABAPだ。
ServiceNowを理解し、
そのプラットフォームから情報を取得するかもしれない。
そしてその結果を別のNIMに渡し、
NIMはそのNIMで計算を行うかもしれない。
最適化ソフトウェアかもしれないし、組み合わせ最適化アルゴリズムかもしれない。基本的な計算機かもしれない。
Pandasで数値解析をするかもしれない。
そして、その答えが他の人の答えと組み合わされ、
これが正しい答えだ、と提示される。
製造計画や予測、顧客からの警告、バグ・データベースなど、どんなことでも毎日、毎時最初にレポートを受け取ることができる。そして、これらのNIMSはパッケージ化されているので、データセンターやクラウドにNVIDIA GPUがある限り、NIMSはチームとして連携し、驚くべきことをやってのけます。
そこで私たちは、これは素晴らしいアイデアだ、
それを実行に移そうと決めました。
それで、エヌビディアは社内のいたるところでNIMSを稼働させています。
もちろん、最も重要なチャットボットのひとつは、
チップ設計者のチャットボットです。
驚かれないかもしれませんが、
私たちはチップの製造にとても関心があります。
ですから、私たちはエンジニアと共同設計するチャットボット、AIコ・パイロットを作りたいと思っています。そこで、このような方法を取りました。
私たちはLama 2を手に入れました。
これは70Bで、NIMにパッケージされている。
CTLとは何か?その結果、CTLは内部プログラムで、
内部独自の言語を持っていることがわかったんですが、
CTLは組み合わせタイミング・ロジックだと思っていたので、
従来のCTLの知識が記述されていました。
しかし、それでは我々にとってあまり役に立たないので、
新しい例をたくさん与えた。
これは従業員の入社式と変わりません。
私たちは、その答えをありがとうと言う。完全に間違っています。そして、CTLとはこういうものだと彼らに提示するのです。
なるほど。これがエヌビディアのCTLです。
CTLは見ての通り、コンピュート・トレース・ライブラリの略です。
私たちは常にコンピュートサイクルをトレースしています。
そしてCTLがプログラムを書いた。すごいと思いませんか?
だからチップ設計者の生産性が上がるんです。
これがNIMでできることです。
まず、NIMでできることはカスタマイズです。NEMOマイクロサービスと呼ばれるサービスがあり、データをキュレートして、AIに教えることができるようにデータを準備します。AIを微調整し、それを監視します。答えを評価し、他の例に対するパフォーマンスを評価することもできます。これがNEMOマイクロサービスと呼ばれるものです。
さて、ここで浮かび上がってくるのは次のようなことです。
私たちがやっていることには3つの要素、3つの柱があります。
第一の柱は、
もちろん、AIモデルの技術を発明し、AIモデルを実行し、
それをパッケージ化することです。
2つ目は、
それを修正するためのツールを作ることです。
第一はAI技術を持つこと。2つ目は、それを修正する手助けをすること。
そして3つ目は、
それを微調整するためのインフラです。
そしてお望みであれば、それをデプロイします。
DGXクラウドと呼ばれる私たちのインフラに導入することもできますし、オンプレミスに導入することもできます。好きな場所にデプロイすることができます。
一度開発すれば、どこにでも持っていくことができます。TSMCがチップを製造しているのと同じように、私たちはAIに関してお客様や業界に貢献します。ですから、私たちは大きなアイデアを持ってTSMCに行きます。TSMCはそれを製造し、私たちはそれを持ち帰ります。ここでもまったく同じことが言えます。
AIファウンドリーは、
NIMS、NEMOマイクロサービス、DGXクラウドの3つを柱としています。
もうひとつ、NIMに教えることができるのは、お客様の専有情報を理解することです。社内では、データの大部分はクラウド上にありません。社内にあるのだ。データはずっとそこに置かれ、常に使用されており、基本的にはエヌビディアのインテリジェンスなのです。私たちはそのデータを取得し、その意味を学習したいと考えています。今お話しした他のほとんどのものの意味を学習したように、その意味を学習し、その知識をベクトル・データベースと呼ばれる新しいタイプのデータベースに再インデックス化します。そして、その知識をベクトル・データベースと呼ばれる新しいタイプのデータベースに再インデックス化するのです。
つまり、構造化されたデータまたは非構造化されたデータを受け取り、
その意味を学習し、その意味をエンコードすることで、
これがAIデータベースになります。
どんなことができるのか、例を挙げてみましょう。
マルチモダリティのデータがたくさんあるとします。
PDFをすべて取り出します。
お気に入りのもの、会社独自のもの、
会社にとって重要なものをすべて取り出します。
猫のピクセルをエンコードするように、
それをエンコードすることができます。
PDFをすべてエンコードしてベクターに変換し、
それをベクター・データベースに保存する。
私たちのソフトウェア・チームは、バグ・データベースとチャットするだけで、昨晩は何件のバグがあったのか、進捗状況はどうなのか、そしてこのバグ・データベースとのチャットが終わったら、セラピーが必要になる、ニモ・レトリバーと呼んでいます。その理由は、このボットの最終的な仕事は、できるだけ早く情報を取りに行くことだからです。あなたはただ話しかけるだけです。おい、この情報を持ってきてくれ。そうすると、ニモが情報を持ってきてくれるんだ。これのことか?そう、完璧だ。それで、これをニモ・リトリーバーと呼んでいるんだ。
Nemoサービスは、あなたがこれらのものをすべて作成するのに役立ちます。NIMもいろいろあります。デジタル人間のNIMもあります。私はレイチェル、あなたのAIケアマネージャーです。
さて、本当に短いクリップですが、皆さんにお見せしたいビデオがたくさんあり、また、他にもお見せしたいデモがたくさんありましたので、今回は短く切らせていただきました。
でもこれはダイアナ。彼女はデジタル・ヒューマン・ニムです。
この場合、彼女はヒポクラテスAIのヘルスケアのための大規模な言語モデルに接続されています。本当に素晴らしい。彼女は医療に関して非常に賢い。
私のソフトウェア・エンジニアリング担当副社長のドワイトがバグ・データベースのチャットボットと話した後、あなたはダイアンと話すんです。ダイアンは完全にAIで動いていて、デジタルヒューマンなんだ。
構築したい企業はたくさんあります。
彼らは金鉱の上に座っているのです。
エンタープライズIT業界は金鉱の上に座っている。
なぜなら、彼らは仕事の進め方を熟知しているからだ。
長年かけて作られた素晴らしいツールがあり、たくさんのデータがある。
もし彼らがその金鉱を利用し、共同パイロットに変えることができれば、
共同パイロットは私たちの仕事を助けてくれるでしょう。
だから、人々が使う価値あるツールを持つ世界中のITフランチャイズやITプラットフォームは、共同パイロットの金鉱の上に眠っているのだ。そして、彼らは独自のコ・パイロットやチャットボットを作りたいと考えている。そこで私たちは、NVIDIA AI Foundryが世界の偉大な企業と協力していることを発表します。
SAPは世界の商取引の87%を生み出しています。
基本的に、世界はSAPで動いています。我々はSAPで動いている。
NVIDIAとSAPは、NVIDIA NEMOとDGX Cloudを使用して、
SAP Jewel co-pilotを構築しています。
ServiceNowは、世界のフォーチュン500社の85%がServiceNow上で従業員やカスタマーサービスを運営しています。
そして、ServiceNow Assistバーチャルアシスタントを構築するためにNVIDIA AI Foundryを使用しています。
Cohesityは世界中のデータをバックアップしている。
彼らはデータの宝庫の上にいる。数百エクサバイトのデータ、10,000社以上の企業。NVIDIA AI Foundryは同社と協力し、Gaia Generative AIエージェントの構築を支援している。Snowflakeは、世界のデジタルウェアハウスをクラウドに保管し、1万社の企業顧客に1日30億以上のクエリを提供している企業です。スノーフレークはNVIDIA AI Foundryと協力し、NVIDIA NemoとNIMSを使ったコ・パイロットを構築している。ネットアップ、世界のファイルの半分近くがオンプレミスでネットアップに保存されている。NVIDIA AI Foundryは、チャットボットや、NVIDIA NemoとNIMSを使ったベクターデータベースやリトリーバーのようなコ・パイロットの構築を支援しています。
デルとも素晴らしいパートナーシップを結んでいます。
チャットボットやジェネレーティブAIを構築している皆さんは、
それを実行する準備ができたら、AIファクトリーが必要になります。
デルほど、企業向けの非常に大規模なエンド・ツー・エンドのシステムを構築するのが得意な企業はありません。
ですから、誰でも、どの企業でも、AI工場を構築する必要があります。
マイケルはここにいる。
では、
ロボット工学の次の波についてお話ししましょう
ロボット工学の次の波 物理的AI これまでのところ、
私たちがお話ししてきたAIはすべて1台のコンピュータにデータを取り込んでいます。AIは次の言葉を予測するために、多くの言語を読み取ることで私たちを真似る。
AIは、すべてのパターンと他のすべての過去の例を研究することによって、あなたの真似をするのです。もちろん、文脈なども理解しなければならない。しかし、いったん文脈を理解すれば、基本的にはあなたの真似をする。私たちはすべてのデータをDGXのようなシステムに入れ、大規模な言語モデルに圧縮します。何兆、何兆ものパラメータが何十億、何百億、何兆ものトークンが何十億ものパラメータになり、この何十億ものパラメータがあなたのAIになるのです。AIが物理世界を理解するという、AIの次の波に行くためには、3つのコンピューターが必要です。
最初のコンピューターは、これまでと同じコンピューターです。
そのAIコンピューターは、ビデオを見たり、合成データを生成したり、人間の例をたくさん見たりします。AIは私たちを見て、何が起こっているのかを理解し、それを自分の文脈に適応させようとします。そして、このような基礎モデルを使って一般化することができるため、おそらくこれらのロボットは物理的な世界でもかなり一般的なパフォーマンスを発揮することができるでしょう。つまり、ロボット工学のためのチャットGPTの瞬間がすぐそこまで来ている可能性があることを除けば、本質的に大規模言語モデルで起こっていることを非常にシンプルな言葉で説明したに過ぎない。
私たちは、
ロボット工学のためのエンド・ツー・エンドのシステムをしばらく構築してきました。私はこの仕事をとても誇りに思っています。
DGXというAIシステムがあります。
自律システム用のAGXと呼ばれる下位システムもあります。
世界初のロボティクス・プロセッサです。
私たちが最初にこれを作ったとき、人々は「君たちは何を作っているんだ?これはSOCで、ワンチップで、非常に低消費電力に設計されていますが、高速センサー処理とAI用に設計されています。自動車や動くものに変圧器を搭載したいのであれば、最適なコンピューターがあります。ジェットソンと呼ばれています。
DGXはAIのトレーニング用で、Jetsonは自律プロセッサです。
そしてその中間には、もう1台のコンピューターが必要です。
一方、大規模な言語モデルには、例文を提供し、強化学習による人間のフィードバックを行うという利点があります。ロボットの強化学習による人間のフィードバックとは?強化学習による物理的フィードバックです。そうやってロボットの位置を合わせます。そうすることで、ロボットが関節運動能力や操作能力を学習する際に、物理法則に正しく適応することを知ることができるのです。
そのため、
ロボットのためにデジタルで世界を表現する
シミュレーション・エンジンが必要なのです。
私たちはその仮想世界をオムニバースと呼んでいます。
オムニバースを動かすコンピューターはOVXと呼ばれています。
OVXは、コンピュータ自体はAzureクラウドでホストされています。
基本的に、私たちはこれら3つのもの、3つのシステムを構築しました。
その上に、それぞれのアルゴリズムがあります。
では、AIとOmniverseがどのように連携するか、
1つの超例をお見せしましょう。
これからお見せするのは、ちょっと非常識な例ですが、
とてもとても明日に近いものです。
それはロボット工学の建物です。
このロボットの建物は倉庫と呼ばれています。
ロボット工学棟の中には、いくつかの自律システムが設置されます。
自律システムの一部は人間と呼ばれるようになる。
フォークリフトと呼ばれる自律システムもある。
そしてこれらの自律システムは、もちろん自律的に相互に作用し合うことになる。そしてこの倉庫は、皆が危険な目に遭わないように見過ごされることになる。倉庫は基本的に航空管制官だ。そして何かが起こるのを察知するたびに、トラフィックをリダイレクトし、ロボットや人々に新しいウェイポイントを与える。この倉庫、この建物と話すこともできる。もちろん、話しかけることもできます。
やあ、SAPセンター、今日の調子はどうだい?とか。
倉庫に同じ質問をすることもできます。
基本的に、今説明したシステムにはOmniverse Cloudがホスティングされており、仮想シミュレーションとAIがDGX Cloud上で実行され、これらすべてがリアルタイムで実行されます。
見てみよう。
重工業の未来はデジタル・ツインから始まる。
ロボット、労働者、インフラが複雑な産業空間で予測不可能な出来事をナビゲートするのを助けるAIエージェントは、まず洗練されたデジタルツインで構築され、評価される。この10万平方フィートの倉庫のオムニバースデジタルツインは、デジタルワーカー、NVIDIA ISAACレセプタースタックを実行するAMR、NVIDIA Metropolisを使用した100台のシミュレートされた天井マウントカメラからの倉庫全体の集中アクティビティマップ、およびNVIDIA Co-opを使用したAMRルートプランニングを統合したシミュレーション環境として動作しています。この物理的に正確なシミュレート環境におけるAIエージェントのソフトウェア・イン・ループ・テストにより、システムが現実世界の予測不可能な状況にどのように適応するかを評価し、改良することができます。ここでは、AMRがパレットをピックアップするために移動する際に、AMRの計画されたルート上でアクシデントが発生し、その経路がブロックされます。NVIDIA Metropolisはリアルタイムで占有マップを更新し、CoOptに送信します。AMRは隅々まで見渡すことができるようになり、ミッションの効率が向上します。生成的なAIを搭載したMetropolis Vision Foundationのモデルにより、オペレータは自然言語を使って質問することもできます。ビジュアルモデルはニュアンスの異なる活動を理解し、オペレーションを改善するための洞察を即座に提供することができる。センサーデータはすべてシミュレーションで作成され、NVIDIA Inference Microservices(NEMS)として実行されているリアルタイムのAIに渡されます。そして、AIが実際の倉庫である物理的なツインに導入される準備ができたら、MetropolisとIsaac Nimsを実際のセンサーに接続し、デジタルツインとAIモデルの両方を継続的に改善できるようにします。
信じられませんか?
それで...未来の施設、倉庫、工場、ビルはソフトウェアで定義されることを忘れないでください。だからソフトウェアが動いている。
他にどうやってソフトウェアをテストするのですか?つまり、デジタルツインで倉庫や最適化システムを構築するためのソフトウェアをテストするわけです。
ロボットについてはどうですか?
先ほどご覧になったロボットはすべて、
独自の自律型ロボット・スタックを稼働させています。
ですから、将来的にソフトウェアを統合する方法、
つまりロボット・システムのためのCICDは、
デジタル・ツインを使うことになります。
我々はOmniverseへのアクセスをより簡単にしました。
基本的にOmniverseのクラウドAPIを作成し、チャネルに4つのシンプルなAPIを用意し、アプリケーションを接続できるようにします。ですから、Omniverseは将来的に素晴らしく、美しくシンプルなものになるでしょう。そしてこれらのAPIを使えば、魔法のようなデジタルツイン機能を手に入れることができる。私たちはオムニバースをAI化しました。私たちはオムニバースをAI化し、人間とオムニバースの共通言語である米ドルで会話する機能を統合しました。そのため、特定のオブジェクトや特定の条件、特定のシナリオを尋ねることができ、そのシナリオを探してきてくれる。また、生成においてあなたと協力することもできます。あなたは3Dで何かをデザインすることができます。3Dでシミュレーションすることもできるし、AIを使って3Dで何かを生成することもできる。これがどのように機能するか見てみましょう。
私たちはシーメンスと素晴らしいパートナーシップを結んでいます。
シーメンスは世界最大の産業エンジニアリング・オペレーション・プラットフォームです。
現在、産業界にはさまざまな企業があります。
重工業はITの最大の最終フロンティアのひとつです。
そして、私たちは今ようやく、実際にインパクトを与えるために必要なテクノロジーを手に入れたのです。
シーメンスは産業用メタバースを構築しています。
そして今日、私たちはシーメンスが彼らのクラウン・ジュエル・アクセラレータをNVIDIA Omniverseに接続することを発表します。
見てみましょう。
シーメンスのテクノロジーは、すべての人のために日々変革しています。
シーメンス・アクセラレータ・プラットフォームの製品ライフサイクル管理ソフトウェアであるTeamcenter Xは、大規模な製品を開発し提供するために、私たちの顧客によって毎日使用されています。
NVIDIAのAIとOmnibusの技術をTeamcenter Xに統合することで、
現実とデジタルの世界をさらに近づけることができます。
OmnibusのAPIは、
データの相互運用性と物理ベースのレンダリングを産業スケールの設計および製造プロジェクトに提供します。
当社の顧客であるHD&A社は、持続可能な船舶製造のマーケットリーダーであり、アンモニアと水素を動力源とする船舶を建造しています。
オムニバースのAPITeamcenter Xにより、
HD Hyundaiのような企業は、これらの膨大な
エンジニアリングデータセットを統合してインタラクティブに視覚化し、
ジェネレーティブAIを統合して3DオブジェクトやHDRI背景を生成し、
プロジェクトの状況を確認することができます。
その結果は?超直感的でフォトリアル、物理ベースのデジタル・ツインにより、無駄やエラーを排除し、コストと時間の大幅な削減を実現します。また、Siemens NXやStar CCM PlusのようなSiemens Acceleratorツール間であれ、同じシーンでお気に入りのデバイスに取り組むチーム間であれ、コラボレーションのためにこれを構築しています。これは始まりに過ぎません。NVIDIAと協力し、シーメンス・アクセラレータのポートフォリオ全体にアクセラレータ・コンピューティング、ジェネレーティブAI、Omniverseの統合をもたらします。
プロの声優は、偶然にもシーメンスのCEOである私の親友、
ローランド・ブッシュです。
Omniverseをワークフローやエコシステムに組み込めば、
設計の初期段階からエンジニアリング、製造計画、
デジタル・ツイン・オペレーションに至るまで、すべてがつながります。
すべてをつなげれば、生産性が飛躍的に向上します。
それは本当に素晴らしいことです。
突然、すべての人が同じ真実の土台の上で動くようになる。
データを交換したり、変換したり、間違いを犯したりする必要がない。
誰もが同じ真実に基づいて仕事をしている。
デザイン部門からアート部門、建築部門、エンジニアリング部門、
そしてマーケティング部門まで。
日産がどのようにオムニバースをワークフローに統合したかを見てみよう。
あれはアニメーションではない。あれはオムニバースだ。
今日、
私たちはオムニバース・クラウドが
Vision Proにストリーミング配信されることを発表します。
私が車から降りるとき、
バーチャルドアの周りを歩くのはとても奇妙なことです。
そして、みんなそれをやっている。本当に、本当に驚きです。
Vision ProはOmniverseに接続されており、
Omniverseに入ることができます。
そして、これらのCADツールやさまざまなデザインツールがすべて統合され、Omniverseに接続されているので、このようなワークフローを実現できるのです。本当に素晴らしいことです。ロボット工学について話しましょう。動くものはすべてロボット化されるでしょう。それは間違いない。より安全で、より便利です。そして、最大の産業のひとつは自動車になるでしょう。私たちは上から下へとロボット・スタックを構築します。先ほど申し上げたように、コンピューター・システムから、自動運転車の場合は自動運転アプリケーションも含めてです。今年の終わりか来年の初めにはメルセデスに、そしてそのすぐ後にはJLRにも出荷する予定です。これらの自律走行ロボットシステムはソフトウェアで定義されています。コンピュータ・ビジョン、人工知能、制御、プランニングなど、あらゆる種類の非常に複雑な技術を駆使し、洗練させるのに何年もかかります。我々はスタック全体を構築している。しかし、私たちはスタック全体を自動車産業全体に開放しています。これが私たちの仕事のやり方です。どの業界においても、私たちはできる限り多くのものを構築し、それを理解するように努めています。
AIを実行できる世界で唯一の完全で機能的、
安全なASIL-Dシステムである私たちのコンピューターだけを購入したいかどうか。この機能的で安全なASIL-D品質のコンピュータ、またはその上のオペレーティング・システム、あるいはもちろん私たちのデータ・センター(基本的に世界中のすべてのAV企業に設置されています)、どのような楽しみ方をしていただいても、私たちは大歓迎です。
今日、世界最大のEV会社であるBYDが、
私たちの次世代を採用することを発表します。
トールという名前です。
トールはトランスエンジン用に設計されています。
私たちの次世代AVコンピューター「トール」がBYDに採用される。
皆さんはおそらくご存じないと思いますが、
私たちには100万人以上のロボット開発者がいます。
私たちはこのロボットコンピュータ、ジェットソンを作りました。
私たちはそれをとても誇りに思っています。
その上に乗っているソフトウェアの量は、正気の沙汰ではありません。
しかし、なぜそれができるかというと、100%CUDA互換だからです。
私たちが行うこと、私たちの会社で行うことはすべて、開発者のためのものです。そして、私たちがこの豊かなエコシステムを維持し、あなたが私たちからアクセスするすべてのものと互換性を持たせることで、私たちはこの小さなコンピュータに信じられないような能力のすべてをもたらすことができるのです。私たちはJetsonをロボティクス・コンピューターと呼んでいます。
また本日、信じられないほど先進的な新しいSDKを発表します。
私たちはこれをアイザック・パーセプターと呼んでいます。
アイザック・パーセプターは、今日のほとんどのロボットがあらかじめプログラムされています。地上のレールやデジタルレールに従うか、4月のタグに従うかのどちらかです。でも将来的には、ロボットは知覚を持つようになる。なぜそれを望むかというと、簡単にプログラムできるからだ。A地点からB地点に行きたいと言えば、そこまでのナビゲーションを考えてくれる。つまり、ウェイポイントをプログラムするだけで、ルート全体を適応させることができる。最初に倉庫でお見せしたように、環境全体をプログラムし直すことができるのです。事前にプログラムされた無人搬送車では、このようなことはできません。箱が倒れれば、箱はすべてぐちゃぐちゃになり、誰かがそれを片付けに来るのを待つだけです。アイザック・パーセプターは、最先端のオドメトリー、3D再構築、そして3D再構築に加えて奥行き知覚を備えています。その理由は、世界で何が起きているのかを監視するために、2つのモダリティを持つことができるからです。
アイザック・パーセプター現在、最も使われているロボットはマニピュレーター、製造アームで、これらもあらかじめプログラムされています。コンピューター・ビジョンのアルゴリズム、AIのアルゴリズム、ジオメトリーを意識した制御やパス・プランニングのアルゴリズムは、信じられないほど計算集約的です。私たちはこれらをCUDAアクセラレーションで実現しました。そのため、ジオメトリを認識する世界初のCUDAアクセラレーションによるモーションプランナーを開発しました。目の前に何かを置くと、新しいプランを考え出し、その周囲をアーティキュレーションします。また、3Dオブジェクトのポーズ推定にも優れています。単に2Dでのポーズではなく、3Dでのポーズだ。そのため、周囲に何があるのか、どのようにつかむのがベストなのかを想像しなければならない。そのため、基礎となるポーズ、グリップの基礎、そして関節のアルゴリズムが利用できるようになりました。私たちはこれをアイザック・マニピュレーターと呼んでいます。また、これらはNVIDIAのコンピュータ上で動作します。私たちは次世代のロボット工学において、本当に素晴らしい仕事を始めています。
次世代のロボット工学は、
おそらくヒューマノイド・ロボットになるでしょう。
私たちは今、必要な技術を持っており、先ほど説明したように、一般化された人間型ロボットを想像するのに必要な技術を持っています。ある意味、人間型ロボットの方が簡単かもしれません。その理由は、私たちは非常によく似た構造をしているため、ロボットに提供できる模倣訓練データがたくさんあるからです。ヒューマノイド・ロボットは、私たちの世界においてより有用なものになる可能性が高い。私たちがワークステーションや製造、ロジスティクスを設置する方法は、人間のために設計されたものです。人間のために設計されたのです。ですから、このようなヒューマノイド・ロボットは、より生産的に導入することができるでしょう。私たちは、他のロボットと同じように、スタック全体を構築しています。まず基礎となるモデルは、ビデオや人間の例を見て学習します。それはビデオ形式かもしれない。バーチャルリアリティの形でもいい。そして、アイザック強化学習ジムと呼ばれる、ヒューマノイドロボットが物理的な世界に適応する方法を学習するためのジムを作りました。そして、ロボットカーに搭載されるのと同じ、驚異的なコンピューターが、トールという人型ロボットの中で稼働します。これはトランスフォーマーエンジン用に設計されています。これらのいくつかを1つのビデオにまとめました。きっと気に入っていただけるはずです。ご覧ください。
人間は想像するだけでは十分ではありません。
私たちは発明し、探求し、既成のものを超えていかなければならない。
より賢く、より速く創造する。失敗させ、学ばせる。私たちはそれを教える。新たな挑戦に絶対的な精度で挑み、成功させるために、理解を広げる。そして、知覚し、動き、理性さえも持たせ、私たちと世界を共有できるようにする。これがインスピレーションが私たちを導く場所であり、次のフロンティアです。これがNVIDIA Project Rootです。ヒューマノイドロボット学習のための汎用基礎モデル。グループモデルは、マルチモーダルな指示と過去のインタラクションを入力とし、ロボットが実行すべき次のアクションを生成します。私たちは、Omniverse Isaac SimでGrootを訓練するためのロボット学習アプリケーション、Isaac Labを開発しました。そして、トレーニング用のDGXシステムとシミュレーション用のOVXシステム間でワークフローを調整する新しいコンピュート・オーケストレーション・サービスであるOsmoでスケールアウトしました。これらのツールを使うことで、物理ベースのシミュレーションでグルートを訓練し、ゼロショットを実世界に転送することができます。グルート・モデルは、ロボットが人間の手によるデモンストレーションから学習することを可能にし、日常的な作業を支援できるようにします。
これは、動画から人間を理解し、モデルとシミュレーションを訓練し、
最終的には物理的なロボットに直接展開することができるエヌビディアの技術によって可能になります。
Grootを大規模な言語モデルに接続することで、自然言語の指示に従って動作を生成することさえ可能になります。やあ、GL1。ハイタッチしていいよ。ハイタッチしよう。かっこいい動きをしてくれる?もちろんこの驚異的な知性はすべて 新しいジェットソン・ソー ロボット船によって動かされる グルートのために設計され 未来のために作られるアイザック・ラボ、オスモ、グルートで次世代のAIロボット工学の構成要素を提供しています。
ほぼ同じ大きさ。
エヌビディアの魂、コンピュータグラフィックス、物理学、
人工知能の交差点。そのすべてがこの瞬間に結実した。
そのプロジェクトの名前、ジェネラル・ロボティクス003。
分かってる、超いい。超いい。
さて、特別ゲストをお招きしましょう。そうなのか?やあ君たちはジェットソンで動いてるんだろ?ジェットソン・ロボット・コンピューターだアイザック・シムで 歩けるようになったんだ皆さん、これがオレンジ、そしてこれが有名なグリーンです。ディズニーのBDXロボットです。驚くべきディズニーの研究。さあ、皆さん、まとめましょう。
行こう5つのことどこに行くの?5つのことここに座る怖がらないで来なさい、グリーン。急げ何言ってるの?食べる時間じゃない。食べる時間じゃない。すぐにおやつをあげるから。早く終わらせて。さあ、グリーン、急いで。時間を無駄にしないで。
5つだ。5つだ
第一に、
新しい産業革命。
すべてのデータセンターを加速させること。
兆ドル相当のデータセンターが今後数年間で近代化される。
第二に、
我々がもたらした計算能力のおかげで、ソフトウェアの新しいやり方が出現した。ジェネレーティブAIは、ひとつのことだけを行うことに特化した新しいインフラを生み出すだろう。マルチユーザーデータセンターではなく、AIジェネレーターだ。これらのAI世代は、信じられないほど価値のあるソフトウェアを生み出すだろう。新たな産業革命だ。
第二に、この革命のコンピューターは...この世代のコンピューター、ジェネレーティブAI、兆単位のパラメーター、ブラックウェル。非常識な量のコンピューターとコンピューティング。
第三に、
集中しようとしている。よくやったサード新しいコンピューターは新しいタイプのソフトウェアを生み出す。新しいタイプのソフトウェアは、一方ではクラウドのエンドポイントとして使いやすく、他方では自分のインテリジェンスであるため持ち運びができるように、新しい方法で配布されるべきである。インテリジェンスは持ち運べるようにパッケージ化されるべきなのです。私たちはこれをNIMSと呼んでいる。
第三に、これらのNIMSは、将来に向けて新しいタイプのアプリケーションを作るのに役立ちます。NIMS、AIテクノロジー、ツール、NEMO、そしてインフラストラクチャーであるDGX Cloudの間に素晴らしい機能があり、私たちのAIファウンドリーで、独自のアプリケーションや独自のチャットボットを作ることができます。そして最後に、将来的に動くものはすべてロボットになるでしょう。あなただけではありません。これらのロボットシステムは、ヒューマノイド、AMR、自動運転車、フォークリフト、操作アームなど、その種類を問いません。必要なものは1つだ。巨大スタジアム、倉庫、工場。ロボット化された工場、ロボット化された工場の指揮、ロボット化された製造ライン、ロボット化された自動車の製造。これらのシステムには、1つのものが必要だ。それはプラットフォーム、デジタル・プラットフォーム、デジタル・ツイン・プラットフォームであり、私たちはこれをオムニバースと呼んでいる。
これが今日お話しした5つのことです。
エヌビディアはどう見えるか?
エヌビディアはどのような会社ですか?
GPUの話をするとき、
GPUについて聞かれたときに私が抱くイメージは大きく異なります。
まず、ソフトウェア・スタックやそのようなものの束を目にします。そしてもうひとつは、これです。これが今日発表したものです。
これがBlackwellです。これがプラットフォームです。
驚くべきプロセッサー、MV-Linkスイッチ、ネットワーキング・システム、そしてシステム設計は奇跡だ。
これがBlackwellだ。
これが私の中でのGPUの姿だ。
聞いてくれ、オレンジ、グリーン、
みんなにもう一つご馳走があると思うんだ。どう思う?どうする?
よし、もう一つ見せたいものがある。
巻いて。
それがいいありがとうありがとうありがとう素晴らしいGTCを!
ご来場ありがとうございました!ありがとうございました!
Live全文:英文
Please welcome to the stage, NVIDIA founder and CEO, Jensen Wong.
Welcome to GTC. I hope you realize this is not a concert. You have arrived at a developer's conference. There will be a lot of science described, algorithms, computer architecture, mathematics. I sensed a very heavy weight in the room all of a sudden, almost like you were in the wrong place. No conference in the world. Is there a greater assembly of researchers from such diverse fields of science? From climate tech to radio sciences trying to figure out how to use AI to robotically control MIMOs for next generation 6G radios. Robotic self-driving cars. Even artificial intelligence. Even artificial intelligence. Everybody's... First, I noticed a sense of relief there all of a sudden. Also, this conference is represented by some amazing companies. This list, this is not the attendees. These are the presenters. And what's amazing is this. If you take away all of my friends, close friends, Michael Dell is sitting right there, in the IT industry. All of the friends I grew up with in the industry. If you take away that list, this is what's amazing. These are the presenters of the non-IT industries using accelerated computing to solve problems that normal computers can't. It's represented in life sciences, healthcare, genomics, transportation, of course, retail, logistics, manufacturing, industrial. the gamut of industries represented is truly amazing. And you're not here to attend only, you're here to present, to talk about your research. $100 trillion of the world's industries is represented in this room today. This is absolutely amazing. There is absolutely something happening. There is something going on. the industry is being transformed, not just ours. Because the computer industry, the computer is the single most important instrument of society today, fundamental transformations in computing affects every industry. But how did we start? How did we get here? I made a little cartoon for you. Literally, I drew this. In one page, this is NVIDIA's journey. Started in 1993. this might be the rest of the talk. 1993, this is our journey. We were founded in 1993. There are several important events that happened along the way. I'll just highlight a few. In 2006, CUDA, which has turned out to have been a revolutionary computing model, we thought it was revolutionary then. It was going to be an overnight success, and almost 20 years later, it happened. We saw it coming. Two decades later. In 2012, AlexNet, AI, and CUDA made first contact. In 2016, recognizing the importance of this computing model, we invented a brand new type of computer. We called it DGX-1. 170 teraflops in this supercomputer. Eight GPUs connected together for the very first time. I hand delivered the very first DGX1 to a startup located in San Francisco called OpenAI. DGX1 was the world's first AI supercomputer. Remember 170 teraflops. 2017, the transformer arrived. 2022, chat GPT captured the world's imaginations, have people realize the importance and the capabilities of artificial intelligence. And 2023, generative AI emerged and a new industry. begins. Why? Why is a new industry? Because the software never existed before. We are now producing software, using computers to write software, producing software that never existed before. It is a brand new category. It took share from nothing. It's a brand new category. And the way you produce the software is unlike anything we've ever done before. In data centers, generating tokens, producing floating point numbers at very large scale. As if in the beginning of this last industrial revolution, when people realized that you would set up factories, apply energy to it. And this invisible, valuable thing called electricity came out. AC generators. And 100 years later, 200 years later, we are now creating new types of electrons, tokens, using infrastructure we call factories, AI factories, to generate this new, incredibly valuable thing called artificial intelligence. A new industry has emerged. Well... We're going to talk about many things about this new industry. We're going to talk about how we're going to do computing next. We're going to talk about the type of software that you build because of this new industry, the new software, how you would think about this new software. What about applications in this new industry? And then maybe what's next and how can we start preparing today for what is about to come next? Well, but before I start, I want to show you the soul of NVIDIA, the soul of our company, at the intersection of computer graphics, physics, and artificial intelligence, all intersecting inside a computer, in omniverse, in a virtual world simulation. Everything we're going to show you today, literally everything we're going to show you today. is a simulation, not animation. It's only beautiful because it's physics. The world is beautiful. It's only amazing because it's being animated with robotics. It's being animated with artificial intelligence. What you're about to see all day is completely generated, completely simulated, and omniverse, and all of it, what you're about to enjoy is the world's first concert where everything is homemade. Everything is homemade. You're about to watch some home videos. So sit back and enjoy yourself. Thank you. I think that's a good point. I think that's a good point. I think that's a great question. I think that's a great question. So, I'm going to take a few minutes to introduce you to the program. I'm going to introduce you to the program. I'm going to introduce you to the program. I'm going to introduce you to the program. I'm going to introduce you to the program. I'm going to introduce you to the program. I'm going to introduce you to the program. I'm going to introduce you God, I love him, did you? Accelerated computing has reached the tipping point. General purpose computing has run out of steam. We need another way of doing computing so that we can continue to scale, so that we can continue to drive down the cost of computing, so that we can continue to consume more and more computing while being sustainable. Accelerated computing is a dramatic speed-up over general purpose computing. And in every single industry we engage, and I'll show you many, the impact is dramatic. But in no industry is it more important than our own. The industry of using simulation tools to create products. In this industry, it is not about driving down the cost of computing. It's about driving up the scale of computing. We would like to be able to simulate the entire product that we do completely in full fidelity, completely digitally, and essentially what we call digital twins. We would like to design it, build it, simulate it, operate it completely digitally. In order to do that... we need to accelerate an entire industry. And today, I would like to announce that we have some partners who are joining us in this journey to accelerate their entire ecosystem so that we can bring the world into accelerated computing. But there's a bonus. When you become accelerated, your infrastructure is CUDA GPUs. And when that happens, it's exactly the same infrastructure for generative AI. And so I'm just delighted to announce several very important partnerships. They're some of the most important companies in the world. ANSYS does engineering simulation for what the world makes. We're partnering with them to CUDA accelerate the ANSYS ecosystem, to connect ANSYS to the Omniverse Digital Twin. Incredible. The thing that's really great is that the install base of NVIDIA GPU accelerated systems are all over the world, in every cloud, in every system. all over enterprises. And so the applications they accelerate will have a giant install base to go serve. End users will have amazing applications. And of course, system makers and CSPs will have great customer demand. Synopsys. Synopsys is NVIDIA's literally first software partner. They were there in the very first day of our company. Synopsys revolutionized the chip industry with high-level design. We are going to CUDA accelerate synopsis. We're accelerating computational lithography, one of the most important applications that nobody's ever known about. In order to make chips, we have to push lithography to the limit. NVIDIA has created a library, a domain-specific library, that accelerates computational lithography incredibly. Once we can accelerate and software-define all of TSMC, who is announcing today that they're going to go into production with NVIDIA Koolitho. Once it's software-defined and accelerated, the next step is to apply generative AI to the future of semiconductor manufacturing, pushing geometry even further. Cadence builds the world's essential EDA and SDA tools. We also use cadence. Between these three companies, Ansys, Synopsys, and Cadence, we basically build NVIDIA. Together we are CUDA accelerating Cadence. They're also building a supercomputer out of NVIDIA GPUs so that their customers could do fluid dynamic simulation at a hundred, a thousand times scale. Basically a wind tunnel in real time. Cadence Millennium, a supercomputer with NVIDIA GPUs inside. A software company building supercomputers. I love seeing that. Building Cadence co-pilots together. Imagine a day... When Cadence could synopsis ANSYS tool providers would offer you AI co-pilots so that we have thousands and thousands of co-pilot assistants helping us design chips, design systems. And we're also going to connect Cadence Digital Twin Platform to Omniverse. As you can see the trend here, we're accelerating. the world's CAE, EDA, and SDA, so that we could create our future in digital twins. And we're going to connect them all to Omniverse, the fundamental operating system for future digital twins. One of the industries that benefited tremendously from scale, and you all know this one very well, large language models. Basically, after the transformer was invented, we were able to scale large language models at incredible rates, effectively doubling every six months. Now, how is it possible that by doubling every six months that we have grown the industry, we have grown the computational requirements so far? And the reason for that is quite simply this. If you double the size of the model, you double the size of your brain, you need twice as much information to go fill it. And so every time you double your... parameter count, you also have to appropriately increase your training token count. The combination of those two numbers becomes the computation scale you have to support. The latest, the state-of-the-art OpenAI model is approximately 1.8 trillion parameters. 1.8 trillion parameters required several trillion tokens to go train. So, a few trillion parameters on the order of, a few trillion tokens on the order of, when you multiply the two of them together, approximately 30, 40, 50 billion, quadrillion floating point operations per second. Now, we just have to do some CO math right now. Just hang with me. So, you have 30 billion quadrillion. A quadrillion is like a peta. And so if you had a petaflop GPU, you would need 30 billion seconds to go compute, to go train that model. 30 billion seconds is approximately 1,000 years. Well, 1,000 years, it's worth it. I'd like to do it sooner, but it's worth it. which is usually my answer when most people tell me, hey, how long is it going to take to do something? 20 years? It's worth it. But can we do it next week? And so 1,000 years, 1,000 years. So what we need, what we need are bigger GPUs. we need much, much bigger GPUs. We recognized this early on. And we realized that the answer is to put a whole bunch of GPUs together. And of course, innovate a whole bunch of things along the way, like inventing tensor cores, advancing MV-Linux so that we could create essentially virtually giant GPUs. and connecting them all together with amazing networks from a company called Mellanox, Infiniband, so that we could create these giant systems. And so DGX1 was our first version, but it wasn't the last. We built supercomputers all the way, all along the way. In 2021, we had Selene, 4,500 GPUs or so. And then in 2023, we built one of the largest AI supercomputers in the world. It's just come online. EOS. And as we're building these things, we're trying to help the world build these things. And in order to help the world build these things, we got to build them first. We build the chips, the systems, the networking, all of the software necessary to do this. You should see these systems. Imagine writing a piece of software that runs across the entire system, distributing the computation across thousands of GPUs, but inside are thousands of smaller GPUs. millions of GPUs to distribute work across all of that and to balance the workload so that you can get the most energy efficiency, the best computation time, keep your costs down. And so those fundamental innovations is what got us here. And here we are, as we see the miracle of ChatGPT emerge in front of us, we also realize we have a long ways to go. we need even larger models. We're going to train it with multimodality data, not just text on the internet, but we're going to train it on text and images and graphs and charts. And just as we learn watching TV. And so there's going to be a whole bunch of watching video so that these models can be grounded in physics, understand that an arm doesn't go through a wall. And so these models would have common sense. by watching a lot of the world's video combined with a lot of the world's languages. They'll use things like synthetic data generation, just as you and I do. When we try to learn, we might use our imagination to simulate how it's going to end up, just as I did when I was preparing for this keynote. I was simulating it all along the way. I hope it's going to turn out as well as I had it in my head. as I was simulating how this keynote was going to turn out, somebody did say that another performer did her performance completely on a treadmill so that she could be in shape to deliver it with full energy. I didn't do that. If I get a little winded about 10 minutes into this, you know what happened. And so, where were we? We're sitting here using synthetic data generation. We're going to use reinforcement learning. We're going to practice it in our mind. We're going to have AI working with AI training each other, just like student, teacher, debaters. All of that is going to increase the size of our model. It's going to increase the amount of data that we have, and we're going to have to build even bigger GPUs. Hopper is fantastic, but we need bigger GPUs. And so, ladies and gentlemen. I would like to introduce you to a very, very big GPU. Named after David Blackwell. Mathematician. Game theorist. Probability. We thought it was a perfect name. Blackwell, ladies and gentlemen, enjoy this. Thank you. I'm going to go ahead and start with the question of how you can do this. I'm going to go ahead and start with the question of how you can do this. Blackwell is not a chip. Blackwell is the name of a platform. People think we make GPUs, and we do, but GPUs don't look the way they used to. Here's the, if you will, the heart of the Blackwell system. And this inside the company is not called Blackwell, it's just a number. And this, This is Blackwell sitting next to, oh, this is the most advanced GPU in the world in production today. This is Hopper. This is Hopper. Hopper changed the world. This is Blackwell. It's okay, Hopper. You're very good. Good boy. Good girl. 208 billion transistors. And so you could see, I can see, there's a small line between two dyes. This is the first time two dyes have abutted like this together in such a way that the two dyes think it's one chip. There's 10 terabytes of data between it, 10 terabytes per second, so that these two sides of the Blackwell chip have no clue which side they're on. there's no memory locality issues, no cache issues. It's just one giant chip. And so when we were told that Blackwell's ambitions were beyond the limits of physics, the engineer said, so what? And so this is what happened. And so this is the Blackwell chip, and it goes into two types of systems. The first one... Is form-fit function compatible to hopper? And so you slide on hopper and you push in blackwell. That's the reason why one of the challenges of ramping is going to be so efficient. There are installations of hoppers all over the world, and they could be the same infrastructure, same design, the power, the electricity, the thermals, the software. identical, push it right back. And so this is a hopper version for the current HGX configuration. And this is what the second hopper looks like this. Now, this is a prototype board. And Janine, could I just borrow? Ladies and gentlemen, Janine Paul. And so this is a fully functioning board, and I'll just be careful here. This right here is, I don't know, $10 billion. The second one's five. It gets cheaper after that, so any customers in the audience, it's okay. All right, but this one's quite expensive. This is the bring-up board. And the way it's going to go to production is like this one here, okay? And so you're going to take this. It has two Blackwell chips and four Blackwell dies connected to a Grace CPU. The Grace CPU has a super fast chip-to-chip link. What's amazing is this computer is the first of its kind where this much computation... First of all, fits into this small of a place. Second, it's memory coherent. They feel like they're just one big happy family working on one application together. And so everything is coherent within it. Just the amount of, you know, you saw the numbers. There's a lot of terabytes this and terabytes that. But this is a miracle. This is a, this, let's see, what are some of the things on here? There's a MVLink on top, PCI Express on the bottom. On your, which one is my, and your left? One of them, it doesn't matter. One of the. One of them is a CPU chip-to-chip link. It's my left or your, depending on which side. I was trying to sort that out, and I just kind of, doesn't matter. Hopefully it comes plugged in, so. Okay, so this is the Grace Blackwell system. But there's more. So it turns out, it turns out, all of the specs is fantastic, but we need a whole lot of new features. In order to push the limits beyond, if you will, the limits of physics, we would like to always get a lot more X factors. And so one of the things that we did was we invented another transformer engine, another transformer engine, the second generation. It has the ability to dynamically. and automatically rescale and recast. numerical formats to a lower precision whenever it can. Remember, artificial intelligence is about probability. And so you kind of have, you know, 1.7, approximately 1.7 times approximately 1.4 to be approximately something else. Does that make sense? And so the ability for the mathematics to retain the precision and the range necessary in that particular stage of the pipeline, super important. And so... This is not just about the fact that we designed a smaller ALU. The world's not quite that simple. You've got to figure out when you can use that across a computation that is thousands of GPUs. It's running for weeks and weeks and weeks, and you want to make sure that the training job is going to converge. And so this new transformer engine, we have a fifth generation NVLink. It's now twice as fast as Hopper, but very importantly, it has computation in the network. And the reason for that is because when you have so many different GPUs working together, we have to share our information with each other. We have to synchronize and update each other. And every so often, we have to reduce the partial products and then rebroadcast out the partial products, the sum of the partial products back to everybody else. And so there's a lot of what is called all reduce and all to all and all gather. It's all part of this area of synchronization and collectives so that we can have GPUs working with each other. Having extraordinarily fast links and being able to do mathematics right in the network allows us to essentially amplify even further. So even though it's 1.8 terabytes per second, it's effectively higher than that. And so it's many times that of Hopper. The likelihood of a supercomputer running for weeks on end is approximately zero. And the reason for that is because there's so many components working at the same time, the statistic, the probability of them working continuously is very low. And so we need to make sure that whenever there is a, well, we checkpoint and restart as often as we can, but if we have the ability to detect a weak chip or a weak node early, we can retire it and maybe swap in another processor. That ability to keep the utilization of the supercomputer high, especially when you just spent $2 billion building it, is super important. And so we put in a RAS engine, a reliability engine, that does 100% self-test, in-system test of every single gate, every single bit of memory on the Blackwell chip and all the memory that's connected to it. It's almost as if we shipped with every single chip its own advanced tester that we test our chips with. This is the first time we're doing this. Super excited about it. Secure AI. Only this conference today clapped for RAS. Secure AI. Obviously, you've just spent hundreds of millions of dollars creating a very important AI. And the code, the intelligence of that AI is encoded in the parameters. You want to make sure that on the one hand, you don't lose it. On the other hand, it doesn't get contaminated. And so we now have the ability to encrypt data, of course, at rest, but also in transit and while it's being computed. It's. all encrypted. And so we now have the ability to encrypt and transmission, and when we're computing it, it is in a trusted, trusted environment, trusted engine environment. And the last thing is decompression. Moving data in and out of these nodes when the compute is so fast becomes really essential. And so we've put in a high line speed compression engine and effectively moves data 20 times faster in and out of these computers. These computers are so powerful and they're such a large investment. The last thing we want to do is have them be idle. And so all of these capabilities are intended to keep Blackwell fed and as busy as possible. Overall, compared to Hopper. It is two and a half times, two and a half times the FP8 performance for training per chip. It also has this new format called FP6, so that even though the computation speed is the same, the bandwidth that's amplified because of the memory, the amount of parameters you can store in the memory is now amplified. FP4 effectively doubles the throughput. This is vitally important for inference. One of the things that is becoming very clear is that whenever you use a computer with AI on the other side, when you're chatting with the chatbot, when you're asking it to review or make an image, remember, in the back is a GPU generating tokens. Some people call it inference, but it's more appropriately generation. The way that computing has done in the past was retrieval. You would grab your phone, you would touch something, some signals go off, basically an e-mail goes off to some storage somewhere. There's pre-recorded content, somebody wrote a story or somebody made an image or somebody recorded a video. That pre-recorded content is then streamed back to the phone and recomposed in a way based on a recommender system to present the information to you. You know that in the future, the vast majority of that content will not be retrieved. And the reason for that is because that was pre-recorded by somebody who doesn't understand the context, which is the reason why we have to retrieve so much content. If you can be working with an AI that understands the context, who you are, for what reason you're fetching this information, and produces the information for you just the way you like it. the amount of energy we save, the amount of networking bandwidth we save, the amount of wasted time we save, will be tremendous. The future is generative, which is the reason why we call it generative AI, which is the reason why this is a brand new industry. The way we compute is fundamentally different. We created a processor for the generative AI era. And one of the most important parts of it is content token generation. We call it, this format is FP4. Well, that's a lot of computation. 5X, the token generation, 5X, the inference capability of Hopper seems like enough. But why stop there? The answer is it's not enough, and I'm going to show you why. I'm going to show you why. And so we would like to have a bigger GPU, even bigger than this one. And so we decided to scale it. And notice, but first let me just tell you how we've scaled. Over the course of the last eight years, we've increased computation by 1,000 times. Eight years, 1,000 times. Remember back in the good old days of Moore's Law. It was 2x, well, 5x every, what, 10x every five years. That's easiest math. 10x every five years, 100 times every 10 years. 100 times every 10 years in the middle of the heydays of the PC revolution. 100 times every 10 years. In the last eight years, we've gone 1,000 times. We have two more years to go. And so that puts it in perspective. The rate at which we're advancing computing is insane, and it's still not fast enough, so we built another chip. This chip is just an incredible chip. We call it the MV-Link switch. It's 50 billion transistors. It's almost the size of Hopper all by itself. This switch chip has four MV-Links in it. each 1.8 terabytes per second, and it has computation in it, as I mentioned. What is this chip for? If we were to build such a chip, we can have every single GPU talk to every other GPU at full speed at the same time. That's insane. it doesn't even make sense. But if you could do that, if you can find a way to do that and build a system to do that, that's cost effective. That's cost effective. How incredible would it be that we could have all these GPUs connect over a coherent link? so that they effectively are one giant GPU. Well, one of the great inventions in order to make it cost-effective is that this chip has to drive copper directly. The Surdeys of this chip is just a phenomenal invention so that we could do direct drive to copper. And as a result, you can build a system that looks like this. Now, this system is kind of insane. This is one DGX. This is what a DGX looks like now. Remember, just six years ago, it was pretty heavy, but I was able to lift it. I delivered the first DGX-1 to OpenAI, and the researchers there, the pictures are on the Internet, and we all autographed it. If you come to my office, it's autographed there, it's really beautiful. But you can lift it. This DGX, that DGX, by the way, was 170 teraflops. If you're not familiar with the numbering system, that's 0.17 petaflops. So this is 720. The first one I delivered to OpenAI was 0.17. You can round it up to 0.2, it won't make any difference. But back then it was like, wow, you know, 30 more teraflops. And so this is now 720 petaflops, almost an exaflop for training, and the world's first one exaflops machine in one rack. Just so you know, there are only a couple, two, three Exaflops machines on the planet as we speak. And so this is an Exaflops AI system in one single rack. Well, let's take a look at the back of it. So this is what makes it possible. That's the back, the DGX MV-Link spine. 130 terabytes per second. goes through the back of that chassis. That is more than the aggregate bandwidth of the Internet. So we could basically send everything to everybody within a second. And so we have 5,000 cables, 5,000 NVLink cables, in total, two miles. Now, this is the amazing thing. If we had to use optics, we would have had to use transceivers and retimers. And those transceivers and retimers alone would have cost 20,000 watts, two kilowatts of just transceivers alone, just to drive the MV-Link spine. As a result, We did it completely for free over MVLink Switch, and we were able to save the 20 kilowatts for computation. This entire rack is 120 kilowatts, so that 20 kilowatts makes a huge difference. It's liquid cooled. What goes in is 25 degrees C about room temperature. What comes out is 45 degrees C about your jacuzzi. So room temperature goes in, jacuzzi comes out, 2 liters per second. we could sell a peripheral. 600,000 parts. Somebody used to say, you know, you guys make GPUs, and we do, but this is what a GPU looks like to me. When somebody says GPU, I see this. Two years ago, when I saw a GPU, it was the HGX. It was 70 pounds, 35,000 parts. Our GPUs now are 600,000 parts. parts and 3,000 pounds. 3,000 pounds. 3,000 pounds, that's kind of like the weight of a, you know, carbon fiber Ferrari. I don't know if that's a useful metric, but everybody's going, I feel it. I feel it. I get it. I get that. Now that you mention that, I feel it. I don't know what's 3,000 pounds. Okay, so 3,000 pounds, ton and a half. So it's not quite an elephant. So this is what a DGX looks like. Now let's see what it looks like in operation. Okay, let's imagine, how do we put this to work and what does that mean? Well, if you were to train a GPT model, 1.8 trillion parameter model, it took about, apparently about three to five months or so with 25,000 amperes. If we were to do it with Hopper, it would probably take something like 8,000 GPUs and it would consume 15 megawatts. 8,000 GPUs and 15 megawatts. It would take 90 days, about three months. And that would allow you to train something that is, you know, this groundbreaking AI model. And this is obviously not as expensive as anybody would think, but it's 8,000 GPUs. It's still a lot of money. And so 8,000 GPUs, 15 megawatts. If you were to use Blackwell to do this, it would only take 2,000 GPUs. 2,000 GPUs, same 90 days. But this is the amazing part. only four megawatts of power. So from 15, that's right. And that's our goal. Our goal is to continuously drive down the cost and the energy. They're directly proportional to each other. Cost and energy associated with the computing so that we can continue to expand and scale up the computation that we have to do to train the next generation models. Well, this is training. Inference or generation is vitally important going forward. You know, probably some half of the time that NVIDIA GPUs are in the cloud these days, it's being used for token generation. You know, they're either doing copilot this or chat, you know, chat GPT that or all these different models that are being used when you're interacting with it or generating images or generating videos, generating proteins, generating chemicals. There's a bunch of generation going on. All of that is in the category of computing we call inference. But inference is extremely hard. for large language models, because these large language models have several properties. One, they're very large. And so it doesn't fit on one GPU. This is, imagine, imagine Excel doesn't fit on one GPU, you know, and imagine some application you're running on a daily basis doesn't run out, doesn't fit on one computer, like a video game doesn't fit on one computer. And most, in fact, do. And many times in the past, in hyperscale computing, many applications for many people fit on the same computer. And now, all of a sudden, this one inference application where you're interacting with this chatbot, that chatbot requires a supercomputer in the back to run it. And that's the future. The future is generative with these chatbots, and these chatbots are trillions of tokens, trillions of parameters, and they have to generate tokens At interactive rates now, what does that mean? Oh well? Three tokens is about a word You know the the You know space the final frontier these are the adventures that that's like that's like 80 tokens Okay, I don't know if that's useful to you and so You know, the art of communications is selecting good analogies. Yeah, this is not going well. I don't know what he's talking about. Never seen Star Trek. And so here we are, we're trying to generate these tokens. When you're interacting with it, you're hoping that the tokens come back to you as quickly as possible and as quickly as you can read it. And so the ability for generation tokens is really important. You have to parallelize the work of this model across many, many GPUs so that you could achieve several things. One, on the one hand, you would like throughput because that throughput reduces the cost, the overall cost per token of generating. So your throughput... dictates the cost of delivering the service. On the other hand, you have another interactive rate, which is another tokens per second, where it's about per user. And that has everything to do with quality of service. And so these two things compete against each other. And we have to find a way to distribute work across all of these different GPUs and parallelize it in a way that allows us to achieve both. And it turns out the search space is enormous. I told you there's going to be math involved. Everybody's going, oh dear. I heard some gasps just now when I put up that slide. So this right here, the y-axis is tokens per second, data center throughput. The x-axis is tokens per second, interactivity of the person. Notice the upper right is the best. You want interactivity to be very high. Number of tokens per second per user. You want the tokens per second per data center to be very high. The upper right is terrific. However, it's very hard to do that. And in order for us to search for the best answer across every single one of those intersections, X, Y coordinates, so just look at every single X, Y coordinate, all those blue dots came from some repartitioning of the software. Some optimizing solution has to go and figure out whether to use tensor parallel, expert parallel, pipeline parallel, or data parallel, and distribute this enormous model across all these different GPUs and sustain the performance that you need. this exploration space would be impossible if not for the programmability of NVIDIA's GPUs. And so we could, because of CUDA, because we have such a rich ecosystem, we could explore this universe and find that green roofline. It turns out that green roofline, notice you got TP2EP8DP4. It means two tensor parallel. Tenser parallel across two GPUs, expert parallel across eight, data parallel across four. Notice on the other end, you got tensor parallel across four and expert parallel across 16. The configuration, the distribution of that software, it's a different, different runtime that would produce these different results. And you have to go discover that roofline. Well, that's just one model. And this is just one configuration of a computer. Imagine all of the models being created around the world and all the different configurations of systems that are going to be available. So now that you understand the basics, let's take a look at inference of Blackwell compared to Hopper. And this is the extraordinary thing. In one generation, because we created a system that's designed for Trillium parameter generative AI, the inference capability of Blackwell is off the charts. And in fact, it is some 30 times hopper. Yeah. For large language models like ChatGPT and others like it, the blue line is Hopper. I gave you, imagine we didn't change the architecture of Hopper. We just made it a bigger chip. We just used the latest, you know, greatest 10 terabyte. You know, terabytes per second, we connected the two chips together, we got this giant 208 billion per annum chip. How would we have performed if nothing else changed? And it turns out quite wonderfully, quite wonderfully, and that's the purple line, but not as great as it could be. And that's where the FP4 Tensor Core, the new transformer engine, and very importantly, the NVLink switch. And the reason for that is because all these GPUs have to share the results, partial products. Whenever they do all-to-all, all-gather, whenever they communicate with each other, that NVLink switch is communicating almost 10 times faster than what we could do in the past using the fastest networks. Okay, so... Blackwell is going to be just an amazing system for generative AI. And in the future, in the future, data centers are going to be thought of, as I mentioned earlier, as an AI factory. An AI factory's goal in life is to generate revenues. Generate, in this case, intelligence in this facility. not generating electricity as in AC generators, but of the last industrial revolution and this industrial revolution, the generation of intelligence. And so this ability is super, super important. The excitement of Blackwell is really off the charts. You know, when we first, when we first, you know, this is a year and a half ago, two years ago, I guess two years ago, when we first started to go to market with Hopper. You know, we had the benefit of two CSPs joined us in a lunch, and we were, you know, delighted. And so we had two customers. We have more now. Unbelievable excitement for Blackwell. Unbelievable excitement. And there's a whole bunch of different configurations. Of course, I showed you the configurations that slide into the hopper form factor, so that's easy to upgrade. I showed you examples that are liquid-cooled, that are the extreme versions of it. One entire rack that's connected by MV-Link-72. Blackwell is going to be ramping to the world's AI companies, of which there are so many now, doing amazing work in different modalities. The CSPs, every CSP is geared up. All the OEMs and ODMs, regional clouds, sovereign AIs, and telcos all over the world are signing up to launch with Blackwell. Blackwell would be the most successful product launch in our history. And so I can't wait to see that. I want to thank some partners that are joining us in this. AWS is gearing up for Blackwell. They're going to build the first GPU with secure AI. They're building out a 222 exaflops system. You know, just now when we animated just now the digital twin, if you saw all of those clusters coming down. By the way. that is not just art. That is a digital twin of what we're building. That's how big it's going to be. Besides infrastructure, we're doing a lot of things together with AWS. We're CUDA accelerating SageMaker AI. We're CUDA accelerating Bedrock AI. Amazon Robotics is working with us using NVIDIA Omniverse and Isaac Sim. AWS Health has NVIDIA Health integrated into it. So AWS has really leaned into accelerated computing. Google is gearing up for Blackwell. GCP already has A100s, H100s, T4s, L4s. A whole fleet of NVIDIA CUDA GPUs, and they recently announced the GEMMA model that runs across all of it. We're working to optimize and accelerate every aspect of GCP. We're accelerating Dataproc for data processing, their data processing engine, JAX, XLA, Vertex AI, and Mujoko for robotics. So we're working with Google and GCP across a whole bunch of initiatives. Oracle is gearing up for Blackwell. Oracle is a great partner of ours. for NVIDIA DGX Cloud. And we're also working together to accelerate something that's really important to a lot of companies, Oracle Database. Microsoft is accelerating and Microsoft is gearing up for Blackwell. Microsoft NVIDIA has a wide-ranging partnership. We're accelerating CUDA, accelerating all kinds of services. When you chat, obviously, and AI services that are in Microsoft Azure, it's very, very likely NVIDIA is in the back doing the inference and the token generation. They built the largest NVIDIA InfiniBand supercomputer, basically a digital twin of ours or a physical twin of ours. We're bringing the NVIDIA ecosystem to Azure. NVIDIA DGRX Cloud to Azure. NVIDIA Omniverse is now hosted in Azure. NVIDIA Healthcare is in Azure. All of it is deeply integrated and deeply connected with Microsoft Fabric. The whole industry is gearing up for Blackwell. This is what I'm about to show you. Most of the scenes that you've seen so far of Blackwell are the full fidelity design of Blackwell. Everything in our company has a digital twin. And in fact, this digital twin idea is really spreading and it helps companies build very complicated things perfectly the first time. And what could be more exciting than... creating a digital twin to build a computer that was built in a digital twin. And so let me show you what Wistron is doing. To meet the demand for NVIDIA accelerated computing, Wistron, one of our leading manufacturing partners, is building digital twins of NVIDIA DGX and HGX factories using custom software developed with Omniverse SDKs and APIs. For their newest factory, Wistron started with the Digital Twin to virtually integrate their multi-CAD and process simulation data into a unified view. Testing and optimizing layouts in this physically accurate digital environment increased worker efficiency by 51%. During construction, the Omniverse Digital Twin was used to verify that the physical build matched the digital plans. Identifying any discrepancies early has helped avoid costly change orders. And the results have been impressive. Using a digital twin helped bring Wisdrawn's factory online in half the time, just two and a half months instead of five. In operation, the Omniverse Digital Twin helps Wistron rapidly test new layouts to accommodate new processes or improve operations in the existing space, and monitor real-time operations using live IoT data from every machine on the production line, which ultimately enabled Wistron to reduce end-to-end cycle times by 50% and defect rates by 40%. With NVIDIA AI and Omniverse, NVIDIA's global ecosystem of partners are building a new era of accelerated AI-enabled digitalization. That's the way it's going to be in the future when I'm manufacturing everything digitally first, and then we'll manufacture it physically. People ask me, how did it start? What got you guys so excited? What was it that you saw that caused you to put it all in on this incredible idea? And it's this. Hang on a second. Guys, that was going to be such a moment. That's what happens when you don't rehearse. This, as you know, was First Contact. 2012, AlexNet. You put a cat into this computer, and it comes out and it says, cat. And we said, oh my God, this is going to change everything. You take one million numbers across three channels, RGB. These numbers make no sense to anybody. You put it into this software, and it compresses, it dimensionally reduces it. It reduces it from a million dimensions, a million dimensions. It turns it into three letters, one vector, one number. And it's generalized. you could have the cat be different cats. And you could have it be the front of the cat and the back of the cat. And you look at this thing, you say, unbelievable. You mean any cats? Yeah, any cat. And it was able to recognize all these cats. And we realized how it did it. Systematically, structurally, it's scalable. How big can you make it? Well, how big do you want to make it? And so we imagine that this is a completely new way of writing software. And now today, as you know, you can have you type in the word C-A-T. And what comes out is a cat. It went the other way. Am I right? Unbelievable. how is it possible? That's right. How is it possible you took three letters and you generated a million pixels from it and it made sense? Well, that's the miracle. And here we are, just literally 10 years later, 10 years later, where we recognize text, we recognize images, we recognize videos and sounds and images. Not only do we recognize them, we understand their meaning. We understand the meaning of the text. That's the reason why it can chat with you. It can summarize for you. It understands the text. It understood, not just recognizes the English, it understood the English. It doesn't just recognize the pixels, it understood the pixels. And you can even condition it between two modalities. You can have language condition image and generate all kinds of interesting things. Well, if you can understand these things, what else can you understand that you've digitized? The reason why we started with text and images is because we digitized those. But what else have we digitized? Well, it turns out we digitized a lot of things. Proteins and genes and brain waves. Anything you can digitize, so long as there's structure, we can probably learn some patterns from it. And if we can learn the patterns from it, we can understand its meaning. If we can understand its meaning, we might be able to generate it as well. And so therefore, the generative AI revolution is here. Well, what else can we generate? What else can we learn? Well, one of the things that we would love to learn, we would love to learn, is we would love to learn climate. We would love to learn extreme weather. We would love to learn how we can predict future weather at regional scales, at sufficiently high resolution, such that we can keep people out of harm's way before harm comes. Extreme weather cost the world $150 billion. Surely more than that, it's not evenly distributed. $150 billion is concentrated in some parts of the world, and of course, to some people of the world. We need to adapt, and we need to know what's coming. And so we're creating Earth 2, a digital twin of the Earth for predicting weather, and we've... made an extraordinary invention called CoreDiv, the ability to use generative AI to predict weather at extremely high resolution. Let's take a look. As the Earth's climate changes, AI-powered weather forecasting is allowing us to more accurately predict and track severe storms, like Super Typhoon Chanthu, which caused widespread damage in Taiwan and the surrounding region in 2021. Current AI forecast models can accurately predict the track of storms, but they are limited to 25 km resolution, which can miss important details. NVIDIA's CORDIF is a revolutionary new generative AI model trained on high-resolution, radar-assimilated, dwarf weather forecasts and ERA5 reanalysis data. Using Cordiff, extreme events like Chanthu can be super resolved from 25km to 2km resolution, with 1000 times the speed and 3000 times the energy efficiency of conventional weather models. By combining the speed and accuracy of NVIDIA's weather forecasting model ForecastNet and generative AI models like Cordiff, we can explore hundreds or even thousands of kilometer scale regional weather forecasts to provide a clear picture of the best, worst and most likely impacts of a storm. This wealth of information can help minimize loss of life and property damage. Today, CORDiF is optimized for Taiwan, but soon, generative supersampling will be available as part of the NVIDIA Earth-2 inference service for many regions across the globe. The weather company has to trust the source of global weather prediction. We are working together to accelerate their weather simulation. First principled base of simulation. However, they're also going to integrate Earth to CoreDiff so that they can help businesses and countries do regional high resolution weather prediction. And so if you have some weather prediction you'd like to know, like to do, reach out to the weather company. Really exciting, really exciting work. NVIDIA Healthcare. Something we started 15 years ago. We're super, super excited about this. This is an area where we're very, very proud. Whether it's medical imaging or gene sequencing or computational chemistry, it is very likely that NVIDIA is the computation behind it. We've done so much work in this area. Today, we're announcing that we're going to do something really, really cool. Imagine all of these AI models that are being used. to generate images and audio, but instead of images and audio, because it understood images and audio, all the digitization that we've done for genes and proteins and amino acids, that digitization capability is now passed through machine learning so that we understand the language of life. The ability to understand the language of life, of course, we saw the first evidence of it with AlphaFold. This is really quite an extraordinary thing. After decades of painstaking work, the world had only digitized and reconstructed using cryo-electron microscopy or x-ray crystallography. These different techniques painstakingly reconstructed the protein, 200,000 of them, in just, what is it, less than a year or so? Alpha-fold. has reconstructed 200 million proteins. Basically, every protein, every living thing that's ever been sequenced. This is completely revolutionary. Well, those models are incredibly hard to use, incredibly hard for people to build. And so what we're going to do is we're going to build them. We're going to build them for the researchers around the world. And it won't be the only one. There'll be many other models that we create. And so let me show you what we're going to do with it. Virtual screening for new medicines is a computationally intractable problem. Existing techniques can only scan billions of compounds and require days on thousands of standard compute nodes to identify new drug candidates. NVIDIA Bionemo NIMS enable a new generative screening paradigm. Using NIMS for protein structure prediction with AlphaFold, molecule generation with MolMIM, and docking with DiffDock, we can now generate and screen candidate molecules in a matter of minutes. MolMIM can connect to custom applications to steer the generative process, iteratively optimizing for desired properties. These applications can be defined with Bionemo microservices or built from scratch. Here, a physics-based simulation optimizes for a molecule's ability to bind to a target protein while optimizing for other favorable molecular properties in parallel. MolMIM generates high-quality drug-like molecules that bind to the target and are synthesizable, translating to a higher probability of developing successful medicines faster. BioNemo is enabling a new paradigm in drug discovery with NIMS, providing on-demand microservices that can be combined to build powerful drug discovery workflows like de novo protein design or guided molecule generation for virtual screening. BioNemo NIMS are helping researchers and developers reinvent computational drug design. NIMS is helping researchers and developers reinvent computational drug design. MoMEM, CoreDiff, there's a whole bunch of other models. A whole bunch of other models. Computer vision models, robotics models, and even, of course, some really, really terrific open source language models. These models are groundbreaking. However, it's hard for companies to use. How would you use it? How would you bring it into your company and integrate it into your workflow? How would you package it up and run it? Remember, earlier I just said, here's what we need to do. that inference is an extraordinary computation problem. How would you do the optimization for each and every one of these models and put together the computing stack necessary to run that supercomputer so that you can run these models in your company? And so we have a great idea. We're going to invent a new way for you to receive and operate software. This software... comes basically in a digital box. We call it a container. And we call it the NVIDIA Inference Microservice, a NIM. And let me explain to you what it is. A NIM. It's a pre-trained model. So it's pretty clever. And it is packaged and optimized to run across NVIDIA's installed base, which is very, very large. What's inside it is incredible. You have all these pre-trained, state-of-the-art open source models. They could be open source. They could be from one of our partners. It could be created by us, like NVIDIA Moment. It is packaged up with all of its dependencies. So CUDA, the right version. CUDNN, the right version. TensorRT, LLM, distributed across the multiple GPUs. Trident Inference Server, all completely packaged together. It's optimized. Depending on whether you have a single GPU, multi-GPU, or multi-node of GPUs, it's optimized for that. And it's connected up with APIs that are simple to use. Now, think about what an AI API is. An AI API is an interface that you just talk to. And so this is a piece of software in the future that has a really simple API. And that API is called human. And these packages, incredible bodies of software, will be optimized and packaged, and we'll put it on a website. And you can download it. You can take it with you. You can run it in any cloud. You can run it in your own data center. You can run it in workstations if it fit. And all you have to do is come to ai.nvidia.com. We call it NVIDIA Inference Microservice, but inside the company, we all call it NIMS. Okay? Just imagine, you know, one of some, someday there's going to be one of these chatbots, and these chatbots is going to just be in a NIM. And you'll assemble a whole bunch of chatbots. And that's the way software is going to be built someday. How do we build software in the future? It is unlikely that you'll write it from scratch or write a whole bunch of Python code or anything like that. It is very likely that you assemble a team of AIs. There's probably going to be a super AI that you use that takes the mission that you give it and breaks it down into an execution plan. Some of that execution plan could be handed off to another NIM. That NIM would maybe understand SAP. The language of SAP is ABAP. It might understand ServiceNow and go retrieve some information from their platforms. It might then hand that result to another NIM who goes off and does some calculation on it. Maybe it's an optimization software, a combinatorial optimization algorithm. Maybe it's just some basic calculator. Maybe it's Pandas to do some numerical analysis on it. And then it comes back with its answer. and it gets combined with everybody else's, and because it's been presented with, this is what the right answer should look like, it knows what right answers to produce, and it presents it to you. We can get a report every single day, you know, top of the hour, that has something to do with a build plan or some forecast or some customer alert or some bugs database or whatever it happens to be, and we could assemble it using all these NIMs. And because these NIMs have been packaged up in... ready to work on your systems, so long as you have NVIDIA GPUs in your data center or in the cloud, this NIMS will work together as a team and do amazing things. And so we decided, this is such a great idea, we're going to go do that. And so NVIDIA has NIMS running all over the company. We have chatbots being created all over the place, and one of the most important chatbots, of course, is a chip designer chatbot. You might not be surprised, we care a lot about building chips. And so we want to build chatbots, AI co-pilots that are co-designers with our engineers. And so this is the way we did it. So we got ourselves a Lama 2. This is a 70B, and it's packaged up in a NIM. And we asked it, you know, what is a CTL? It turns out CTL is an internal program and it has an internal proprietary language, but it thought the CTL was a combinatorial timing logic, and so it describes conventional knowledge of CTL. But that's not very useful to us, and so we gave it a whole bunch of new examples. You know, this is no different than employee, onboarding an employee. We say, you know, thanks for that answer. It's completely wrong. And then we present to them, this is what a CTL is. Okay. And so this is what a CTL is at NVIDIA. And the CTL, as you can see, you know, CTL stands for compute trace library, which makes sense. You know, we're tracing compute cycles all the time. And it wrote the program. Isn't that amazing? And so the productivity of our chip designers can go up. This is what you can do with a NIM. First thing you can do with it is customize it. We have a service called NEMO Microservice that helps you curate the data, preparing the data so that you could teach this, onboard this AI. You fine tune them and then you guard rail it. You can even evaluate the answer, evaluate its performance against other examples. And so that's called the NEMO Microservice. Now, the thing that's emerging here is this. There are three elements, three pillars of what we're doing. The first pillar is, of course, inventing the technology for AI models and running AI models and packaging it up for you. The second is to create tools to help you modify it. First is having the AI technology. Second is to help you modify it. And third is infrastructure for you to fine tune it. And if you like, deploy it. You could deploy it on our infrastructure called DGX Cloud or you can deploy it on-prem. You can deploy it anywhere you like. Once you develop it, it's yours to take anywhere. And so we are effectively an AI foundry. we will do for you and the industry on AI what TSMC does for us building chips. And so we go to TSMC with our big ideas. They manufacture it, and we take it with us. And so exactly the same thing here. AI Foundry, and the three pillars are the NIMS, NEMO microservice, and DGX Cloud. The other thing that you could teach the NIM to do is to understand your proprietary information. Remember, inside our company, the vast majority of our data is not in the cloud. It's inside our company. It's been sitting there, you know, being used all the time and, gosh, it's basically NVIDIA's intelligence. We would like to take that data, learn its meaning, like we learned the meaning of almost anything else that we just talked about, learn its meaning, and then re-index that knowledge into a new type of database called a vector database. And so you essentially take structured data or unstructured data, you learn its meaning, you encode its meaning, so now this becomes an AI database, and that AI database, in the future, once you create it, you can talk to it. And so let me give you an example of what you could do. So suppose you've got a whole bunch of multi-modality data, and one good example of that is PDF. So you take the PDF, you take all of your PDFs, all your favorite, the stuff that is proprietary to you, critical to your company, you can encode it just as we encode the pixels of a cat, and it becomes the word cat. We can encode all of your PDF and it turns into vectors that are now stored inside your vector database it becomes the proprietary information of your company and once you have that proprietary information you can chat to it it's an it's a smart database so you just chat with data and how how much more enjoyable is that you know for for our software team you know they just chat with the bugs database you know how many bugs was there last night are we making any progress and then after you're done talking to this bugs database you need therapy and so so we have another chat bot for you you can do it Okay, so we call this Nemo Retriever. And the reason for that is because ultimately its job is to go retrieve information as quickly as possible. And you just talk to it. Hey, retrieve me this information. It goes, oh, it brings it back to you. Is it, do you mean this? You go, yeah, perfect. Okay, and so we call it the Nemo Retriever. Well, the Nemo service helps you create all these things. And we have all these different NIMs. We even have NIMs of digital humans. I'm Rachel, your AI care manager. Okay, so it's a really short clip, but there were so many videos to show you, I guess, so many other demos to show you, and so I had to cut this one short. But this is Diana. She is a digital human nim. And you just talked to her, and she's connected, in this case, to Hippocratic AI's large language model for healthcare. And it's truly amazing. She is just super smart about healthcare things. You know, and so after you're done, after my Dwight, my VP of software engineering talks to the chat bot for bugs database, then you come over and talk to Diane. And so Diane is completely animated with AI and she's a digital human. There's so many companies that would like to build. They're sitting on gold mines. The enterprise IT industry is sitting on a gold mine. It's a gold mine because they have so much understanding of the way work is done. They have all these amazing tools that have been created over the years, and they're sitting on a lot of data. If they could take that gold mine and turn them into co-pilots, these co-pilots could help us do things. And so just about every IT franchise, IT platform in the world that has valuable tools that people use is sitting on a goldmine for co-pilots. And they would like to build their own co-pilots and their own chatbots. And so we're announcing that NVIDIA AI Foundry is working with some of the world's great companies. SAP generates 87% of the world's global commerce. Basically, the world runs on SAP. We run on SAP. NVIDIA and SAP are building SAP Jewel co-pilots. using NVIDIA NEMO and DGX Cloud. ServiceNow, they run 85% of the world's Fortune 500 companies run their people and customer service operations on ServiceNow. And they're using NVIDIA AI Foundry to build ServiceNow Assist virtual assistants. Cohesity backs up the world's data. They're sitting on the goldmine of data. Hundreds of exabytes of data, over 10,000 companies. NVIDIA AI Foundry is working with them, helping them build their Gaia Generative AI agent. Snowflake is a company that stores the world's digital warehouse in the cloud and serves over three billion queries a day. for 10,000 enterprise customers. Snowflake is working with NVIDIA AI Foundry to build co-pilots with NVIDIA Nemo and NIMS. NetApp, nearly half of the files in the world are stored on-prem on NetApp. NVIDIA AI Foundry is helping them build chatbots and co-pilots like those vector databases and retrievers with NVIDIA Nemo and NIMS. And we have a great partnership with Dell. Everybody who is building these chatbots and generative AI, when you're ready to run it, you're going to need an AI factory. Nobody is better at building end-to-end systems of very large scale for the enterprise than Dell. So anybody, any company, every company will need to build AI factories. And it turns out that Michael is here. He's happy to take your order Ladies and gentlemen, Michael Dell Okay, let's talk about the next wave of robotics the next wave of AI robotics physical AI So far all of the AI that we've talked about is one computer Data comes into one computer, lots of the world's, if you will, experience in digital text form. The AI imitates us by reading a lot of the language to predict the next words. It's imitating you by studying all of the patterns and all the other previous examples. Of course, it has to understand context and so on and so forth. But once it understands the context, it's essentially imitating you. We take all of the data, we put it into a system like DGX, we compress it into a large language model, trillions and trillions of parameters become billions and billions, trillions of tokens become billions of parameters, these billions of parameters become your AI. Well, in order for us to go to the next wave of AI, where the AI understands the physical world, we're going to need three computers. The first computer is still the same computer. It's that AI computer that now is going to be watching video, and maybe it's doing synthetic data generation, and maybe there's a lot of human examples. Just as we have human examples in text form, we're going to have human examples in articulation form, and the AIs will watch us, understand what is happening, and try to adapt it for themselves into the context. And because it can generalize with these foundation models, maybe these robots can also perform in the physical world fairly generally. So I just described in very simple terms, essentially what just happened in large language models, except the chat GPT moment for robotics may be right around the corner. And so we've been building the end-to-end systems for robotics for some time. I'm super, super proud of the work. We have the AI system, DGX. We have the lower system which is called AGX for autonomous systems. The world's first robotics processor. When we first built this thing, people were like, what are you guys building? It's an SOC, it's one chip, it's designed to be very low power, but it's designed for high speed sensor processing and AI. And so if you want to run transformers in a car or you want to run transformers in anything that moves, we have the perfect computer for you. It's called the Jetson. And so the DGX on top for training the AI, the Jetson is the autonomous processor. And in the middle, we need another computer. Whereas large language models have the benefit of you providing your examples and then doing reinforcement learning human feedback. What is the reinforcement learning human feedback of a robot? Well, it's reinforcement learning physical feedback. That's how you align the robot. That's how the robot knows that as it's learning these articulation capabilities and manipulation capabilities, it's going to adapt properly into the laws of physics. And so we need a simulation engine. that represents the world digitally for the robot, so that the robot has a gym to go learn how to be a robot. We call that virtual world Omniverse. The computer that runs Omniverse is called OVX. OVX, the computer itself is hosted in the Azure Cloud. So basically we built these three things, these three systems. On top of it, we have algorithms for every single one. Now, I'm going to show you one super example of how AI and Omniverse are going to work together. The example I'm going to show you is kind of insane, but it's going to be very, very close to tomorrow. It's a robotics building. This robotics building is called a warehouse. Inside the robotics building are going to be some autonomous systems. Some of the autonomous systems are going to be called humans. And some of the autonomous systems are going to be called forklifts. And these autonomous systems are going to interact with each other, of course, autonomously. And it's going to be overlooked upon by this warehouse to keep everybody out of harm's way. The warehouse is essentially an air traffic controller. And whenever it sees something happening, it will redirect traffic and give new waypoints, just new waypoints to the robots and the people. And they'll know exactly what to do. this warehouse, this building, you can also talk to. Of course you could talk to it. Hey, you know, SAP Center, how are you feeling today? For example. And so you could ask the same, the warehouse the same questions. Basically, the system I just described will have Omniverse Cloud that's hosting. the virtual simulation, and AI running on DGX Cloud, and all of this is running in real time. Let's take a look. The future of heavy industries starts as a digital twin. The AI agents helping robots, workers, and infrastructure navigate unpredictable events in complex industrial spaces will be built and evaluated first in sophisticated digital twins. This omniverse digital twin of a 100,000 square foot warehouse is operating as a simulation environment that integrates digital workers, AMRs running the NVIDIA ISAAC receptor stack, centralized activity maps of the entire warehouse from 100 simulated ceiling mount cameras using NVIDIA Metropolis, and AMR route planning with NVIDIA Co-op. Software-in-loop testing of AI agents in this physically accurate simulated environment enables us to evaluate and refine how the system adapts to real-world unpredictability. Here, an incident occurs along this AMR's planned route, blocking its path as it moves to pick up a pallet. NVIDIA Metropolis updates and sends a real-time occupancy map to CoOpt where a new optimal route is calculated. The AMR is enabled to see around corners and improve its mission efficiency. With generative AI-powered Metropolis Vision Foundation models, operators can even ask questions using natural language. The visual model understands nuanced activity and can offer immediate insights to improve operations. All of the sensor data is created in simulation and passed to the real-time AI, running as NVIDIA Inference Microservices, or NEMS. And when the AI is ready to be deployed in the physical twin, the real warehouse, We connect Metropolis and Isaac Nims to real sensors with the ability for continuous improvement of both the digital twin and the AI models. Isn't that incredible? And so... Remember, a future facility, warehouse, factory, building, will be software defined. And so the software is running. How else would you test the software? So you test the software to building the warehouse, the optimization system in the digital twin. What about all the robots? All of those robots you were seeing just now, they're all running their own autonomous robotic stack. And so the way you integrate software in the future, CICD in the future, for robotic systems is with digital twins. We've made Omniverse a lot easier to access. We're gonna create basically Omniverse cloud APIs, four simple API in a channel, and you can connect your application to it. So this is going to be as wonderfully, beautifully simple in the future that Omniverse is going to be. And with these APIs, you're gonna have these magical digital twin capability. We also have turned Omniverse into an AI. and integrated it with the ability to chat USD the the language of our languages you know human and omniverse is language as it turns out is universal scene description and so that language is rather complex and so we've taught our omniverse that language and so you can speak to it in English and it would directly generate USD and it would talk back in USD but converse back to you in English you could also look for information in this world semantically Instead of the world being encoded semantically in language, now it's encoded semantically in scenes. And so you can ask it of certain objects or certain conditions or certain scenarios, and it can go and find that scenario for you. It also can collaborate with you in generation. You could design some things in 3D. It could simulate some things in 3D, or you could use AI to generate something in 3D. Let's take a look at how this is all going to work. We have a great partnership with Siemens. Siemens is the world's largest industrial engineering and operations platform. You've seen now so many different companies in the industrial space. Heavy Industries is one of the greatest final frontiers of IT. And we finally now have the necessary technology to go and make a real impact. Siemens is building the industrial metaverse. And today we're announcing that Siemens is connecting their crown jewel accelerator to NVIDIA Omniverse. Let's take a look. Siemens technology is transformed every day for everyone. Teamcenter X, our leading product lifecycle management software from the Siemens Accelerator platform, is used every day by our customers to develop and deliver products at scale. Now we are bringing the real and the digital worlds even closer by integrating NVIDIA AI and Omniverse technologies into Teamcenter X. Omnibus APIs enable data interoperability and physics-based rendering to industrial-scale design and manufacturing projects. Our customers, HD&A, market leader in sustainable ship manufacturing, builds ammonia and hydrogen-powered ships, often comprising over 7 million discrete parts. Omniverse APIs. Teamcenter X lets companies like HD Hyundai unify and visualize these massive engineering data sets interactively and integrate generative AI to generate 3D objects or HDRI backgrounds to see their projects in context. The result? An ultra-intuitive, photoreal, physics-based digital twin that eliminates waste and errors, delivering huge savings in cost and time. And we are building this for collaboration, whether across more Siemens Accelerator tools like Siemens NX or Star CCM Plus, or across teams working on their favorite devices in the same scene together. This is just the beginning. Working with NVIDIA, we will bring Accelerator Computing, Generative AI, and Omniverse integration across the Siemens Accelerator portfolio. The professional voice actor happens to be a good friend of mine, Roland Bush, who happens to be the CEO of Siemens. Once you get Omniverse connected into your workflow, your ecosystem, from the beginning of your design to engineering, to manufacturing planning, all the way to digital twin operations. Once you connect everything together, it's insane how much productivity you can get. And it's just really, really wonderful. All of a sudden, everybody's operating on the same ground truth. You don't have to exchange data and convert data, make mistakes. Everybody is working on the same ground truth. From the design department to the art department, the architecture department, all the way to the engineering and even the marketing department. Let's take a look at how Nissan has integrated Omniverse into their workflow. And it's all because it's connected by all these wonderful tools and these developers that we're working with. Take a look. I'm going to go ahead and start. I'm going to go ahead and start. I'm going to go ahead and start. I'm going to go ahead and start. This is a question that I have for you. This is a question that I have for you. This is a question that I have for you. This is a question that I have for you. That was not an animation. That was Omniverse. Today we're announcing that Omniverse Cloud streams to the Vision Pro. And it is very, very strange that you walk around virtual doors when I was getting out of that car. And everybody does it. It is really, really quite amazing. Vision Pro connected to Omniverse. portals you into Omniverse. And because all of these CAD tools and all these different design tools are now integrated and connected to Omniverse, you can have this type of workflow. Really incredible. Let's talk about robotics. everything that moves will be robotic. There's no question about that. It's safer, it's more convenient. And one of the largest industries is going to be automotive. We build the robotic stack from top to bottom, as I was mentioned, from the computer system, but in the case of self-driving cars, including the self-driving application. At the end of this year, or I guess beginning of next year, we will be shipping in Mercedes, and then shortly after that, JLR. And so these autonomous robotic systems are software defined. They take a lot of work to do, has computer vision, has obviously artificial intelligence, control and planning, all kinds of very complicated technology and takes years to refine. We're building the entire stack. However, we open up our entire stack for all of the automotive industry. This is just the way we work. The way we work in every single industry, we try to build as much of it as we can so that we understand it, but then we open it up so that everybody can access it. Whether you would like to buy just our computer, which is the world's only full, functional, safe, ASIL-D system that can run AI. This functional, safe, ASLD quality computer, or the operating system on top, or of course our data centers, which is in basically every AV company in the world, however you would like to enjoy it, we're delighted by it. Today, we're announcing that BYD, the world's largest EV company, is adopting our next generation. It's called Thor. Thor is designed for transformer engines. Thor, our next generation AV computer, will be used by BYD. You probably don't know this fact that we have over a million robotics developers. We created Jetson, this robotics computer. We're so proud of it. The amount of software that goes on top of it is insane. But the reason why we can do it at all is because it's 100% CUDA compatible. Everything that we do, everything that we do in our company is in service of our developers. And by us being able to maintain this rich ecosystem and make it compatible with everything that you access from us, we can bring all of that incredible capability to this little tiny computer. We call Jetson a robotics computer. We also today are announcing this incredibly advanced new SDK. We call it Isaac Perceptor. Isaac Perceptor, most of the robots today are pre-programmed. They're either following rails on the ground, digital rails, or they'd be following April tags. But in the future, they're going to have perception. And the reason why you want that is so that you could easily program it. You say, I would like to go from point A to point B, and it will figure out a way to navigate its way there. So by only programming waypoints, the entire route could be adaptive. The entire environment could be reprogrammed, just as I showed you at the very beginning with the warehouse. You can't do that with pre-programmed AGVs. If those boxes fall down, they just all gum up and they just wait there for somebody to come clear it. So now with the Isaac Perceptor, We have incredible state-of-the-art vision odometry, 3D reconstruction, and in addition to 3D reconstruction, depth perception. The reason for that is so that you can have two modalities to keep an eye on what's happening in the world. Isaac Perceptor. The most used robot today is the manipulator, manufacturing arms, and they are also pre-programmed. The computer vision algorithms, the AI algorithms, the control and path planning algorithms that are geometry aware, incredibly computationally intensive. We have made these CUDA accelerated. So we have the world's first CUDA accelerated motion planner that is geometry aware. You put something in front of it, it comes up with a new plan and articulates around it. It has excellent perception for pose estimation of a 3D object. Not just, not its pose in 2D, but its pose in 3D. So it has to imagine what's around and how best to grab it. So the foundation pose, the grip foundation, and the articulation algorithms are now available. We call it Isaac Manipulator. And they also just run on NVIDIA's computers. We are starting to do some really great work in the next generation of robotics. The next generation of robotics will likely be a humanoid robotics. We now have the necessary technology, and as I was describing earlier, the necessary technology to imagine generalized human robotics. In a way, human robotics is likely easier, and the reason for that is because we have a lot more... imitation training data that we can provide the robots because we are constructed in a very similar way. It is very likely that the humanoid robotics will be much more useful in our world because we created the world to be something that we can interoperate in and work well in. And the way that we set up our workstations and manufacturing and logistics, they were designed for humans. They were designed for people. And so these humanoid robotics will likely be much more productive to deploy. Well, we're creating, just like we're doing with the others, the entire stack. Starting from the top, a foundation model that learns from watching video, human examples. It could be in video form. It could be in virtual reality form. We then created a gym for it called Isaac Reinforcement Learning Gym, which allows the humanoid robot to learn how to adapt to the physical world. And then an incredible computer, the same computer that's going to go into a robotic car, this computer will run inside a humanoid robot called Thor. It's designed for transformer engines. We've combined several of these into one video. This is something that you're going to really love. Take a look. It's not enough for humans to imagine. We have to invent and explore and push beyond what's been done. We create smarter and faster. We push it to fail so it can learn. We teach it. then help it teach itself. We broaden its understanding to take on new challenges with absolute precision and succeed. We make it perceive and move and even reason. so it can share our world with us. This is where inspiration leads us, the next frontier. This is NVIDIA Project Root. A general purpose foundation model for humanoid robot learning. The group model takes multimodal instructions and past interactions as input and produces the next action for the robot to execute. We developed Isaac Lab, a robot learning application to train Groot on Omniverse Isaac Sim. And we scale out with Osmo, a new compute orchestration service that coordinates workflows across DGX systems for training and OVX systems for simulation. With these tools, we can train Groot in physically-based simulation and transfer zero-shot to the real world. The Groot model will enable a robot to learn from a handful of human demonstrations, so it can help with everyday tasks. and emulate human movement just by observing us. This is made possible with NVIDIA's technologies that can understand humans from videos, train models and simulation, and ultimately deploy them directly to physical robots. Connecting Groot to a large language model even allows it to generate motions by following natural language instructions. Hi, GL1. Here, give me a high five. Sure thing. Let's high five. Can you give us some cool moves? Sure. Check this out. All this incredible intelligence is powered by the new Jetson Thor robotic ships, designed for Groot, built for the future. With Isaac Lab, Osmo, and Groot, we're providing the building blocks for the next generation of AI-powered robotics. About the same size. The soul of NVIDIA, the intersection of computer graphics, physics, artificial intelligence. It all came to bear at this moment. The name of that project, General Robotics 003. I know, super good. Super good. Well, I think we have some special guests. Do we? Hey, guys. So I understand you guys are powered by Jetson. They're powered by Jetsons. little Jetson robotics computers inside. They learned to walk in Isaac Sim. Ladies and gentlemen, this is orange, and this is the famous green. They are the BDX robots of Disney. Amazing Disney research. Come on, you guys, let's wrap up. Let's go. Five things. Where are you going? Five things. I sit right here. Don't be afraid. Come here, Green. Hurry up. What are you saying? No, it's not time to eat. It's not time to eat. I'll give you a snack in a moment. Let me finish up real quick. Come on, Green, hurry up. Stop wasting time. Five things. Five things. First, a new industrial revolution. Every data center should be accelerated. A trillion dollars worth of installed data centers. will become modernized over the next several years. Second, because of the computational capability we brought to bear, a new way of doing software has emerged. Generative AI, which is going to create new infrastructure dedicated to doing one thing and one thing only. Not for multi-user data centers, but AI generators. These AI generation will create incredibly valuable software. A new industrial revolution. Second, the computer of this revolution... The computer of this generation, generative AI, trillion parameters, Blackwell. Insane amounts of computers and computing. Third, I'm trying to concentrate. Good job. Third. New computer creates new types of software. New type of software should be distributed in a new way so that it can, on the one hand, be an endpoint in the cloud and easy to use, but still allow you to take it with you because it is your intelligence. Your intelligence should be packaged up in a way that allows you to take it with you. We call them NIMS. And third, these NIMS are going to help you create a new type of application for the future, not one that you wrote completely from scratch, But you're going to integrate them like teams. create these applications. We have a fantastic capability between NIMS, the AI technology, the tools, NEMO, and the infrastructure, DGX Cloud, in our AI foundry to help you create proprietary applications, proprietary chatbots. And then lastly, everything that moves in the future will be robotic. You're not going to be the only one. And these robotic systems, whether they are humanoid, AMRs, self-driving cars, forklifts, manipulating arms. They will all need one thing. Giant stadiums, warehouses, factories. There can be factories that are robotic, orchestrating factories, manufacturing lines that are robotics, building cars that are robotics. These systems all need one thing. They need a platform, a digital platform, a digital twin platform, and we call that Omniverse, the operating system of the robotics world. These are the five things that we talked about today. What does NVIDIA look like? What does NVIDIA look like? When we talk about GPUs, there's a very different image that I have when people ask me about GPUs. First, I see a bunch of software stacks and things like that. And second, I see this. This is what we announced to you today. This is Blackwell. This is the platform. Amazing processors, MV-Link switches, networking systems, and the system design is a miracle. This is Blackwell. This to me is what a GPU looks like in my mind. Listen, Orange, Green, I think we have one more treat for everybody. What do you think? Should we? Okay, we have one more thing to show you. Roll it. I think that's a good thing. Thank you. Thank you! Thank you! Have a great, have a great GTC! Thank you all for coming! Thank you!
この記事が気に入ったらサポートをしてみませんか?